一、简介和Setup
seaborn 是 python 数据可视化中重要的库,它是基于 matplotlib 的一个拓展,相较于 matplotlib, seaborn 更加更加方便快捷的做出复杂、美观的图。 你可以通过以下方式导入 seaborn:
import matplotlib.pyplot as plt
import seaborn as sns
二、seaborn作图的一些特点
- 函数传入一个DataFrame对象作为
data 参数。 - 字符串应传入到
x 和y 参数中,表示在data 中需要展示的列;如果不传入DataFrame对象作为data 时,x 和y 可以像 matplotlib 一样传入数据。 hue 作为一个很有特色的参数,可以根据hue 中类别对数据进行分类展示,从而在图表中用不同的颜色展示它们。(也就是seaborn里面的FacetGrid绘图方式)
三、分类
1. 趋势
(1) sns.lineplot
sns.lineplot将DataFrame中的每一列的数据随index的变化趋势展示出来。
plt.figure(figsize=(12,6))
sns.lineplot(data = museum_data)
plt.title("Monthly Visitors to Los Angeles City Museums")
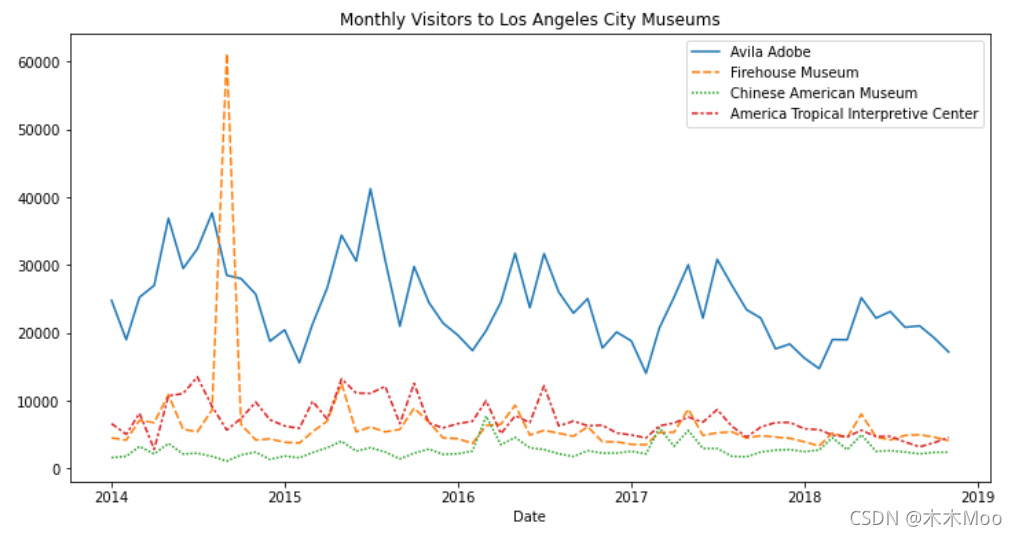
2. 关系
(2) sns.barplot
