前言
最近,想学习下flask部署算法,想到前段时间基于efficientnet弄了个猫狗分类,故就拿它来练手吧。
一、模型训练
Efficientnet分类任务的训练及测试代码可参考我的另一篇文章:Efficientnet训练和测试
二、flask页面设计
这部分在网上的下了一些开源的代码,因为本人不太会写前端的东西(嫌麻烦),链接如下:flask页面,个人感觉这个页面设计挺好的。
三、接口设计
这里话不多说,直接上代码:
from flask import Flask,jsonify,render_template,request
import re
from PIL import Image
import base64,io
import cv2
import numpy as np
import torch,argparse,torchvision
device="cuda" if torch.cuda.is_available() else "cpu"
app=Flask(__name__)
def parser_opt():
parser=argparse.ArgumentParser()
parser.add_argument("--weights",type=str,default="./weight/cat_dog/best.pth",help="model path")
parser.add_argument("--imgsz",type=int,default=224,help="test image size")
opt=parser.parse_known_args()[0]
return opt
class Test_model():
def __init__(self,opt):
self.imgsz=opt.imgsz
self.model=(torch.load(opt.weights)).to(device)
self.model.eval()
self.class_name=["cat","dog"]
def expend_img(self, img,mode="RGB", fill_pix=0):
'''
:param img: 图片数据
:param mode: 图片格式,如RGB或者BGR等
:param fill_pix: 填充像素,默认为灰色,自行更改
:return:
'''
if mode=="RGB":
w, h = img.size
padd_length = int(abs(w - h) / 2)
box = (padd_length, 0) if w < h else (0, padd_length)
new_img = Image.new("RGB", (max(h, w), max(h, w)), color=(fill_pix,fill_pix,fill_pix))
new_img.paste(img, box)
else:
h, w = img.shape[:2]
if h >= w:
padd_width = int(h - w) // 2
padd_h, padd_b, padd_l, padd_r = 0, 0, padd_width, padd_width
elif h < w:
padd_high = int(w - h) // 2
padd_h, padd_b, padd_l, padd_r = padd_high, padd_high, 0, 0
new_img = cv2.copyMakeBorder(img, padd_h, padd_b, padd_l, padd_r, borderType=cv2.BORDER_CONSTANT,
value=[fill_pix, fill_pix, fill_pix])
return new_img
def pred_one(self, img,mode="RGB"):
data_transorform = torchvision.transforms.Compose([
torchvision.transforms.Resize((self.imgsz,self.imgsz)),
torchvision.transforms.CenterCrop((self.imgsz,self.imgsz)),
torchvision.transforms.ToTensor(),
torchvision.transforms.Normalize(mean=[0.485, 0.456, 0.406], std=[0.229, 0.224, 0.225])
])
if mode=="RGB":
new_img = self.expend_img(img)
else:
img = cv2.cvtColor(np.asarray(img), cv2.COLOR_RGB2BGR)
new_img = self.expend_img(img)
new_img = Image.fromarray(cv2.cvtColor(new_img, cv2.COLOR_BGR2RGB))
img = data_transorform(new_img)
img = torch.reshape(img, (-1, 3, self.imgsz, self.imgsz)).to(device)
pred = self.model(img)
_, pred = torch.max(pred, 1)
outputs = self.class_name[pred]
return outputs
