Matplot pyplot绘制单图,多子图不同样式详解,这一篇就够了
matplotlib.pyplot 是使 matplotlib 像 MATLAB 一样工作的函数集合。这篇博客将介绍
- 单图单线
- 单图多线不同样式(红色圆圈、蓝色实线、绿色三角等)
- 使用关键字字符串绘图(data 可指定依赖值为:numpy.recarray 或 pandas.DataFrame)
- 使用分类变量绘图(绘制条形图、散点图、折线图)
- 多子表多轴及共享轴
- 多子表(水平堆叠、垂直堆叠、水平垂直堆叠、水平垂直堆叠共享轴、水平垂直堆叠去掉子图中间的冗余xy标签及间隙、极坐标风格轴示例);
-
plt.set_title(‘simple plot’, loc = ‘left’) 设置表示标题,默认位于中间,也可位于left靠左,right靠右 -
t = plt.xlabel(‘Smarts’, fontsize=14, color=‘red’) 表示设置x标签的文本,字体大小,颜色 -
plt.plot([1, 2, 3, 4]) 如果提供的单个列表或数组,matplot假定提供的是y的值;x默认跟y个数一致,但从0开始,表示x:[0,1,2,3] -
plt.plot([1, 2, 3, 4], [1, 4, 9, 16]) 表示x:[1,2,3,4],y:[1,4,9,16] -
plt.plot([1, 2, 3, 4], [1, 4, 9, 16], ‘ro’) 表示x:[1,2,3,4],y:[1,4,9,16],然后指定线的颜色和样式(ro:红色圆圈,b-:蓝色实线等) -
plt.axis([0, 6, 0, 20]) plt.axix[xmin, xmax, ymin, ymax] 表示x轴的刻度范围[0,6], y轴的刻度范围[0,20] -
plt.bar(names, values) # 条形图 -
plt.scatter(names, values) # 散点图 -
plt.plot(names, values) # 折线图 -
fig, ax = plt.subplots(2, sharex=True, sharey=True) 可创建多表,并指定共享x,y轴,然后ax[0],ax[1]绘制data -
fig, (ax1, ax2) = plt.subplots(2, sharex=True, sharey=True) 可创建多表,并指定共享x,y轴 -
fig, (ax1, ax2) = plt.subplots(2, sharex=True, sharey=True) 可创建多表,垂直堆叠表 2行1列 -
fig, (ax1, ax2) = plt.subplots(1, 2, sharex=True, sharey=True) 可创建多表,水平堆叠表 1行2列 -
fig, (ax1, ax2) = plt.subplots(2, 2, sharex=True, sharey=True,hspace=0,wspace=0) 可创建多表,水平垂直堆叠表 2行2列,共享x,y轴,并去除子图宽度和高度之间的缝隙
