Apollo | common | math | kd-tree
0.引言
Apollo中的KD-Tree实现代码.
KD-Tree参数:
struct AABoxKDTreeParams {
int max_depth = -1;
int max_leaf_size = -1;
double max_leaf_dimension = -1.0;
};
对外接口,模板类:
template <class ObjectType>
class AABoxKDTree2d {
public:
using ObjectPtr = const ObjectType *;
AABoxKDTree2d(const std::vector<ObjectType> &objects,
const AABoxKDTreeParams ¶ms) {
if (!objects.empty()) {
std::vector<ObjectPtr> object_ptrs;
for (const auto &object : objects) {
object_ptrs.push_back(&object);
}
root_.reset(new AABoxKDTree2dNode<ObjectType>(object_ptrs, params, 0));
}
}
ObjectPtr GetNearestObject(const Vec2d &point) const {
return root_ == nullptr ? nullptr : root_->GetNearestObject(point);
}
std::vector<ObjectPtr> GetObjects(const Vec2d &point,
const double distance) const {
if (root_ == nullptr) {
return {};
}
return root_->GetObjects(point, distance);
}
AABox2d GetBoundingBox() const {
return root_ == nullptr ? AABox2d() : root_->GetBoundingBox();
}
private:
std::unique_ptr<AABoxKDTree2dNode<ObjectType>> root_ = nullptr;
};
1.建树过程
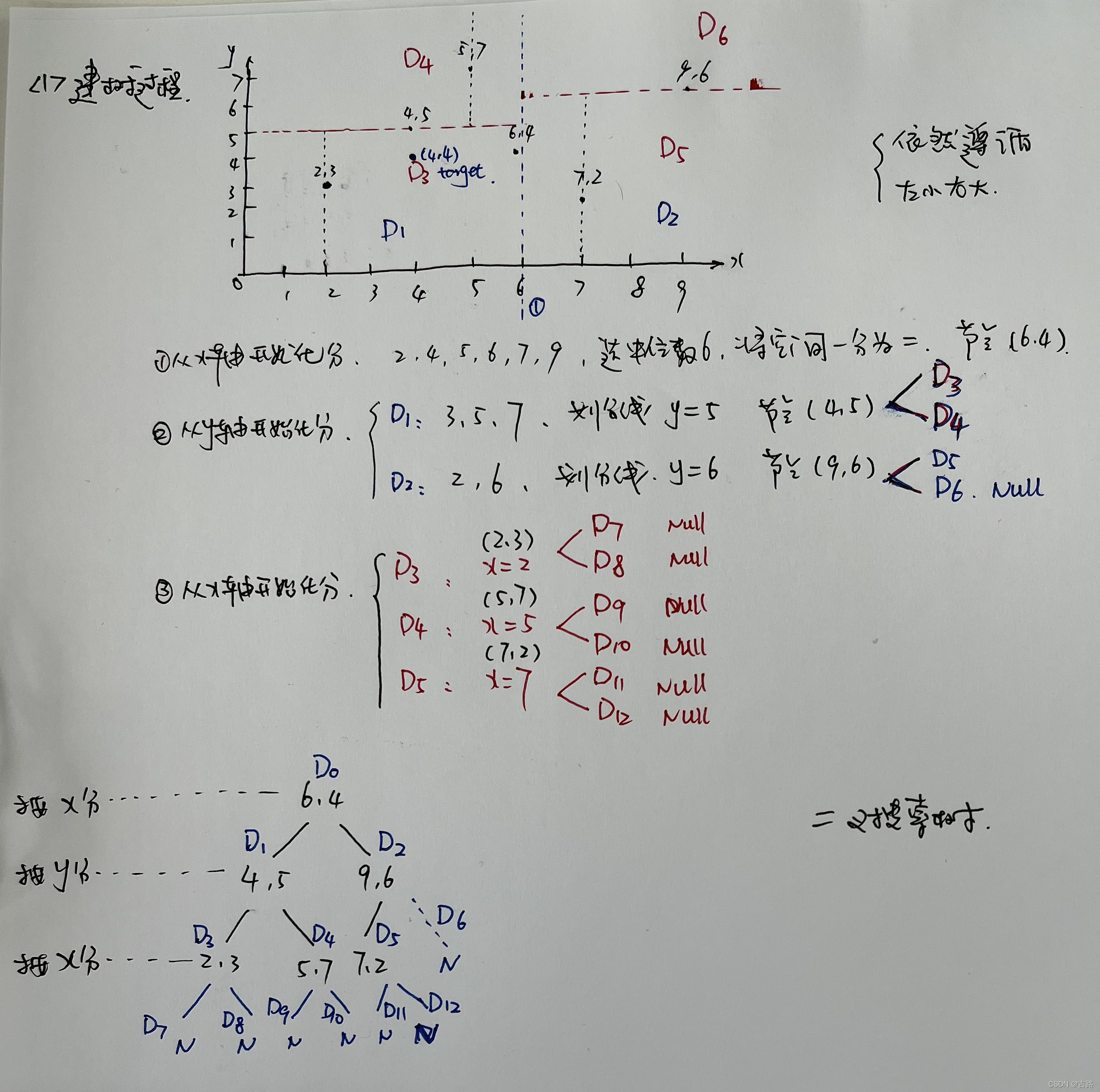
AABoxKDTree2dNode(const std::vector<ObjectPtr> &objects,
const AABoxKDTreeParams ¶ms, int depth)
: depth_(depth) {
CHECK(!objects.empty());
ComputeBoundary(objects);
ComputePartition();
if (SplitToSubNodes(objects, params)) {
std::vector<ObjectPtr> left_subnode_objects;
std::vector<ObjectPtr> right_subnode_objects;
PartitionObjects(objects, &left_subnode_objects, &right_subnode_objects);
if (!left_subnode_objects.empty()) {
left_subnode_.reset(new AABoxKDTree2dNode<ObjectType>(
left_subnode_objects, params, depth + 1));
}
if (!right_subnode_objects.empty()) {
right_subnode_.reset(new AABoxKDTree2dNode<ObjectType>(
right_subnode_objects, params, depth + 1));
}
} else {
InitObjects(objects);
}
}
void ComputeBoundary(const std::vector<ObjectPtr> &objects) {
min_x_ = std::numeric_limits<double>::infinity();
min_y_ = std::numeric_limits<double>::infinity();
max_x_ = -std::numeric_limits<double>::infinity();
