教程来源:https://datawhalechina.github.io/fantastic-matplotlib
参考资料:
1、Python数据可视化matplotlib:第五回:样式色彩秀芳华_林遠夏的博客-程序员秘密:https://url.cy/wryWK2
2、学会这6个可视化配色基本技巧,还原数据本身的意义:https://zhuanlan.zhihu.com/p/88892542
3、RGB颜色和HEX颜色之间是可以一一对应的,以下网址提供了两种色彩表示方法的转换工具:https://www.colorhexa.com/
4、[matplotlib] 颜色设置及Matplotlib颜色对照表:https://zhuanlan.zhihu.com/p/65220518
5、matplotlib基本颜色演示:https://www.matplotlib.org.cn/gallery/color/color_demo.html
6、五种colormap的字符串表示和颜色图的对应关系:https://matplotlib.org/stable/tutorials/colors/colormaps.html
7、rcParam支持的参数列表可以参照官方文档的相关说明: https://matplotlib.org/stable/api/matplotlib_configuration_api.html?highlight=rcparams#matplotlib.rcParams
import matplotlib as mpl
import matplotlib.pyplot as plt
import numpy as np
详细介绍matplotlib中样式和颜色的使用。 绘图样式和颜色是丰富可视化图表的重要手段,因此熟练掌握本章可以让可视化图表变得更美观,突出重点和凸显艺术性。
关于绘图样式,常见的有3种方法,分别是修改预定义样式,自定义样式和rcparams。
关于颜色使用,本章介绍了常见的5种表示单色颜色的基本方法,以及colormap多色显示的方法。
1、matplotlib的绘图样式(style)
在matplotlib中,要想设置绘制样式,最简单的方法是在绘制元素时单独设置样式。 但是有时候,当用户在做专题报告时,往往会希望保持整体风格的统一而不用对每张图一张张修改,因此matplotlib库还提供了四种批量修改全局样式的方式
1.1、matplotlib预先定义样式
matplotlib贴心地提供了许多内置的样式供用户使用,使用方法很简单,只需在python脚本的最开始输入想使用style的名称即可调用,尝试调用不同内置样式,比较区别
matplotlib究竟内置了那些样式供使用呢?总共以下26种丰富的样式可供选择,如下:
plt.style.use('default')
plt.plot([1,2,3,4],[2,3,4,5]);
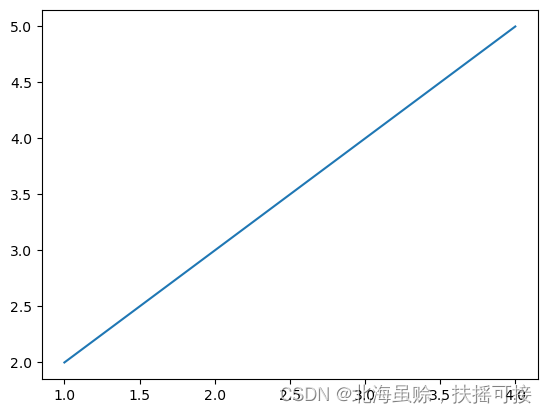
plt.style.use('ggplot')
plt.plot([1,2,3,4],[2,3,4,5]);
![[外链图片转存失败,源站可能有防盗链机制,建议将图片保存下来直接上传(img-3DvOZu8K-1664085596499)(output_7_0.png)]](https://img-blog.csdnimg.cn/3691540b13c44db29ac5c44ec53a1cc2.png#pic_center)
print(plt.style.available)
len(plt.style.available)
['Solarize_Light2', '_classic_test_patch', '_mpl-gallery', '_mpl-gallery-nogrid', 'bmh', 'classic', 'dark_background', 'fast', 'fivethirtyeight', 'ggplot', 'grayscale', 'seaborn', 'seaborn-bright', 'seaborn-colorblind', 'seaborn-dark', 'seaborn-dark-palette', 'seaborn-darkgrid', 'seaborn-deep', 'seaborn-muted', 'seaborn-notebook', 'seaborn-paper', 'seaborn-pastel', 'seaborn-poster', 'seaborn-talk', 'seaborn-ticks', 'seaborn-white', 'seaborn-whitegrid', 'tableau-colorblind10']
28
