1 Logistic Regression
在这个部分你将建立一个基于两次考试成绩来预测学生是否会被大学录取的模型。
我们将会有之前的申请者的数据作为训练集。
1.1 visualizing the data
数据的可视化在后期判断使用什么算法时是非常有用的。
可惜的是,我还是对数据可视化有一点摸不着头脑,复杂一点的图就搞不懂了,所以接下来会更新一些matplotlib的学习笔记~大家如果有需要可以跟着我一起学习哦!
这边由于是完成作业,我会先分析吴恩达给出的图上有的图示要求~ 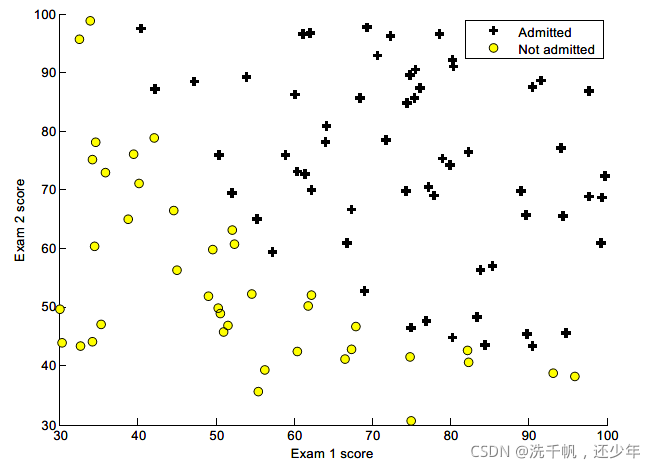 可以看到它的图示区分是按照录取和未录取的~ 所以我们需要先对数据区分开录取的和未录取的~(区分正负样本)
path = 'ex2data1.txt'
data = pd.read_csv(path,header=None,names=['Exam 1','Exam s2','Admitted'])
print(data.head())
positive = data[data['Admitted'].isin([1])]
negative = data[data['Admitted'].isin([0])]
fig,ax = plt.subplots(figsize=(12,8))
ax.scatter(positive['Exam 1'],positive['Exam 2'],s=50,c='b',marker='o',label='Admitted')
ax.scatter(negative['Exam 1'],negative['Exam 2'],s=50,c='r',marker='*',label='Not Admitted')
ax.legend()
ax.set_xlabel('Exam 1 Score')
ax.set_ylabel('Exam 2 Score')
plt.show()
最后图如下: 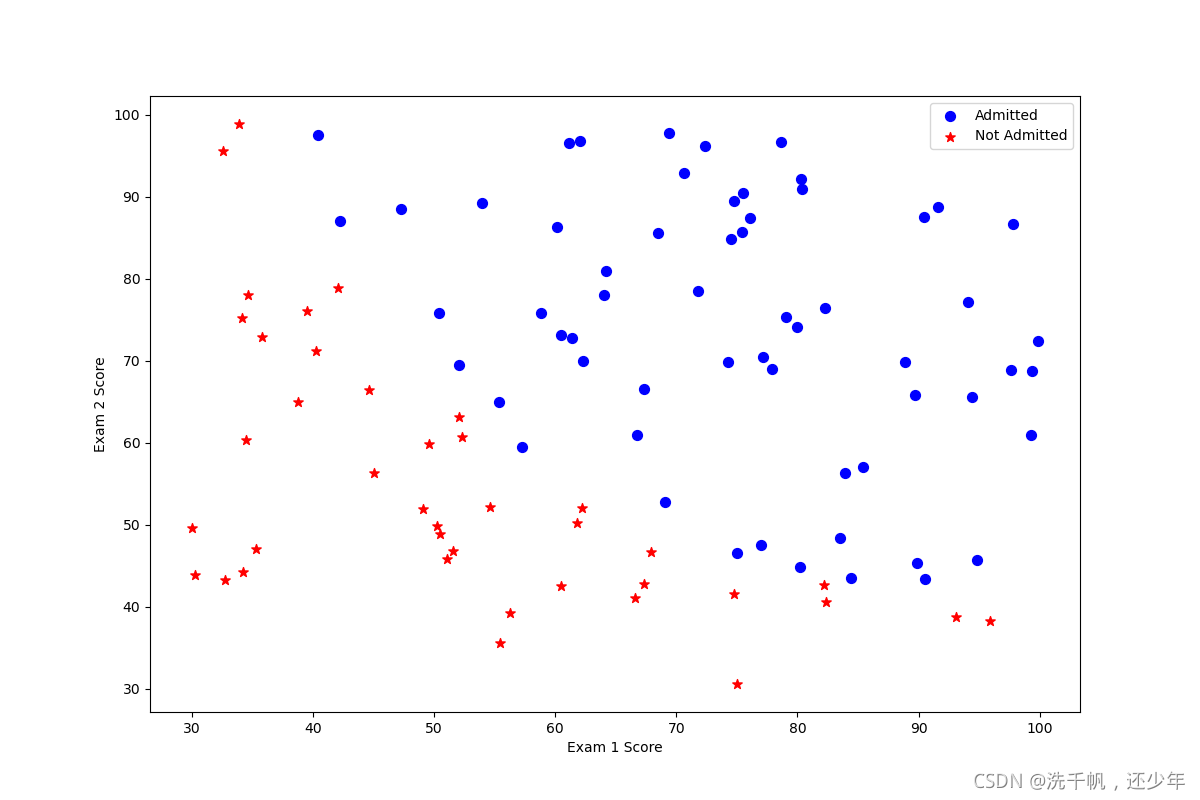
1.2 implementation
1.2.1 sigmoid function
这边我们先回顾一下sigmoid函数~
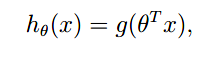 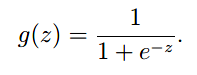 使用sigmoid函数的原因是因为如果用线性拟合的话就会导致预测值可能 >1 or <0,而sigmoid函数恒在这个区间内
from math import e
def Sigmoid(z):
bottom = 1 + pow(e,-z)
return 1/bottom
def Hypothesis(x,theta):
return Sigmoid(theta.T*x)
1.2.2 Cost function and gradient
代价函数和梯度这里的写法和之前有所区分哦~
看似梯度和线性回归的式子类似,但是其中的h(x)是不同的
同样来回顾一下logistic回归的代价函数:  梯度: 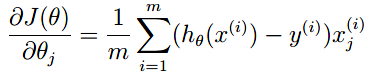 如果实现顺利的话,我们可以使用theta的初始值带入,获得初始的cost为0.693.
def cost(theta, X, y):
theta = np.matrix(theta)
X = np.matrix(X)
y = np.matrix(y)
first = np.multiply(-y, np.log(hypo(X,theta)))