max_y_ = -std::numeric_limits<double>::infinity();
for (ObjectPtr object : objects) {
min_x_ = std::fmin(min_x_, object->aabox().min_x());
max_x_ = std::fmax(max_x_, object->aabox().max_x());
min_y_ = std::fmin(min_y_, object->aabox().min_y());
max_y_ = std::fmax(max_y_, object->aabox().max_y());
}
mid_x_ = (min_x_ + max_x_) / 2.0;
mid_y_ = (min_y_ + max_y_) / 2.0;
CHECK(!std::isinf(max_x_) && !std::isinf(max_y_) && !std::isinf(min_x_) &&
!std::isinf(min_y_))
<< "the provided object box size is infinity";
}
- step 2 计算按哪个方向划分以及划分的点(作为当前节点)
KD-Tree划分计算:长和宽哪个更大就按照哪个方向进行划分,划分的点也比较粗暴,直接采用中点进行划分,因此这里和笔记中的选择划分点有区别,笔记中或一般 kd-tree 的节点(划分点)选取依然是输入数据中的点,而这里不是,这里直接以物理空间进行“均分”。
void ComputePartition() {
if (max_x_ - min_x_ >= max_y_ - min_y_) {
partition_ = PARTITION_X;
partition_position_ = (min_x_ + max_x_) / 2.0;
} else {
partition_ = PARTITION_Y;
partition_position_ = (min_y_ + max_y_) / 2.0;
}
}
- step 3 分裂当前空间(Node)为左右子空间(左右子树)
在 step 3.1中构建了当前节点的数据,于是看可以看出建树的过程是一个前序遍历建树的过程。
if (SplitToSubNodes(objects, params)) {
std::vector<ObjectPtr> left_subnode_objects;
std::vector<ObjectPtr> right_subnode_objects;
PartitionObjects(objects, &left_subnode_objects, &right_subnode_objects);
if (!left_subnode_objects.empty()) {
left_subnode_.reset(new AABoxKDTree2dNode<ObjectType>(
left_subnode_objects, params, depth + 1));
}
if (!right_subnode_objects.empty()) {
right_subnode_.reset(new AABoxKDTree2dNode<ObjectType>(
right_subnode_objects, params, depth + 1));
}
}
首先检测是否需要继续分裂:
bool SplitToSubNodes(const std::vector<ObjectPtr> &objects,
const AABoxKDTreeParams ¶ms) {
if (params.max_depth >= 0 && depth_ >= params.max_depth) {
return false;
}
if (static_cast<int>(objects.size()) <= std::max(1, params.max_leaf_size)) {
return false;
}
if (params.max_leaf_dimension >= 0.0 &&
std::max(max_x_ - min_x_, max_y_ - min_y_) <=
params.max_leaf_dimension) {
return false;
}
return true;
}
开始划分左右子空间:
void PartitionObjects(const std::vector<ObjectPtr> &objects,