fig = plt.figure(figsize = (60, 30))
for i in range(len(plt.style.available)):
plt.subplot(4, 7, i+1)
plt.title(plt.style.available[i],fontsize=50,color='k')
plt.style.use(plt.style.available[i])
plt.plot([1,2,3,4],[2,3,4,5])
fig.suptitle('style',fontsize=100, color='b',fontweight='bold')
plt.tight_layout()
plt.show()
![[外链图片转存失败,源站可能有防盗链机制,建议将图片保存下来直接上传(img-h6AvuRws-1664085596499)(output_9_0.png)]](https://img-blog.csdnimg.cn/2e09022cf0634a6e9015f0eaaa62f27a.png#pic_center)
1.2、用户自定义stylesheet
在任意路径下创建一个后缀名为mplstyle的样式清单,编辑文件添加以下样式内容:
axes.titlesize : 24 axes.labelsize : 20 lines.linewidth : 3 lines.markersize : 10 xtick.labelsize : 16 ytick.labelsize : 16
plt.style.use('presentation.mplstyle')
plt.plot([1,2,3,4],[2,3,4,5]);
![[外链图片转存失败,源站可能有防盗链机制,建议将图片保存下来直接上传(img-5VdBJD7L-1664085596499)(output_12_0.png)]](https://img-blog.csdnimg.cn/8367d3573cdf44718967a6ee4ef9fa52.png#pic_center)
plt.style.use(['dark_background', 'presentation.mplstyle'])
plt.plot([1,2,3,4],[2,3,4,5]);
![[外链图片转存失败,源站可能有防盗链机制,建议将图片保存下来直接上传(img-BBpz7PDV-1664085596499)(output_13_0.png)]](https://img-blog.csdnimg.cn/040f52e66c5f4960815c3f779da6a0fb.png#pic_center)
1.3、设置rcparams
我们还可以通过修改默认rc设置的方式改变样式,所有rc设置都保存在一个叫做 matplotlib.rcParams的变量中。
修改过后再绘图,可以看到绘图样式发生了变化。
plt.style.use('default')
plt.plot([1,2,3,4],[2,3,4,5]);
![[外链图片转存失败,源站可能有防盗链机制,建议将图片保存下来直接上传(img-uKXFYb6C-1664085596500)(output_15_0.png)]](https://img-blog.csdnimg.cn/222839fe997b47d6a113ae0bd033aa43.png#pic_center)
mpl.rcParams['lines.linewidth'] = 2
mpl.rcParams['lines.linestyle'] = '--'
plt.plot([1,2,3,4],[2,3,4,5]);
![[外链图片转存失败,源站可能有防盗链机制,建议将图片保存下来直接上传(img-el5Acjl3-1664085596500)(output_16_0.png)]](https://img-blog.csdnimg.cn/5555a4736bbd41f99ec64b9aec7290bb.png#pic_center)
另外matplotlib也还提供了一种更便捷的修改样式方式,可以一次性修改多个样式。
mpl.rc('lines', linewidth=4, linestyle='-.')
plt.plot([1,2,3,4],[2,3,4,5]);
![[外链图片转存失败,源站可能有防盗链机制,建议将图片保存下来直接上传(img-1P6IOqAm-1664085596501)(output_18_0.png)]](https://img-blog.csdnimg.cn/a630783a22cc459b9ec2e1efee882926.png#pic_center)
mpl.rc('lines', linewidth=4, linestyle='-.')
