import numpy as np
import pandas as pd
import matplotlib.pyplot as plt
plt.rcParams['font.sans-serif'] = [u'SimHei']
plt.rcParams['axes.unicode_minus'] = False
x = np.linspace(0,10,100)
y1 = np.sin(x)
y2 = np.cos(x)
plt.plot(x,y,ls=,lw=,c=,marker=,markersize=,markeredgecolor=,markerfacecolor, label=)
-
x: x轴上的数值 -
y: y轴上的数值 -
ls: 折线的风格(‘-‘, ’–‘, ’-.‘和’:‘) -
lw: 线条宽度 -
c: 颜色 -
marker: 线条上点的形状 -
markersize: 线条上点的形状大小 -
markeredgecolor: 点的边框色 -
markerfacecolor: 点的填充色 -
label: 文本标签 -
添加文本: 使用plt.title添加标题, 使用plt.xlabel()和ylabel()添加轴标签 -
添加图例: 使用plt.legend()将图例说明添加到图表中 -
添加网格: 使用plt.grid()绘制网格线 -
保存图形: 使用plt.savefig()可以将图形保存为pdf或者其他格式 -
绘制参考线: 使用plt.axhline和plt.axvline绘制参考线
图例legend中loc中的位置和数字关系的使用
- ‘best’ 0
- ‘upper rught’ 1
- ‘upper left’ 2
- ‘lower left’ 3
- ‘lower right’ 4
- ‘right’ 5
- ‘center left’ 6
- ‘center right’ 7
plt.figure(figsize=(20,8),dpi=80)
plt.plot(x,y1,c='red',lw=2,ls='-',marker='o',markersize=6,markeredgecolor='red',markerfacecolor='yellow',label='y=sin(x)')
plt.plot(x,y2,c='c',lw=2,ls='--',marker='*',markersize=6,markeredgecolor='c',markerfacecolor='y',label='y=cos(x)')
plt.text(6,0.2,'sin(x)',weight='bold',color='red',fontsize=14)
plt.text(5,0.2,'cos(x)',weight='bold',color='c',fontsize=14)
plt.title('y=sin(x)、cos(x)')
plt.xlabel('X')
plt.ylabel('Y')
plt.legend(loc=1,fontsize=12)
plt.grid(ls='--',c='blue')
plt.show()
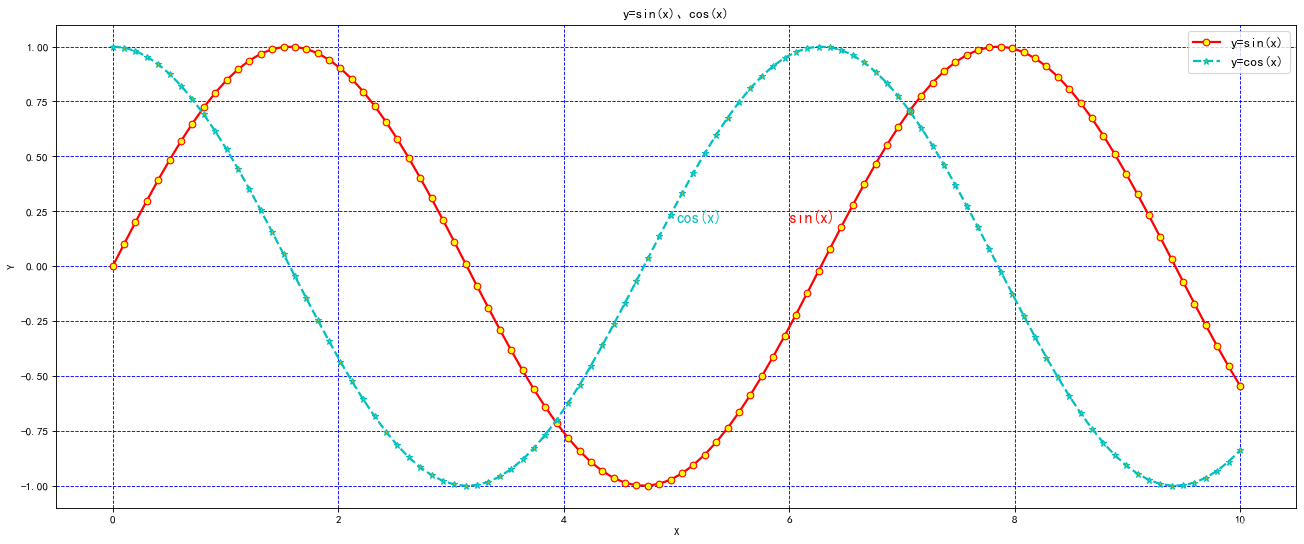
data = pd.read_csv(r'E:\python\Python数据分析与挖掘从零开始到实战\数据分析篇\学习笔记\数据\air_data.csv')
data.head()
| MEMBER_NO | FFP_DATE | FIRST_FLIGHT_DATE | GENDER | FFP_TIER | WORK_CITY | WORK_PROVINCE | WORK_COUNTRY | AGE | LOAD_TIME | ... | ADD_Point_SUM | Eli_Add_Point_Sum | L1Y_ELi_Add_Points | Points_Sum | L1Y_Points_Sum | Ration_L1Y_Flight_Count | Ration_P1Y_Flight_Count | Ration_P1Y_BPS | Ration_L1Y_BPS | Point_NotFlight |
---|
0 | 54993 | 2006/11/2 | 2008/12/24 | 男 | 6 | . | 北京 | CN | 31.0 | 2014/3/31 | ... | 39992 | 114452 | 111100 | 619760 | 370211 | 0.509524 | 0.490476 | 0.487221 | 0.512777 | 50 |
---|
1 | 28065 | 2007/2/19 | 2007/8/3 | 男 | 6 | NaN | 北京 | CN | 42.0 | 2014/3/31 | ... | 12000 | 53288 | 53288 | 415768 | 238410 | 0.514286 | 0.485714 | 0.489289 | 0.510708 | 33 |
---|
2 | 55106 | 2007/2/1 | 2007/8/30 | 男 | 6 | . | 北京 | CN | 40.0 | 2014/3/31 | ... | 15491 | 55202 | 51711 | 406361 | 233798 | 0.518519 | 0.481481 | 0.481467 | 0.518530 | 26 |
---|
3 | 21189 | 2008/8/22 | 2008/8/23 | 男 | 5 | Los Angeles | CA | US | 64.0 | 2014/3/31 | ... | 0 | 34890 | 34890 | 372204 | 186100 | 0.434783 | 0.565217 | 0.551722 | 0.448275 | 12 |
---|
4 | 39546 | 2009/4/10 | 2009/4/15 | 男 | 6 | 贵阳 | 贵州 | CN | 48.0 | 2014/3/31 | ... | 22704 | 64969 | 64969 | 338813 | 210365 | 0.532895 | 0.467105 | 0.469054 | 0.530943 | 39 |
---|
5 rows × 44 columns
data['FFP_DATE'] = pd.to_datetime(data['FFP_DATE'],format='%Y/%m/%d',errors='coerce')
data.head()
| MEMBER_NO | FFP_DATE | FIRST_FLIGHT_DATE | GENDER | FFP_TIER | WORK_CITY | WORK_PROVINCE | WORK_COUNTRY | AGE | LOAD_TIME | ... | ADD_Point_SUM | Eli_Add_Point_Sum | L1Y_ELi_Add_Points | Points_Sum | L1Y_Points_Sum | Ration_L1Y_Flight_Count | Ration_P1Y_Flight_Count | Ration_P1Y_BPS | Ration_L1Y_BPS | Point_NotFlight |
---|