共享x,y轴表示共享x,y轴的刻度及值域;
- plt.text(60, .025, r’
μ
=
100
,
?
σ
=
15
\mu=100,\ \sigma=15
μ=100,?σ=15’)
表示在60,0.025处进行文本(
μ
=
100
,
?
σ
=
15
\mu=100,\ \sigma=15
μ=100,?σ=15)的标注
1. 单图单线
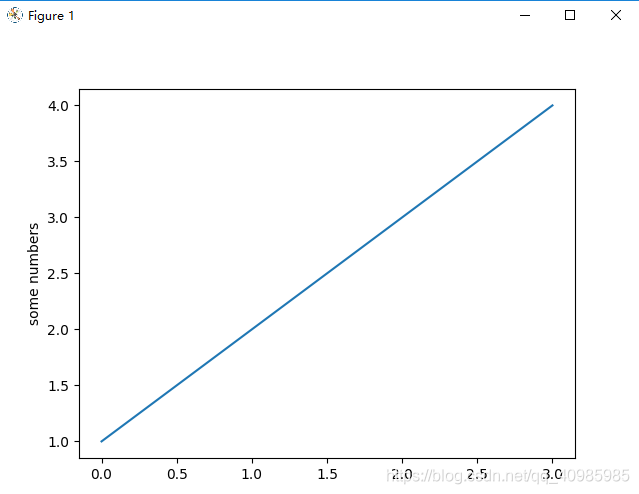 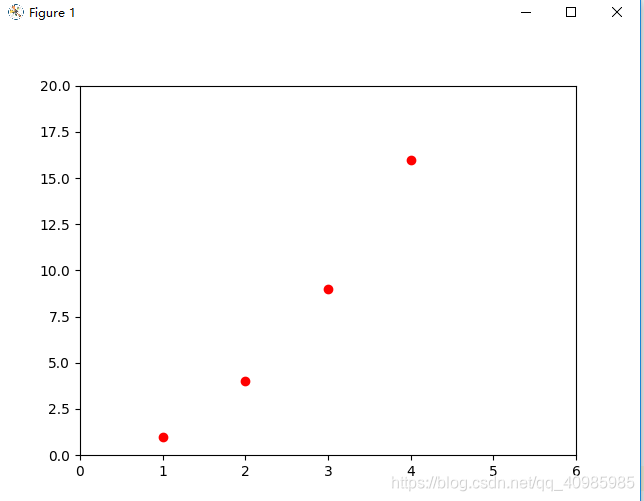
import matplotlib.pyplot as plt
plt.plot([1, 2, 3, 4])
plt.ylabel('some numbers')
plt.show()
plt.plot([1, 2, 3, 4], [1, 4, 9, 16])
plt.show()
plt.plot([1, 2, 3, 4], [1, 4, 9, 16], 'ro')
plt.axis([0, 6, 0, 20])
plt.show()
2. 单图多线不同样式(红色圆圈、蓝色实线、绿色三角等)
- ’b-‘:蓝色实线
- ‘ro’:红色圆圈
- ‘r–’:红色破折号
- ‘bs’:蓝色方形
- ‘g^’:绿色三角
线的颜色和样式可参考
mark标记:
‘.’ point marker ‘,’ pixel marker ‘o’ circle marker ‘v’ triangle_down marker ‘^’ triangle_up marker ‘<’ triangle_left marker ‘>’ triangle_right marker ‘1’ tri_down marker ‘2’ tri_up marker ‘3’ tri_left marker ‘4’ tri_right marker ‘8’ octagon marker ‘s’ square marker ‘p’ pentagon marker ‘P’ plus (filled) marker ‘*’ star marker ‘h’ hexagon1 marker ‘H’ hexagon2 marker ‘+’ plus marker ‘x’ x marker ‘X’ x (filled) marker ‘D’ diamond marker ‘d’ thin_diamond marker ‘|’ vline marker ‘_’ hline marker
‘b’ blue 蓝色 ‘g’ green 绿色 ‘r’ red 红色 ‘c’ cyan 兰青色 ‘m’ magenta 紫色 ‘y’ yellow 黄色 ‘k’ black 黑色 ‘w’ white 白色
‘-’ solid line style 实线 ‘–’ dashed line style 虚线 ‘-.’ dash-dot line style 点划线 ‘:’ dotted line style 虚线
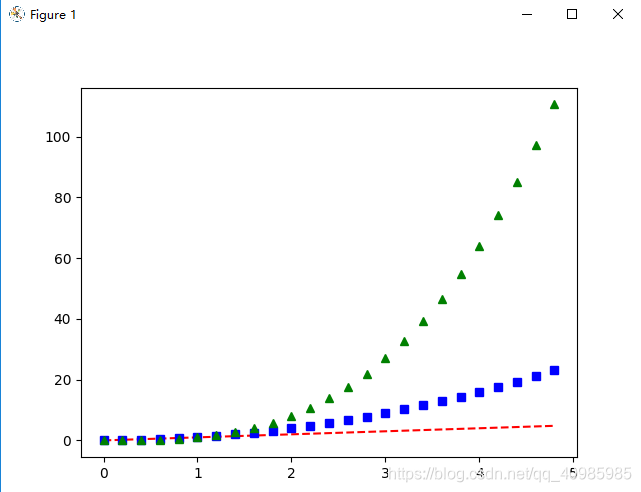
