教程来源:https://datawhalechina.github.io/fantastic-matplotlib 参考资料: 1、python画分布、密度等图形 2、python - 如何注释海底联合网格/联合图中的边际图/分布图 3、python可视化48|最常用11个分布(Distribution)关系图
import numpy as np
import pandas as pd
import seaborn as sns
import matplotlib.pyplot as plt
plt.rcParams['font.sans-serif'] = ['SimHei']
plt.rcParams['axes.unicode_minus'] = False
1、子图
1.1、使用 plt.subplots() 绘制均匀状态下的子图
返回元素分别是画布和子图构成的列表,第一个数字为行,第二个为列,不传入时默认值都为1 figsize 参数可以指定整个画布的大小 sharex 和 sharey 分别表示是否共享横轴和纵轴刻度 tight_layout 函数可以调整子图的相对大小使字符不会重叠
fig, axs = plt.subplots(2, 5, figsize=(10, 4), sharex=False, sharey=False)
fig.suptitle('样例1', size=20)
for i in range(2):
for j in range(5):
axs[i][j].scatter(np.random.randn(10), np.random.randn(10))
axs[i][j].set_title('第%d行,第%d列'%(i+1,j+1))
axs[i][j].set_xlim(-5,5)
axs[i][j].set_ylim(-5,5)
if i==1:
axs[i][j].set_xlabel('横坐标')
if j==0:
axs[i][j].set_ylabel('纵坐标')
fig.tight_layout()
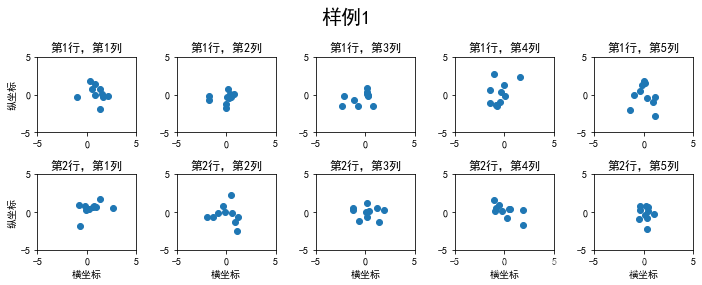
subplots是基于OO模式的写法,显式创建一个或多个axes对象,然后在对应的子图对象上进行绘图操作。
还有种方式是使用subplot这样基于pyplot模式的写法,每次在指定位置新建一个子图,并且之后的绘图操作都会指向当前子图,本质上subplot也是Figure.add_subplot的一种封装。
plt.figure()
plt.subplot(2,2,1)
plt.plot([1,2], 'r')
plt.subplot(2,2,2)
plt.plot([1,2], 'b')
plt.subplot(224)
plt.plot([1,2], 'g');
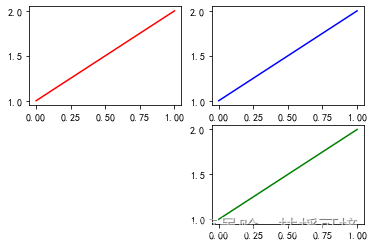
N = 5
r = 2 * np.random.rand(N)
theta = 2 * np.pi * np.random.rand(N)