0 | 54993 | 2006-11-02 | 2008/12/24 | 男 | 6 | . | 北京 | CN | 31.0 | 2014/3/31 | ... | 39992 | 114452 | 111100 | 619760 | 370211 | 0.509524 | 0.490476 | 0.487221 | 0.512777 | 50 |
---|
1 | 28065 | 2007-02-19 | 2007/8/3 | 男 | 6 | NaN | 北京 | CN | 42.0 | 2014/3/31 | ... | 12000 | 53288 | 53288 | 415768 | 238410 | 0.514286 | 0.485714 | 0.489289 | 0.510708 | 33 |
---|
2 | 55106 | 2007-02-01 | 2007/8/30 | 男 | 6 | . | 北京 | CN | 40.0 | 2014/3/31 | ... | 15491 | 55202 | 51711 | 406361 | 233798 | 0.518519 | 0.481481 | 0.481467 | 0.518530 | 26 |
---|
3 | 21189 | 2008-08-22 | 2008/8/23 | 男 | 5 | Los Angeles | CA | US | 64.0 | 2014/3/31 | ... | 0 | 34890 | 34890 | 372204 | 186100 | 0.434783 | 0.565217 | 0.551722 | 0.448275 | 12 |
---|
4 | 39546 | 2009-04-10 | 2009/4/15 | 男 | 6 | 贵阳 | 贵州 | CN | 48.0 | 2014/3/31 | ... | 22704 | 64969 | 64969 | 338813 | 210365 | 0.532895 | 0.467105 | 0.469054 | 0.530943 | 39 |
---|
5 rows × 44 columns
每年注册人数
data1 = data.groupby(data['FFP_DATE'].dt.year)['MEMBER_NO'].count()
绘制参考线
plt.figure(figsize=(20,8),dpi=80)
plt.plot(data1.index,data1.values,label='不同年份会员注册数')
plt.legend(loc='best')
plt.axhline(y=5000,c='r',ls='--',lw=2)
plt.axvline(x=2012,c='r',ls='--',lw=2)
plt.savefig('001')
plt.show()
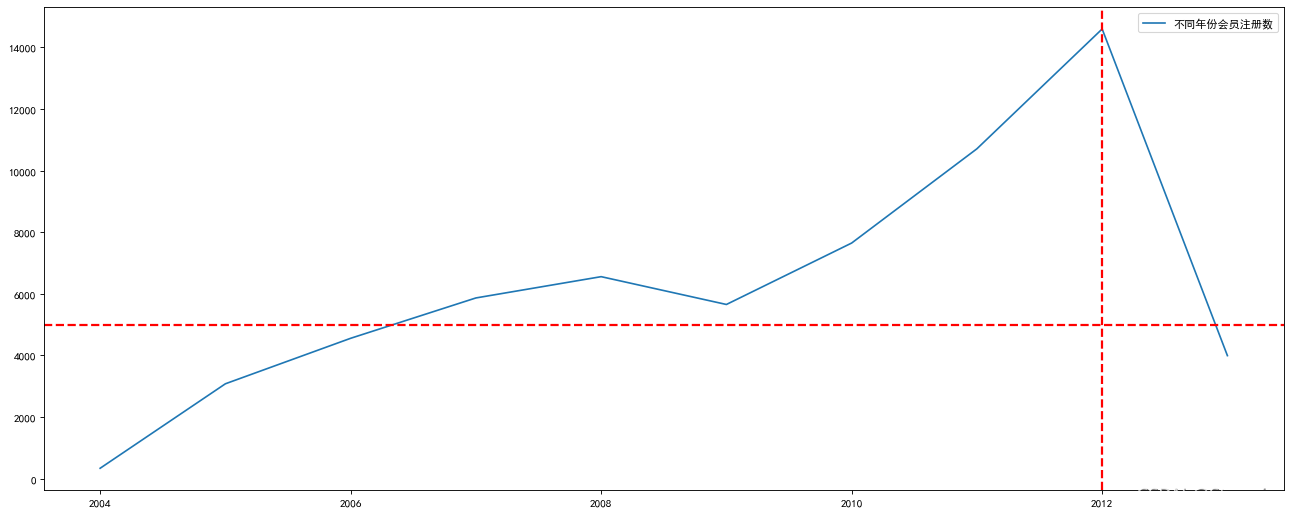
绘制参考区域
plt.figure(figsize=(20,8),dpi=80)
plt.plot(data1.index,data1.values,label='不同年份会员注册数')
plt.legend(loc='best')
plt.axvspan(xmin=2016,xmax=2008,facecolor='red',alpha=0.6)
plt.axhspan(ymin=1250,ymax=5000,facecolor='yellow',alpha=0.3)
plt.savefig('002')
plt.show()
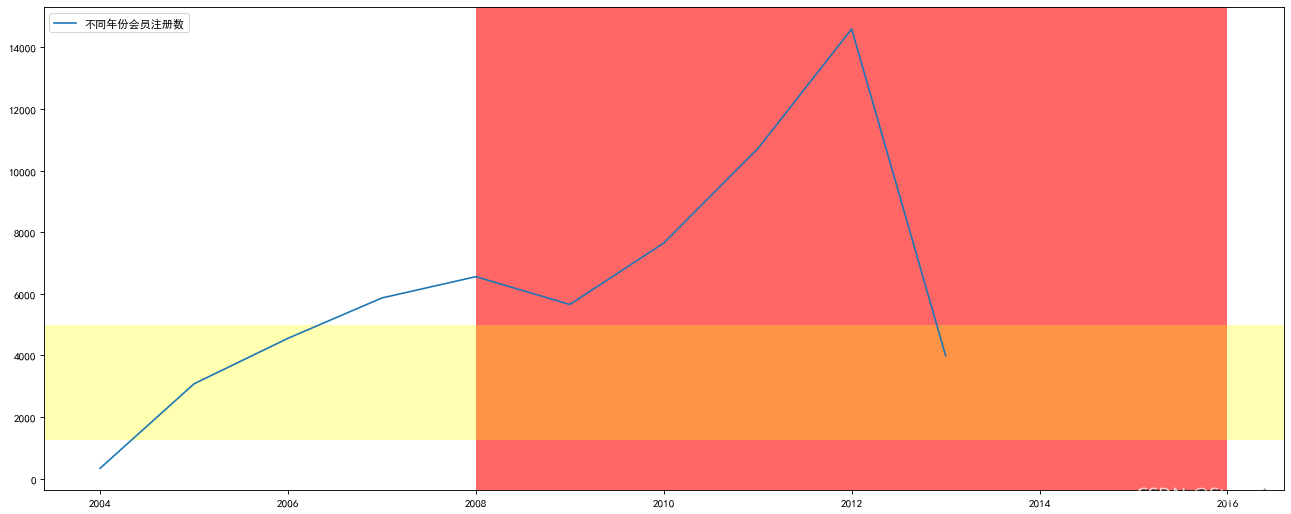
常见图形绘制
df = pd.read_excel(r'E:\python\Python数据分析与挖掘从零开始到实战\数据分析篇\学习笔记\数据\sales_details.xlsx')
df.head()
| 创建日期 | 物料编号 | 应发库 | 销售订单数量 | 交货数量 |
---|
0 | 2013.05.30 | 10482258-00 | sz | 60.0 | 60.0 |
---|
1 | 2013.05.30 | 10261297-00 | sz | 3.0 | 3.0 |
---|
2 | 2013.05.30 | 10134443-00 | jn | 50.0 | 50.0 |
---|
3 | 2013.05.30 | 10195108-00 | jn | 20.0 | 20.0 |
---|
4 | 2013.05.30 | 10261297-00 | jn | 1.0 | 1.0 |
---|
df.info()
<class 'pandas.core.frame.DataFrame'>
RangeIndex: 423214 entries, 0 to 423213
Data columns (total 5 columns):
# Column Non-Null Count Dtype
--- ------ -------------- -----
0 创建日期 423213 non-null object
1 物料编号 423213 non-null object
2 应发库 423213 non-null object
3 销售订单数量 423213 non-null float64
4 交货数量 423213 non-null float64
dtypes: float64(2), object(3)
memory usage: 16.1+ MB
df.columns
Index(['创建日期', '物料编号', '应发库', '销售订单数量', ' 交货数量'], dtype='object')
df.rename(columns={' 交货数量':'交货数量'},inplace=True)
df.columns
Index(['创建日期', '物料编号', '应发库', '销售订单数量', '交货数量'], dtype='object')
df['年份'] = df['创建日期'].str.split('.',expand=True)[0]
df.head()
| 创建日期 | 物料编号 | 应发库 | 销售订单数量 | 交货数量 | 年份 |
---|
0 | 2013.05.30 | 10482258-00 | sz | 60.0 | 60.0 | 2013 |
---|
1 | 2013.05.30 | 10261297-00 | sz | 3.0 | 3.0 | 2013 |
---|
2 | 2013.05.30 | 10134443-00 | jn | 50.0 | 50.0 | 2013 |
---|
3 | 2013.05.30 | 10195108-00 | jn | 20.0 | 20.0 | 2013 |
---|
4 | 2013.05.30 | 10261297-00 | jn | 1.0 | 1.0 | 2013 |
---|
df['创建日期'] = pd.to_datetime(df['创建日期'],format ='%Y.%m.%d',errors = 'coerce')
data_bak = df.groupby(['年份'])['物料编号'].count()
data_bak
年份
2013 50489
2014 110831
2015 177040
2016 84853
Name: 物料编号, dtype: int64
df.info()
<class 'pandas.core.frame.DataFrame'>
RangeIndex: 423214 entries, 0 to 423213
Data columns (total 6 columns):