import matplotlib.pyplot as plt
import numpy as np
t = np.arange(0., 5., 0.2)
plt.plot(t, t, 'r--', t, t ** 2, 'bs', t, t ** 3, 'g^')
plt.show()
3. 使用关键字字符串绘图(data 可指定依赖值为:numpy.recarray 或 pandas.DataFrame)
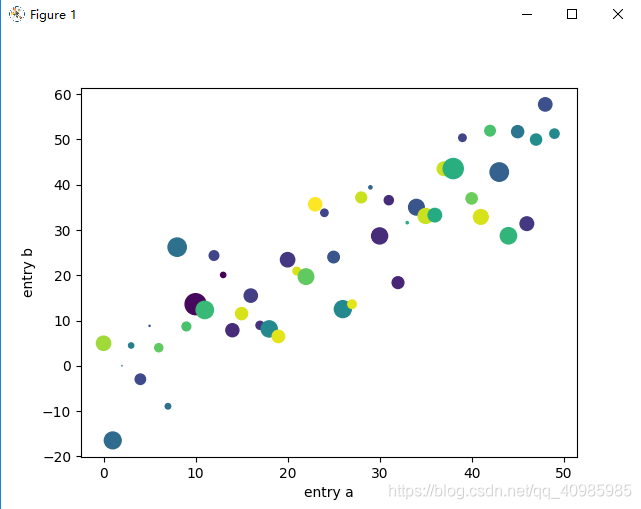
import matplotlib.pyplot as plt
import numpy as np
data = {'a': np.arange(50),
'c': np.random.randint(0, 50, 50),
'd': np.random.randn(50)}
data['b'] = data['a'] + 10 * np.random.randn(50)
data['d'] = np.abs(data['d']) * 100
print(np.arange(50))
print(np.random.randint(0, 50, 50))
print(np.random.randn(50))
print(data['b'])
print(data['d'])
plt.scatter('a', 'b', c='c', s='d', data=data)
plt.xlabel('entry a')
plt.ylabel('entry b')
plt.show()
4. 使用分类变量绘图(绘制条形图、散点图、折线图)
- plt.bar(names, values) # 条形图
- plt.scatter(names, values) # 散点图
- plt.plot(names, values) # 折线图
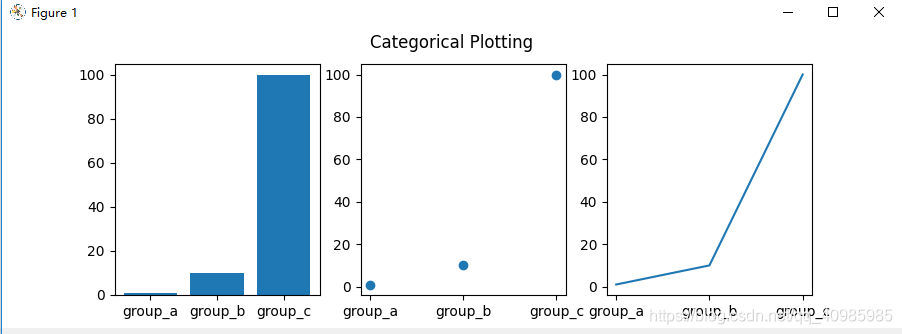
import matplotlib.pyplot as plt
import numpy as np
names = ['group_a', 'group_b', 'group_c']
values = [1, 10, 100]
plt.figure(figsize=(9, 3))
plt.subplot(131)
plt.bar(names, values)
plt.subplot(132)
plt.scatter(names, values)
plt.subplot(133)
plt.plot(names, values)
plt.suptitle('Categorical Plotting')
plt.show()
lines = plt.plot(10, 20, 30, 100)
plt.setp(lines, color='r', linewidth=2.0)
plt.setp(lines, 'color', 'r', 'linewidth', 2.0)