std::vector<ObjectPtr> *const left_subnode_objects,
std::vector<ObjectPtr> *const right_subnode_objects) {
left_subnode_objects->clear();
right_subnode_objects->clear();
std::vector<ObjectPtr> other_objects;
if (partition_ == PARTITION_X) {
for (ObjectPtr object : objects) {
if (object->aabox().max_x() <= partition_position_) {
left_subnode_objects->push_back(object);
} else if (object->aabox().min_x() >= partition_position_) {
right_subnode_objects->push_back(object);
} else {
other_objects.push_back(object);
}
}
} else {
for (ObjectPtr object : objects) {
if (object->aabox().max_y() <= partition_position_) {
left_subnode_objects->push_back(object);
} else if (object->aabox().min_y() >= partition_position_) {
right_subnode_objects->push_back(object);
} else {
other_objects.push_back(object);
}
}
}
InitObjects(other_objects);
}
void InitObjects(const std::vector<ObjectPtr> &objects) {
num_objects_ = static_cast<int>(objects.size());
objects_sorted_by_min_ = objects;
objects_sorted_by_max_ = objects;
std::sort(objects_sorted_by_min_.begin(), objects_sorted_by_min_.end(),
[&](ObjectPtr obj1, ObjectPtr obj2) {
return partition_ == PARTITION_X
? obj1->aabox().min_x() < obj2->aabox().min_x()
: obj1->aabox().min_y() < obj2->aabox().min_y();
});
std::sort(objects_sorted_by_max_.begin(), objects_sorted_by_max_.end(),
[&](ObjectPtr obj1, ObjectPtr obj2) {
return partition_ == PARTITION_X
? obj1->aabox().max_x() > obj2->aabox().max_x()
: obj1->aabox().max_y() > obj2->aabox().max_y();
});
objects_sorted_by_min_bound_.reserve(
num_objects_);
for (ObjectPtr object : objects_sorted_by_min_) {
objects_sorted_by_min_bound_.push_back(partition_ == PARTITION_X
? object->aabox().min_x()
: object->aabox().min_y());
}
objects_sorted_by_max_bound_.reserve(num_objects_);
for (ObjectPtr object : objects_sorted_by_max_) {
objects_sorted_by_max_bound_.push_back(partition_ == PARTITION_X
? object->aabox().max_x()
: object->aabox().max_y());
}
}
2.查询
构建树的目的就是降低查找的时间复杂度。可以查找最近邻,也可以查找k近邻。
(1)最近邻查找