def base64_to_pil(img_base64,mode="RGB"):
"""
Convert base64 image data to PIL image
"""
image_data = re.sub('^data:image/.+;base64,', '', img_base64)
if mode=="RGB":
pil_image = Image.open(io.BytesIO(base64.b64decode(image_data)))
return pil_image
elif mode=="BGR":
mat_image=base64.b64decode(image_data)
mat_image=cv2.imdecode(np.fromstring(mat_image,np.uint8),cv2.COLOR_RGB2BGR)
return mat_image
@app.route('/',methods=["GET"])
def index():
return render_template("index.html")
@app.route("/predict",methods=["GET","POST"])
def predict():
if request.method=="POST":
img=base64_to_pil(request.json)
pred=test_img.pred_one(img)
return jsonify(result=pred)
if __name__ == '__main__':
opt = parser_opt()
test_img = Test_model(opt)
app.run(port=3000,debug=True)
其中需要注意的是,在一些函数中有个参数mode,这个根据自己的实际需求去设定,最好从图片的预处理耗时和推理效果进行对比。
四、效果展示
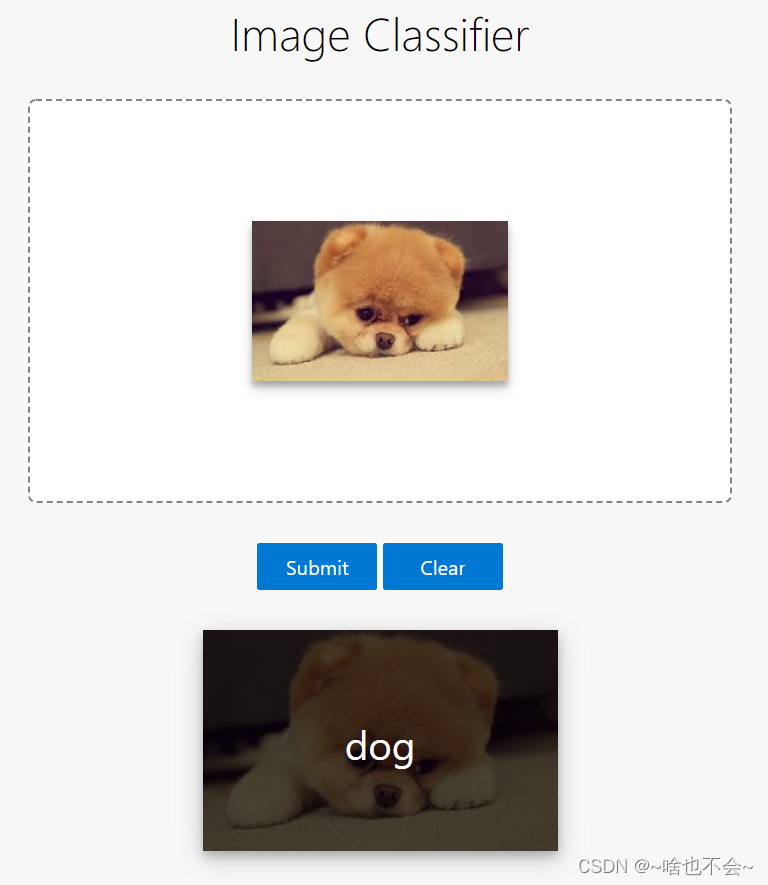
五、扩充部分(图片互转)
这里记录了一些图片互想转换的方式,如PIL->base64等,代码如下:
import cv2
import numpy as np
from PIL import Image
import base64,io
import time
def base64_2_pil(imgpath):
'''
:param imgpath: 图片路径
:return:
'''
with open(imgpath,"rb") as f:
img_base64=base64.b64encode(f.read())
pil_image=Image.open(io.BytesIO(base64.b64decode(img_base64)))
return pil_image
def pil_2_base64(imgpath):
'''
:param imgpath: 图片路径
:return:
'''
image=Image.open(imgpath)
img_buff=io.BytesIO()
image.save(img_buff,format="JPEG")
img_str=base64.b64encode(img_buff.getvalue())
return img_str
def opencv_base64(imgpath):
'''
:param imgpath: 图片路径
:return:
'''
img=cv2.imread(imgpath)
img_str=base64.b64encode(cv2.imencode(".jpg",img)[1].tostring())
return img_str
def base64_opencv(imgpath):
'''
:param imgpath: 图片路径
:return:
'''
with open(imgpath,"rb") as f:
img_base64=base64.b64encode(f.read())
image=cv2.imdecode(np.fromstring(base64.b64decode(img_base64),np.uint8),cv2.COLOR_RGB2BGR)
return image
def pil_opencv(imgpath):
'''
:param imgpath: 图片路径
:return:
'''
pil_img=Image.open(imgpath)
img=cv2.cvtColor(np.asarray(pil_img),cv2.COLOR_RGB2BGR)
return img
def opencv_pil(imgpath):
'''
:param imgpath: 图片路径
:return:
'''
img=cv2.imread(imgpath)
pil_img=Image.fromarray(cv2.cvtColor(img,cv2.COLOR_BGR2RGB))
return pil_img
if __name__ == '__main__':
imgpath=r"./1.jpg"
base64_2_pil(imgpath)
pil_2_base64(imgpath)
opencv_base64(imgpath)
base64_opencv(imgpath)
pil_opencv(imgpath)
opencv_pil(imgpath)
总结
以上就是这篇文章的全部内容,如有bug或有问题的,请在评论区交流或者加入qq群:995760755交流。
|