目录
1. 多进程/多线程/协程asyncio的简单使用
多进程
多线程
asyncio异步IO
2.进程池/线程池/协程并发的使用
进程池
multiprocessing.Pool
ProcessPoolExecutor
线程池
asyncio异步IO
使用python并发执行任务首先想到的是使用多线程和多进程,或者是协程asyncio,本篇文章做一下使用的总结。
使用场景说明一下:
- 多进程:适用于计算密集型的任务,比如要进行大量的运算,或者多cpu计算依赖较多的任务
- 多线程:适用于IO密集型的任务,比如说网络请求、文件读写这些比较耗时的任务。
- asyncio:使用场景同多线程,因为asycnio本质上是在单线程中执行的。
- 在使用线程池/进程池的时候,往往需要设置池的数量,一般默认情况是cpu的核数,可以使用os.cpu_count()获取
1. 多进程/多线程/协程asyncio的简单使用
多进程
Python 实现多进程的模块最常用的是multiprocessing, 一个简单的多进程程序示例是:
from multiprocessing import Process
def bar(name):
print('bar: ', name)
def foo(name):
print('foo: ', name)
if __name__ == '__main__':
p1 = Process(target=foo, args=('hello',))
p2 = Process(target=bar, args=('hello',))
p1.start()
p2.start()
p1.join()
p2.join()
join方法会阻塞主进程直到该进程完成,但不会阻塞其他进程,与之相反的方法是设置守护进程p1.daemon,主进程退出后该子进程也随之退出,即时它还在执行中。
多线程
使用方式和多进程差不多,只是导入的库不一样,Python 实现多线程的模块最常用的是threading, 一个简单的多线程程序示例是:
import time
from threading import Thread
def bar(name):
time.sleep(2)
print('bar: ', name)
def foo(name):
time.sleep(2)
print('foo: ', name)
if __name__ == '__main__':
p1 = Thread(target=foo, args=('hello',))
p2 = Thread(target=bar, args=('hello',))
p1.start()
p2.start()
p1.join()
p2.join()
asyncio异步IO
asyncio 是用来编写?异步 I/O并发?代码的库,使用?async/await?语法。
import asyncio
async def bar(name):
await asyncio.sleep(2)
print('bar: ', name)
async def foo(name):
await asyncio.sleep(2)
print('foo: ', name)
async def main():
task1 = asyncio.create_task(bar('hello'))
task2 = asyncio.create_task(foo('hello'))
# 两个任务并发执行
await task1
await task2
# Python 3.7+
asyncio.run(main())
2.进程池/线程池/协程并发的使用
进程池
进程池最常用的模块是multiprocessing.Pool,还有一个用法是使用内置模块ProcessPoolExecutor,该模块是对multiprocessing的高级封装。
multiprocessing.Pool
multiprocessing.Pool(processes=None, initializer=None, initargs=(), maxtasksperchild=None)
参数
processes :进程数量,如果?processes ?是?None 那么使用?os.cpu_count() 返回的数量或者为1initializer : 如果?initializer 不是?None ,那么每一个工作进程在开始的时候会调用initializer(*initargs) maxtasksperchild :工作进程退出之前可以完成的任务数,完成后用一个新的工作进程来替代原进程,来让闲置的资源被释放。maxtasksperchild ?默认是None ,意味着只要Pool 存在工作进程就会一直存活context : 用在制定工作进程启动时的上下文,一般使用?multiprocessing.Pool() ?或者一个context 对象的Pool() 方法来创建一个池,两种方法都适当的设置了context
注意,进程池对象的方法只有创建它的进程能够调用。
警告
multiprocessing.pool?对象具有需要正确管理的内部资源 (像任何其他资源一样),具体方式是将进程池用作上下文管理器,或者手动调用?close()?和?terminate()。 未做此类操作将导致进程在终结阶段挂起。
请注意依赖垃圾回收器来销毁进程池是?不正确的?做法,因为 CPython 并不保证进程池终结器会被调用。
实例方法
apply() :同步阻塞执行,上一个子进程结束后才能进行下一个子进程(不推荐)apply_async() :异步非阻塞执行,每个子进程都是异步执行的(并行)(推荐)map() :同步阻塞map_async() :异步非阻塞imap() :内存不够用可以采用此种方式,速度慢于?map() imap_unordered :imap() ?的无序版本(不会按照调用顺序返回,而是按照结束顺序返回),返回迭代器实例
一个多进程的使用示例
from multiprocessing import Pool
def bar(name):
print("bar: ", name)
data = []
def callback(result):
data.append(result)
def foo(x):
xx = x * x
data.append(xx)
return xx
if __name__ == '__main__':
with Pool(processes=8) as pool:
# 单个传参并发apply_async
res = pool.apply_async(foo, (10,), callback=callback)
print(res.get(timeout=1)) # 100
# 迭代对象传参并发map_async
res = pool.map_async(foo, range(3), callback=callback)
print(res.get(timeout=1)) # [0, 1, 4, 9, 16]
print(data) # [100, [0, 1, 4, 9, 16]]
res = pool.apply_async(bar, ("hello",)) # bar: hello
ProcessPoolExecutor
concurrent.futures ?是 python3.2 ?中引入的新模块,它为异步执行可调用对象提供了高层接口,分为两类:
