1.创建虚拟环境
硬件及系统:RTX3070 + Ubuntu20.04 3070只能用cuda11+的版本进行加速,官方教程中的配置都是cuda10。选择使用pytorch1.8.1及cuda11.1。 想使用GPU加速则必须安装mmcv-full库,该库与mmcv不兼容(不要用pip install mmcv)。 mmcv-full版本可以到https://download.openmmlab.com/mmcv/dist/内查找确认,我使用了1.3.8版本。
conda create -n lab python=3.8 -y
conda activate lab
pip3 install torch==1.8.1+cu111 torchvision==0.9.1+cu111 torchaudio==0.8.1 -f https://download.pytorch.org/whl/lts/1.8/torch_lts.html
pip install mmcv-full==1.3.8 -f https://download.openmmlab.com/mmcv/dist/cu111/torch1.8.0/index.html
2.克隆项目mmsegmentation
git clone https://github.com/open-mmlab/mmsegmentation.git
cd mmsegmentation
3.其他配置
数据集: 推荐使用软链接方式,也可以更改数据集配置文件内的路径。我使用的是ADE20K数据集、软链接方式,链接前注意mmseg对数据集形式的要求。数据集的配置文件路径在configs/base/datasets下,更改对应数据集的data_root项即可替换数据集路径。 软链接方式如下:
pip install -r requirements.txt
mkdir data
ln -s $你自己的数据集路径 data
4.训练前准备
我使用的网络模型为swin-T(3070显存只有8G,其他的也比较难运行起来),模型基础配置都存储在configs/base/models下,打开upernet_swin.py。 首先mmseg默认多GPU并行训练,修改upernet_swin.py第一行配置将其更改为单卡训练:
norm_cfg = dict(type='BN', requires_grad=True)
5.开始训练
python tools/train.py configs/swin/upernet_swin_tiny_patch4_window7_512x512_160k_ade20k_pretrain_224x224_1K.py
6.其他可修改的参数
