一、Tensorflow+Keras 参考文章:
https://blog.csdn.net/baidu_36669549/article/details/84993772
在tensorflow官网的图像识别的中文介绍中,介绍了如何用Tensorflow的模型代码库中的classify_image.py进行图像识别。里面有介绍如何测试,而且还提供了最后一层的112048维的特征提取方式,所以在这里介绍一下。
......
with tf.Session() as sess:
softmax_tensor = sess.graph.get_tensor_by_name('softmax:0')
.......
源码就是在这里进行的介绍,有三个接口,
‘softmax:0’: A tensor containing the normalized prediction across 1000 labels.
‘pool_3:0’: A tensor containing the next-to-last layer containing 2048 float description of the image.
‘DecodeJpeg/contents:0’: A tensor containing a string providing JPEG encoding of the image.
预测的话直接调’softmax:0’:和’DecodeJpeg/contents:0’:可以进行图像识别的测试
如果想要提取特征就像这样
fc_tensor = sess.graph.get_tensor_by_name('pool_3:0')
pool_1 = sess.run(fc_tensor,{'DecodeJpeg/contents:0': image_data})
就可以,保存的话可以选择CSV或者.mat文件
import tensorflow as tf
import numpy as np
import os
from PIL import Image
import matplotlib.pyplot as plt
import scipy.io as scio
model_dir='F:/fqh/models-master/tutorials/image/imagenet/2015'
image = 'F:/fqh/models-master/tutorials/image/imagenet/data_set/face/faces96_152_20_180-200jpgview-depth/'
target_path=image+'wjhugh/'
class NodeLookup(object):
def __init__(self, label_lookup_path=None, uid_lookup_path=None):
if not label_lookup_path:
label_lookup_path = os.path.join(
model_dir, 'imagenet_2012_challenge_label_map_proto.pbtxt')
if not uid_lookup_path:
uid_lookup_path = os.path.join(
model_dir, 'imagenet_synset_to_human_label_map.txt')
self.node_lookup = self.load(label_lookup_path, uid_lookup_path)
def load(self, label_lookup_path, uid_lookup_path):
if not tf.gfile.Exists(uid_lookup_path):
tf.logging.fatal('File does not exist %s', uid_lookup_path)
if not tf.gfile.Exists(label_lookup_path):
tf.logging.fatal('File does not exist %s', label_lookup_path)
proto_as_ascii_lines = tf.gfile.GFile(uid_lookup_path).readlines()
uid_to_human = {}
for line in proto_as_ascii_lines:
line = line.strip('\n')
parse_items = line.split('\t')
uid = parse_items[0]
human_string = parse_items[1]
uid_to_human[uid] = human_string
proto_as_ascii = tf.gfile.GFile(label_lookup_path).readlines()
node_id_to_uid = {}
for line in proto_as_ascii:
if line.startswith(' target_class:'):
target_class = int(line.split(': ')[1])
if line.startswith(' target_class_string:'):
target_class_string = line.split(': ')[1]
node_id_to_uid[target_class] = target_class_string[1:-2]
node_id_to_name = {}
for key, val in node_id_to_uid.items():
if val not in uid_to_human:
tf.logging.fatal('Failed to locate: %s', val)
name = uid_to_human[val]
node_id_to_name[key] = name
return node_id_to_name
def id_to_string(self, node_id):
if node_id not in self.node_lookup:
return ''
return self.node_lookup[node_id]
def create_graph():
with tf.gfile.FastGFile(os.path.join(
model_dir, 'classify_image_graph_def.pb'), 'rb') as f:
graph_def = tf.GraphDef()
graph_def.ParseFromString(f.read())
tf.import_graph_def(graph_def, name='')
create_graph()
list0=[]
for root, dirs,files in os.walk(image):
list0.append(dirs)
img_list=[]
for ii in list0[0]:
img_list.append(ii)
list_img_name=np.array(img_list)
list_img_name.sort()
with tf.Session() as sess:
softmax_tensor = sess.graph.get_tensor_by_name('softmax:0')
for jj in range(0,len(list_img_name)):
target_path=image+list_img_name[jj]+'/'