area = 1000 * r**2
colors = theta
plt.subplots(figsize=(8,8))
plt.subplot(projection='polar')
plt.scatter(theta, r, c=colors, s=area, cmap='hsv', alpha=0.75);
plt.savefig('test1.png',dpi=400,bbox_inches='tight',transparent=True)
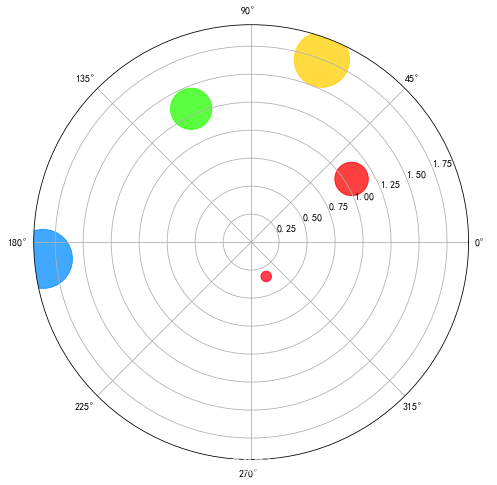
Python Matplotlib.axes.Axes.bar()用法及代码示例: https://vimsky.com/examples/usage/matplotlib-axes-axes-bar-in-python.html
Matplotlib.axes.Axes.bar()函数 matplotlib库的axiss模块中的Axes.bar()函数用于制作条形图。
用法: Axes.bar(self, x, height, width=0.8, bottom=None, *, align=’center’, data=None, **kwargs)
参数:此方法接受以下描述的参数:
x:此参数是水平坐标的顺序。 height:此参数是的高度。 width:此参数是可选参数。它是条的宽度,默认值为0.8。 bottom:此参数也是可选参数。它是条形底的y坐标,默认值为0。 alighn:此参数也是可选参数。它用于将条形图与x坐标对齐。
Python Matplotlib.axes.Axes.text()用法及代码示例: https://vimsky.com/examples/usage/matplotlib-axes-axes-text-in-python.html
matplotlib库的axiss模块中的Axes.text()函数还用于将文本s添加到数据坐标中x,y位置的轴上。
用法:
Axes.text(self, x, y, s, fontdict=None, withdash=, **kwargs) 参数:此方法接受以下描述的参数:
s:此参数是要添加的文本。 xy:此参数是放置文本的点(x,y)。 fontdict:此参数是一个可选参数,并且是一个覆盖默认文本属性的字典。 withdash:此参数也是可选参数,它创建TextWithDash实例而不是Text实例。 返回值:此方法返回作为创建的文本实例的文本。
y=20
x=np.pi/2
w=np.pi/2
color=(206/255,32/255,69/255)
edgecolor=(206/255,32/255,69/255)
fig=plt.figure(figsize=(13.44,7.5))
ax=fig.add_subplot(111,projection='polar')
ax.bar(x=np.pi/2,height=y,width=w,bottom=10,color=color,edgecolor=color)
