先上图:
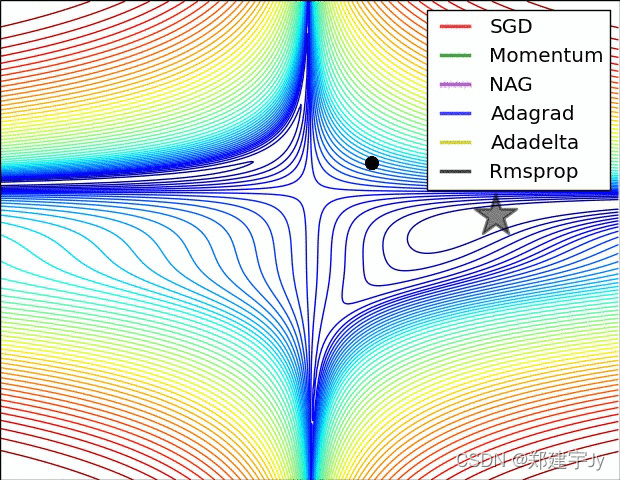 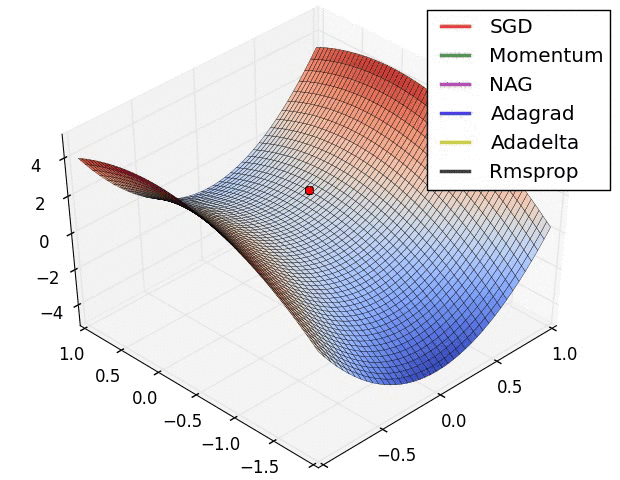
研一寒假导师要求我们几个把这两幅动图用程序画出来,当时搜遍了网上没找到源代码,甚至还去推特问了图的原作者,后来没登录过,不知道回没回我哈哈。下面是两幅图的代码,可能不是非常还原。然后可以自己改一下线条颜色注释等等,用matplotlib画图的基础知识可以看一下B站莫烦的视频,链接在这儿:【莫烦Python】Matplotlib Python 画图教程_哔哩哔哩_bilibili如果某天你发现自己要学习 Matplotlib, 很可能是因为:Matplotlib 是一个非常强大的 Python 画图工具;手中有很多数据, 可是不知道该怎么呈现这些数据.Code: https://github.com/MorvanZhou/tutorials/tree/master/matplotlibTUT莫烦Python: https://mofanpy.com支持莫烦: https: https://www.bilibili.com/video/BV1Jx411L7LU?spm_id_from=333.337.search-card.all.click
还有参考了几份博文的资料,也写得非常不错,放在这儿:《机器学习十讲》第七讲 - 公鸡不下蛋 - 博客园源地址(相关案例在视频下方): http://cookdata.cn/auditorium/course_room/10018/ 《机器学习十讲》——第七讲(最优化) 机器学习的优化目标 最小化损失函 https://www.cnblogs.com/lihaodeworld/p/14480929.htmlhttp://louistiao.me/notes/visualizing-and-animating-optimization-algorithms-with-matplotlib/#3D-Surface-Plot http://louistiao.me/notes/visualizing-and-animating-optimization-algorithms-with-matplotlib/#3D-Surface-Plot
?下面是代码:首先是最小值的
import matplotlib.pyplot as plt
import autograd.numpy as np
from mpl_toolkits.mplot3d import Axes3D
from matplotlib.colors import LogNorm
from matplotlib import animation
from IPython.display import HTML
from autograd import elementwise_grad, value_and_grad, grad
from scipy.optimize import minimize
from collections import defaultdict
from itertools import zip_longest
from functools import partial
f = lambda x, y: (1.5 - x + x * y) ** 2 + (2.25 - x + x * y ** 2) ** 2 + (2.625 - x + x * y ** 3) ** 2
xmin, xmax, xstep = -4.5, 4.5, .01
ymin, ymax, ystep = -4.5, 4.5, .01
x, y = np.meshgrid(np.arange(xmin, xmax + xstep, xstep), np.arange(ymin, ymax + ystep, ystep))
z = f(x, y)
minima = np.array([2.95, .5])
minima_ = minima.reshape(-1, 1)
x0 = np.array([1., 1.5])
def make_minimize_cb(path=[]):