second = np.multiply((1 - y), np.log(1 - hypo(X,theta)))
return np.sum(first - second) / (len(X))
def gradient(theta,X,y):
X=np.matrix(X)
y=np.matrix(y)
theta=np.matrix(theta)
parameters = int(theta.ravel().shape[1])
grad = np.zeros(parameters)
error=hypo(X,theta)-y
for i in range(parameters):
term = np.multiply(error,X[:,i])
grad[i]=np.sum(term)/len(X)
return grad
数据预处理部分省略,和线性回归类似 进行测试:
print(cost(theta,X,y))
输出:
0.6931471805599453
1.2.3 Learning parameters using fminunc
高级优化算法,这里的算法是matlab里面的。(下面一段话是引用的)
这次,我们不自己写代码实现梯度下降,我们会调用一个已有的库。这就是说,我们不用自己定义迭代次数和步长,功能会直接告诉我们最优解。 andrew ng在课程中用的是Octave的“fminunc”函数,由于我们使用Python,我们可以用scipy.optimize.fmin_tnc做同样的事情。 (另外,如果对fminunc有疑问的,可以参考下面这篇百度文库的内容https://wenku.baidu.com/view/2f6ce65d0b1c59eef8c7b47a.html ) 如果一切顺利的话,最有θ对应的代价应该是0.203
* scipy的一些内容介绍
我们来看一下python的scipy.optimiza.fmin_tnc吧~ 这里如果因为直接安装anaconda,使用anaconda中集成的scipy包报错的问题可以看一下我另一篇文章(https://blog.csdn.net/qq_45040216/article/details/119920676)
scipy中的optimize子包中提供了常用的最优化算法函数实现,我们可以直接调用这些函数完成我们的优化问题。
scipy.optimize包提供了几种常用的优化算法。 该模块包含以下几个方面
- 使用各种算法(例如BFGS,Nelder-Mead单纯形,牛顿共轭梯度,COBYLA或SLSQP)的无约束和约束最小化多元标量函数(minimize())
- 全局(蛮力)优化程序(例如,anneal(),basinhopping())
- 最小二乘最小化(leastsq())和曲线拟合(curve_fit())算法
- 标量单变量函数最小化(minim_scalar())和根查找(newton())
- 使用多种算法(例如,Powell,Levenberg-Marquardt混合或Newton-Krylov等大规模方法)的多元方程系统求解
fmin_tnc()
调用:
scipy.optimize.fmin_tnc(func, x0, fprime=None, args=(), approx_grad=0, bounds=None, epsilon=1e-08, scale=None, offset=None, messages=15, maxCGit=-1, maxfun=None, eta=-1, stepmx=0, accuracy=0, fmin=0, ftol=-1, xtol=-1, pgtol=-1, rescale=-1, disp=None, callback=None)