# Column Non-Null Count Dtype
--- ------ -------------- -----
0 创建日期 423213 non-null datetime64[ns]
1 物料编号 423213 non-null object
2 应发库 423213 non-null object
3 销售订单数量 423213 non-null float64
4 交货数量 423213 non-null float64
5 年份 423213 non-null object
dtypes: datetime64[ns](1), float64(2), object(3)
memory usage: 19.4+ MB
df.head()
| 创建日期 | 物料编号 | 应发库 | 销售订单数量 | 交货数量 | 年份 |
---|
0 | 2013-05-30 | 10482258-00 | sz | 60.0 | 60.0 | 2013 |
---|
1 | 2013-05-30 | 10261297-00 | sz | 3.0 | 3.0 | 2013 |
---|
2 | 2013-05-30 | 10134443-00 | jn | 50.0 | 50.0 | 2013 |
---|
3 | 2013-05-30 | 10195108-00 | jn | 20.0 | 20.0 | 2013 |
---|
4 | 2013-05-30 | 10261297-00 | jn | 1.0 | 1.0 | 2013 |
---|
df['创建日期'].dt.year
0 2013.0
1 2013.0
2 2013.0
3 2013.0
4 2013.0
...
423209 2015.0
423210 2015.0
423211 2015.0
423212 2016.0
423213 2016.0
Name: 创建日期, Length: 423214, dtype: float64
data = df.groupby(df['创建日期'].dt.year).size()
data
创建日期
2013.0 50489
2014.0 110831
2015.0 177040
2016.0 84853
dtype: int64
data.index = data.index.astype(int)
data
创建日期
2013 50489
2014 110831
2015 177040
2016 84853
dtype: int64
1、饼图
饼图用于实现可视化离散型变量的分布的一种图形
- pie(x, explode,labels,colors,autopct,pctdistance,shadow,startangle,radius,wedgeprops,textprops,center)
- –labels: 标签
- –counterclock: 是否逆时针呈现:
- –colors:颜色
- –wedgeprops: 设置饼图内外边界的属性
- –autopct: 百分比
- –textprops: 设置饼图中文本属性
- –pctdistance: 百分比标签与圆心距离
- –center: 设置中心位置
- –shadow: 是否添加饼图阴影效果
- –labeldistance: 设置各扇形标签与圆心距离
- –startangle: 设置饼图的初始摆放角度
- –radius: 设置饼图半径大小
data_x = data.values
data_y = data.index
plt.figure(figsize=(20,8),dpi=80)
plt.axes(aspect='equal')
explode = [0,0.1,0,0]
plt.pie(data_x,
labels = data_y,
explode = explode,
colors = ['orchid','lightgreen','hotpink','green'],
autopct = '%.2f%%',
pctdistance = 0.5,
shadow = True,
startangle = 240,
radius = 1.2,
wedgeprops = {'linewidth':'1.5','edgecolor':'gray'},
textprops ={'fontsize':12,'color':'black'},
counterclock = False
)
plt.title('饼图-订单数量分布',pad=30,fontsize=14)
plt.legend(loc='best')
plt.show()
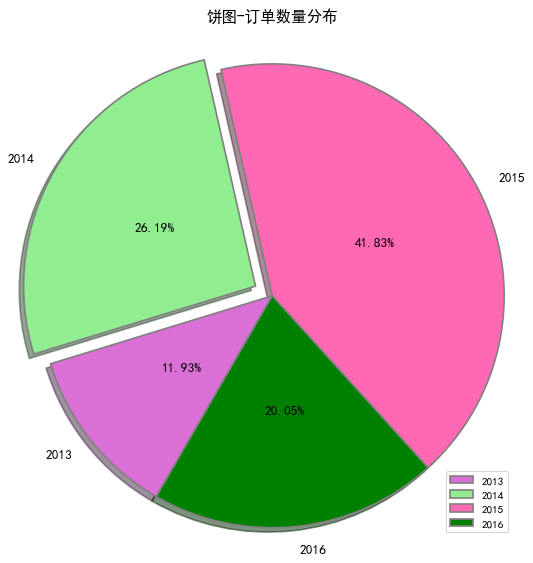
2、柱状图
柱状图(条形图)不仅适合分类水平较多的变量也更加适合对比差异
bar(x, height,width,bottom,color,linewidth,tick_label,align)
- x: 指定x轴上数值
- height: 指定y轴上数值
- width: 指定条形图宽度
- color: 条形图的填充色
- edge: 条形图的边框色
- bottom: 百分比标签与圆心距离
- linewidth: 条形图边框宽度
- tick_label: 条形图的刻度标签
- align: 指定x轴上对齐方式
data_b = df.groupby(df['创建日期'].dt.year).sum()['销售订单数量']
data_b.index = data_b.index.astype(int)
data_b_x = data_b.index
data_b_y = data_b.values
data_b_x
Int64Index([2013, 2014, 2015, 2016], dtype='int64', name='创建日期')
data_b_y = data_b_y.astype(int)
data_b_y
array([ 976024, 1760160, 2568675, 1182377])
plt.figure(figsize=(20,8),dpi=80)
plt.bar(x=data_b_x,
height = data_b_y,
width = 0.3,
color = 'hotpink',
bottom = 1,
linewidth = 0.2,
tick_label = data_b_x,
align = 'center'
)
plt.title('柱状图-每个年份的销售订单数量总和',pad=30,fontsize=14)
plt.xlabel('年份')
plt.ylabel('订单数量')
plt.grid()
for x, y in enumerate(data_b):
plt.text(x + 2013, y, "%s" %y,fontsize=12)
plt.show()
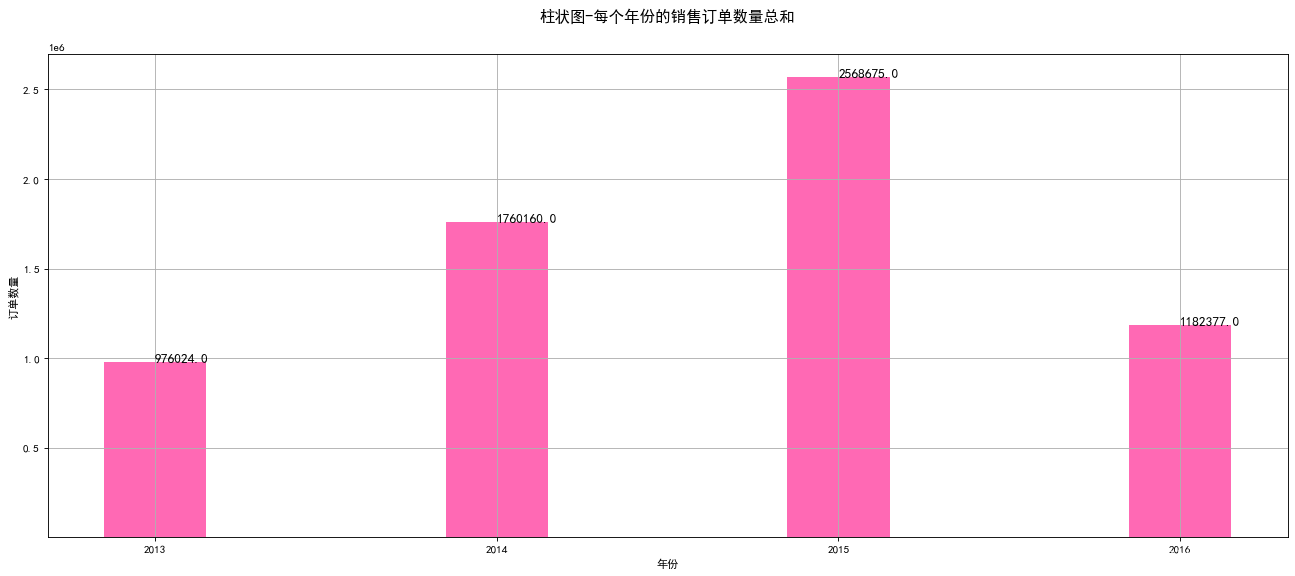
3、直方图
对于连续型变量,往往需要查看其分布图,直方图就是用来观察数据的分布形态 plt.hist(x,bins,range,normed,cumulative,bottom,align,rwidth,color,edgecolor,label)
- x: 数据
- bins: 条形个数
- range: 上下界
- normed: 是否将频数转换成频率
- cumulative: 是否计算累计频率
- bottom: 为直方图的每个条形添加基准线,默认为0
- align: 对齐方式
- rwidth: 条形的宽度