fig.savefig('test2.png',dpi=400,bbox_inches='tight',transparent=True)
![[外链图片转存失败,源站可能有防盗链机制,建议将图片保存下来直接上传(img-ELE7knLj-1663591476496)(output_11_0.png)]](https://img-blog.csdnimg.cn/228d6a828ca643d8b00a97e17b097f1c.png#pic_center)
fig = plt.figure(figsize=(10,6))
ax = plt.subplot(111,projection='polar')
ax.set_theta_direction(-1)
ax.set_theta_zero_location('N')
r = np.arange(100,800,20)
theta = np.linspace(0, np.pi * 2, len(r), endpoint=False)
ax.bar( theta,r,
width=0.18,
color=np.random.random((len(r),3)),
align='edge',
bottom=100)
ax.text(np.pi*3/2-0.2,90,'Origin',fontsize=14)
for angle,height in zip(theta,r):
ax.text(angle+0.03,height+105,str(height),
fontsize=height/80)
plt.axis('off')
plt.tight_layout()
plt.savefig('polarBar.png',dpi=480)
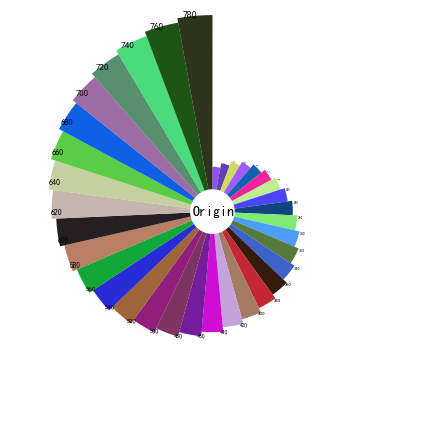
import numpy as np
import matplotlib.pyplot as plt
fig = plt.figure(figsize=(15,15))
ax = plt.subplot(projection='polar')
ax.set_theta_direction(-1)
ax.set_theta_zero_location('N')
r = np.arange(100, 800, 20)
theta = np.linspace(0, np.pi * 2, len(r), endpoint=False)
ax.bar(theta, r, width=0.18, color=np.random.random((len(r), 3)),
align='edge', bottom=100)
ax.text(np.pi*3/2-0.2, 80, 'Origin', fontsize=30)
for angle, height in zip(theta, r):
ax.text(angle + 0.03, height + 120, str(height), fontsize=height / 20)
plt.axis('off')
plt.tight_layout()
plt.show()