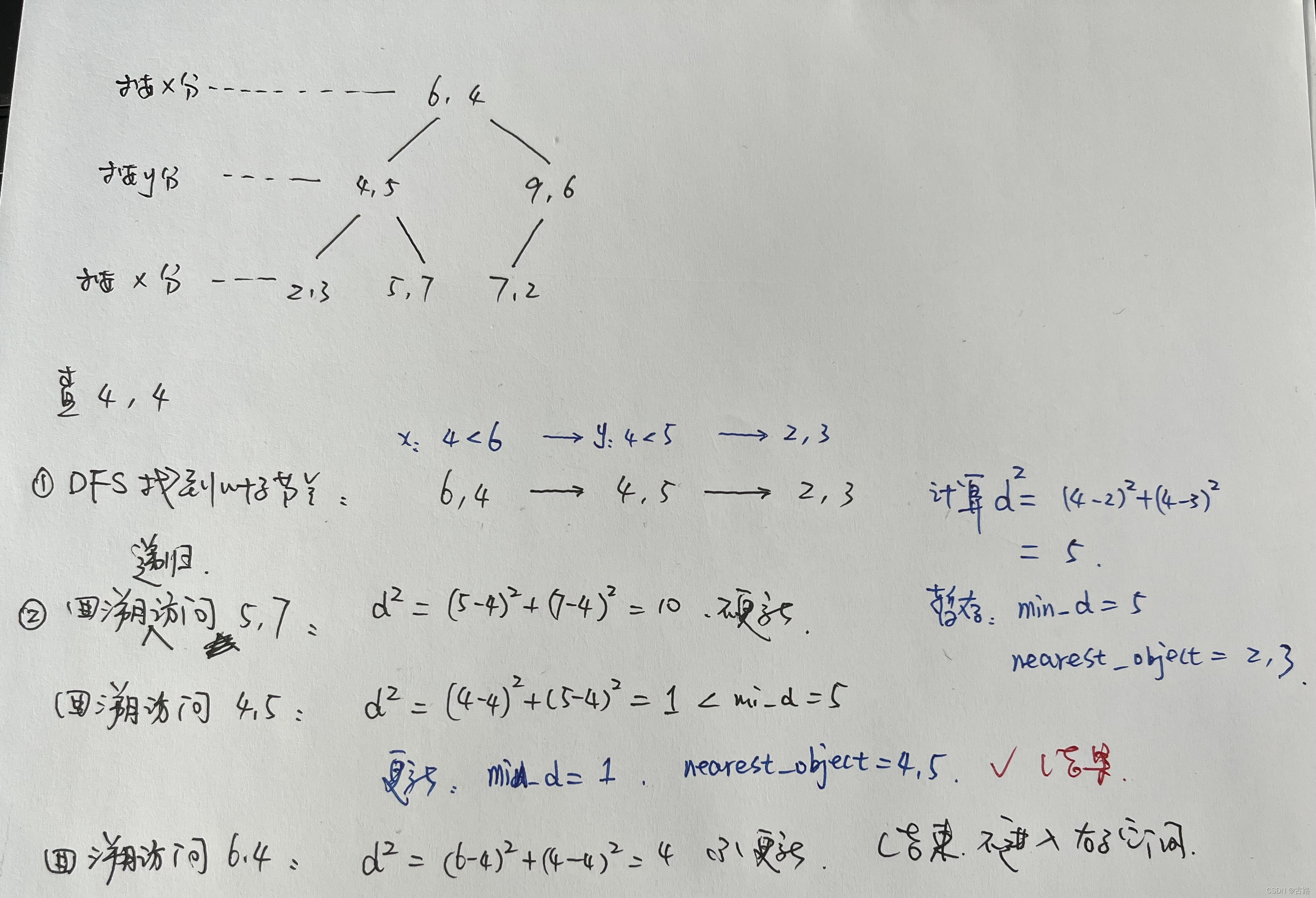
-
1.首先要找到该目标点的叶子节点进行判断,记录最近距离与nearest_object; -
2.回溯判断另一半空间是否需要计算,若需要在另一个子树继续搜索最近邻,更新记录值。 -
3.当回溯到根节点时,算法结束,此时保存的最近邻节点就是最终的最近邻。 -
参考阅读 详细看查询距离。
void GetNearestObjectInternal(
const Vec2d &point,
double *const min_distance_sqr,
ObjectPtr *const nearest_object) const {
if (LowerDistanceSquareToPoint(point) >= *min_distance_sqr - kMathEpsilon) {
return;
}
const double pvalue = (partition_ == PARTITION_X ? point.x() : point.y());
const bool search_left_first = (pvalue < partition_position_);
if (search_left_first) {
if (left_subnode_ != nullptr) {
left_subnode_->GetNearestObjectInternal(point, min_distance_sqr,
nearest_object);
}
} else {
if (right_subnode_ != nullptr) {
right_subnode_->GetNearestObjectInternal(point, min_distance_sqr,
nearest_object);
}
}
if (*min_distance_sqr <= kMathEpsilon) {
return;
}
if (search_left_first) {
for (int i = 0; i < num_objects_; ++i) {
const double bound = objects_sorted_by_min_bound_[i];
if (bound > pvalue && Square(bound - pvalue) > *min_distance_sqr) {
break;
}
ObjectPtr object = objects_sorted_by_min_[i];
const double distance_sqr = object->DistanceSquareTo(point);
if (distance_sqr < *min_distance_sqr) {
*min_distance_sqr = distance_sqr;
*nearest_object = object;
}
}
} else {
for (int i = 0; i < num_objects_; ++i) {
const double bound = objects_sorted_by_max_bound_[i];
if (bound < pvalue && Square(bound - pvalue) > *min_distance_sqr) {
break;
}
ObjectPtr object = objects_sorted_by_max_[i];
const double distance_sqr = object->DistanceSquareTo(point);
if (distance_sqr < *min_distance_sqr) {
*min_distance_sqr = distance_sqr;
*nearest_object = object;
}
}
}
if (*min_distance_sqr <= kMathEpsilon) {
return;
}
if (search_left_first) {
if (right_subnode_ != nullptr) {
right_subnode_->GetNearestObjectInternal(point, min_distance_sqr,
nearest_object);
}
} else {
if (left_subnode_ != nullptr) {
left_subnode_->GetNearestObjectInternal(point, min_distance_sqr,
nearest_object);
}
}
}
按距离查找原理类似。
|