ThreadPoolExecutor :多线程编程ProcessPoolExecutor :多进程编程
两者实现了同样的接口,这些接口由抽象类?Executor ?定义;这个模块提供了两大类型:
Executor :执行器,用于管理工作池Future :管理工作计算出的结果
实例方法
- submit(fn, *args, **kwargs): 将参数提交给fn函数执行,返回 Future 对象,可用 future.result() 获取执行结果
map (func,?*iterables,?timeout=None,?chunksize=1):将可迭代对象提交给func函数立即并发执行shutdown (wait=True,?*,?cancel_futures=False):当待执行的 future 对象完成执行后向执行者发送信号,它就会释放正在使用的任何资源。
?以下例子使用进程池计算PRIMES列表中的数字是否是质数
import concurrent.futures
import math
PRIMES = [
112272535095293,
112582705942171,
112272535095293,
115280095190773,
115797848077099,
1099726899285419]
def is_prime(n):
if n < 2:
return False
if n == 2:
return True
if n % 2 == 0:
return False
sqrt_n = int(math.floor(math.sqrt(n)))
for i in range(3, sqrt_n + 1, 2):
if n % i == 0:
return False
return True
def main():
with concurrent.futures.ProcessPoolExecutor() as executor:
for number, prime in zip(PRIMES, executor.map(is_prime, PRIMES)):
print('%d is prime: %s' % (number, prime))
if __name__ == '__main__':
main()
线程池
线程池我们使用的是ThreadPoolExecutor 模块,跟?ProcessPoolExecutor的使用方式差不多一样
一个线程池案例:访问多个url网页,并获取网页内容返回
import concurrent.futures
import urllib.request
URLS = ['http://www.baidu.com/',
'http://www.taobao.com/',
'http://www.csdn.net/',
'http://www.openeuler.org/']
# 获取网页的内容并返回
def load_url(url, timeout):
with urllib.request.urlopen(url, timeout=timeout) as conn:
return conn.read()
# 使用with语句来确保及时清理线程
with concurrent.futures.ThreadPoolExecutor(max_workers=5) as executor:
# 执行器调用submit提交url,future_to_url保存未来可能得到的结果
future_to_url = {executor.submit(load_url, url, 60): url for url in URLS}
for future in concurrent.futures.as_completed(future_to_url):
url = future_to_url[future]
try:
data = future.result()
except Exception as exc:
print('%r generated an exception: %s' % (url, exc))
else:
print('%r page is %d bytes' % (url, len(data)))
需要注意的是:max_workers参数,源码如下
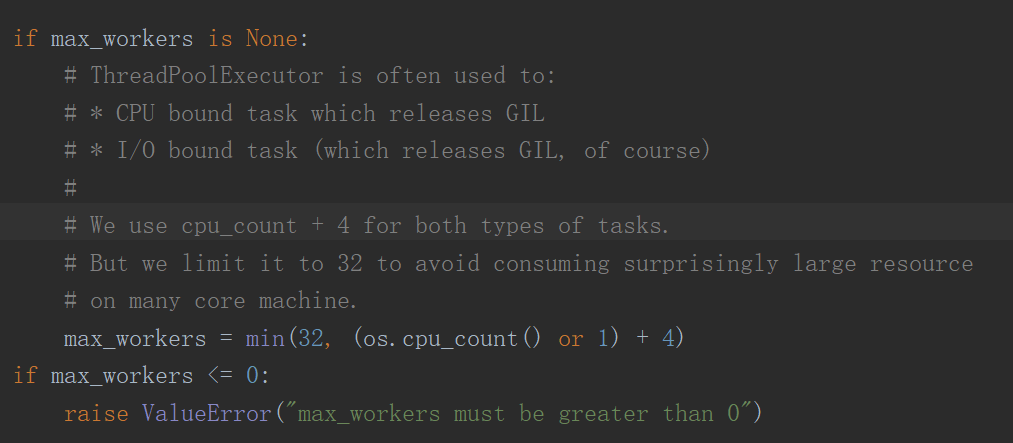
在 3.8 版更改: max_workers 的默认值已改为 min(32, os.cpu_count() + 4)。 这个默认值会保留至少 5 个工作线程用于 I/O 密集型任务。 对于那些释放了 GIL 的 CPU 密集型任务,它最多会使用 32 个 CPU 核心。这样能够避免在多核机器上不知不觉地使用大量资源。?
asyncio异步IO
import aiohttp
import asyncio
import time
async def dl_coroutine(session, url):
print(f'开始下载{url}')
async with session.get(url, verify_ssl=False) as res:
content = await res.content.read()
t = time.time()
filename = url.split('.')[1]
with open(filename, mode='wb') as f:
f.write(content)
print(f'下载完成{filename}')
async def main(urls):
async with aiohttp.ClientSession() as session:
tasks = [dl_coroutine(session, url) for url in urls]
# asyncio.as_completed(tasks,timeout=5)
await asyncio.gather(*tasks)
if __name__ == '__main__':
URLS = ['http://www.baidu.com/',
'http://www.taobao.com/',
'http://www.csdn.net/', ]
t1 = int(round(time.time() * 1000))
asyncio.run(main(URLS)) # 如果执行报错RuntimeError: Event loop is closed,把这一行注释,放下面两行执行
# loop = asyncio.get_event_loop()
# loop.run_until_complete(main(URLS))
t2 = int(round(time.time() * 1000))
print('执行了{} 毫秒'.format(t2 - t1))
以上只是简单列举了一些使用demo,还有很多常用的情景没有说明到,包括进程/线程之间怎么共享变量,同步锁,队列等等,有需要的参考官网文档
多进程:concurrent.futures --- 启动并行任务 — Python 3.10.4 文档
多线程:concurrent.futures --- 启动并行任务 — Python 3.10.4 文档
asyncio:协程与任务 — Python 3.10.4 文档
|