fig.savefig('test3.png',dpi=400,bbox_inches='tight',transparent=True)
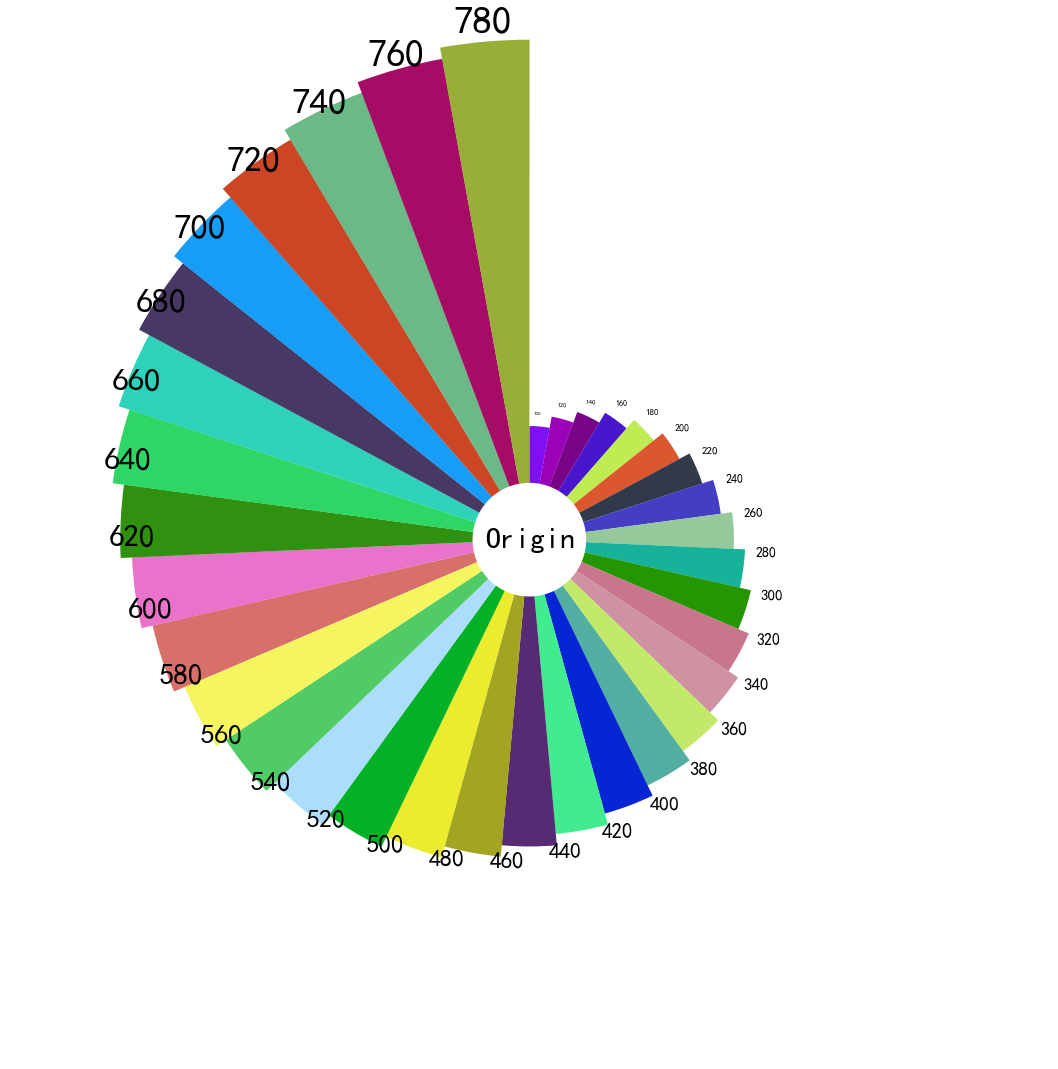
1.2、使用 GridSpec 绘制非均匀子图
所谓非均匀包含两层含义,第一是指图的比例大小不同但没有跨行或跨列,第二是指图为跨列或跨行状态
利用 add_gridspec 可以指定相对宽度比例 width_ratios 和相对高度比例参数 height_ratios
fig = plt.figure(figsize=(10, 4))
spec = fig.add_gridspec(nrows=2, ncols=5, width_ratios=[1,2,3,4,5], height_ratios=[1,3])
fig.suptitle('样例2', size=20)
for i in range(2):
for j in range(5):
ax = fig.add_subplot(spec[i, j])
ax.scatter(np.random.randn(10), np.random.randn(10))
ax.set_title('第%d行,第%d列'%(i+1,j+1))
if i==1:
ax.set_xlabel('横坐标')
if j==0:
ax.set_ylabel('纵坐标')
fig.tight_layout()
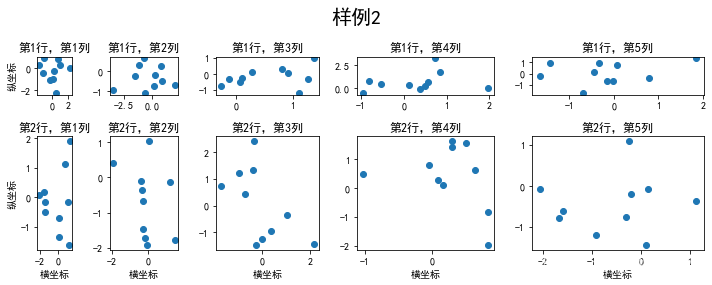
fig = plt.figure(figsize=(10, 4))
spec = fig.add_gridspec(nrows=2, ncols=6, width_ratios=[2,2.5,3,1,1.5,2], height_ratios=[1,2])
fig.suptitle('样例3', size=20,x=0.5,y=1)
ax = fig.add_subplot(spec[0, :3])
ax.scatter(np.random.randn(10), np.random.randn(10))
ax = fig.add_subplot(spec[0, 3:5])
ax.scatter(np.random.randn(10), np.random.randn(10))
ax = fig.add_subplot(spec[:, 5])
ax.scatter(np.random.randn(10), np.random.randn(10))
ax = fig.add_subplot(spec[1, 0])
ax.scatter(np.random.randn(10), np.random.randn(10))
ax = fig.add_subplot(spec[1, 1:5])
ax.scatter(np.random.randn(10), np.random.randn(10))
fig.tight_layout()
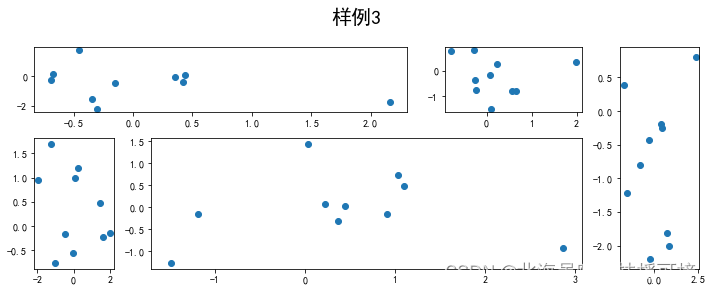