def minimize_cb(xk):
path.append(np.copy(xk))
return minimize_cb
class TrajectoryAnimation(animation.FuncAnimation):
def __init__(self, *paths, labels=[], fig=None, ax=None, frames=None, interval=60, repeat_delay=5, blit=True,
**kwargs):
if fig is None:
if ax is None:
fig, ax = plt.subplots()
else:
fig = ax.get_figure()
else:
if ax is None:
ax = fig.gca()
self.fig = fig
self.ax = ax
self.paths = paths
if frames is None:
frames = max(path.shape[1] for path in paths)
self.lines = [ax.plot([], [], label=label, lw=2)[0]
for _, label in zip_longest(paths, labels)]
self.points = [ax.plot([], [], 'o', color=line.get_color())[0]
for line in self.lines]
super(TrajectoryAnimation, self).__init__(fig, self.animate, init_func=self.init_anim,
frames=frames, interval=interval, blit=blit,
repeat_delay=repeat_delay, **kwargs)
def init_anim(self):
for line, point in zip(self.lines, self.points):
line.set_data([], [])
point.set_data([], [])
x = self.lines + self.points
return x
def animate(self, i):
for line, point, path in zip(self.lines, self.points, self.paths):
line.set_data(*path[::, :i])
point.set_data(*path[::, i - 1:i])
x = self.lines + self.points
return x
methods = [
"SGD",
"Momentum",
"NAG",
"Adagrad",
"Adadelta",
"Rmsprop",
"Adam"
]
def SGDUpdate(function, x0, y0, learning_rate, num_steps):
allX = [x0]
allY = [y0]
x = x0
y = y0
for _ in range(num_steps):
dz_dx = grad(function, argnum=0)(x, y)
dz_dy = grad(function, argnum=1)(x, y)
x = x - dz_dx * learning_rate
y = y - dz_dy * learning_rate
allX.append(x)
allY.append(y)
return np.array([allX, allY])
def MomentumUpdate(function, x0, y0, learning_rate, num_steps, momentum=0.9):
allX = [x0]
allY = [y0]
x = x0
y = y0
x_v = 0
y_v = 0
for _ in range(num_steps):
dz_dx = grad(function, argnum=0)(x, y)
dz_dy = grad(function, argnum=1)(x, y)