最常使用的参数:
- func:优化的目标函数
- x0:初值
- fprime:提供优化函数func的梯度函数,不然优化函数func必须返回函数值和梯度,或者设置approx_grad=True
- approx_grad :如果设置为True,会给出近似梯度
- args:元组,是传递给优化函数的参数
返回:
- x : 数组,返回的优化问题目标值
- nfeval : 整数,function evaluations的数目。
在进行优化的时候,每当目标优化函数被调用一次,就算一个function evaluation。在一次迭代过程中会有多次function evaluation。这个参数不等同于迭代次数,而往往大于迭代次数。 - rc : int,Return code, see below
使用scipy
接下来使用:
result = opt.fmin_tnc(func=cost,x0=theta,fprime=gradient,args=(X,y))
print(result)
[out]:
array([-25.16131866, 0.20623159, 0.20147149]), 36, 0
array数组中存放的是最优的theta参数
我们来看一下最优的cost是多少:0.203
theta_new = np.matrix(result[0])
cost = cost(theta_new,X,y)
print(cost)
[out]:
0.2034977015894744
1.2.4 绘制决策边界
positive = data[data['Admitted'].isin([1])]
negative = data[data['Admitted'].isin([0])]
fig, ax = plt.subplots(figsize=(12, 8))
ax.scatter(positive['Exam 1'], positive['Exam 2'], s=30, c='b', marker='o', label='Admitted')
ax.scatter(negative['Exam 1'], negative['Exam 2'], s=30, c='r', marker='x', label='Not Admitted')
ax.legend()
ax.set_xlabel('Exam 1 Score')
ax.set_ylabel('Exam 2 Score')
x_prex = np.linspace(30,100,100)
y_prex = (-result[0][0]-result[0][1]*x_prex)/result[0][2]
ax.plot(x_prex,y_prex,'y',label='prediction')
ax.legend()
图如下: 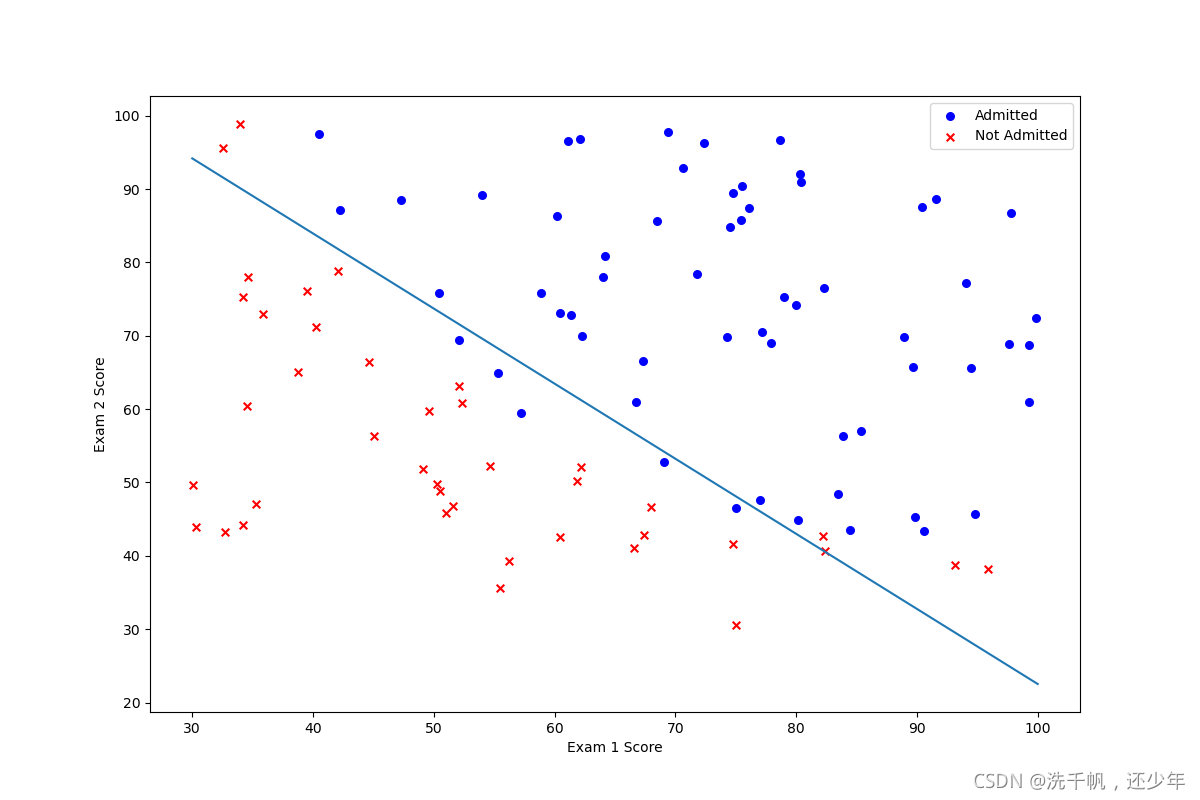
1.2.5 Evaluating logistic regression
对一个学生预测其录取概率:第一次考试成绩:45;第二次考试成绩:85
首先先定义一个预测函数
def predict_fun(theta,X):
probability = hypo(X,theta)
return [1 if x>=0.5 else 0 for x in probability]
score=[1,45,85]