- color: 填充色
- edgecolor: 设置直方图边框
df = pd.read_csv(r'E:\python\Python数据分析与挖掘从零开始到实战\数据分析篇\学习笔记\数据\air_data.csv')
df.head()
| MEMBER_NO | FFP_DATE | FIRST_FLIGHT_DATE | GENDER | FFP_TIER | WORK_CITY | WORK_PROVINCE | WORK_COUNTRY | AGE | LOAD_TIME | ... | ADD_Point_SUM | Eli_Add_Point_Sum | L1Y_ELi_Add_Points | Points_Sum | L1Y_Points_Sum | Ration_L1Y_Flight_Count | Ration_P1Y_Flight_Count | Ration_P1Y_BPS | Ration_L1Y_BPS | Point_NotFlight |
---|
0 | 54993 | 2006/11/2 | 2008/12/24 | 男 | 6 | . | 北京 | CN | 31.0 | 2014/3/31 | ... | 39992 | 114452 | 111100 | 619760 | 370211 | 0.509524 | 0.490476 | 0.487221 | 0.512777 | 50 |
---|
1 | 28065 | 2007/2/19 | 2007/8/3 | 男 | 6 | NaN | 北京 | CN | 42.0 | 2014/3/31 | ... | 12000 | 53288 | 53288 | 415768 | 238410 | 0.514286 | 0.485714 | 0.489289 | 0.510708 | 33 |
---|
2 | 55106 | 2007/2/1 | 2007/8/30 | 男 | 6 | . | 北京 | CN | 40.0 | 2014/3/31 | ... | 15491 | 55202 | 51711 | 406361 | 233798 | 0.518519 | 0.481481 | 0.481467 | 0.518530 | 26 |
---|
3 | 21189 | 2008/8/22 | 2008/8/23 | 男 | 5 | Los Angeles | CA | US | 64.0 | 2014/3/31 | ... | 0 | 34890 | 34890 | 372204 | 186100 | 0.434783 | 0.565217 | 0.551722 | 0.448275 | 12 |
---|
4 | 39546 | 2009/4/10 | 2009/4/15 | 男 | 6 | 贵阳 | 贵州 | CN | 48.0 | 2014/3/31 | ... | 22704 | 64969 | 64969 | 338813 | 210365 | 0.532895 | 0.467105 | 0.469054 | 0.530943 | 39 |
---|
5 rows × 44 columns
plt.figure(figsize=(20,8),dpi=80)
plt.hist(df['AGE'],
bins=60,
color = 'chocolate',
edgecolor = 'black'
)
plt.title('直方图',fontsize=14)
plt.grid()
plt.xlabel('客户年龄',fontsize=14)
plt.ylabel('频数',fontsize=14)
plt.show()
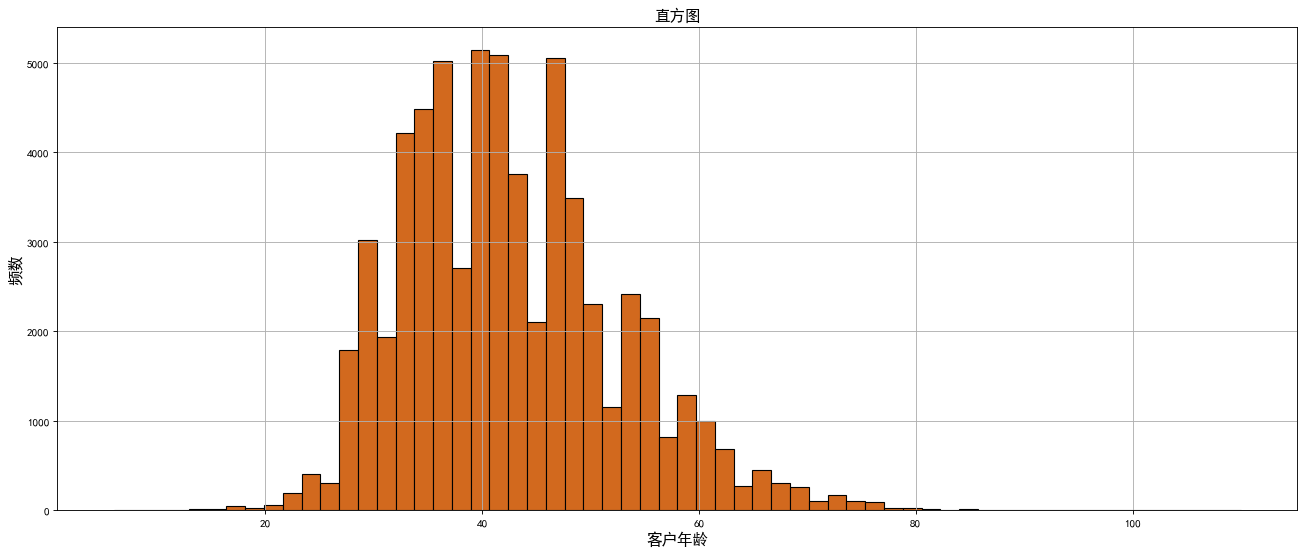
4、散点图
散点图一般用来展示2个连续型变量的的关系,可以通过散点图来判断两个变量之间是否存在某种关系,例如线性还是非线性关系
plt.scatter(x, y, s, c, marker, cmap, norm, alpha, linewidths, edgecolors)
- x: x数据
- y: y轴数据
- s: 散点大小
- c: 散点颜色
- marker: 散点图形状
- cmap: 指定某个colormap值,该参数一般不用,用默认值
- alpha: 散点的透明度
- linewidths: 散点边界线的宽度
- edgecolors: 设置散点边界线的颜色
df.head()
| MEMBER_NO | FFP_DATE | FIRST_FLIGHT_DATE | GENDER | FFP_TIER | WORK_CITY | WORK_PROVINCE | WORK_COUNTRY | AGE | LOAD_TIME | ... | ADD_Point_SUM | Eli_Add_Point_Sum | L1Y_ELi_Add_Points | Points_Sum | L1Y_Points_Sum | Ration_L1Y_Flight_Count | Ration_P1Y_Flight_Count | Ration_P1Y_BPS | Ration_L1Y_BPS | Point_NotFlight |
---|
0 | 54993 | 2006/11/2 | 2008/12/24 | 男 | 6 | . | 北京 | CN | 31.0 | 2014/3/31 | ... | 39992 | 114452 | 111100 | 619760 | 370211 | 0.509524 | 0.490476 | 0.487221 | 0.512777 | 50 |
---|
1 | 28065 | 2007/2/19 | 2007/8/3 | 男 | 6 | NaN | 北京 | CN | 42.0 | 2014/3/31 | ... | 12000 | 53288 | 53288 | 415768 | 238410 | 0.514286 | 0.485714 | 0.489289 | 0.510708 | 33 |
---|
2 | 55106 | 2007/2/1 | 2007/8/30 | 男 | 6 | . | 北京 | CN | 40.0 | 2014/3/31 | ... | 15491 | 55202 | 51711 | 406361 | 233798 | 0.518519 | 0.481481 | 0.481467 | 0.518530 | 26 |
---|
3 | 21189 | 2008/8/22 | 2008/8/23 | 男 | 5 | Los Angeles | CA | US | 64.0 | 2014/3/31 | ... | 0 | 34890 | 34890 | 372204 | 186100 | 0.434783 | 0.565217 | 0.551722 | 0.448275 | 12 |
---|
4 | 39546 | 2009/4/10 | 2009/4/15 | 男 | 6 | 贵阳 | 贵州 | CN | 48.0 | 2014/3/31 | ... | 22704 | 64969 | 64969 | 338813 | 210365 | 0.532895 | 0.467105 | 0.469054 | 0.530943 | 39 |
---|
5 rows × 44 columns
df['SEG_KM_SUM']
0 580717
1 293678
2 283712
3 281336
4 309928
...