x_v = (momentum * x_v) - (dz_dx * learning_rate)
y_v = (momentum * y_v) - (dz_dy * learning_rate)
x = x + x_v
y = y + y_v
allX.append(x)
allY.append(y)
return np.array([allX, allY])
def NAGUpdate(function, x0, y0, learning_rate, num_steps, momentum=0.9):
allX = [x0]
allY = [y0]
x = x0
y = y0
x_v = 0
x_v_prev = 0
y_v = 0
y_v_prev = 0
for _ in range(num_steps):
dz_dx = grad(function, argnum=0)(x, y)
dz_dy = grad(function, argnum=1)(x, y)
x_v_prev = x_v
x_v = (momentum * x_v) - (dz_dx * learning_rate)
x = x - momentum * x_v_prev + (1 + momentum) * x_v
y_v_prev = y_v
y_v = (momentum * y_v) - (dz_dy * learning_rate)
y = y - momentum * y_v_prev + (1 + momentum) * y_v
allX.append(x)
allY.append(y)
return np.array([allX, allY])
def AdagradUpdate(function, x0, y0, learning_rate, num_steps):
allX = [x0]
allY = [y0]
x = x0
y = y0
x_cache = 0
y_cache = 0
for _ in range(num_steps):
dz_dx = grad(function, argnum=0)(x, y)
dz_dy = grad(function, argnum=1)(x, y)
x_cache = x_cache + dz_dx ** 2
x = x - learning_rate * dz_dx / (np.sqrt(x_cache) + 1e-7)
y_cache = y_cache + dz_dy ** 2
y = y - learning_rate * dz_dy / (np.sqrt(y_cache) + 1e-7)
allX.append(x)
allY.append(y)
return np.array([allX, allY])
def AdadeltaUpdate(function, x0, y0, learning_rate, num_steps, decay_rate=0.9):
allX = [x0]
allY = [y0]
x = x0
y = y0
x_cache = 0
y_cache = 0
for _ in range(num_steps):
dz_dx = grad(function, argnum=0)(x, y)
dz_dy = grad(function, argnum=1)(x, y)
x_cache = decay_rate * x_cache + (1 - decay_rate) * dz_dx ** 2
x = x - learning_rate * dz_dx / (np.sqrt(x_cache) + 1e-7)
y_cache = decay_rate * y_cache + (1 - decay_rate) * dz_dy ** 2
y = y - learning_rate * dz_dy / (np.sqrt(y_cache) + 1e-7)
allX.append(x)
allY.append(y)
return np.array([allX, allY])
def RmspropUpdate(function, x0, y0, learning_rate, num_steps, decay_rate=0.9):