for root, dirs, files in os.walk(target_path):
for file in files:
img_path = target_path+file
image_data = tf.gfile.FastGFile(img_path, 'rb').read()
fc_tensor = sess.graph.get_tensor_by_name('pool_3:0')
pool_1 = sess.run(fc_tensor,{'DecodeJpeg/contents:0': image_data})
pool_2 = pool_1[0,0,0,:]
img_path=img_path[:len(img_path)-4]
scio.savemat(img_path+'.mat', {"pool_2": pool_2})
pi= (jj/(len(list_img_name)-1))*100
print("%4.2f %%" % pi)
将向量拉直并遍历整个数据集
二、Pytorh版
import os.path
import torch
import torch.nn as nn
from torchvision import models, transforms
from torch.autograd import Variable
import numpy as np
from PIL import Image
features_dir ='./features'
data_list =[]
path='./FullFrame1'
for filename in os.listdir(path):
transform1 = transforms.Compose([
transforms.Resize(299),
transforms.CenterCrop(299),
transforms.ToTensor(),
transforms.Normalize(mean=[0.485,0.456,0.406],
std=[0.229,0.224,0.225])
])
print(filename)
img = Image.open(path +'/'+ filename)
img1 = transform1(img)
inception_v3_feature_extractor = models.inception_v3(pretrained=True)
inception_v3_feature_extractor.fc = nn.Linear(2048,2048)
torch.nn.init.eye(inception_v3_feature_extractor.fc.weight)
for param in inception_v3_feature_extractor.parameters():
param.requires_grad =False
print(img1.shape)
x = Variable(torch.unsqueeze(img1, dim=0).float(), requires_grad=False)
print(x.shape)
inception_v3_feature_extractor.training=False
y = inception_v3_feature_extractor(x)
y = y.data.numpy()
data_list.append(y)
data_npy = np.array(data_list)
print(data_npy.shape)
np.save('1.npy',data_list)
修改后,每个图片的特征保存为一个.txt文件(也可以是.csv文件): 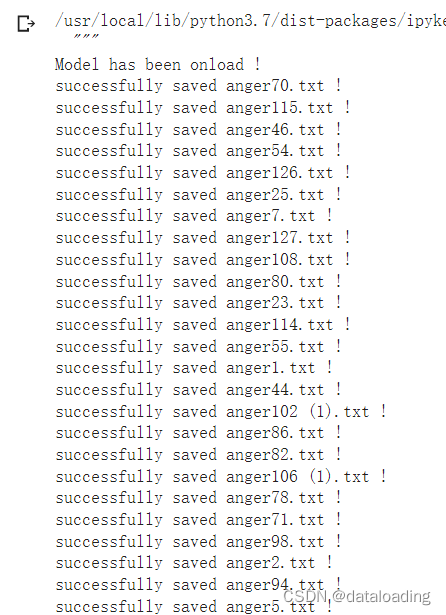
import os.path
import torch
import torch.nn as nn
from torchvision import models, transforms
from torch.autograd import Variable
import numpy as np
from PIL import Image
def feature_extract(file,save_path):
transform1 = transforms.Compose([
transforms.Resize(299),
transforms.CenterCrop(299),
transforms.ToTensor(),
transforms.Normalize(mean=[0.485,0.456,0.406],std=[0.229,0.224,0.225])
])
img = Image.open(file)
img1 = transform1(img)
x = Variable(torch.unsqueeze(img1, dim=0).float(), requires_grad=False)
inception_v3_feature_extractor.training=False
y = inception_v3_feature_extractor(x)
y = y.data.numpy()
y = y.reshape(1,2048)
np.savetxt(save_path + '.txt', y, fmt='%s')
def read_image(rootdir,save_path):
list = os.listdir(rootdir)
for file in os.listdir(rootdir):
savePath = os.path.join(save_path,file[:-4])
filename = os.path.join(rootdir,file)
feature_extract(filename,savePath)
print("successfully saved " + file[:-4] + ".txt !")
if __name__ == '__main__':
inception_v3_feature_extractor = models.inception_v3(pretrained=True)
inception_v3_feature_extractor.fc = nn.Linear(2048,2048)
torch.nn.init.eye(inception_v3_feature_extractor.fc.weight)
for param in inception_v3_feature_extractor.parameters():
param.requires_grad = False
print("Model has been onload !")
save_path ='/content/drive/MyDrive/ImageClassify_feature_extract/inception_V3_ck_feature'
root_dir='/content/drive/MyDrive/ImageClassify_feature_extract/ck+/data2'
read_image(root_dir,save_path)
print("work has been done !")
参考链接:
https://wenku.baidu.com/view/993bc45a24284b73f242336c1eb91a37f111326b.html 来源:百度文库 著作权归作者所有。商业转载请联系作者获得授权,非商业转载请注明出处。
|