predict=hypo(score,theta_new)
print(predict)
评估这个模型的准确性:
X=np.matrix(X)
predictions = predict_fun(theta_new,X)
correct=0
y=np.matrix(y)
for i in range(len(y)):
if predictions[i] == y[i]:
correct+=1
print('accuracy = '+str(correct)+ '%')
1.3 完整代码
import numpy as np
import pandas as pd
import matplotlib.pyplot as plt
import scipy.optimize as opt
from math import e
def Sigmoid(z):
bottom = 1 + np.exp(-z)
return 1/bottom
def hypo(X,theta):
return Sigmoid(X*theta.T)
def cost(theta, X, y):
theta = np.matrix(theta)
X = np.matrix(X)
y = np.matrix(y)
first = np.multiply(-y, np.log(hypo(X,theta)))
second = np.multiply((1 - y), np.log(1 - hypo(X,theta)))
return np.sum(first - second) / (len(X))
def gradient(theta,X,y):
X=np.matrix(X)
y=np.matrix(y)
theta=np.matrix(theta)
parameters = int(theta.ravel().shape[1])
grad = np.zeros(parameters)
error=hypo(X,theta)-y
for i in range(parameters):
term = np.multiply(error,X[:,i])
grad[i]=np.sum(term)/len(X)
return grad
def predict_fun(theta,X):
probability = hypo(X,theta)
return [1 if x>=0.5 else 0 for x in probability]
path = 'ex2data1.txt'
data = pd.read_csv(path,header=None,names=['Exam 1','Exam 2','Admitted'])
data.insert(0,'Ones',1)
cols = data.shape[1]
X=data.iloc[:,0:cols-1]
y=data.iloc[:,cols-1:cols]
theta=np.zeros(3)
print(X.shape,y.shape,theta.shape)
result = opt.fmin_tnc(func=cost,x0=theta,fprime=gradient,args=(X,y))
print(result)
theta_new = np.matrix(result[0])
cost = cost(theta_new,X,y)
print(cost)
positive = data[data['Admitted'].isin([1])]
negative = data[data['Admitted'].isin([0])]