allX = [x0]
allY = [y0]
x = x0
y = y0
x_cache = 0
y_cache = 0
for _ in range(num_steps):
dz_dx = grad(function, argnum=0)(x, y)
dz_dy = grad(function, argnum=1)(x, y)
x_cache = decay_rate * x_cache + (1 - decay_rate) * dz_dx ** 2
x = x - learning_rate * dz_dx / (np.sqrt(x_cache) + 1e-7)
y_cache = decay_rate * y_cache + (1 - decay_rate) * dz_dy ** 2
y = y - learning_rate * dz_dy / (np.sqrt(y_cache) + 1e-7)
allX.append(x)
allY.append(y)
return np.array([allX, allY])
def AdamUpdate(function, x0, y0, learning_rate, num_steps, beta1, beta2):
allX = [x0]
allY = [y0]
x = x0
y = y0
m_x = 0
m_y = 0
x_v = 0
y_v = 0
for step in range(1, num_steps + 1):
dz_dx = grad(function, argnum=0)(x, y)
dz_dy = grad(function, argnum=1)(x, y)
m_x = beta1 * m_x + (1 - beta1) * dz_dx
m_step_x = m_x / (1 - beta1 ** step)
x_v = beta2 * x_v + (1 - beta2) * (dz_dx ** 2)
v_step_x = x_v / (1 - beta2 ** step)
x = x - learning_rate * m_step_x / (np.sqrt(v_step_x) + 1e-8)
m_y = beta1 * m_y + (1 - beta1) * dz_dy
m_step_y = m_y / (1 - beta1 ** step)
y_v = beta2 * y_v + (1 - beta2) * (dz_dy ** 2)
v_step_y = y_v / (1 - beta2 ** step)
y = y - learning_rate * m_step_y / (np.sqrt(v_step_y) + 1e-8)
allX.append(x)
allY.append(y)
return np.array([allX, allY])
learning_rate = 0.003
num_steps=100
SGDPath = SGDUpdate(f, x0[0], x0[1], learning_rate, num_steps)
MomentumPath = MomentumUpdate(f, x0[0], x0[1], learning_rate, num_steps)
NAGPath = NAGUpdate(f, x0[0], x0[1], learning_rate, num_steps)
AdagradPath = AdagradUpdate(f, x0[0], x0[1], 0.5, num_steps)
AdadeltaPath = AdadeltaUpdate(f, x0[0], x0[1], 0.1, num_steps=70)
RmspropPath = RmspropUpdate(f, x0[0], x0[1], 0.12, num_steps=80)
AdamPath = AdamUpdate(f, x0[0], x0[1], 0.1, num_steps, 0.9, 0.999)
paths = [SGDPath, MomentumPath, NAGPath, AdagradPath, AdadeltaPath,RmspropPath, AdamPath]