line, = plt.plot(np.arange(50), np.arange(50) + 10, '-')
plt.show()
5. 多子表多轴及共享轴
多子表多轴,先用点再用线连接,效果图如下: 多子表多轴,绘制点效果图如下: 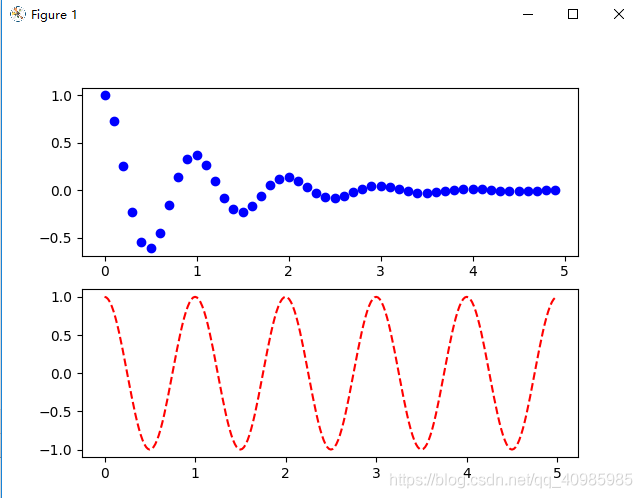
import matplotlib.pyplot as plt
import numpy as np
def f(t):
return np.exp(-t) * np.cos(2 * np.pi * t)
t1 = np.arange(0.0, 5.0, 0.1)
t2 = np.arange(0.0, 5.0, 0.02)
plt.figure()
plt.subplot(211)
plt.plot(t1, f(t1), 'bo', t2, f(t2), 'k')
plt.subplot(212)
plt.plot(t2, np.cos(2 * np.pi * t2), 'r--')
plt.show()
plt.figure()
plt.subplot(211)
plt.plot(t1, f(t1), 'bo')
plt.subplot(212)
plt.plot(t2, np.cos(2 * np.pi * t2), 'r--')
plt.show()
多子表共享y轴,效果图如下:
fig, (ax1, ax2) = plt.subplots(2, sharey=True) 共享y轴
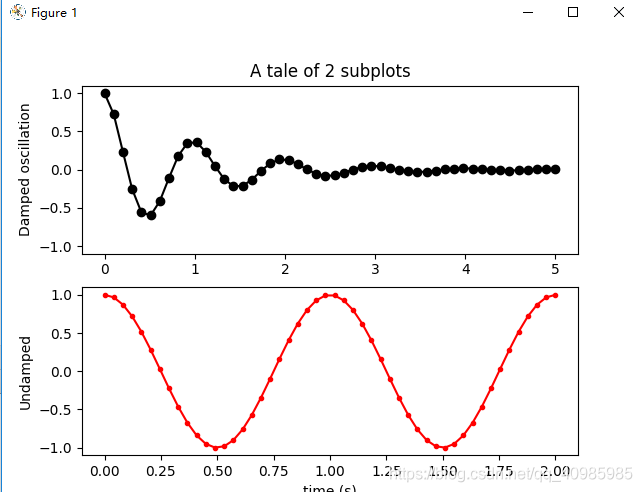
import numpy as np
import matplotlib.pyplot as plt
x1 = np.linspace(0.0, 5.0)
x2 = np.linspace(0.0, 2.0)
y1 = np.cos(2 * np.pi * x1) * np.exp(-x1)
y2 = np.cos(2 * np.pi * x2)
fig, (ax1, ax2) = plt.subplots(2, sharey=True)
ax1.plot(x1, y1, 'ko-')
ax1.set(title='A tale of 2 subplots', ylabel='Damped oscillation')
ax2.plot(x2, y2, 'r.-')
ax2.set(xlabel='time (s)', ylabel='Undamped')
plt.show()
6. 多子表
6.1 多子表垂直堆叠
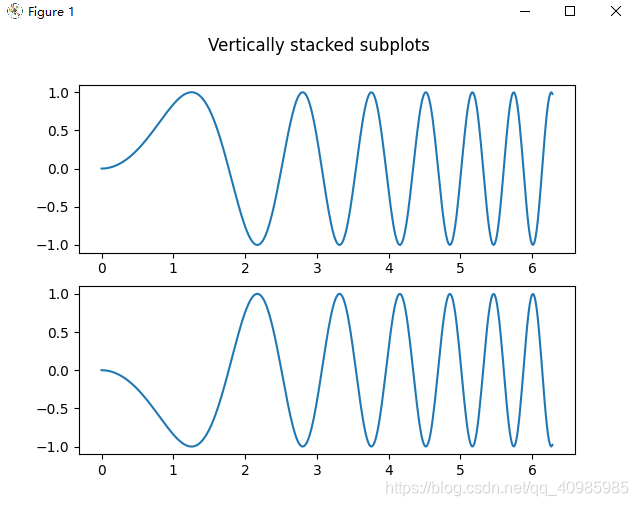
6.2 多子表水平堆叠
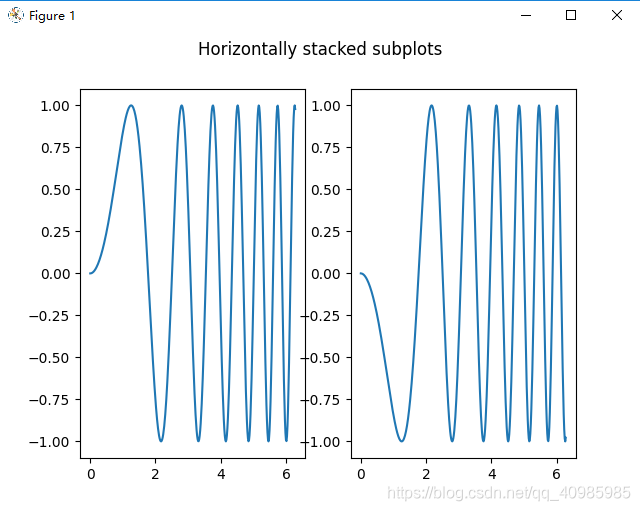
6.3 多子表垂直水平堆叠
隐藏顶部2子图的x标签,和右侧2子图的y标签,效果图如下: 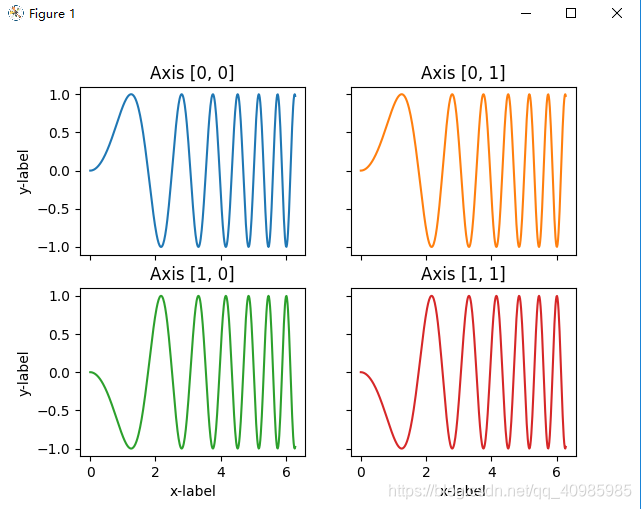 同上图,共享x列,y行的坐标轴~ 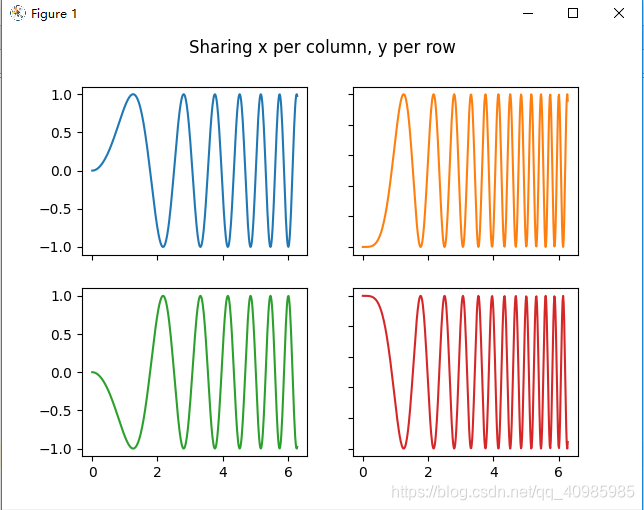