fig, ax = plt.subplots(figsize=(12, 8))
ax.scatter(positive['Exam 1'], positive['Exam 2'], s=30, c='b', marker='o', label='Admitted')
ax.scatter(negative['Exam 1'], negative['Exam 2'], s=30, c='r', marker='x', label='Not Admitted')
ax.legend()
ax.set_xlabel('Exam 1 Score')
ax.set_ylabel('Exam 2 Score')
x_prex = np.linspace(30,100,100)
y_prex = (-result[0][0]-result[0][1]*x_prex)/result[0][2]
ax.plot(x_prex,y_prex,'y',label='prediction')
ax.legend()
score=[1,45,85]
predict=hypo(score,theta_new)
print(predict)
X=np.matrix(X)
predictions = predict_fun(theta_new,X)
correct=0
y=np.matrix(y)
for i in range(len(y)):
if predictions[i] == y[i]:
correct+=1
print('accuracy = '+str(correct)+ '%')
2 Regularized logistic regression
设想你是工厂的生产主管,你有一些芯片在两次测试中的测试结果,测试结果决定是否芯片要被接受或丢弃。你有一些历史数据,帮助你构建一个逻辑回归模型。
2.1 Visualizing the data
这边就不在赘述了,直接上图和代码
import numpy as np
import pandas as pd
import matplotlib.pyplot as plt
path = 'ex2data2.txt'
data = pd.read_csv(path,header=None,names=['Test 1','Test 2','Result'])
positive = data[data['Result'].isin([1])]
negative = data[data['Result'].isin([0])]
fig,ax=plt.subplots(figsize=(12,8))
ax.scatter(positive['Test 1'],positive['Test 2'],c='b',marker='*',label='accepted')
ax.scatter(negative['Test 1'],negative['Test 2'],c='y',marker='o',label='rejected')
ax.legend()
ax.set_xlabel('Microchip Test 1')
ax.set_ylabel('Microchip Test 2')
plt.show()
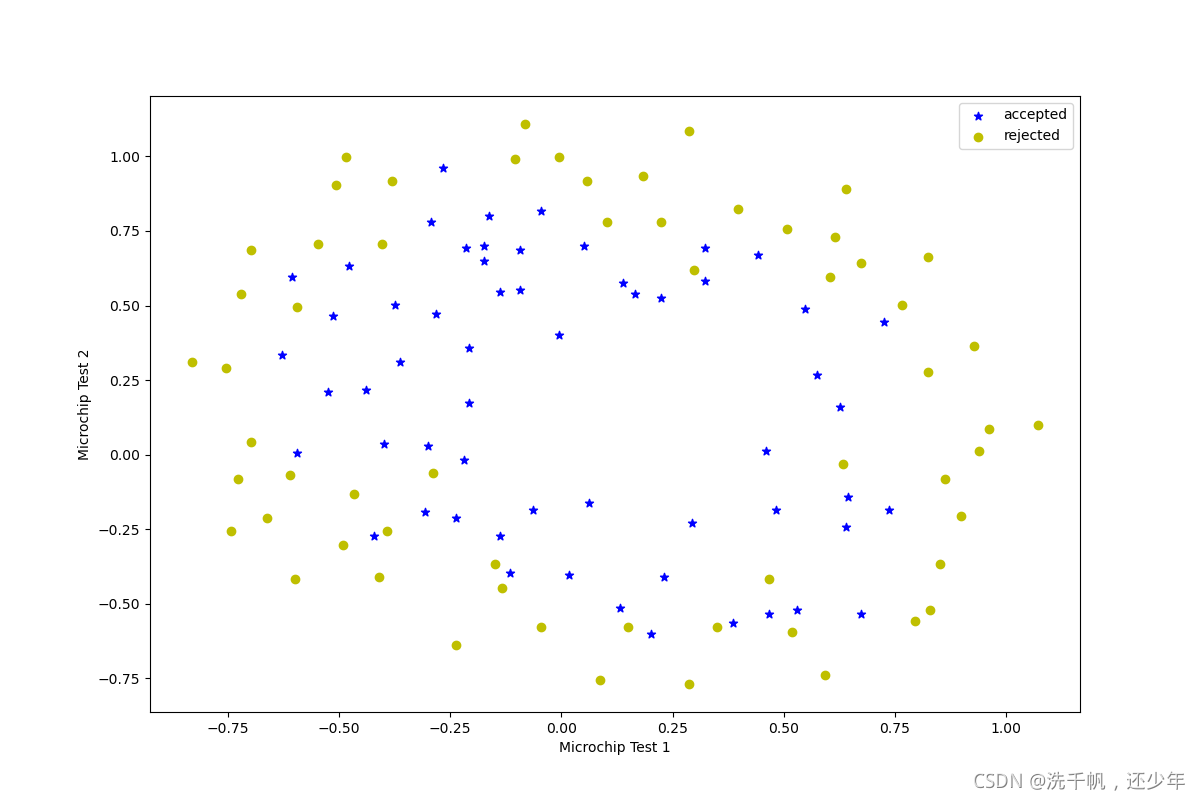
2.2 Feature mapping
我们可以看到这个数据用直线显然是无法拟合的。而原有的逻辑回归只适用于线性的分割,所以,这个数据集不适合直接使用逻辑回归。 所以我们可以使用特征映射的方式,增加多项式项。 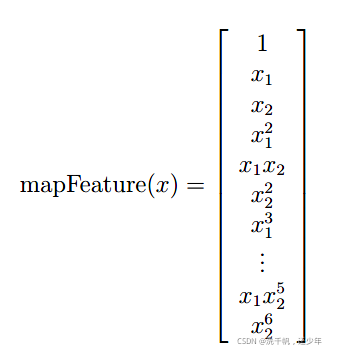 将特征增加到6维,这里使用一个循环