mpl.rc('axes', titlesize = 24, labelsize = 30)
plt.xlabel('xlabel')
plt.title("This is title")
plt.plot([1,2,3,4],[2,3,4,5]);
![[外链图片转存失败,源站可能有防盗链机制,建议将图片保存下来直接上传(img-NnsMC8cM-1664085596501)(output_19_0.png)]](https://img-blog.csdnimg.cn/376cb38deffc4181ad847e65f8b974c8.png#pic_center)
1.4、修改 matplotlibrc
由于matplotlib是使用matplotlibrc文件来控制样式的,也就是上一节提到的rc setting,所以我们还可以通过修改matplotlibrc文件的方式改变样式。
mpl.matplotlib_fname()
'E:\\Anaconda3\\envs\\pytorch\\lib\\site-packages\\matplotlib\\mpl-data\\matplotlibrc'
![[外链图片转存失败,源站可能有防盗链机制,建议将图片保存下来直接上传(img-S5pFXvkm-1664085596501)(attachment:image.png)]](https://img-blog.csdnimg.cn/152e9d77477942918957f39c943b1b7d.png#pic_cente)
2、matplotlib的色彩设置(color)
在可视化中,如何选择合适的颜色和搭配组合也是需要仔细考虑的,色彩选择要能够反映出可视化图像的主旨。 从可视化编码的角度对颜色进行分析,可以将颜色分为色相、亮度和饱和度三个视觉通道。
通常来说:
色相: 没有明显的顺序性、一般不用来表达数据量的高低,而是用来表达数据列的类别。
明度和饱和度: 在视觉上很容易区分出优先级的高低、被用作表达顺序或者表达数据量视觉通道。
具体关于色彩理论部分的知识,不属于本教程的重点,请参阅有关拓展材料学习:https://zhuanlan.zhihu.com/p/88892542
2.1、RGB或RGBA
plt.style.use('default')
plt.plot([1,2,3],[4,5,6],color=(0.1, 0.2, 0.5))
plt.plot([4,5,6],[1,2,3],color=(0.1, 0.2, 0.5, 0.5));
![[外链图片转存失败,源站可能有防盗链机制,建议将图片保存下来直接上传(img-e5vw2hjr-1664085596501)(output_26_0.png)]](https://img-blog.csdnimg.cn/73d4fa596bc248d08859a690f4ab4cc5.png#pic_center)
2.2、HEX RGB 或 RGBA
plt.plot([1,2,3],[4,5,6],color='#0f0f0f')
plt.plot([4,5,6],[1,2,3],color='#0f0f0f80');
![[外链图片转存失败,源站可能有防盗链机制,建议将图片保存下来直接上传(img-DDdMPaKR-1664085596502)(output_28_0.png)]](https://img-blog.csdnimg.cn/0c5296e9ecbf4a7dbfec879090ad43ef.png#pic_center)
RGB颜色和HEX颜色之间是可以一一对应的,以下网址提供了两种色彩表示方法的转换工具:https://www.colorhexa.com/
2.3、灰度色阶
plt.plot([1,2,3],[4,5,6],color='0.5');
![[外链图片转存失败,源站可能有防盗链机制,建议将图片保存下来直接上传(img-QtzPJJdz-1664085596502)(output_31_0.png)]](https://img-blog.csdnimg.cn/f9cea69ba8044a9ab7c65be1d99df246.png#pic_center)
2.4、单字符基本颜色
plt.plot([1,2,3],[4,5,6],color='m');
![[外链图片转存失败,源站可能有防盗链机制,建议将图片保存下来直接上传(img-auZ53ryg-1664085596502)(output_33_0.png)]](https://img-blog.csdnimg.cn/43ef7ba7da694038aceba271e72f923c.png#pic_center)
2.5、颜色名称
plt.plot([1,2,3],[4,5,6],color='tan');
![[外链图片转存失败,源站可能有防盗链机制,建议将图片保存下来直接上传(img-eLJz0yVn-1664085596503)(output_35_0.png)]](https://img-blog.csdnimg.cn/fe5a9668d0d84a449d82185a6ec93c70.png#pic_center)
![[外链图片转存失败,源站可能有防盗链机制,建议将图片保存下来直接上传(img-rmE0DrPQ-1664085596503)(attachment:image.png)]](https://img-blog.csdnimg.cn/1368d2dbb5634041a1491126f0da1673.png#pic_center)
![[外链图片转存失败,源站可能有防盗链机制,建议将图片保存下来直接上传(img-fFRNzLSh-1664085596503)(attachment:image.png)]](https://img-blog.csdnimg.cn/6c8aaac9c32e490396f9abc7c6748446.png#pic_center)
2.6、使用colormap设置一组颜色
有些图表支持使用colormap的方式配置一组颜色,从而在可视化中通过色彩的变化表达更多信息。