2、子图上的方法
补充介绍一些子图上的方法
常用直线的画法为: axhline, axvline, axline (水平、垂直、任意方向)
matplotlib库的pyplot模块中的axhline()函数用于在轴上添加一条水平线。
用法: matplotlib.pyplot.axhline(y=0, xmin=0, xmax=1, **kwargs)
参数:此方法接受以下描述的参数:
y:该参数是可选的,它是在水平线的数据坐标中的位置。 xmin:此参数是标量,是可选的。其默认值为0。 xmax:此参数是标量,是可选的。默认值为1。 返回值:这将返回以下内容:
line:这将返回此函数创建的行。
axline函数的签名为: matplotlib.pyplot.axline(xy1, xy2=None, *, slope=None, **kwargs) 其中:
xy1:直线贯穿的点的坐标。浮点数二元组(float, float)。 xy2:直线贯穿的点的坐标。浮点数二元组(float, float)。默认值为None。xy2和slope同时只能有一个值有效。两者既不能同时为None,也不能同时为非空值。
slope:直线的斜率。浮点数,默认值为None。 **kwargs:除’transform’之外的Line2D属性。 axline函数有两种应用模式: axline(xy1, xy2) axline(xy1, slope)
axline函数的返回值为Line2D对象。
fig, ax = plt.subplots(figsize=(4,3))
ax.axhline(0.5,0.2,0.8)
ax.axvline(0.5,0.2,0.8)
ax.axline([0.3,0.3],[0.7,0.7])
<matplotlib.lines.Line2D at 0x1b6f930f7c0>
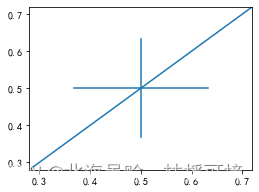
fig, ax = plt.subplots(figsize=(4,3))
ax.grid(True)
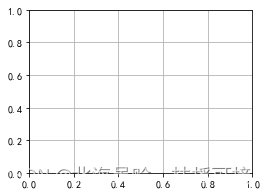
fig, axs = plt.subplots(1, 2, figsize=(10, 4))
for j in range(2):
axs[j].plot(list('abcd'), [10**i for i in range(4)])
if j==0:
axs[j].set_yscale('log')
else:
pass
fig.tight_layout()
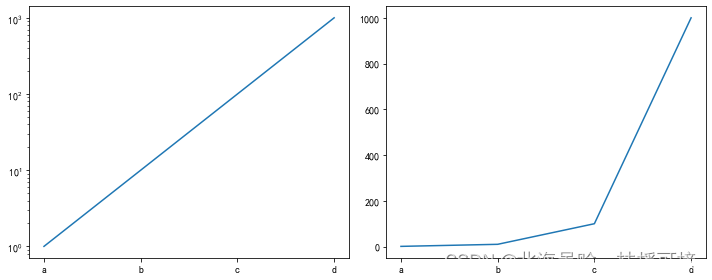
3、思考题
3.1、墨尔本1981年至1990年的每月温度情况
import csv
from matplotlib.pyplot import MultipleLocator
tem = []
with open('fantastic-matplotlib-main\data\layout_ex1.csv', 'r') as f:
reader = csv.reader(f)
for i in reader:
tem.append(i[1])
del tem[0]
temperature = [round(float(i)) for i in tem]
fig, axs = plt.subplots(2, 5, figsize=(15, 4), sharex=False, sharey=True)
fig.suptitle('墨尔本1981年至1990年的每月温度曲线', size=10)
k = 0
year = list(range(1981,1991))
x = list(range(1,13))
for i in range(2):
for j in range(5):
y = temperature[k*12:(k+1)*12]
axs[i][j].plot(x,y,marker='*')
axs[i][j].set_title('%d年' %(year[k]),size=10)
k += 1
axs[i][j].set_xlim(0.5,12.5)
axs[i][j].set_ylim(4.5,19.5)
if j==0:
axs[i][j].set_ylabel('气温')
fig.tight_layout()
fig.savefig('test4.png',dpi=400,bbox_inches='tight',transparent=True)
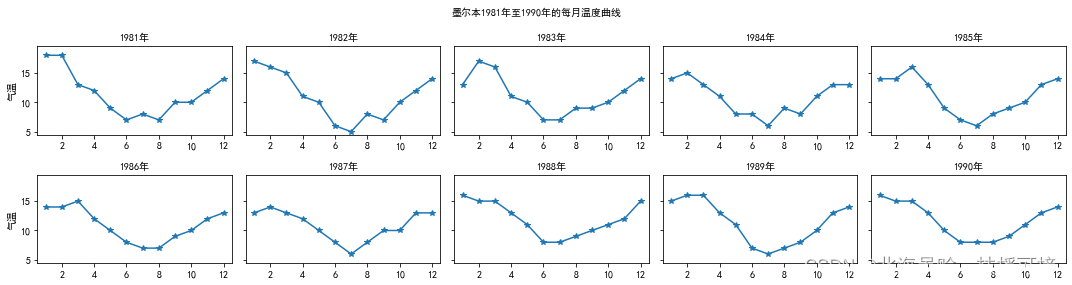
3.2、画出数据的散点图和边际分布
用 np.random.randn(2, 150) 生成一组二维数据,使用两种非均匀子图的分割方法,做出该数据对应的散点图和边际分布图
fig = plt.figure(figsize=(10, 10))
spec = fig.add_gridspec(nrows=2, ncols=2, width_ratios=[4,1], height_ratios=[1,4])
x = np.random.randn(2, 150)
ax1 = fig.add_subplot(spec[0, 0])
ax1.hist(x[0],width=0.4)
ax1.patch.set_edgecolor('g')
plt.axis('off')
ax2 = fig.add_subplot(spec[1, 0])
ax2.scatter(x[0],x[1])
ax2.grid(True)
ax2.set_xlabel('my_data_x')
ax2.set_ylabel('my_data_y')
ax3 = fig.add_subplot(spec[1, 1])
ax3.hist(x[1],orientation='horizontal')
plt.axis('off')
fig.tight_layout()
fig.savefig('test5.png',dpi=400,bbox_inches='tight',transparent=True)
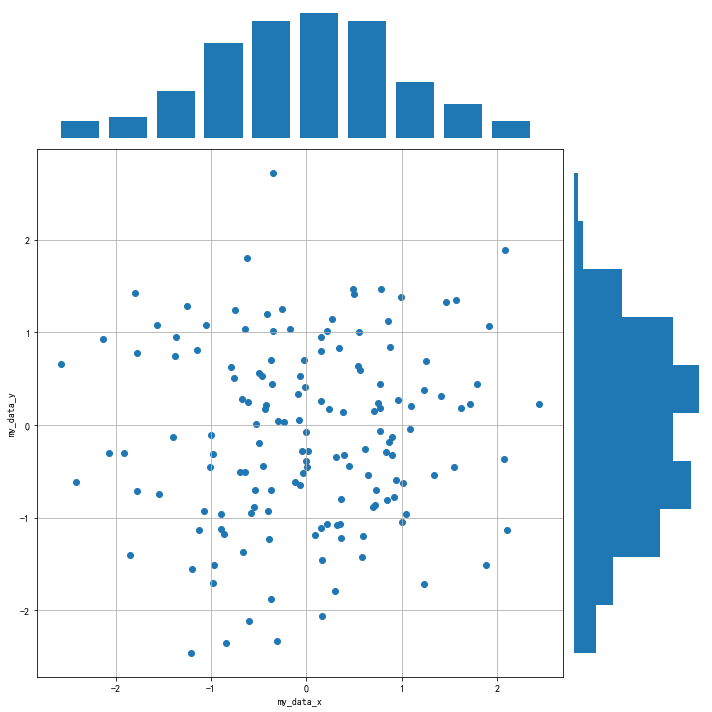