62983 1134
62984 8016
62985 2594
62986 3934
62987 4222
Name: SEG_KM_SUM, Length: 62988, dtype: int64
plt.figure(figsize=(20,8),dpi=80)
plt.scatter(x=df['FLIGHT_COUNT'],
y=df['SEG_KM_SUM'],
s=50,
c='blue',
marker='o',
linewidths=1,
edgecolors='tan'
)
plt.title('飞行次数与总飞行公里数的关系',pad=10,fontsize=14)
plt.xlabel('飞行次数',fontsize=14,labelpad=10)
plt.ylabel('飞行公里数',fontsize=14,labelpad=10)
plt.show()
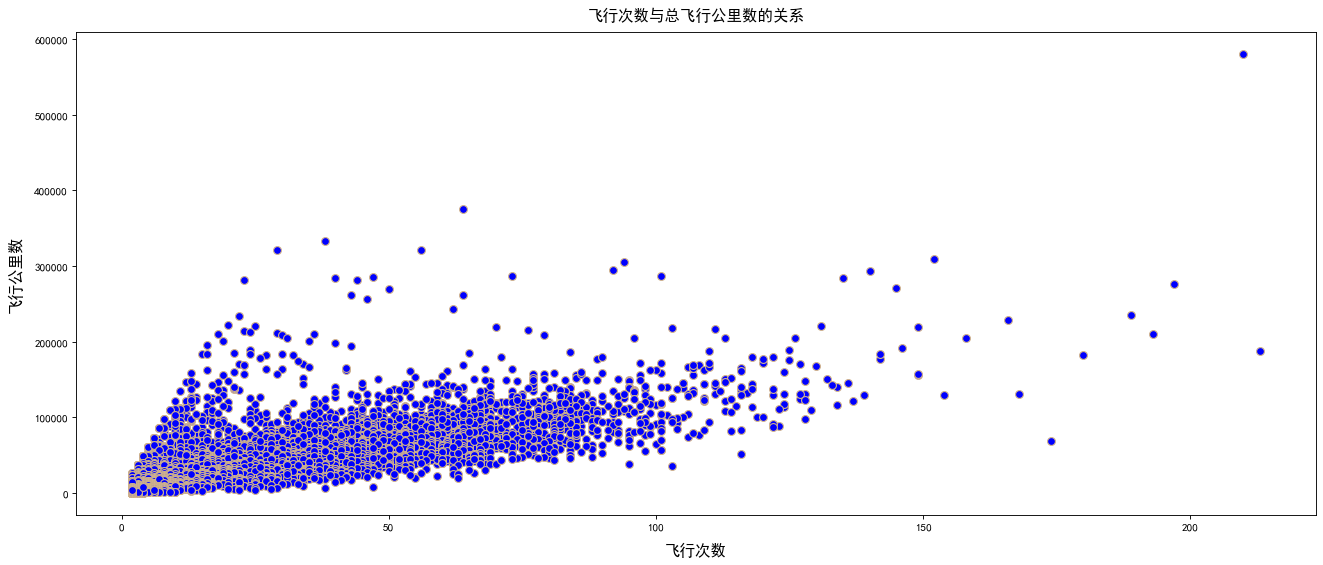
5、箱线图
箱线图是由一个箱体和一对箱须组成的统计图形。箱体是由第一四分位数,中位数和第三四分位数所组成的。在箱须的末端之外的数值可以理解成离群值,箱线图可以对一组数据范围大致进行直观的描述
plt.boxplot(x,notch,sym,vert,whis,positions,widths,patch_artist,meanline,showmeans, boxprops,labels,flierprops)
- x: 数据
- patch_artist: 是否填充箱体颜色
- meanline:是否显示均值
- showmeans: 是否显示均值
- meanprops;设置均值属性,如点的大小,颜色等
- medianprops:设置中位数的属性,如线的类型,大小等
- showfliers: 是否表示有异常值
- boxprops:设置箱体的属性,边框色和填充色
df['AGE'].isnull().any()
True
df['AGE'].isnull().sum()
420
age = df[df['AGE'].notnull()]['AGE']
plt.figure(figsize=(20,8),dpi=80)
plt.boxplot(age,
patch_artist=True,
meanline=True,
showmeans=True,
flierprops={'marker':'o','markerfacecolor':'red','markersize':5},
meanprops = {'marker':'o','markerfacecolor':'yellow','markersize':6},
medianprops = {'linestyle':'--','color':'orange'},
showfliers=True,
boxprops = {'color':'red','facecolor':'steelblue'}
)
plt.title('航空公司客户年龄分布箱线图',fontsize=14,pad=20)
plt.show()
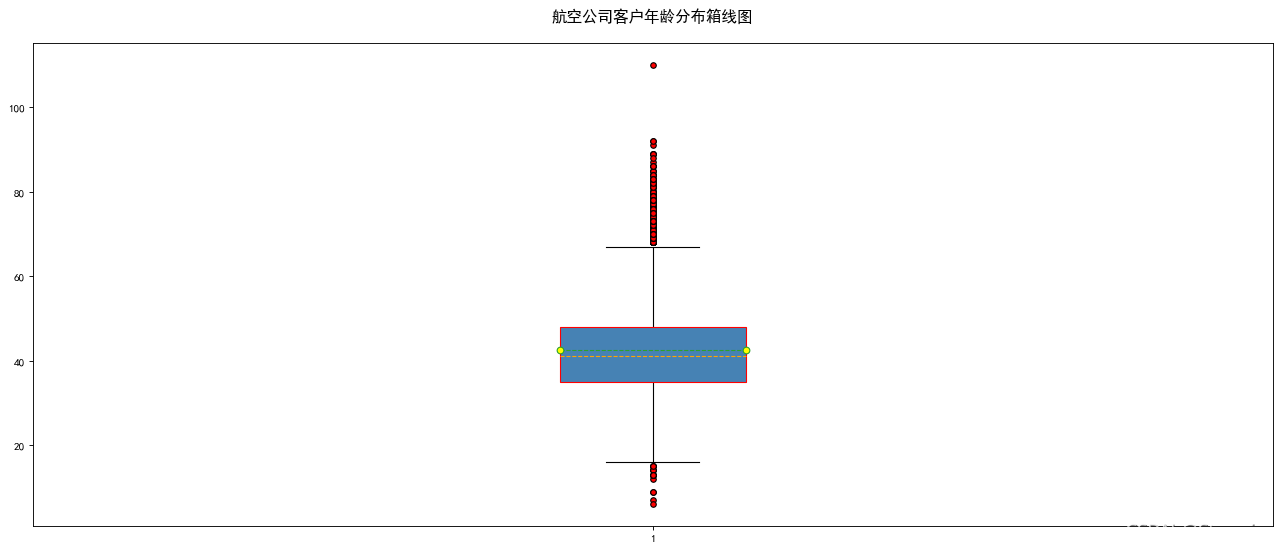
完善图形设置
df = pd.read_csv(r'E:\python\Python数据分析与挖掘从零开始到实战\数据分析篇\学习笔记\数据\air_data.csv')
df.head()
| MEMBER_NO | FFP_DATE | FIRST_FLIGHT_DATE | GENDER | FFP_TIER | WORK_CITY | WORK_PROVINCE | WORK_COUNTRY | AGE | LOAD_TIME | ... | ADD_Point_SUM | Eli_Add_Point_Sum | L1Y_ELi_Add_Points | Points_Sum | L1Y_Points_Sum | Ration_L1Y_Flight_Count | Ration_P1Y_Flight_Count | Ration_P1Y_BPS | Ration_L1Y_BPS | Point_NotFlight |
---|
0 | 54993 | 2006/11/2 | 2008/12/24 | 男 | 6 | . | 北京 | CN | 31.0 | 2014/3/31 | ... | 39992 | 114452 | 111100 | 619760 | 370211 | 0.509524 | 0.490476 | 0.487221 | 0.512777 | 50 |