在matplotlib中,colormap共有五种类型:
顺序(Sequential):通常使用单一色调,逐渐改变亮度和颜色渐渐增加,用于表示有顺序的信息
发散(Diverging):改变两种不同颜色的亮度和饱和度,这些颜色在中间以不饱和的颜色相遇;当绘制的信息具有关键中间值(例如地形)或数据偏离零时,应使用此值。
循环(Cyclic):改变两种不同颜色的亮度,在中间和开始/结束时以不饱和的颜色相遇。用于在端点处环绕的值,例如相角,风向或一天中的时间。
定性(Qualitative):常是杂色,用来表示没有排序或关系的信息。
杂色(Miscellaneous):一些在特定场景使用的杂色组合,如彩虹,海洋,地形等。
在以下官网页面可以查询上述五种colormap的字符串表示和颜色图的对应关系:https://matplotlib.org/stable/tutorials/colors/colormaps.html
import numpy as np
import matplotlib as mpl
import matplotlib.pyplot as plt
from colorspacious import cspace_converter
cmaps = {}
gradient = np.linspace(0, 1, 256)
gradient = np.vstack((gradient, gradient))
def plot_color_gradients(category, cmap_list):
nrows = len(cmap_list)
figh = 0.35 + 0.15 + (nrows + (nrows - 1) * 0.1) * 0.22
fig, axs = plt.subplots(nrows=nrows + 1, figsize=(6.4, figh))
fig.subplots_adjust(top=1 - 0.35 / figh, bottom=0.15 / figh,
left=0.2, right=0.99)
axs[0].set_title(f'{category} colormaps', fontsize=14)
for ax, name in zip(axs, cmap_list):
ax.imshow(gradient, aspect='auto', cmap=mpl.colormaps[name])
ax.text(-0.01, 0.5, name, va='center', ha='right', fontsize=10,
transform=ax.transAxes)
for ax in axs:
ax.set_axis_off()
cmaps[category] = cmap_list
2.6.1、顺序(Sequential)
plot_color_gradients('Perceptually Uniform Sequential',
['viridis', 'plasma', 'inferno', 'magma', 'cividis'])
![[外链图片转存失败,源站可能有防盗链机制,建议将图片保存下来直接上传(img-iwbiLOUR-1664085596504)(output_43_0.png)]](https://img-blog.csdnimg.cn/8c2980bd6f6a4811960c7ea243dc509d.png#pic_center)
plot_color_gradients('Sequential',
['Greys', 'Purples', 'Blues', 'Greens', 'Oranges', 'Reds',
'YlOrBr', 'YlOrRd', 'OrRd', 'PuRd', 'RdPu', 'BuPu',
'GnBu', 'PuBu', 'YlGnBu', 'PuBuGn', 'BuGn', 'YlGn'])
![[外链图片转存失败,源站可能有防盗链机制,建议将图片保存下来直接上传(img-Bz9zhaYl-1664085596504)(output_44_0.png)]](https://img-blog.csdnimg.cn/6435a9d18411406183a3a787b2a24496.png#pic_center)
plot_color_gradients('Sequential (2)',
['binary', 'gist_yarg', 'gist_gray', 'gray', 'bone',
'pink', 'spring', 'summer', 'autumn', 'winter', 'cool',
'Wistia', 'hot', 'afmhot', 'gist_heat', 'copper'])
![[外链图片转存失败,源站可能有防盗链机制,建议将图片保存下来直接上传(img-pNA0qdA3-1664085596504)(output_45_0.png)]](https://img-blog.csdnimg.cn/275018bd6e0b4ebaa71f41acd2dfea43.png#pic_center)
2.6.2、发散(Diverging)
plot_color_gradients('Diverging',
['PiYG', 'PRGn', 'BrBG', 'PuOr', 'RdGy', 'RdBu', 'RdYlBu',
'RdYlGn', 'Spectral', 'coolwarm', 'bwr', 'seismic'])
![[外链图片转存失败,源站可能有防盗链机制,建议将图片保存下来直接上传(img-QpoVU7ww-1664085596504)(output_47_0.png)]](https://img-blog.csdnimg.cn/18d2c2fd79dd4f8abaa7a45e8f58ea37.png#pic_center)
2.6.3、循环(Cyclic)
plot_color_gradients('Cyclic', ['twilight', 'twilight_shifted', 'hsv'])