fig = plt.figure(figsize=(10, 10))
spec = fig.add_gridspec(nrows=5, ncols=5, width_ratios=[1,1,1,1,1], height_ratios=[1,1,1,1,1])
x = np.random.randn(2, 150)
ax1 = fig.add_subplot(spec[0, :4])
ax1.hist(x[0],width=0.4)
ax1.patch.set_edgecolor('g')
plt.axis('off')
ax2 = fig.add_subplot(spec[1: , :4])
ax2.scatter(x[0],x[1])
ax2.grid(True)
ax2.set_xlabel('my_data_x')
ax2.set_ylabel('my_data_y')
ax3 = fig.add_subplot(spec[1:, -1])
ax3.hist(x[1],alpha=1,orientation='horizontal')
plt.axis('off')
fig.tight_layout()
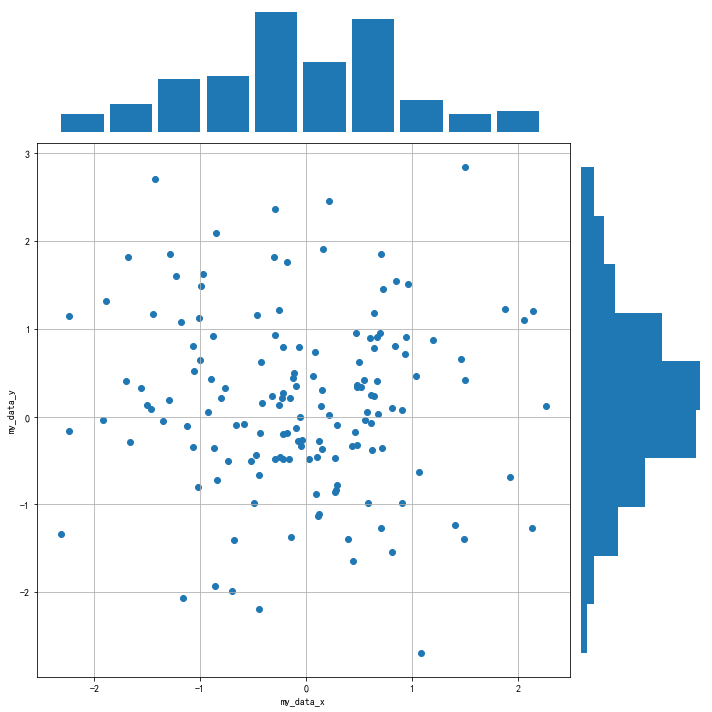
fig=sns.jointplot(x[0], x[1])
fig=fig.plot_joint(plt.scatter)
fig=fig.plot_marginals(sns.distplot,kde=False)
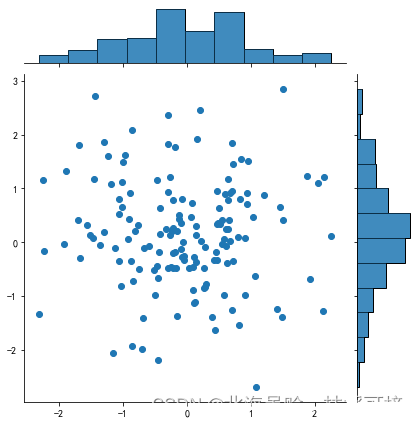
total = float(len(x[0]))
fig=sns.jointplot(x[0], x[1])
for p in fig.ax_marg_x.patches:
height = p.get_height()
fig.ax_marg_x.text(p.get_x()+p.get_width()/2.,height,
'{:1.0f}'.format((height/total)*100), ha="center")
for p in fig.ax_marg_y.patches:
width = p.get_width()
fig.ax_marg_y.text(p.get_x()+p.get_width(),p.get_y()+p.get_height()/2.,
'{:1.0f}'.format((width/total)*100), va="center")
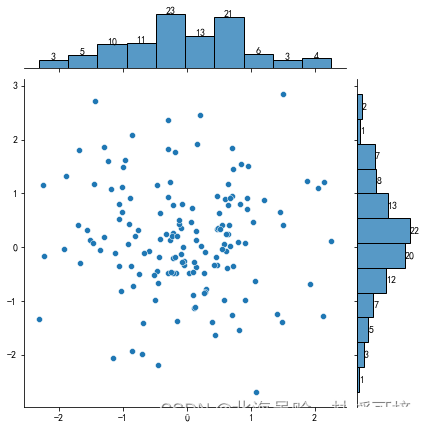
import seaborn as sns
with sns.axes_style("dark"):
sns.jointplot(x[0], x[1], kind="hex")
fig.savefig('test6.png',dpi=400,bbox_inches='tight',transparent=True)
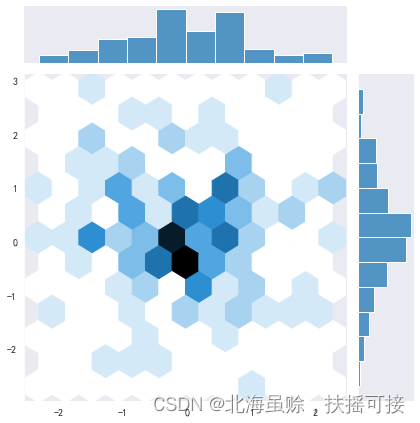
|