6.4 多子表共享轴(去除垂直、水平堆叠子表之间宽度和高度之间的缝隙)
共享x轴并对齐,效果图如下: 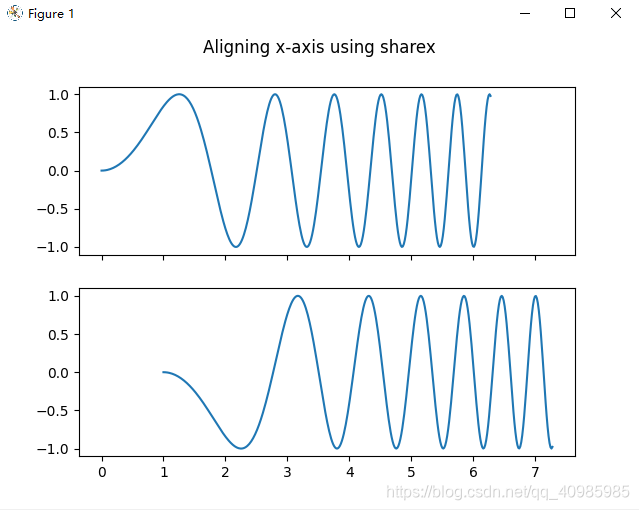 共享x,y轴效果如下图:
共享x,y轴表示共享其值域及刻度;
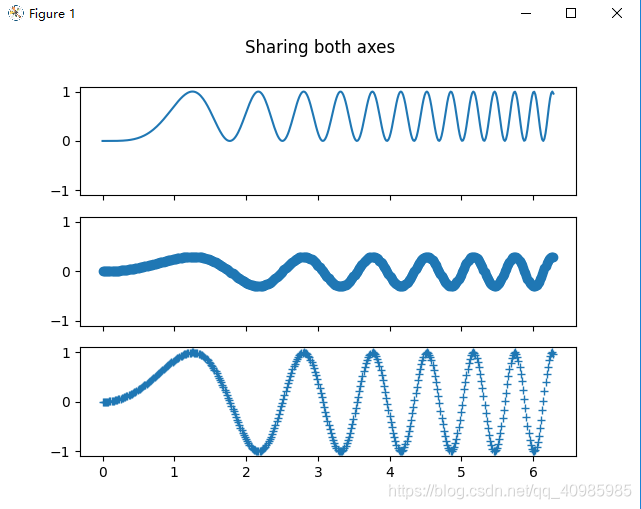
对于共享轴的子图,一组刻度标签就足够了。内部轴的刻度标签由 sharex 和 sharey 自动删除。子图之间仍然有一个未使用的空白空间;
要精确控制子图的定位,可以使用fig.add_gridspec(hspace=0) 减少垂直子图之间的高度。fig.add_gridspec(wspace=0) 减少水平子图之间的高度。
共享x,y轴,并删除多个子表之间高度的缝隙,效果如下图: 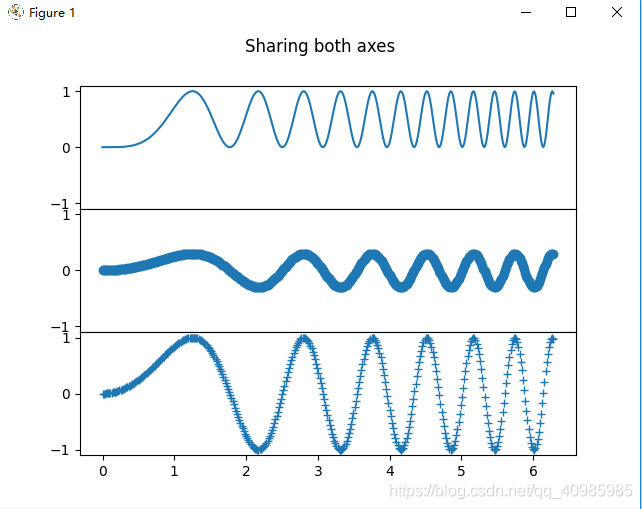 共享x,y轴,并删除多个子表冗余xy标签,及子图之间宽度、高度的缝隙,效果如下图: 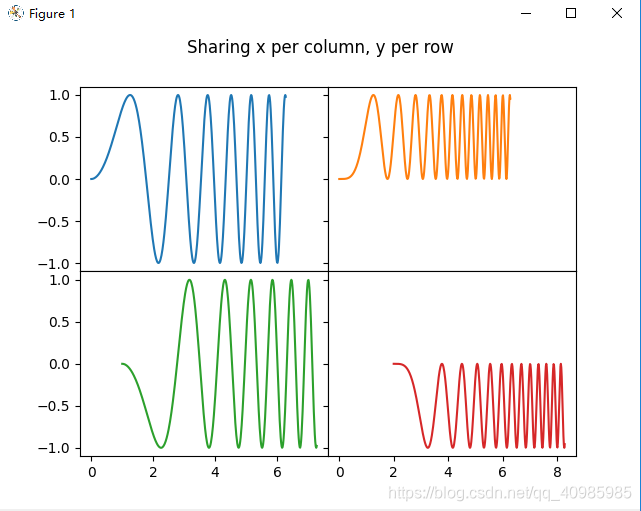
6.5 多子表极坐标风格轴
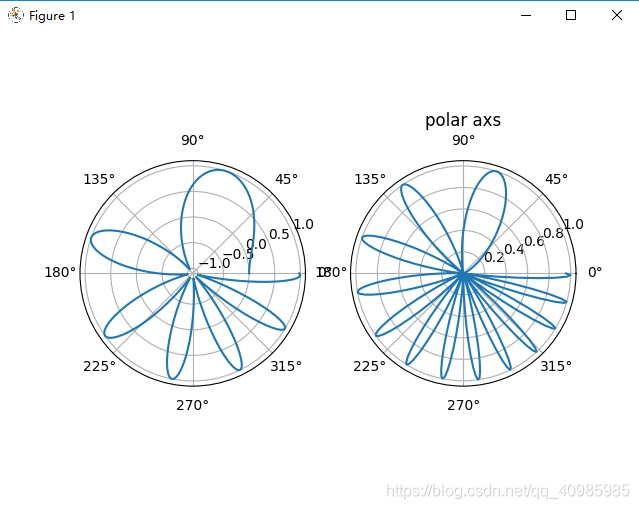
6.6 多子表源码
import matplotlib.pyplot as plt
import numpy as np
x = np.linspace(0, 2 * np.pi, 400)
y = np.sin(x ** 2)
def single_plot():
fig, ax = plt.subplots()
ax.plot(x, y)
ax.set_title('A single plot')
plt.show()
def stacking_plots_one_direction():
fig, axs = plt.subplots(2)
fig.suptitle('Vertically stacked subplots')
axs[0].plot(x, y)
axs[1].plot(x, -y)
plt.show()
fig, (ax1, ax2) = plt.subplots(2)
fig.suptitle('Vertically stacked subplots')
ax1.plot(x, y)
ax2.plot(x, -y)
plt.show()
fig, (ax1, ax2) = plt.subplots(1, 2)
fig.suptitle('Horizontally stacked subplots')
ax1.plot(x, y)
ax2.plot(x, -y)
plt.show()
def stacking_plots_two_directions():
fig, axs = plt.subplots(2, 2)
axs[0, 0].plot(x, y)
axs[0, 0].set_title('Axis [0, 0]')
axs[0, 1].plot(x, y, 'tab:orange')
axs[0, 1].set_title('Axis [0, 1]')