degree = 6
path = 'ex2data2.txt'
data = pd.read_csv(path,names=['Test 1','Test 2','accepted'])
positive = data[data['accepted'].isin([1])]
negative = data[data['accepted'].isin([0])]
x1 = data['Test 1']
x2 = data['Test 2']
data.insert(3,'Ones',1)
for i in range(1, degree+1):
for j in range(0, i+1):
data['F' + str(i-j) + str(j)] = np.power(x1, i-j) * np.power(x2, j)
data.drop(['Test 1','Test 2'],axis=1,inplace=True)
print(data.shape)
修改完的数据如下:
 但是我们知道**,增加维度可以增加拟合程度,但同样可能造成过拟合**,这就是为什么我们提出了正则化(regularization) 利用正则化可以很好地避免过拟合的问题,但是如果正则参数设置的不合适,会导致欠拟合(bias 过大)
2.3 Cost function and gradient
来先回忆一下 正则逻辑回归的 cost function 和梯度,再进行实现:  注意梯度:我们只对j不等于0的项进行惩罚  
def sigmoid(z):
return 1/(1 + np.exp(-z))
def cost(theta,X,y,learningRate):
theta=np.matrix(theta)
X=np.matrix(X)
y=np.matrix(y)
m = len(X)
first = np.multiply(-y,np.log(sigmoid(X*theta.T)))
second = np.multiply((1-y),np.log(1-sigmoid(X*theta.T)))
punish = (learningRate/(2*len(X))) * np.sum(np.power(theta[:,1:theta.shape[1]],2))
return np.sum(first-second)/m + punish
def gradient(theta,X,y,learningRate):
theta=np.matrix(theta)
X=np.matrix(X)
y=np.matrix(y)
parameters = int(theta.ravel().shape[1])
grad = np.zeros(parameters)
error = sigmoid(X*theta.T) - y
for i in range(parameters):
term = np.multiply(error,X[:,i])
if (i == 0):
grad[i] = np.sum(term)/len(X)
else:
grad[i] = (np.sum(term) / len(X)) + ((learningRate / len(X)) * theta[:,i])
return grad
2.3.1 Learning parameters using fminunc
和上篇类似~ 但是注意: cost、gradient函数 需要第一个形参为theta!!!