fig, ax = plt.subplots(figsize=(10, 6))
ax.contour(x, y, z, levels=np.logspace(0, 5, 35), norm=LogNorm(), cmap=plt.cm.jet)
ax.plot(*minima_, 'r*', markersize=24)
ax.set_xlabel('$x$')
ax.set_ylabel('$y$')
ax.set_xlim((xmin, xmax))
ax.set_ylim((ymin, ymax))
anim = TrajectoryAnimation(*paths, labels=methods, ax=ax)
ax.legend(loc='upper right')
plt.show()
下面是鞍点的:
#先引入算法相关的包,matplotlib用于绘图
# %matplotlib inline
import matplotlib.pyplot as plt
import numpy as np
import torch
from torch.autograd import Variable
from mpl_toolkits.mplot3d import Axes3D
from matplotlib import animation
from IPython.display import HTML
from autograd import elementwise_grad, value_and_grad,grad
from scipy.optimize import minimize
from scipy import optimize
from collections import defaultdict
from itertools import zip_longest
plt.rcParams['axes.unicode_minus']=False # 用来正常显示负号
#使用python的匿名函数定义目标函数
f = lambda x,y : -x**2 + 2.5*y**2 #函数定义
f_grad = value_and_grad(lambda args : f(*args)) #函数梯度
#绘制函数曲面
##先借助np.meshgrid生成网格点坐标矩阵。两个维度上每个维度显示范围为-5到5。对应网格点的函数值保存在z中
x,y = np.meshgrid(np.linspace(-5.0,5.0,50), np.linspace(-5.0,5.0,50))
z = f( x,y )
minima = np.array([4, 0])
minima_ = minima.reshape(-1, 1)
##plot_surface函数绘制3D曲面
x0 = np.array([0.0000001, 2.])
def make_minimize_cb(path=[]):
def minimize_cb(xk):
path.append(np.copy(xk))
return minimize_cb
class TrajectoryAnimation3D(animation.FuncAnimation):
def __init__(self, *paths, zpaths, labels=[], fig=None, ax=None, frames=None,
interval=60, repeat_delay=5, blit=True, **kwargs):
if fig is None:
if ax is None:
fig, ax = plt.subplots()
else:
fig = ax.get_figure()
else:
if ax is None:
ax = fig.gca()
self.fig = fig
self.ax = ax
self.paths = paths
self.zpaths = zpaths
if frames is None:
frames = max(path.shape[1] for path in paths)
self.lines = [ax.plot([], [], [], label=label, lw=2)[0]
