一、整体文件夹形式
data_annotated:将原图以及原图生成的json文件都复制进该文件夹。 data_dataset_coco:运行labelme2coco.py后生成的文件夹,运行前需要把该文件夹删了 labelme2coco.py:将自己数据集转化成coco数据集的脚本。 labels.txt:写分类的种类,有固定格式:
__ignore__
_background_
dog
tv/monitor
二、labelme标注数据集
在数据图片中将物体的轮廓标注出来 这里标注完会生成与图片同名的json文件,我们这里把原图和对应的json文件放在同一文件夹中
二、labelme2coco.py代码
#!/usr/bin/env python
import argparse
import collections
import datetime
import glob
import json
import os
import os.path as osp
import sys
import uuid
import imgviz
import numpy as np
import labelme
try:
import pycocotools.mask
except ImportError:
print("Please install pycocotools:\n\n pip install pycocotools\n")
sys.exit(1)
def main():
parser = argparse.ArgumentParser(
formatter_class=argparse.ArgumentDefaultsHelpFormatter
)
parser.add_argument("--input_dir", default='data_annotated')
parser.add_argument("--output_dir", default='data_dataset_coco')
parser.add_argument("--labels", default='labels.txt')
parser.add_argument(
"--noviz", help="no visualization", action="store_true"
)
args = parser.parse_args()
if osp.exists(args.output_dir):
print("Output directory already exists:", args.output_dir)
sys.exit(1)
os.makedirs(args.output_dir)
os.makedirs(osp.join(args.output_dir, "JPEGImages"))
if not args.noviz:
os.makedirs(osp.join(args.output_dir, "Visualization"))
print("Creating dataset:", args.output_dir)
now = datetime.datetime.now()
data = dict(
info=dict(
description=None,
url=None,
version=None,
year=now.year,
contributor=None,
date_created=now.strftime("%Y-%m-%d %H:%M:%S.%f"),
),
licenses=[dict(url=None, id=0, name=None,)],
images=[
],
type="instances",
annotations=[
],
categories=[
],
)
class_name_to_id = {}
for i, line in enumerate(open(args.labels).readlines()):
class_id = i - 1
class_name = line.strip()
if class_id == -1:
assert class_name == "__ignore__"
continue
class_name_to_id[class_name] = class_id
data["categories"].append(
dict(supercategory=None, id=class_id, name=class_name,)
)
out_ann_file = osp.join(args.output_dir, "annotations.json")
label_files = glob.glob(osp.join(args.input_dir, "*.json"))
for image_id, filename in enumerate(label_files):
print("Generating dataset from:", filename)
label_file = labelme.LabelFile(filename=filename)
base = osp.splitext(osp.basename(filename))[0]
out_img_file = osp.join(args.output_dir, "JPEGImages", base + ".jpg")
img = labelme.utils.img_data_to_arr(label_file.imageData)
imgviz.io.imsave(out_img_file, img)
data["images"].append(
dict(
license=0,
url=None,
file_name=osp.relpath(out_img_file, osp.dirname(out_ann_file)),
height=img.shape[0],
width=img.shape[1],
date_captured=None,
id=image_id,
)
)
masks = {}
segmentations = collections.defaultdict(list)
for shape in label_file.shapes:
points = shape["points"]
label = shape["label"]
group_id = shape.get("group_id")
shape_type = shape.get("shape_type", "polygon")
mask = labelme.utils.shape_to_mask(
img.shape[:2], points, shape_type
)
if group_id is None:
group_id = uuid.uuid1()
instance = (label, group_id)
if instance in masks:
masks[instance] = masks[instance] | mask
else:
masks[instance] = mask
if shape_type == "rectangle":
(x1, y1), (x2, y2) = points
x1, x2 = sorted([x1, x2])
y1, y2 = sorted([y1, y2])
points = [x1, y1, x2, y1, x2, y2, x1, y2]
else:
points = np.asarray(points).flatten().tolist()
segmentations[instance].append(points)
segmentations = dict(segmentations)
for instance, mask in masks.items():
cls_name, group_id = instance
if cls_name not in class_name_to_id:
continue
cls_id = class_name_to_id[cls_name]
mask = np.asfortranarray(mask.astype(np.uint8))
mask = pycocotools.mask.encode(mask)
area = float(pycocotools.mask.area(mask))
bbox = pycocotools.mask.toBbox(mask).flatten().tolist()
data["annotations"].append(
dict(
id=len(data["annotations"]),
image_id=image_id,
category_id=cls_id,
segmentation=segmentations[instance],
area=area,
bbox=bbox,
iscrowd=0,
)
)
if not args.noviz:
labels, captions, masks = zip(
*[
(class_name_to_id[cnm], cnm, msk)
for (cnm, gid), msk in masks.items()
if cnm in class_name_to_id
]
)
viz = imgviz.instances2rgb(
image=img,
labels=labels,
masks=masks,
captions=captions,
font_size=15,
line_width=2,
)
out_viz_file = osp.join(
args.output_dir, "Visualization", base + ".jpg"
)
imgviz.io.imsave(out_viz_file, viz)
with open(out_ann_file, "w") as f:
json.dump(data, f)
if __name__ == "__main__":
main()
三、data_dataset_coco解读
文件夹内: 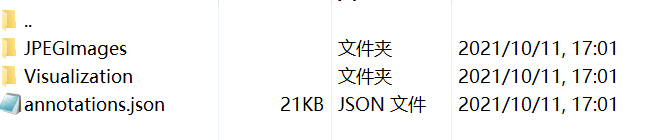 JPEGImages:里面是原图 Visualization:生成的带mask的图片 annotations.json:annotations字段是包含多个annotation实例的一个数组,annotation类型本身又包含了一系列的字段,如这个目标的category id和segmentation mask。segmentation格式取决于这个实例是一个单个的对象(即iscrowd=0,将使用polygons格式)还是一组对象(即iscrowd=1,将使用RLE格式)
|