sns.barplot 以柱状图的形式展示x,y之间统计关系,会对作为横坐标的一方进行合并。
plt.figure(figsize=(12,6))
sns.barplot(
y = ign_data.index,
x = ign_data.Racing
)
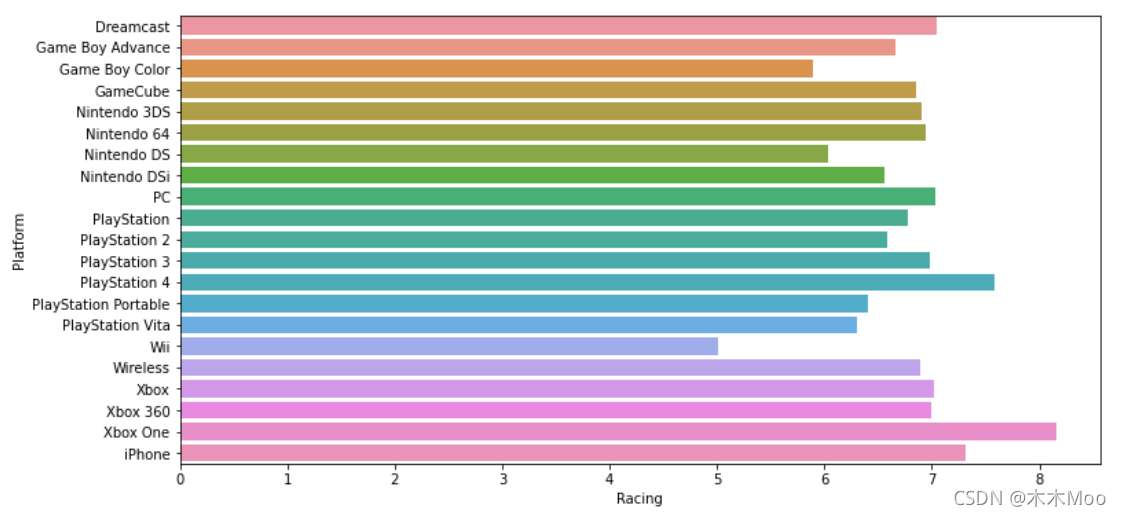
(3) sns.heatmap
sns.heatmap 以颜色矩阵的形式(热力图)展示数据之间的差异,如要展示相关性,可以配合df.corr() 使用。
plt.figure(figsize = (12,6))
sns.heatmap(data = ign_data,annot=True)
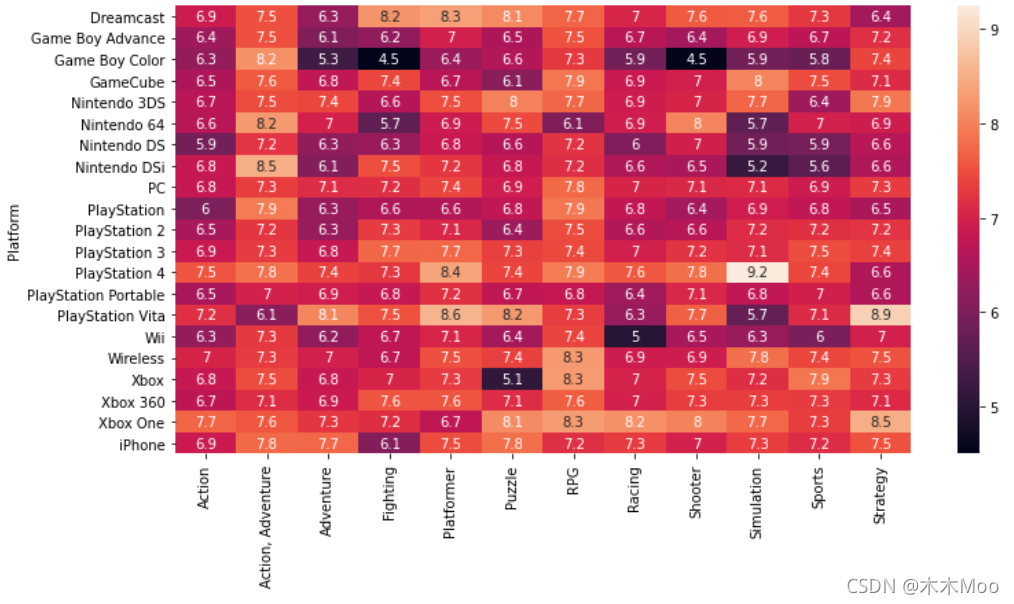
(4) sns.scattermap
sns.scattermap 以 (x,y) 为坐标展示散点。
plt.figure(figsize=(12,6))
sns.scatterplot(
x=candy_data['pricepercent'],
y=candy_data['winpercent'],
hue=candy_data['chocolate']
)
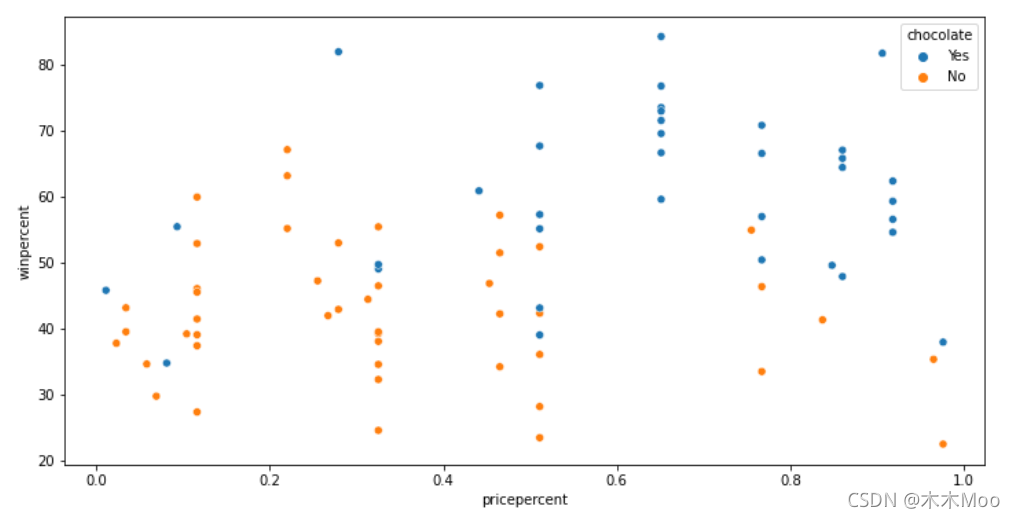
(5) sns.regplot
sns.regplot 在展示散点的同时对数据进行线性回归,展示拟合后的直线已经置信区间。
plt.figure(figsize=(12,6))