![[外链图片转存失败,源站可能有防盗链机制,建议将图片保存下来直接上传(img-9mXPj4Ss-1664085596505)(output_49_0.png)]](https://img-blog.csdnimg.cn/0b803c57b14a4b0f8476abe442a99d7e.png#pic_center)
2.6.4、定性(Qualitative)
plot_color_gradients('Qualitative',
['Pastel1', 'Pastel2', 'Paired', 'Accent', 'Dark2',
'Set1', 'Set2', 'Set3', 'tab10', 'tab20', 'tab20b',
'tab20c'])
![[外链图片转存失败,源站可能有防盗链机制,建议将图片保存下来直接上传(img-PuVUelfI-1664085596505)(output_51_0.png)]](https://img-blog.csdnimg.cn/ec6f65ff3b4b4df6998261c957ce5b4e.png#pic_center)
2.6.5、杂色(Miscellaneous)
plot_color_gradients('Miscellaneous',
['flag', 'prism', 'ocean', 'gist_earth', 'terrain',
'gist_stern', 'gnuplot', 'gnuplot2', 'CMRmap',
'cubehelix', 'brg', 'gist_rainbow', 'rainbow', 'jet',
'turbo', 'nipy_spectral', 'gist_ncar'])
plt.show()
![[外链图片转存失败,源站可能有防盗链机制,建议将图片保存下来直接上传(img-I9W8pWvR-1664085596505)(output_53_0.png)]](https://img-blog.csdnimg.cn/2c4b493f958d4618aeb0f9dc9ccb7589.png#pic_center)
x = np.random.randn(50)
y = np.random.randn(50)
plt.scatter(x,y,c=x,cmap='RdPu');
plt.scatter(x,y,c=x,cmap='flag');
![[外链图片转存失败,源站可能有防盗链机制,建议将图片保存下来直接上传(img-yqQYwjyC-1664085596505)(output_54_0.png)]](https://img-blog.csdnimg.cn/9ce3751ea74741efbe6711b7690cffba.png#pic_center)
3、思考题
学习如何自定义colormap,并将其应用到任意一个数据集中,绘制一幅图像,注意colormap的类型要和数据集的特性相匹配,并做简单解释
import pandas as pd
import numpy as np
import matplotlib.pyplot as plt
from sklearn.datasets import load_iris
from sklearn.model_selection import train_test_split
iris_dataset = load_iris()
iris = load_iris()
X = iris.data[:,:2]
y = iris.target
x_min,x_max = X[:,0].min()-.5, X[:,0].max()+.5
y_min,y_max = X[:,1].min()-.5, X[:,1].max()+.5
plt.figure(2,figsize=(8,6))
plt.clf()
plt.scatter(X[:,0],X[:,1],c=y,cmap=plt.cm.Set1,edgecolor='k')
plt.xlabel('Sepal length')
plt.ylabel('Sepal width')
plt.xlim(x_min, x_max)
plt.ylim(y_min, y_max)
plt.xticks(())
plt.yticks(())
plt.show()
![[外链图片转存失败,源站可能有防盗链机制,建议将图片保存下来直接上传(img-TM7jF0xj-1664085596506)(output_57_0.png)]](https://img-blog.csdnimg.cn/a09d56326e9e4559b0662e2de0be2170.png#pic_center)
使用 matplotlib 自定义Colormap
自定义 colormap 通常要使用 matplotlib.colors 模块中提供的函数和方法。
matplotlib.colors 是用来转换数字列表或颜色参数为 RGB 或 RGBA 的模块。RGB 和 RGBA 是具有3个或4个浮点数且数值在 [0, 1] 之间的序列。
创建 colormap 时通常需要以下两步:
1、使用 Normalize 实例或子类将数据数组归一化为 [0 1]之间的数组
2、使用 Colormap 子类的实例进行数据和颜色的映射
模块中提供了以下两个函数创建 colormap:
LinearSegmentedColormap :有内置 colormap 实例均由此函数创建,但也可以自定义colormap
ListedColormap :从颜色列表创建 colormap
3.1、使用 LinearSegmentedColormap 的 from_list 方法创建 colormap
参考链接:
python colormap_Python matplotlib的使用并自定义colormap的方法:https://blog.csdn.net/weixin_39739661/article/details/110753542