axs[1, 0].plot(x, -y, 'tab:green')
axs[1, 0].set_title('Axis [1, 0]')
axs[1, 1].plot(x, -y, 'tab:red')
axs[1, 1].set_title('Axis [1, 1]')
for ax in axs.flat:
ax.set(xlabel='x-label', ylabel='y-label')
for ax in axs.flat:
ax.label_outer()
plt.show()
fig, ((ax1, ax2), (ax3, ax4)) = plt.subplots(2, 2)
fig.suptitle('Sharing x per column, y per row')
ax1.plot(x, y)
ax2.plot(x, y ** 2, 'tab:orange')
ax3.plot(x, -y, 'tab:green')
ax4.plot(x, -y ** 2, 'tab:red')
for ax in fig.get_axes():
ax.label_outer()
plt.show()
def sharing_axs():
fig, (ax1, ax2) = plt.subplots(2)
fig.suptitle('Axes values are scaled individually by default')
ax1.plot(x, y)
ax2.plot(x + 1, -y)
plt.show()
fig, (ax1, ax2) = plt.subplots(2, sharex=True)
fig.suptitle('Aligning x-axis using sharex')
ax1.plot(x, y)
ax2.plot(x + 1, -y)
plt.show()
fig, axs = plt.subplots(3, sharex=True, sharey=True)
fig.suptitle('Sharing both axes')
axs[0].plot(x, y ** 2)
axs[1].plot(x, 0.3 * y, 'o')
axs[2].plot(x, y, '+')
plt.show()
fig = plt.figure()
gs = fig.add_gridspec(3, hspace=0)
axs = gs.subplots(sharex=True, sharey=True)
fig.suptitle('Sharing both axes')
axs[0].plot(x, y ** 2)
axs[1].plot(x, 0.3 * y, 'o')
axs[2].plot(x, y, '+')
for ax in axs:
ax.label_outer()
plt.show()
fig = plt.figure()
gs = fig.add_gridspec(2, 2, hspace=0, wspace=0)