sns.regplot(
x=candy_data['sugarpercent'],
y=candy_data['winpercent']
)
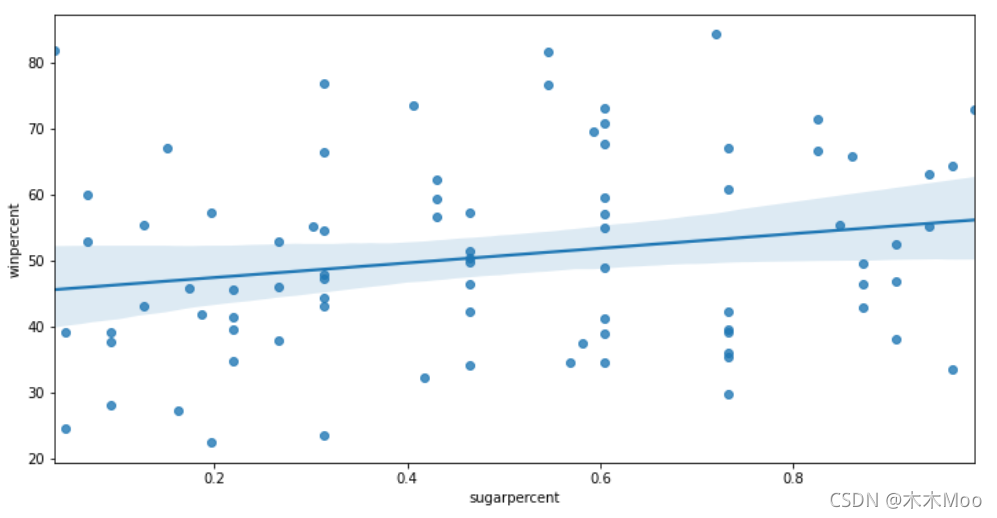
(6) sns.lmplot
sns.lmplot 与sns.regplot 类似,不过允许使用FacetGrid的绘图方式。
sns.lmplot(
x="pricepercent",
y="winpercent",
hue="chocolate",
data=candy_data
)
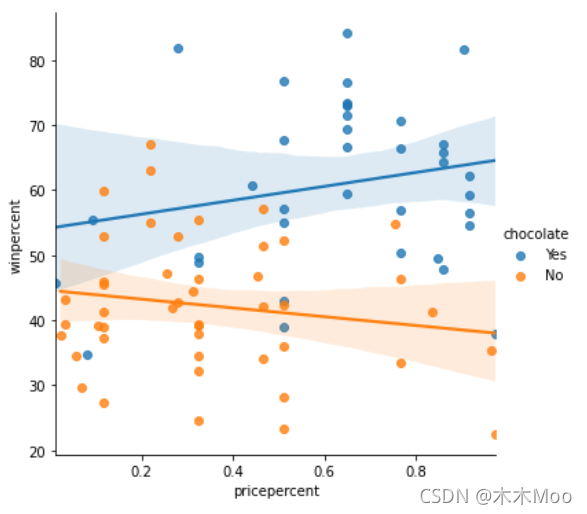
(7) sns.swarmplot
sns.swarmplot 绘制分簇散点图,x轴表示不同的类别,y轴表示数据的分布,图形越”粗“表示数据在此处分布最密集。某种程度上来说也是数据的分布,但是用x对数据进行了分类。
sns.swarmplot(
x=candy_data['chocolate'],
y=candy_data['winpercent']
)
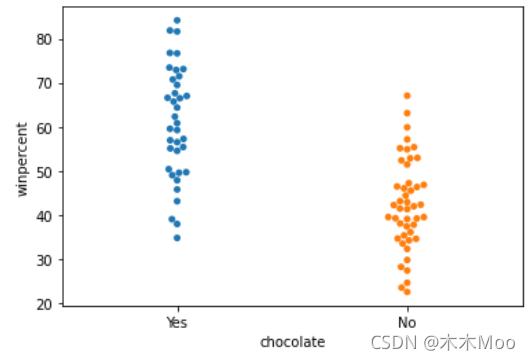
3. 分布
(1) sns.displot
sns.displot 绘制所给数据的频率分布直方图。kde=False 在这种情况下是必须的。需要注意,这里的参数是a 。
sns.distplot(
a=cancer_b_data['Area (mean)'],
label="Benign",
