我们知道,一般程序的执行顺序是从上往下依次执行的。如果有两个任务,一个任务执行时间需要5秒,另一个任务执行时间需要4秒,那么按往常的做法需要9秒才能完成以上两个任务。那能不能让这两个任务同时进行并在5秒内完成呢?当然可以,这里引入我们今天的主角:线程——threading模块。
多线程的基本使用
多线程的出现是为了能够帮助我们解决资源被霸占的问题,下面看看它的基本使用。
import time, datetime
import threading
def func():
"""这里写明线程需要执行的任务"""
print(threading.current_thread().getName(), datetime.datetime.now())
print('hello~')
time.sleep(2)
print(threading.current_thread().getName(), datetime.datetime.now())
def func2():
"""这里写上第二个任务"""
print(threading.current_thread().getName(), datetime.datetime.now())
print('hi~')
time.sleep(3)
print(threading.current_thread().getName(), datetime.datetime.now())
thread1 = threading.Thread(target=func)
thread2 = threading.Thread(target=func2)
thread1.start()
thread2.start()
pycharm下的运行结果
C:\Users\17591\.virtualenvs\test\Scripts\python.exe C:/Users/17591/PycharmProjects/test/books.py
Thread-1 2022-06-20 18:00:54.397643
hello~
Thread-2 2022-06-20 18:00:54.397643
hi~
Thread-1 2022-06-20 18:00:56.403895
Thread-2 2022-06-20 18:00:57.405731
Process finished with exit code 0
可以看到,一个2秒一个3秒的任务只需3秒就完成了,说明这两个任务确实是同时进行的。
命名 每个线程名默认是以 thread-xx 命名的,如果想自己定义的话,可以在创建实例对象时用name进行指明。
thread = threading.Thread(target=func,name="这是我的第一个线程")
传递参数 当我们调用函数需要传递参数时,在创建实例对象时用 args 或 kwargs 指明。
def func(name, age):
print(name, age)
thread = threading.Thread(target=func, name="这是我的第一个线程", kwargs={"name": "lishuaige", 'age': 21})
thread.start()
亦可写成
thread = threading.Thread(target=func, name="这是我的第一个线程", args=('lishuaige',21))
threading的进一步使用
Daemon线程
Daemon线程也叫守护线程。什么是守护线程?看看别人怎么说:守护线程–也称“服务线程”,在 没有用户线程可服务时会自动离开。优先级:守护线程的优先级比较低,用于为系统中的其它对象和线程提供服务。
一般情况下,一段程序会等所有线程执行完毕后,才会关闭。就拿 pycharm 来说,执行完程序后,控制台会返回 Process finished with exit code 0 的字样,这就标志着该程序已经执行完毕了。不同的是,如果Daemon线程存在的话,程序运行完后就算Daemon线程还有任务,也不会等它,直接关闭掉,返回 Process finished with exit code 0 ,随之Daemon本身的任务也会关闭。
打个比方,几个同学约好出去玩,大家都到齐了,唯独daemon还在来的路上,等了好久好久,大伙们都等的不耐烦了,还是没有来,于是一个朋友打了电话给daemon,哼,等了这么久还没来我们走了!随后生气的挂断了电话,就出发了。daemon伤心的叹了口气,哎,我去给你们买零食了…
简单的说,如果有守护线程,那么除守护线程外的所有线程执行完毕后,就会终止程序,守护线程的任务也会随之关闭。
在python中均可将每个线程设置为守护线程。
import time, datetime
import threading
def func():
time.sleep(2)
print('这是守护线程')
thread = threading.Thread(target=lambda :print("这是线程"))
dae = threading.Thread(target=func)