cols = data.shape[1]
X=data.iloc[:,1:cols]
y=data.iloc[:,0:1]
theta =np.matrix(np.zeros(cols-1))
X=np.array(X.values)
y=np.array(y.values)
learningRate = 1
cost_demo=cost(theta,X,y,learningRate)
print(cost_demo)
result = opt.fmin_tnc(func=cost,x0=theta,fprime=gradient,args=(X,y,learningRate))
print(result)
2.3.2 准确率
我们用上一个part的预测函数。 评估一下这个模型的准确率:
def predict(theta,X):
probability = sigmoid(X*theta.T)
return [1 if x>=0.5 else 0 for x in probability]
theta_min = np.matrix(result[0])
predictions = predict(theta_min, X)
correct = [1 if ((a == 1 and b == 1) or (a == 0 and b == 0)) else 0 for (a, b) in zip(predictions, y)]
accuracy = (sum(map(int, correct)) % len(correct))
print ('accuracy = {0}%'.format(accuracy))
2.4 Plotting the decision boundary
这边的绘图是将决策边界分为左右两部分完成的
def hfunc2(theta, x1, x2):
temp = theta[0][0]
place = 0
for i in range(1, degree+1):
for j in range(0, i+1):
temp+= np.power(x1, i-j) * np.power(x2, j) * theta[0][place+1]
place+=1
return temp
def find_decision_boundary(theta):
t1 = np.linspace(-1, 1.5, 1000)
t2 = np.linspace(-1, 1.5, 1000)
cordinates = [(x, y) for x in t1 for y in t2]
x_cord, y_cord = zip(*cordinates)
h_val = pd.DataFrame({'x1':x_cord, 'x2':y_cord})
h_val['hval'] = hfunc2(theta, h_val['x1'], h_val['x2'])
decision = h_val[np.abs(h_val['hval']) < 2 * 10**-3]
return decision.x1, decision.x2
引用部分:
fig, ax = plt.subplots(figsize=(12,8))
ax.scatter(positive['Test 1'], positive['Test 2'], s=50, c='b', marker='o', label='Accepted')
ax.scatter(negative['Test 1'], negative['Test 2'], s=50, c='r', marker='x', label='Rejected')
ax.set_xlabel('Test 1 Score')
ax.set_ylabel('Test 2 Score')
x, y = find_decision_boundary(result)
plt.scatter(x, y, c='y', s=10, label='Prediction')
ax.legend()
plt.show()
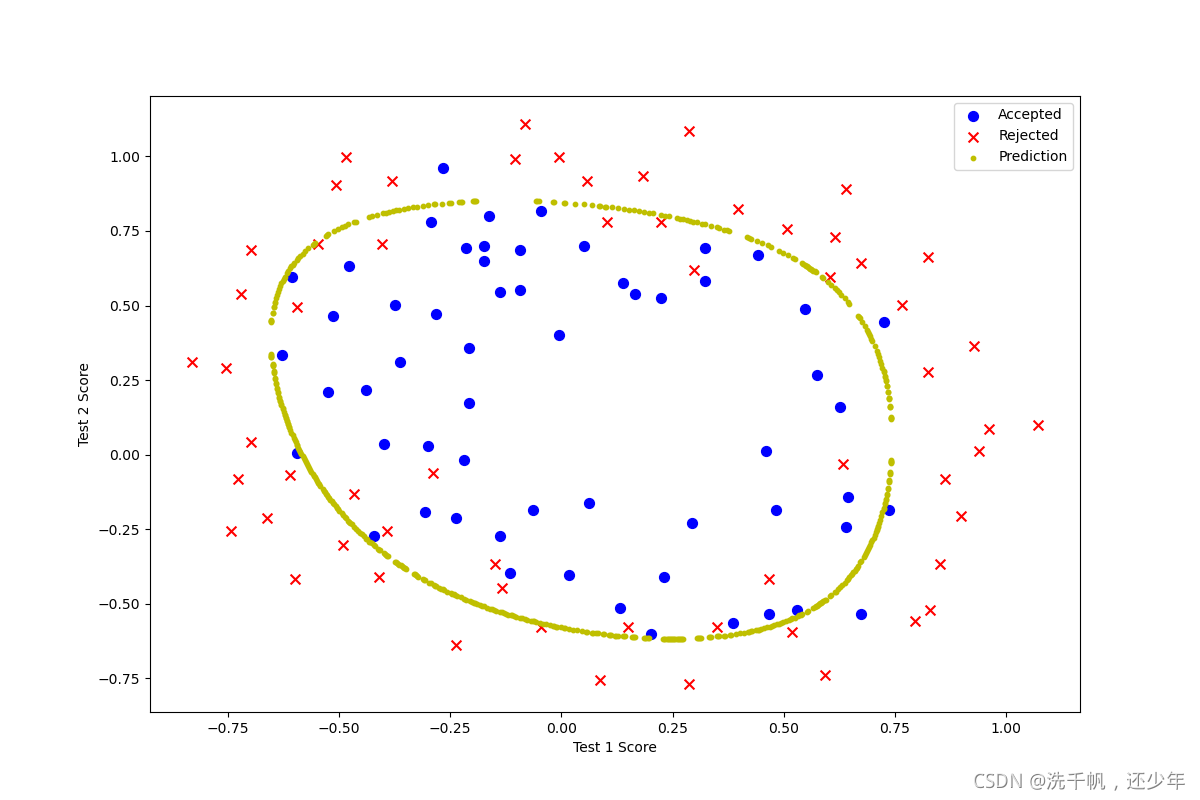
2.5 完整代码
import numpy as np
import pandas as pd
import math
import scipy.optimize as opt
import matplotlib.pyplot as plt
def sigmoid(z):
return 1/(1 + np.exp(-z))
def cost(theta,X,y,learningRate):
theta=np.matrix(theta)
X=np.matrix(X)
y=np.matrix(y)
m = len(X)
first = np.multiply(-y,np.log(sigmoid(X*theta.T)))
second = np.multiply((1-y),np.log(1-sigmoid(X*theta.T)))
punish = (learningRate/(2*len(X))) * np.sum(np.power(theta[:,1:theta.shape[1]],2))
return np.sum(first-second)/m + punish
def gradient(theta,X,y,learningRate):
theta=np.matrix(theta)
X=np.matrix(X)
y=np.matrix(y)