---|
1 | 28065 | 2007/2/19 | 2007/8/3 | 男 | 6 | NaN | 北京 | CN | 42.0 | 2014/3/31 | ... | 12000 | 53288 | 53288 | 415768 | 238410 | 0.514286 | 0.485714 | 0.489289 | 0.510708 | 33 |
---|
2 | 55106 | 2007/2/1 | 2007/8/30 | 男 | 6 | . | 北京 | CN | 40.0 | 2014/3/31 | ... | 15491 | 55202 | 51711 | 406361 | 233798 | 0.518519 | 0.481481 | 0.481467 | 0.518530 | 26 |
---|
3 | 21189 | 2008/8/22 | 2008/8/23 | 男 | 5 | Los Angeles | CA | US | 64.0 | 2014/3/31 | ... | 0 | 34890 | 34890 | 372204 | 186100 | 0.434783 | 0.565217 | 0.551722 | 0.448275 | 12 |
---|
4 | 39546 | 2009/4/10 | 2009/4/15 | 男 | 6 | 贵阳 | 贵州 | CN | 48.0 | 2014/3/31 | ... | 22704 | 64969 | 64969 | 338813 | 210365 | 0.532895 | 0.467105 | 0.469054 | 0.530943 | 39 |
---|
5 rows × 44 columns
WORK_PROVINCE = df['WORK_PROVINCE'].value_counts()[:10]
legend(loc,bbox_to_anchor,ncol,title,shadow,fancybox)
- loc: 位置(可以使用数字)
- bbox_to_anchor: 该参数是一个4元元组,第一个元素代表距离画布左侧的y轴长度的倍数的距离,第二个元素代表距离画布底部X轴长度的倍数,第三个元素和第四个代表框的长度和和宽度
- frameon: 是否要边框
- ncol: 图例的排列个数
- title: 标题
- shadow: shadow线框是否添加阴影
- fancybox: 线框的圆角还是直角
fig = plt.figure(figsize=(20,8),dpi=80)
ax =fig.add_axes([0.2,0.4,0.8,0.7])
plt.plot(WORK_PROVINCE,color='g',marker='o',ls='--',label='注册用户数')
plt.xlabel('地区',fontsize=14,labelpad=10)
plt.ylabel('注册用户数',fontsize=14,labelpad=10)
plt.xticks(WORK_PROVINCE.index,fontsize=12,rotation=45)
plt.yticks(fontsize=12)
plt.title('航空公司注册用户折线图',fontsize=14,pad=10)
plt.legend(bbox_to_anchor=(1,1),loc='best',title='航空公司',frameon=True,shadow=True,fancybox=False,ncol=1)
plt.annotate('注册用户最多省份',xy=(0,17500), xytext=(2,15000),weight='bold',color='black',
arrowprops =dict(arrowstyle='->',connectionstyle = 'arc3',color='red'))
plt.show()
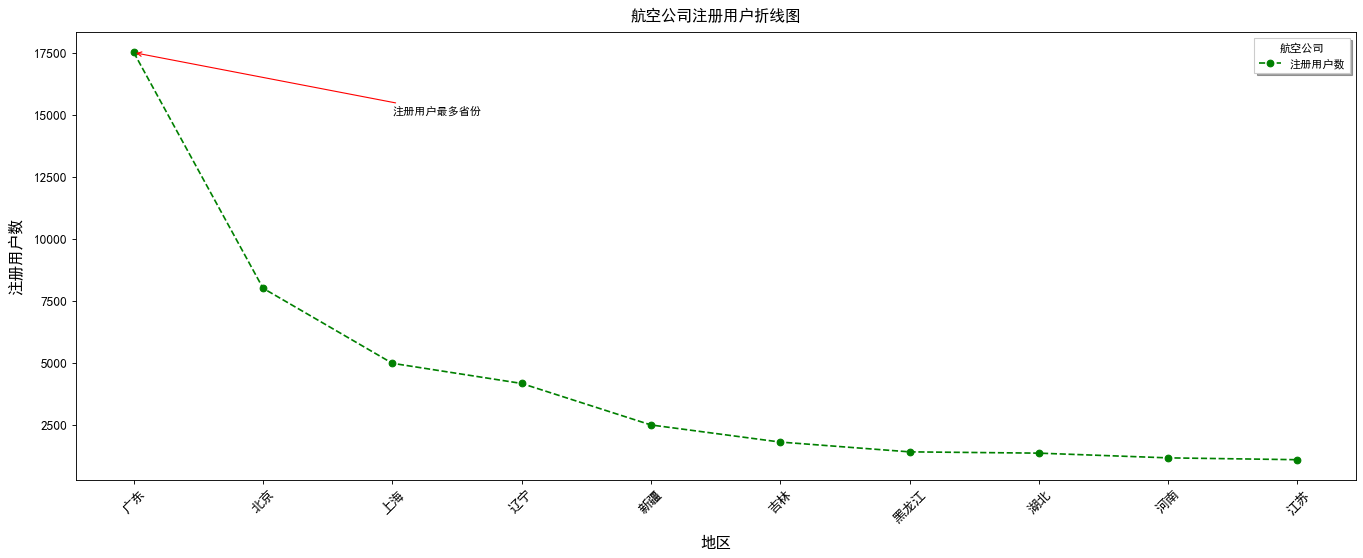
调整刻度值
data_vip = df.groupby(df['FFP_DATE']).size()
data_vip.index
Index(['2004/11/1', '2004/11/10', '2004/11/11', '2004/11/12', '2004/11/13',
'2004/11/14', '2004/11/15', '2004/11/16', '2004/11/17', '2004/11/18',
...