dae.setDaemon(True)
dae.start()
thread.start()
pycharm输出结果是
C:\Users\17591\.virtualenvs\test\Scripts\python.exe C:/Users/17591/PycharmProjects/test/books.py
这是线程
Process finished with exit code 0
通过 dae.setDaemon(True) 的命令将dae设置为了守护线程,因为执行完出守护线程外的线程后就会终止程序,所以“这是守护线程” 这条输出语句并未成功执行。
join() 线程中的join()方法是用来保证该线程顺利执行以及堵塞主线程用的。什么是主线程?回顾一下我们之前写的代码,即使没有导入threading模块也能运行,其实这就是因为主线程在工作。主线程,相当于是执行总程序的线程。 join(timeout),timeout可以不写,那样的话就等该线程执行完后再执行主线程的代码。如果写的话,以秒为单位,表示堵塞多少秒,期间会运行除主线程外的所有已经(用start命令)启动了的线程,当堵塞时间过去后继续执行主线程的代码。
import time, datetime
import threading
def func():
print("启动", datetime.datetime.now())
time.sleep(2)
print("结束", datetime.datetime.now())
thread = threading.Thread(target=func)
the = threading.Thread(target=func)
the.start()
the.join(1)
thread.start()
thread.join(0.5)
pycharm运行结果是
C:\Users\17591\.virtualenvs\test\Scripts\python.exe C:/Users/17591/PycharmProjects/test/books.py
启动 2022-06-20 21:04:47.814156
启动 2022-06-20 21:04:48.826980
结束 2022-06-20 21:04:49.826345
结束 2022-06-20 21:04:50.834772
Process finished with exit code 0
因为thread线程没有启动,所以the线程发起的阻塞只有它自身一个线程在工作,阻塞完后thread线程启动了,并发起0.5秒的阻塞,因为两个线程都启动了,所以该阻塞不会影响到他们,只影响到了主线程。最后三秒完成两个两秒的任务,期间因为阻塞,一个线程晚了一秒执行。
isAlive() 用于判断线程是否在工作。
thread = threading.Thread(target=func)
thread.start()
thread.join()
threading.active_count() 目前工作的线程数,含主线程。注意threading为线程的模块名。
thread = threading.Thread(target=func)
the = threading.Thread(target=func)
thread.start()
the.start()
print(threading.active_count())
threading.enumerate() 迭代出目前所有工作的线程。
print(threading.enumerate())
threading.current_thread() 获取当前工作的线程。
自定义线程
如果你想自定义线程,那么这里同样能够满足你。只需要继承threading.Thread,调用它的__init__方法,最后在run函数中定义你的任务即可。
import time, datetime
import threading
class MyThread(threading.Thread):
def __init__(self, *args, **kwargs):
super().__init__(*args, **kwargs)
def run(self):
print('hello')
time.sleep(2)
thread = MyThread()
thread.start()
其实,线程对象既能用start执行任务,也能用run执行任务。不同的是,run具有类似join的特性,需等待run任务执行完毕后再其进行后面的操作。另外,重写的线程类中可以多次调用run方法,而原始的threading.thread类,仅能有一次run。 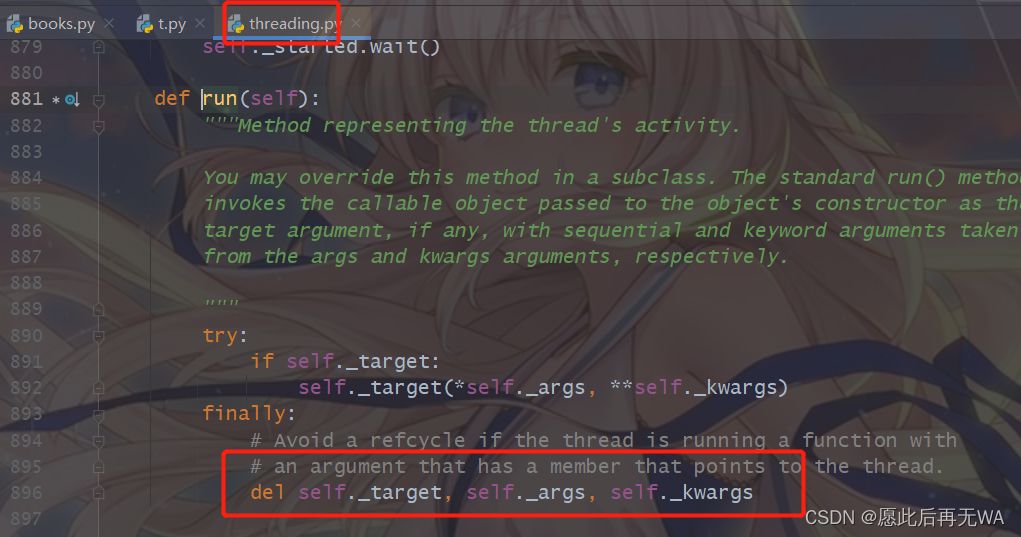 在原类中,当线程执行完毕后就会销毁对象,回收资源。而我们重写后如果没有销毁而且不重复利用的话,会造成资源不必要的浪费。
锁
提到锁,我们先聊一聊:不知大家有没有留意前面“pycharm运行结果”这个词出现过很多次,那么你们知道我为什么强调pycharm?因为,在pycharm或许输出能够工整一点,如果换原生的编译器呢?