kde=False
)
sns.distplot(
a=cancer_m_data['Area (mean)'],
label="Malignant",
kde=False
)
plt.legend()
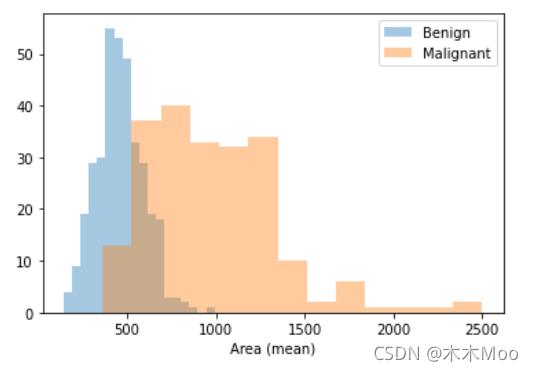
(2) sns.kdeplot
sns.kdeplot 是密度的分布曲线,看起来像是连续的。
sns.kdeplot(
data=cancer_b_data['Radius (worst)'],
shade=True,
label="Benign"
)
sns.kdeplot(
data=cancer_m_data['Radius (worst)'],
shade=True,
label="Malignant"
)
plt.legend()
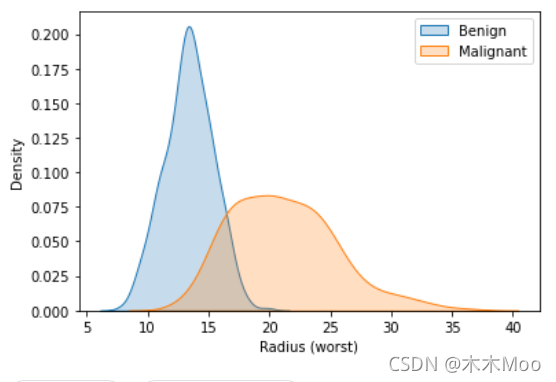
(3) sns.jointplot
sns.jointlpot 绘制2D的kde曲线。
sns.jointplot(
x=iris_data['Petal Length (cm)'],
y=iris_data['Sepal Width (cm)'],
kind="kde"
)
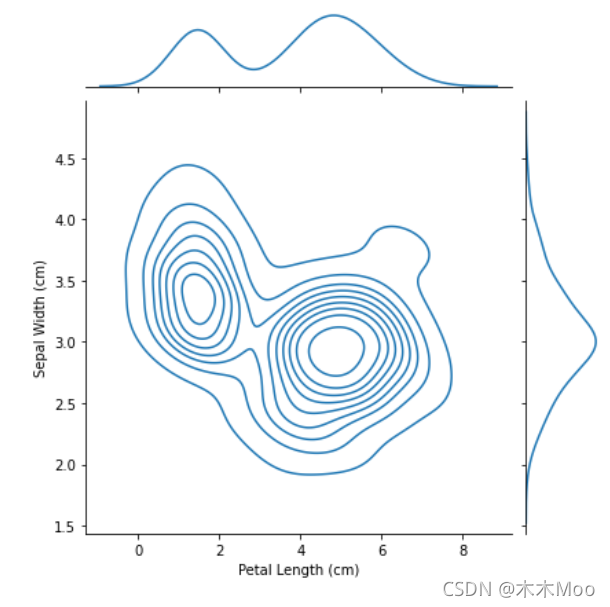
四、总结
seaborn 的绘图功能十分强大,以上列出的只是 seaborn 的一些非常基础的功能,还有一些有关于渲染、网格绘图等高级功能等待学习者们挖掘。
|