'2013/3/29', '2013/3/3', '2013/3/30', '2013/3/31', '2013/3/4',
'2013/3/5', '2013/3/6', '2013/3/7', '2013/3/8', '2013/3/9'],
dtype='object', name='FFP_DATE', length=3068)
import matplotlib as mpl
plt.figure(figsize=(20,8),dpi=80)
ax = plt.gca()
date_format = mpl.dates.DateFormatter("%Y-%m-%d")
ax.xaxis.set_major_formatter(date_format)
xlocator = mpl.ticker.LinearLocator(20)
ax.xaxis.set_major_locator(xlocator)
plt.plot(data_vip.index,
data_vip.values,
linestyle = '-',
linewidth = 2,
color = 'steelblue')
plt.xticks(rotation=45)
plt.ylabel('会员数量')
plt.title('不同年份会员数量变化趋势')
plt.show()
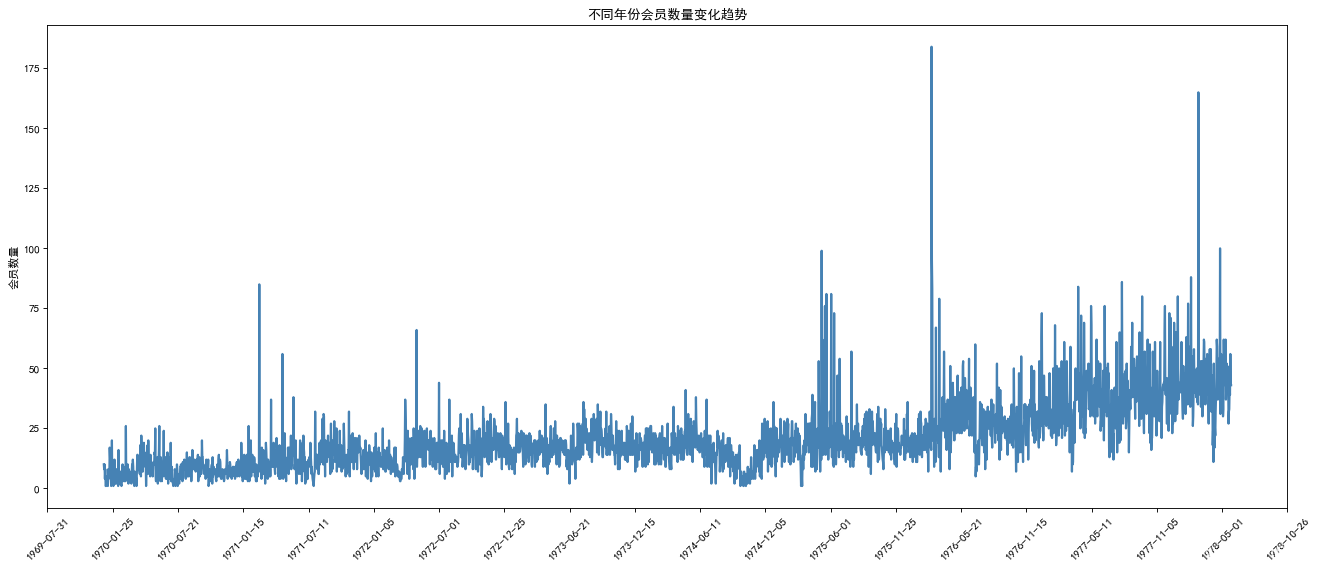
处理日期值
df['FFP_DATE'] = pd.to_datetime(df['FFP_DATE'],format='%Y/%m/%d',errors='coerce')
df['FFP_DATE']
0 2006-11-02
1 2007-02-19
2 2007-02-01
3 2008-08-22
4 2009-04-10
...
62983 2011-05-20
62984 2010-03-08
62985 2006-03-30
62986 2013-02-06
62987 2013-02-17
Name: FFP_DATE, Length: 62988, dtype: datetime64[ns]
df.columns
Index(['MEMBER_NO', 'FFP_DATE', 'FIRST_FLIGHT_DATE', 'GENDER', 'FFP_TIER',
'WORK_CITY', 'WORK_PROVINCE', 'WORK_COUNTRY', 'AGE', 'LOAD_TIME',
'FLIGHT_COUNT', 'BP_SUM', 'EP_SUM_YR_1', 'EP_SUM_YR_2', 'SUM_YR_1',
'SUM_YR_2', 'SEG_KM_SUM', 'WEIGHTED_SEG_KM', 'LAST_FLIGHT_DATE',
'AVG_FLIGHT_COUNT', 'AVG_BP_SUM', 'BEGIN_TO_FIRST', 'LAST_TO_END',
'AVG_INTERVAL', 'MAX_INTERVAL', 'ADD_POINTS_SUM_YR_1',
'ADD_POINTS_SUM_YR_2', 'EXCHANGE_COUNT', 'avg_discount',
'P1Y_Flight_Count', 'L1Y_Flight_Count', 'P1Y_BP_SUM', 'L1Y_BP_SUM',
'EP_SUM', 'ADD_Point_SUM', 'Eli_Add_Point_Sum', 'L1Y_ELi_Add_Points',
'Points_Sum', 'L1Y_Points_Sum', 'Ration_L1Y_Flight_Count',
'Ration_P1Y_Flight_Count', 'Ration_P1Y_BPS', 'Ration_L1Y_BPS',
'Point_NotFlight'],
dtype='object')
data_sum = df.groupby(df['FFP_DATE'].dt.year)[['FLIGHT_COUNT','SEG_KM_SUM']].sum()
data_sum
| FLIGHT_COUNT | SEG_KM_SUM |
---|
FFP_DATE | | |
---|
2004 | 8492 | 13046594 |
---|
2005 | 54584 | 76263647 |
---|
2006 | 74116 | 107466218 |
---|
2007 | 81398 | 115824690 |
---|
2008 | 80384 | 115958959 |
---|
2009 | 73515 | 102908088 |
---|
2010 | 92398 | 133804987 |