import time, datetime
import threading
def func():
"""这里写明线程需要执行的任务"""
print(threading.current_thread().getName(), datetime.datetime.now())
print('hello~')
time.sleep(2)
print(threading.current_thread().getName(), datetime.datetime.now())
thread1 = threading.Thread(target=func)
thread2 = threading.Thread(target=func)
thread1.start()
thread2.start()
以上方代码为例,我们一起看看结果吧! 是不是觉得很不可思议?甚至python 的经典标志 >>> 先跑出来了,执行print(2)命令是可以正常输出的;两次输出的时候有时候他们名字合并了甚至连换行符都没有,而有时候又没有合并,每次执行显示的结果都会有不一样的。实际上,各线程无法预期谁会先取得资源然后进行数据处理,所以会出现争先恐后输出的情况,这种现象称为竞速。为避免这种现象发生,锁的概念也随之到来,它的出现并非真要解决简单的输出问题,或许是因为线程相关的安全问题。我再举个例子:
import time, datetime
import threading
MONEY = 100
def withdrawMoney(amount):
global MONEY
if MONEY >= amount:
time.sleep(0.01)
MONEY -= amount
print(f"已取 {amount} 元,剩余 {MONEY} 元")
else:
print("余额不足!")
thread1 = threading.Thread(target=withdrawMoney,args=[100])
thread2 = threading.Thread(target=withdrawMoney,args=[50])
thread1.start()
thread2.start()
假设你微信有100块钱,想看看能不能钻个漏洞。在手机和电脑上同时登录,并且同一时刻同时提现,这时候如果没有使用锁的话就会出现如下情况。 同一份代码,我运行了三次,会出现多次不一样的结果。
现在我们分析一下出现这些情况的原因。在上面的例子中,有两个线程, 一个任务是取50元,另一个任务是取100元。当前一个线程进入判断语句后,因为服务器出现延迟,所以等待了10毫秒,没有执行体现的操作,那么这时候的MONEY还是100元,几乎同一时刻发生了线程调度切换,另外一个线程也走到了判断语句,因为MONEY还是100元,所以,他也进去了,并没有走余额不足的分支。问题来了,当短暂的服务器延迟过去后,因为两个线程都进入到了提现的步骤上,所以都会进行减的操作,随之也就出现了负数的情况。
那解决以上问题有什么办法呢?有同学说,想办法把服务器的延迟问题解决!但偶尔出现服务器延迟是没有办法避免的。也有同学说看能不能把延迟问题放到判断语句外面?这似乎可以,因为出现延迟一般是网络问题。而像上面的逻辑计算,中间不会因为网络问题而卡顿的。但就算延迟问题在外面,也有可能出现几乎同一时刻两个线程同时进入判断语句内的情况。我将time.sleep()写在判断语句内层只是方便演示,确保两个线程能够百分百进入判断语句内而已。所以,以上出现问题,需要用锁来解决。
首先需要获取lock对象
lock = threading.Lock()
获得锁
lock.acquire()
释放锁
lock.release()
在两者之间写入逻辑不可分割的代码块。
也能使用 with 方法。
import time, datetime
import threading
lock = threading.Lock()
MONEY = 100
def withdrawMoney(amount):
global MONEY
lock.acquire()
if MONEY >= amount:
time.sleep(0.01)
MONEY -= amount
print(f"已取 {amount} 元,剩余 {MONEY} 元")
else:
print("余额不足!")