batch_size batch_size设置在数据集配置文件中,如configs/base/datasets/ade20k中,参数samples_per_gpu即为batch_size。 线程数 同上文件,参数workers_per_gpu为线程数。 迭代次数 configs/base/schedules/下,有20k、40k、80k、160k四种策略,训练迭代次数为runner的max_iters,checkpoint_config的interval表示每多少次训练后保存一次checkpoint,evaluation的interval表示每多少次训练后进行一次验证
7.可能报错的原因
1)Cudnn报错:cuda与mmcv版本或cuda与pytorch版本不匹配,参考第一步的配置步骤重新配置环境; 2)cuda out of meomery:如果没能开始训练,建议调整第六步中的sampler_per_gpu与workers_per_gpu,我都设置成了2(不一定是最优,因为写博客的时候还在训练,没有测试过);如果能够正常训练,在evaluation时报错,是因为验证时读取的图像多,3070每次验证1500张图像就会超出显存,我选择删除一部分验证集图像,最终只保留了1000张(ADE20K标准配置是2000张验证集)进行验证。
现在还在训练中(60900/160000),后续有问题还会更新,如果您遇到了问题,欢迎评论区提出一起交流。 训练已结束。
8.训练结果
aAcc:76.36 mIoU:33.16 mAcc:46.57 绘制训练时指标变化曲线:
python tools/analyze_logs.py work_dirs/upernet_swin_tiny_patch4_window7_512x512_160k_ade20k_pretrain_224x224_1K/20210713_093422.log.json --keys mIoU mAcc aAcc --legend mIoU mAcc aAcc
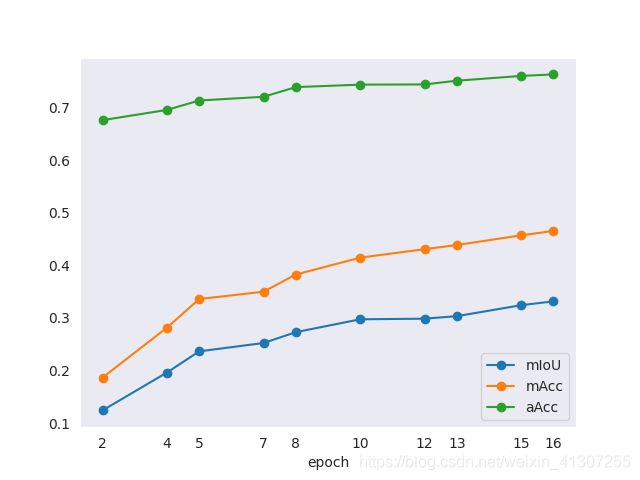
而在http://sceneparsing.csail.mit.edu/中可以查到的指标参数为: aAcc:78.42 mIoU:47.07 测试图例: 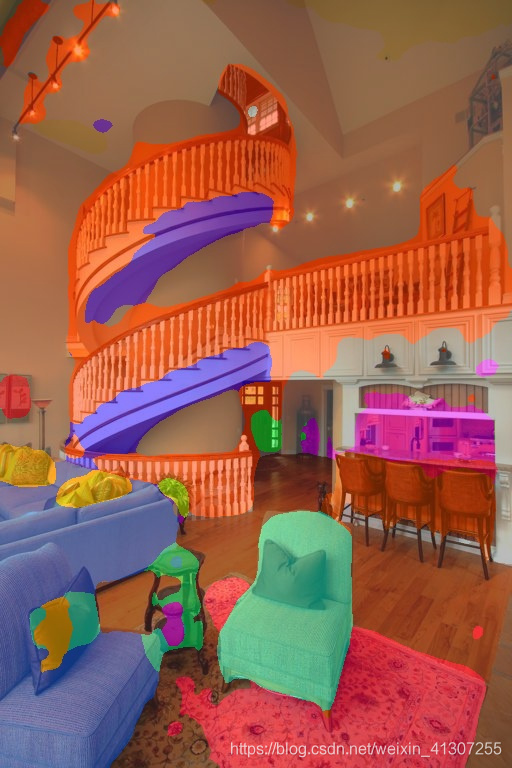 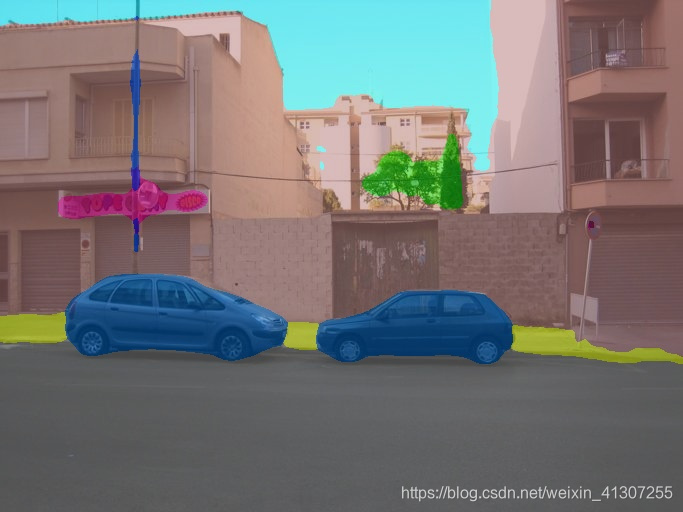 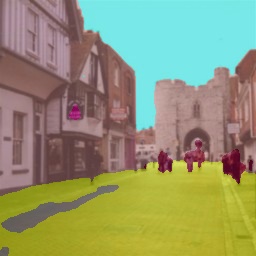 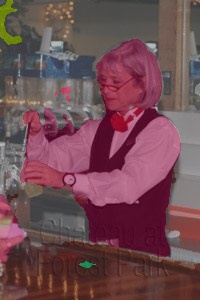
|