---|
2011 | 117870 | 170898377 |
---|
2012 | 135812 | 200675940 |
---|
2013 | 27172 | 41751371 |
---|
绘制双坐标轴
fig = plt.figure(figsize=(20,8),dpi=80)
ax1 = fig.add_subplot(111)
ax1.plot(data_sum.index, data_sum['FLIGHT_COUNT'],label='飞行次数')
ax1.set_ylabel('飞行次数')
ax1.set_title("飞行次数,飞行里程数与年份关系")
plt.legend(loc ='upper left')
ax2 = ax1.twinx()
ax2.plot(data_sum.index, data_sum['SEG_KM_SUM'], 'r',label='飞行里程数')
ax2.set_ylabel('飞行里程数')
plt.legend(loc ='upper right')
plt.show()
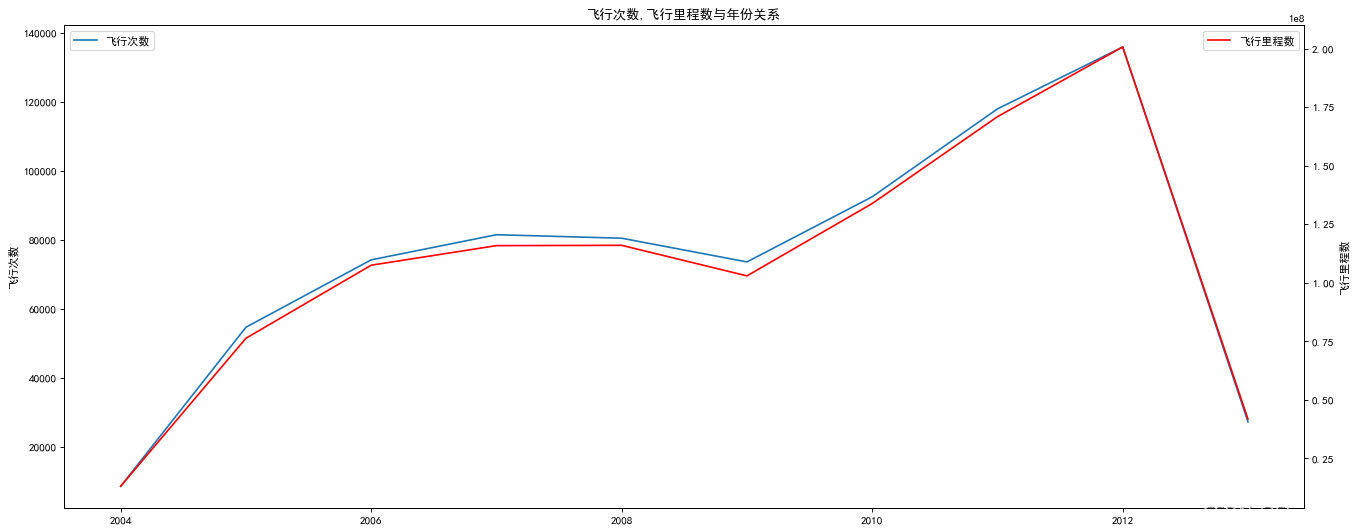
绘制子图
plt.figure(figsize=(20,8),dpi=80)
plt.subplot(121)
plt.bar(x = range(0,len(data_b)),height = data_b,align='center',color='y',tick_label= data_b.index)
plt.xlabel('年份',labelpad = 19)
plt.ylabel('订单数量总和',labelpad =10)
plt.title('不同年份的订单数量总和',pad=15)
plt.subplot(122)
plt.hist(x =df['AGE'],bins=30,color='r',edgecolor='black',density=True)
plt.xlabel('客户年龄',fontsize =15,labelpad =20)
plt.ylabel('频数',fontsize =15,labelpad =20)
plt.title('年龄分布图',fontsize =15,pad =20)
plt.show()
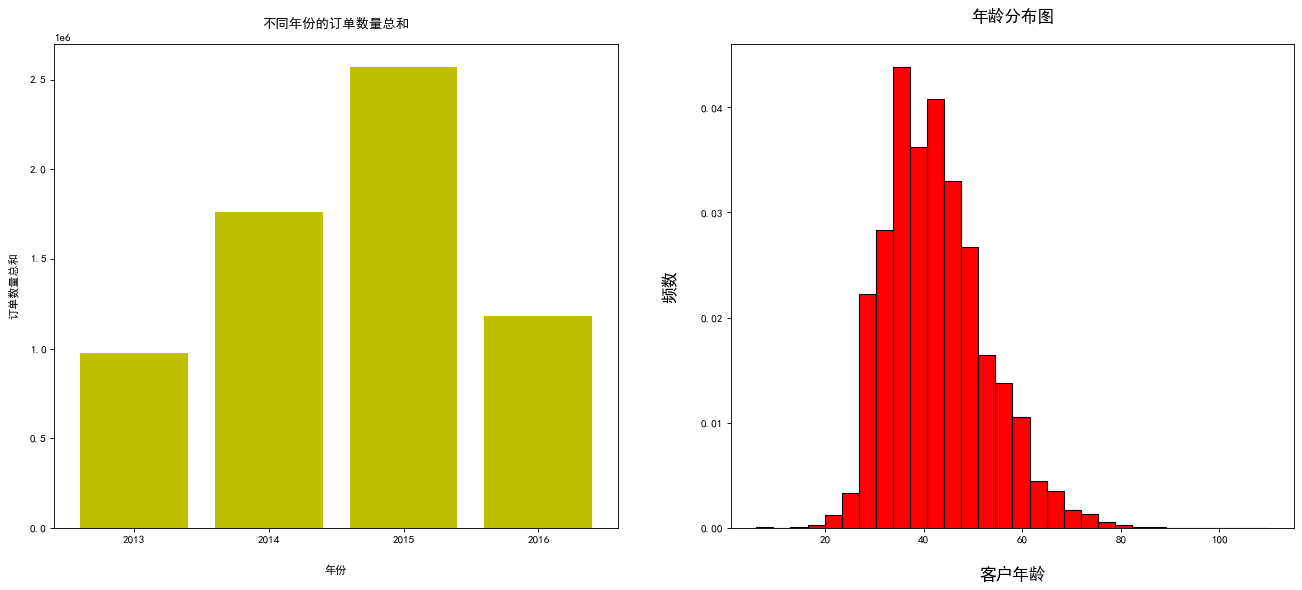
subplot2grid应用
- subplot2grid函数可以让子区跨越固定的网格布局的多个行和列,实现不同的子区布局
- plt.subplot2grid(shape,loc,colspan,rowspan) 参数
- shape: 网格布局
- loc: 表示图形的位置起点
- colspan: 跨越的列数
- rowspan : 跨越的行数
plt.figure(figsize=(16,9))
plt.subplot2grid((2,3),(0,0),colspan = 1,rowspan=2)
plt.bar(x = range(0,len(data_b)),height = data_b,align='center',color='y',tick_label= data_b.index)
plt.xlabel('年份',labelpad = 19)
plt.ylabel('订单数量总和',labelpad =10)
plt.title('不同年份的订单数量总和',pad=15)
plt.subplot2grid((2,3),(0,1),colspan =2,rowspan=1 )
plt.hist(x =df['AGE'],bins=30,color='r',edgecolor='black',density=True)
plt.xlabel('客户年龄',fontsize =15,labelpad =20)
plt.ylabel('频数',fontsize =15,labelpad =20)
plt.title('年龄分布图',fontsize =15,pad =20)
plt.subplot2grid((2,3),(1,1),colspan=2,rowspan=1)
plt.scatter(x = df['FLIGHT_COUNT'],y=df['SEG_KM_SUM'],color= 'steelblue',marker='o', s=100)
plt.xlabel('飞行次数',fontsize=12)
plt.ylabel('飞行总公里数',fontsize=12)
plt.title('飞行次数与总飞行公里数的关系')
plt.tight_layout()
plt.show()
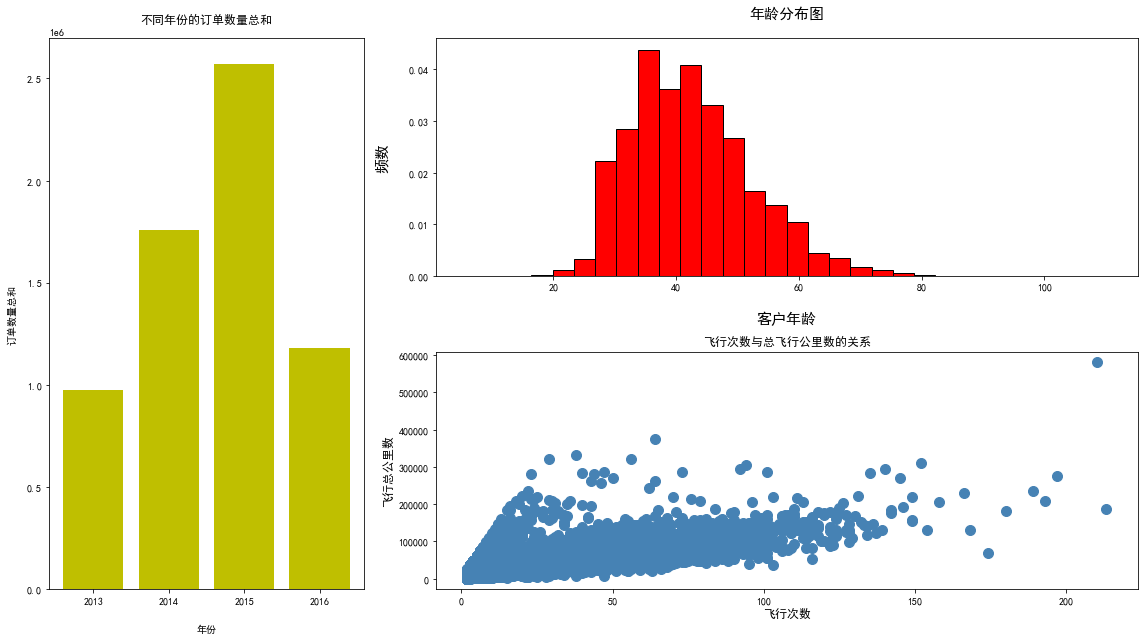
|