lock.release()
thread1 = threading.Thread(target=withdrawMoney,args=[100])
thread2 = threading.Thread(target=withdrawMoney,args=[50])
thread1.start()
thread2.start()
线程池
新建与终止线程都会在时间与性能上造成一定开销,如果可以减少新建与终止线程的操作的话,可以在一定程度上提高代码执行效率,而线程池,就是一套优化方案,其包含两个概念,任务队列和线程池。当有新任务出现时,会将任务放在任务队列里面,线程池中已经包含多个预先建立好的线程,这些线程会处理队列中的任务,并将其弹出任务队列。
我们结合代码讲解:
from concurrent.futures import ThreadPoolExecutor
def add(num):
num += 100
return num
lst = list(range(20))
with ThreadPoolExecutor() as pool:
res = (pool.map(add,lst))
for i in res:
print(i)
首先导入 ThreadPoolExecutor 线程池。定义一个加法函数,使用map方法,让列表中的元素分别加上100,最后打印结果。ThreadPoolExecutor 模块下的map方法与普通map方法的用法基本一致,都是让一个函数分别作用在可迭代对象中的每个元素上。(若想继续了解map用法可查看我的这篇文章https://blog.csdn.net/lishuaigell/article/details/124168814)
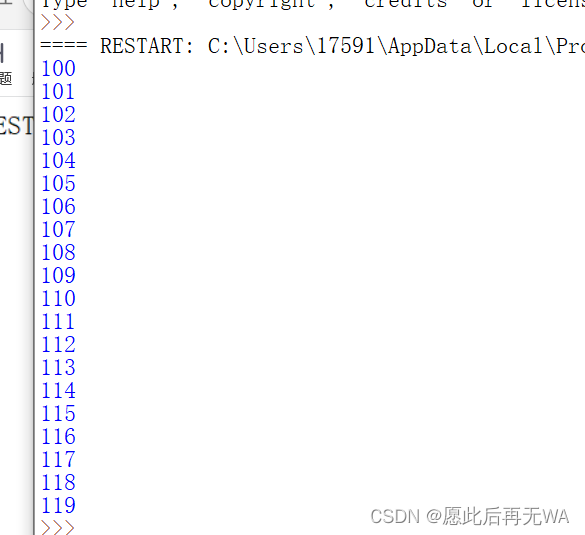 观察结果可以发现,经过处理后的元素都是按顺序输出的。是偶然的吗?不是,map方法处理的结果就是按顺序输出的。这意味着什么?意味着有些先处理完后面任务的线程,因为顺序的缘故,导致无法提交结果,需等待前面的任务完成,提交结果后才能继续,所以被阻塞了!
为解决上述问题又有了新的方法, submit – as_completed。as_completed 需要搭配submit一起使用。
from concurrent.futures import ThreadPoolExecutor,as_completed
def add(num):
num += 100
return num
lst = list(range(20))
with ThreadPoolExecutor() as pool:
futures = (pool.submit(add,l) for l in lst)
for future in as_completed(futures):
print(future.result())
使用方法与前面类似,不同的是,submit 每次只能让函数作用在一个元素上,而 map 每次能让函数作用在每个元素上,另外,如果要获取结果,要用result方法。
注意,线程池本质还是线程,多线程并不适合应对CPU密集型计算,只适合处理IO密集型计算。像上面的加法函数,因为数量级比较小看不出效果,如果式子稍微复杂点,数更大点的话处理时间会比单线程慢得多,因为它属于cpu密集型计算。由于python有GIL(全局解释器锁,据说python3每个线程15毫秒就会检查并释放GIL)的存在,无论你有多少个cpu,同一时刻只会有一个cpu,一个线程在工作。如果计算量大,又出现多线程频繁调度的话,只会提高cpu负荷和等待时间,造成反作用。就好比在家里频繁开关灯一样,如果狂开狂关灯,不出三十个来回,那盏灯恐怕就顶不住了。
为充分利用cpu,python 也出台了相关的应对措施,多进程—— multiprocessing 模块。
在下一篇《python 多进程》中,我会详细讲解 python multiprocessing 模块的基本用法,欢迎关注。
|