parameters = int(theta.ravel().shape[1])
grad = np.zeros(parameters)
error = sigmoid(X*theta.T) - y
for i in range(parameters):
term = np.multiply(error,X[:,i])
if (i == 0):
grad[i] = np.sum(term)/len(X)
else:
grad[i] = (np.sum(term) / len(X)) + ((learningRate / len(X)) * theta[:,i])
return grad
degree = 6
path = 'ex2data2.txt'
data = pd.read_csv(path,names=['Test 1','Test 2','accepted'])
positive = data[data['accepted'].isin([1])]
negative = data[data['accepted'].isin([0])]
x1 = data['Test 1']
x2 = data['Test 2']
data.insert(3,'Ones',1)
for i in range(1, degree+1):
for j in range(0, i+1):
data['F' + str(i-j) + str(j)] = np.power(x1, i-j) * np.power(x2, j)
data.drop(['Test 1','Test 2'],axis=1,inplace=True)
cols = data.shape[1]
X=data.iloc[:,1:cols]
y=data.iloc[:,0:1]
theta =np.matrix(np.zeros(cols-1))
X=np.array(X.values)
y=np.array(y.values)
learningRate = 1
cost_demo=cost(theta,X,y,learningRate)
print(cost_demo)
result = opt.fmin_tnc(func=cost,x0=theta,fprime=gradient,args=(X,y,learningRate))
print(result)
def predict(theta,X):
probability = sigmoid(X*theta.T)
return [1 if x>=0.5 else 0 for x in probability]
theta_min = np.matrix(result[0])
predictions = predict(theta_min, X)
correct = [1 if ((a == 1 and b == 1) or (a == 0 and b == 0)) else 0 for (a, b) in zip(predictions, y)]
accuracy = (sum(map(int, correct)) % len(correct))
print ('accuracy = {0}%'.format(accuracy))
def hfunc2(theta, x1, x2):
temp = theta[0][0]
place = 0
for i in range(1, degree+1):
for j in range(0, i+1):
temp+= np.power(x1, i-j) * np.power(x2, j) * theta[0][place+1]
place+=1
return temp
def find_decision_boundary(theta):
t1 = np.linspace(-1, 1.5, 1000)
t2 = np.linspace(-1, 1.5, 1000)
cordinates = [(x, y) for x in t1 for y in t2]
x_cord, y_cord = zip(*cordinates)
h_val = pd.DataFrame({'x1':x_cord, 'x2':y_cord})
h_val['hval'] = hfunc2(theta, h_val['x1'], h_val['x2'])
decision = h_val[np.abs(h_val['hval']) < 2 * 10**-3]
return decision.x1, decision.x2
fig, ax = plt.subplots(figsize=(12,8))
ax.scatter(positive['Test 1'], positive['Test 2'], s=50, c='b', marker='o', label='Accepted')
ax.scatter(negative['Test 1'], negative['Test 2'], s=50, c='r', marker='x', label='Rejected')
ax.set_xlabel('Test 1 Score')
ax.set_ylabel('Test 2 Score')
x, y = find_decision_boundary(result)
plt.scatter(x, y, c='y', s=10, label='Prediction')
ax.legend()
plt.show()
|