(ax1, ax2), (ax3, ax4) = gs.subplots(sharex='col', sharey='row')
fig.suptitle('Sharing x per column, y per row')
ax1.plot(x, y)
ax2.plot(x, y ** 2, 'tab:orange')
ax3.plot(x + 1, -y, 'tab:green')
ax4.plot(x + 2, -y ** 2, 'tab:red')
for ax in axs.flat:
ax.label_outer()
plt.show()
fig, axs = plt.subplots(2, 2)
axs[0, 0].plot(x, y)
axs[0, 0].set_title("main")
axs[1, 0].plot(x, y ** 2)
axs[1, 0].set_title("shares x with main")
axs[1, 0].sharex(axs[0, 0])
axs[0, 1].plot(x + 1, y + 1)
axs[0, 1].set_title("unrelated")
axs[1, 1].plot(x + 2, y + 2)
axs[1, 1].set_title("also unrelated")
fig.tight_layout()
plt.show()
def polar_axs():
fig, (ax1, ax2) = plt.subplots(1, 2, subplot_kw=dict(projection='polar'))
ax1.plot(x, y)
ax2.plot(x, y ** 2)
plt.title('polar axs')
plt.show()
single_plot()
stacking_plots_one_direction()
stacking_plots_two_directions()
sharing_axs()
polar_axs()
7. 图表中文本标注(箭头注释)
简单文本标注(只设置要标注的文本内容,起始位置),效果图如下:
plt.text(60, .025, r'$\mu=100,\ \sigma=15$')
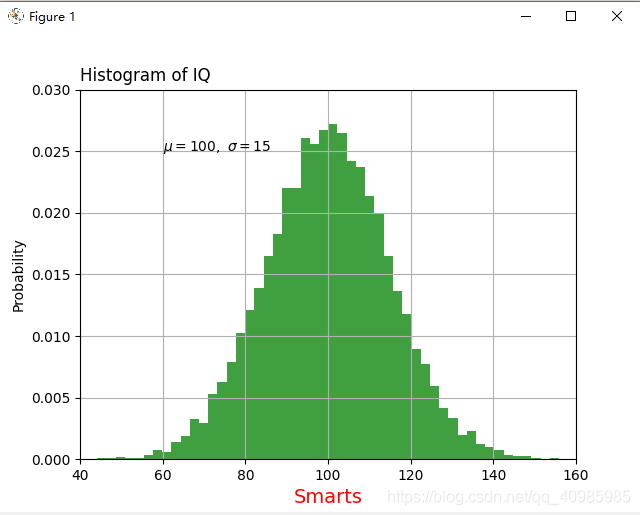
文本标注箭头效果图如下:(需设置箭头起始位置,文本起始位置,文本值)
plt.annotate('local max', xy=(2, 1), xytext=(3, 1.5),
arrowprops=dict(facecolor='yellow', shrink=0.05),
)
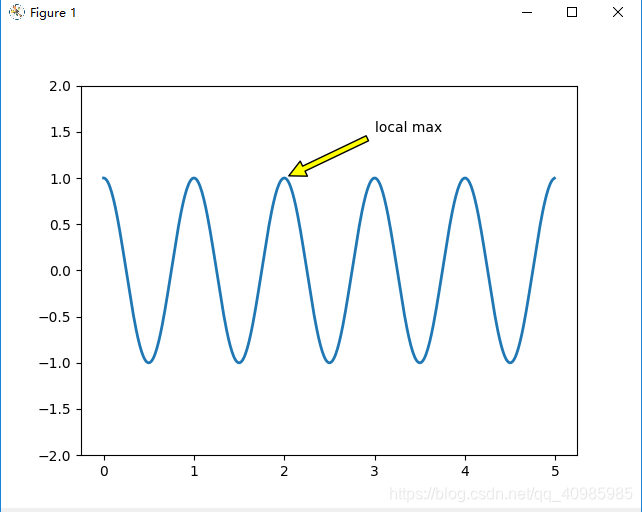
import matplotlib.pyplot as plt
import numpy as np
def simplt_text():
mu, sigma = 100, 15
x = mu + sigma * np.random.randn(10000)
n, bins, patches = plt.hist(x, 50, density=1, facecolor='g', alpha=0.75)