for _, label in zip_longest(paths, labels)]
super(TrajectoryAnimation3D, self).__init__(fig, self.animate, init_func=self.init_anim,
frames=frames, interval=interval, blit=blit,
repeat_delay=repeat_delay, **kwargs)
def init_anim(self):
for line in self.lines:
line.set_data([], [])
line.set_3d_properties([])
return self.lines
def animate(self, i):
for line, path, zpath in zip(self.lines, self.paths, self.zpaths):
line.set_data(*path[::,:i])
line.set_3d_properties(zpath[:i])
return self.lines
methods = [
"SGD",
"Momentum",
"NAG",
"Adagrad",
"Adadelta",
"Rmsprop",
"Adam"
]
def SGDUpdate(function, x0, y0, learning_rate, num_steps):
allX = [x0]
allY = [y0]
x = x0
y = y0
for _ in range(num_steps):
dz_dx = grad(function, argnum=0)(x, y)
dz_dy = grad(function, argnum=1)(x, y)
x = x - dz_dx * learning_rate
y = y - dz_dy * learning_rate
allX.append(x)
allY.append(y)
return np.array([allX, allY])
def MomentumUpdate(function, x0, y0, learning_rate, num_steps, momentum=0.9):
allX = [x0]
allY = [y0]
x = x0
y = y0
x_v = 0
y_v = 0
for _ in range(num_steps):
dz_dx = grad(function, argnum=0)(x, y)
dz_dy = grad(function, argnum=1)(x, y)
x_v = (momentum * x_v) - (dz_dx * learning_rate)
y_v = (momentum * y_v) - (dz_dy * learning_rate)
x = x + x_v
y = y + y_v
allX.append(x)
allY.append(y)
return np.array([allX, allY])
def NAGUpdate(function, x0, y0, learning_rate, num_steps, momentum=0.9):
allX = [x0]
allY = [y0]
x = x0
y = y0
x_v = 0
x_v_prev = 0
y_v = 0
y_v_prev = 0
for _ in range(num_steps):
dz_dx = grad(function, argnum=0)(x, y)
dz_dy = grad(function, argnum=1)(x, y)
x_v_prev = x_v
x_v = (momentum * x_v) - (dz_dx * learning_rate)
x = x - momentum * x_v_prev + (1 + momentum) * x_v
y_v_prev = y_v
y_v = (momentum * y_v) - (dz_dy * learning_rate)
y = y - momentum * y_v_prev + (1 + momentum) * y_v