t = plt.xlabel('Smarts', fontsize=14, color='red')
plt.ylabel('Probability')
plt.title('Histogram of IQ', loc='left')
plt.text(60, .025, r'$\mu=100,\ \sigma=15$')
plt.axis([40, 160, 0, 0.03])
plt.grid(True)
plt.show()
def annotation_text():
ax = plt.subplot()
t = np.arange(0.0, 5.0, 0.01)
s = np.cos(2 * np.pi * t)
line, = plt.plot(t, s, lw=2)
plt.annotate('local max', xy=(2, 1), xytext=(3, 1.5),
arrowprops=dict(facecolor='yellow', shrink=0.05),
)
plt.ylim(-2, 2)
plt.show()
simplt_text()
annotation_text()
8. 对数轴和其他非线性轴
线性轴 VS 对数轴 VS 线性对称轴 VS logit轴:
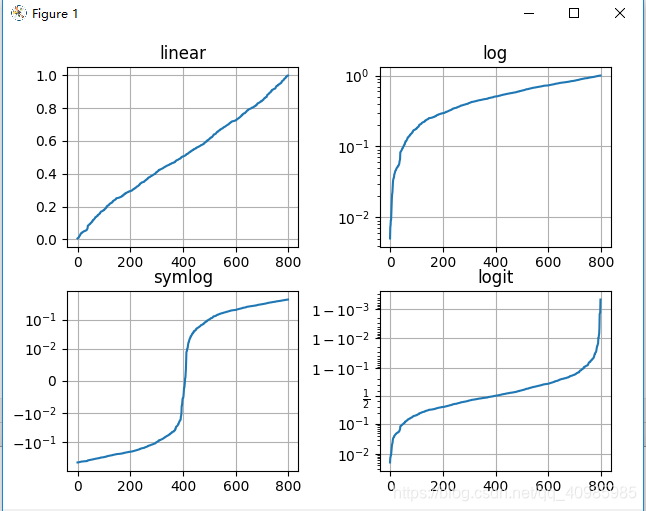
import matplotlib.pyplot as plt
import numpy as np
def logit_plot():
np.random.seed(19680801)
y = np.random.normal(loc=0.5, scale=0.4, size=1000)
y = y[(y > 0) & (y < 1)]
y.sort()
x = np.arange(len(y))
plt.figure()
plt.subplot(221)
plt.plot(x, y)
plt.yscale('linear')
plt.title('linear')
plt.grid(True)
plt.subplot(222)
plt.plot(x, y)
plt.yscale('log')
plt.title('log')
plt.grid(True)
plt.subplot(223)
plt.plot(x, y - y.mean())
plt.yscale('symlog', linthresh=0.01)
plt.title('symlog')
plt.grid(True)
plt.subplot(224)
plt.plot(x, y)
plt.yscale('logit')
plt.title('logit')
plt.grid(True)
plt.subplots_adjust(top=0.92, bottom=0.08, left=0.10, right=0.95, hspace=0.25,
wspace=0.35)
plt.show()
logit_plot()
参考
|