allX.append(x)
allY.append(y)
return np.array([allX, allY])
def AdagradUpdate(function, x0, y0, learning_rate, num_steps):
allX = [x0]
allY = [y0]
x = x0
y = y0
x_cache = 0
y_cache = 0
for _ in range(num_steps):
dz_dx = grad(function, argnum=0)(x, y)
dz_dy = grad(function, argnum=1)(x, y)
x_cache = x_cache + dz_dx ** 2
x = x - learning_rate * dz_dx / (np.sqrt(x_cache) + 1e-7)
y_cache = y_cache + dz_dy ** 2
y = y - learning_rate * dz_dy / (np.sqrt(y_cache) + 1e-7)
allX.append(x)
allY.append(y)
return np.array([allX, allY])
def AdadeltaUpdate(function, x0, y0, learning_rate, num_steps, decay_rate=0.9):
allX = [x0]
allY = [y0]
x = x0
y = y0
x_cache = 0
y_cache = 0
for _ in range(num_steps):
dz_dx = grad(function, argnum=0)(x, y)
dz_dy = grad(function, argnum=1)(x, y)
x_cache = decay_rate * x_cache + (1 - decay_rate) * dz_dx ** 2
x = x - learning_rate * dz_dx / (np.sqrt(x_cache) + 1e-7)
y_cache = decay_rate * y_cache + (1 - decay_rate) * dz_dy ** 2
y = y - learning_rate * dz_dy / (np.sqrt(y_cache) + 1e-7)
allX.append(x)
allY.append(y)
return np.array([allX, allY])
def RmspropUpdate(function, x0, y0, learning_rate, num_steps, decay_rate=0.9):
allX = [x0]
allY = [y0]
x = x0
y = y0
x_cache = 0
y_cache = 0
for _ in range(num_steps):
dz_dx = grad(function, argnum=0)(x, y)
dz_dy = grad(function, argnum=1)(x, y)
x_cache = decay_rate * x_cache + (1 - decay_rate) * dz_dx ** 2
x = x - learning_rate * dz_dx / (np.sqrt(x_cache) + 1e-7)
y_cache = decay_rate * y_cache + (1 - decay_rate) * dz_dy ** 2
y = y - learning_rate * dz_dy / (np.sqrt(y_cache) + 1e-7)
allX.append(x)
allY.append(y)
return np.array([allX, allY])
def AdamUpdate(function, x0, y0, learning_rate, num_steps, beta1, beta2):
allX = [x0]
allY = [y0]
x = x0
y = y0
m_x = 0
m_y = 0
x_v = 0
y_v = 0
for step in range(1, num_steps + 1):
dz_dx = grad(function, argnum=0)(x, y)
dz_dy = grad(function, argnum=1)(x, y)
m_x = beta1 * m_x + (1 - beta1) * dz_dx
m_step_x = m_x / (1 - beta1 ** step)
x_v = beta2 * x_v + (1 - beta2) * (dz_dx ** 2)
v_step_x = x_v / (1 - beta2 ** step)
x = x - learning_rate * m_step_x / (np.sqrt(v_step_x) + 1e-8)
m_y = beta1 * m_y + (1 - beta1) * dz_dy
m_step_y = m_y / (1 - beta1 ** step)
y_v = beta2 * y_v + (1 - beta2) * (dz_dy ** 2)
v_step_y = y_v / (1 - beta2 ** step)
y = y - learning_rate * m_step_y / (np.sqrt(v_step_y) + 1e-8)
allX.append(x)
allY.append(y)
return np.array([allX, allY])
learning_rate = 0.01
num_steps=200
SGDPath = SGDUpdate(f, x0[0], x0[1], learning_rate, num_steps)
MomentumPath = MomentumUpdate(f, x0[0], x0[1], 0.05, num_steps)
NAGPath = NAGUpdate(f, x0[0], x0[1], 0.1, num_steps)
AdagradPath = AdagradUpdate(f, x0[0], x0[1], 0.5, num_steps)
AdadeltaPath = AdadeltaUpdate(f, x0[0], x0[1], 0.1, num_steps=70)
RmspropPath = RmspropUpdate(f, x0[0], x0[1], 0.1, num_steps=80)
AdamPath = AdamUpdate(f, x0[0], x0[1], 0.1, num_steps, 0.9, 0.999)
paths = [SGDPath, MomentumPath, NAGPath, AdagradPath, AdadeltaPath,RmspropPath, AdamPath]
zpaths = [f(*path) for path in paths]
fig = plt.figure(figsize=(8, 8))
ax = plt.axes(projection='3d', elev=50, azim=-50)
ax.plot_surface(x,y, z, alpha=.7, cmap='coolwarm')
ax.plot([minima[0]],[minima[1]],[f(*minima)], 'b*', markersize=6)
ax.set_xlabel('$x1$')
ax.set_ylabel('$y$')
ax.set_zlabel('$f$')
ax.set_xlim((-5, 5))
ax.set_ylim((-5, 5))
anim = TrajectoryAnimation3D(*paths, zpaths=zpaths, labels=methods, ax=ax)
ax.legend(loc='upper left')
plt.show()
注:运行在pycharm-pytorch3.9,如果出现No module named XXXX'的问题,可以左上角
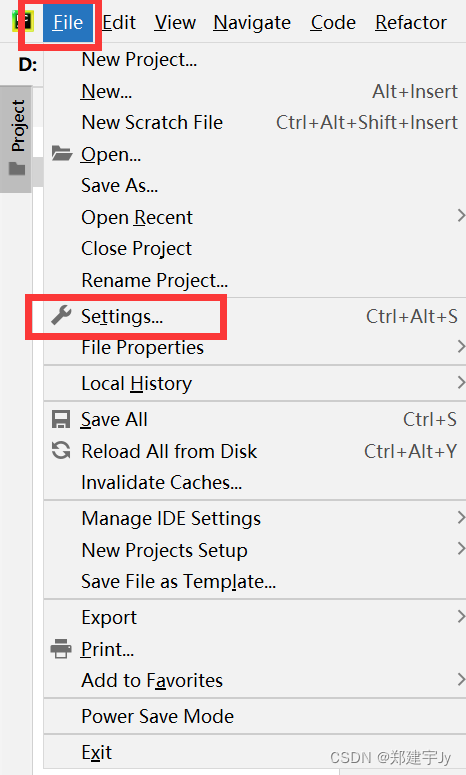
?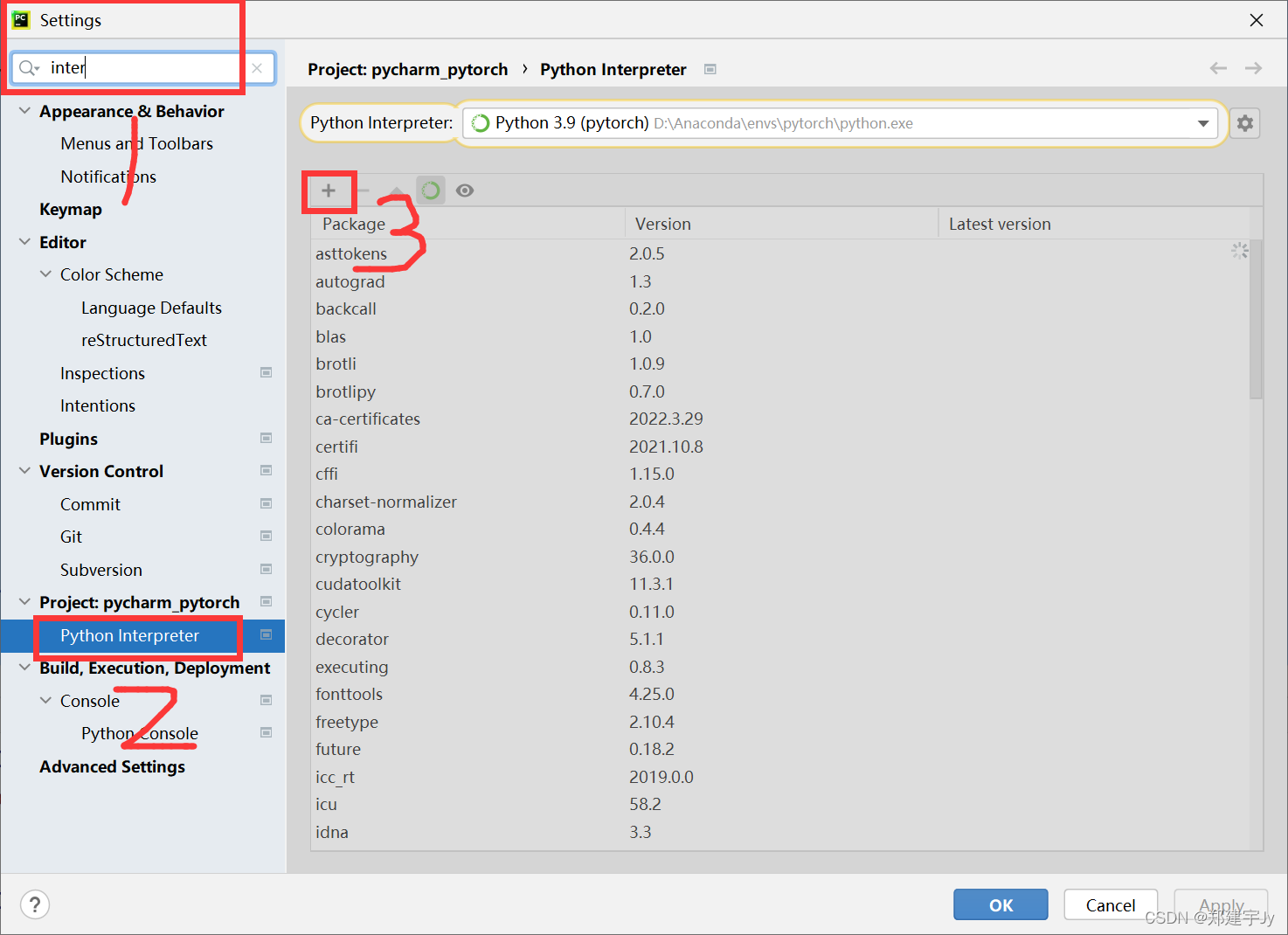
?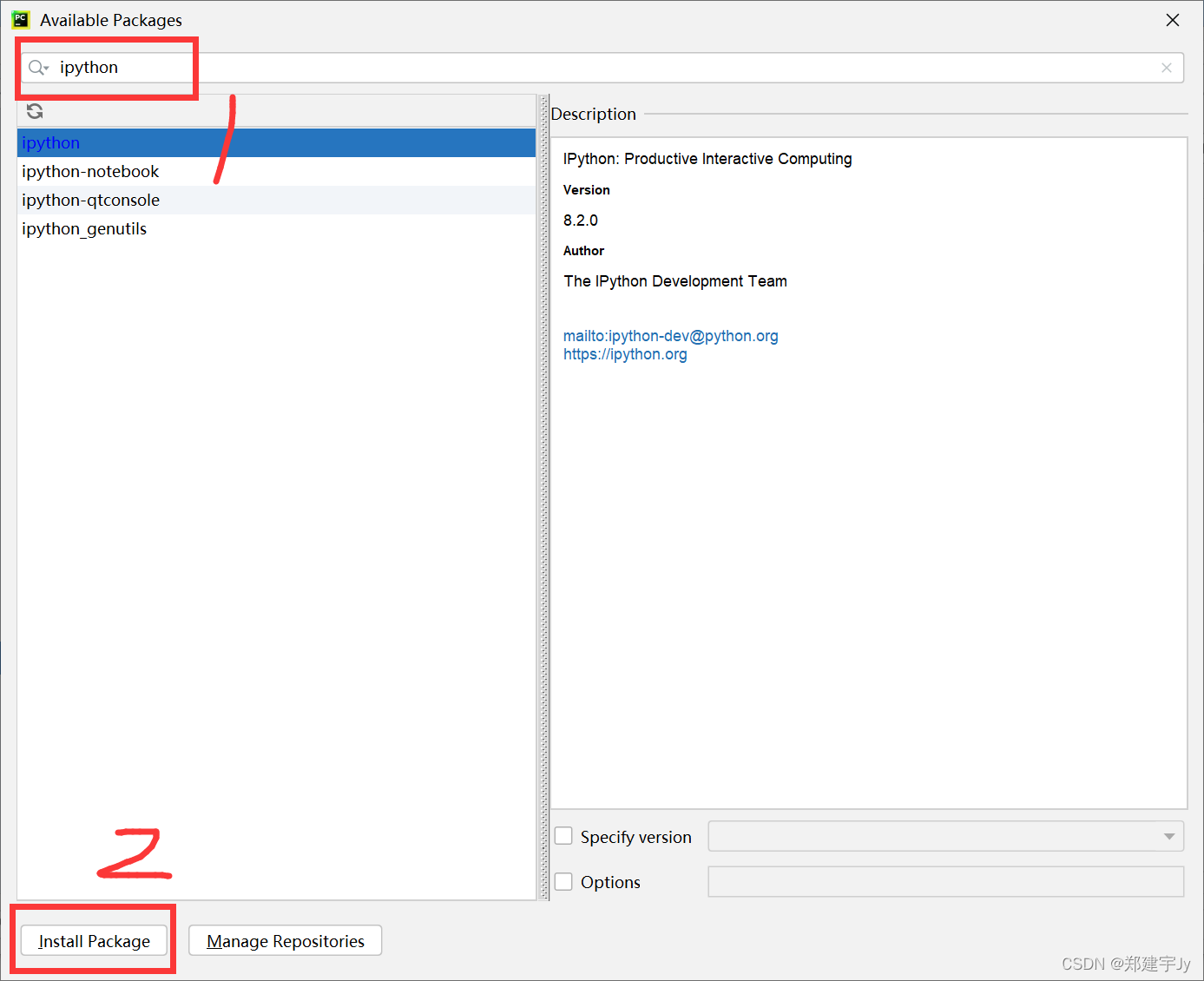
|