使用 matplotlib 自定义Colormap:https://cloud.tencent.com/developer/article/1618345
import matplotlib.pyplot as plt
import matplotlib.image as mpimg
import numpy as np
lena = mpimg.imread('img_188.jpg')
lena.shape
plt.imshow(lena)
plt.axis('off')
(-0.5, 4023.5, 3035.5, -0.5)
![[外链图片转存失败,源站可能有防盗链机制,建议将图片保存下来直接上传(img-PJMm2wQX-1664085596506)(output_62_1.png)]](https://img-blog.csdnimg.cn/b864701b4c3145fe94693a92bddc56c9.png#pic_center)
from matplotlib.colors import LinearSegmentedColormap
import random
import matplotlib.image as mpimg
colors = [(1, 0, 0), (0, 1, 0), (0, 0, 1)]
n_bins = [3, 6, 10, 100]
cmap_name = 'my_cmap'
fig, axs = plt.subplots(2, 2, figsize=(6, 9))
fig.subplots_adjust(left=0.02, bottom=0.06, right=0.95, top=0.94, wspace=0.05)
data=mpimg.imread('img_188.jpg')
for n_bin, ax in zip(n_bins, axs.ravel()):
cm = LinearSegmentedColormap.from_list(cmap_name, colors, N=n_bin)
im = ax.imshow(data, interpolation='nearest', origin='lower', cmap=cm)
ax.set_title("N bins: %s" % n_bin)
fig.colorbar(im, ax=ax)
plt.axis('off')
plt.show()
![[外链图片转存失败,源站可能有防盗链机制,建议将图片保存下来直接上传(img-MWm6aJNl-1664085596507)(output_63_0.png)]](https://img-blog.csdnimg.cn/4ada40ebb4fb413e9bf4a0d480b70ea5.png#pic_center)
from PIL import Image
im=Image.open("img_188.jpg")
im1 = im.convert('L')
plt.subplot(1,2,1)
plt.imshow(im)
plt.axis('off')
plt.subplot(1,2,2)
plt.imshow(im1);
plt.axis('off')
(-0.5, 4023.5, 3035.5, -0.5)
![[外链图片转存失败,源站可能有防盗链机制,建议将图片保存下来直接上传(img-7rYd4TZg-1664085596507)(output_64_1.png)]](https://img-blog.csdnimg.cn/eed38a0e6bfd4d06ae9a600d48a702a1.png#pic_center)
import matplotlib.pyplot as plt
import matplotlib.image as mpimg
import matplotlib as mpl
from PIL import Image
import numpy as np
def colormap():
return mpl.colors.LinearSegmentedColormap.from_list('cmap',
['#FFFFFF', '#98F5FF', '#00FF00', '#FFFF00','#FF0000', '#8B0000'],
256)
plt.subplot(1, 2, 1)
data=mpimg.imread('img_188.jpg')
plt.imshow(data);
plt.subplot(1, 2, 2)
plt.imsave('colormap.jpg',data, cmap=colormap())
plt.imshow(data, cmap=colormap());
![[外链图片转存失败,源站可能有防盗链机制,建议将图片保存下来直接上传(img-hrMEuBdv-1664085596507)(output_65_0.png)]](https://img-blog.csdnimg.cn/f2e68484971d4e8790d0ae766cc80f60.png#pic_center)
3.2、使用 ListedColormap 自定义 colormap
import numpy as np
import matplotlib.pyplot as plt
import matplotlib as mpl
import matplotlib.colors as colors
def colormap():
cdict = ['#FFFFFF', '#9ff113', '#5fbb44', '#f5f329', '#e50b32']
return colors.ListedColormap(cdict, 'indexed')
data=mpimg.imread('img_188.jpg')
fig = plt.figure()
my_cmap = colormap()
ax = fig.add_subplot(221)
ax.imshow(data)
ax = fig.add_subplot(222)
cmap = mpl.cm.bwr
ax.imshow(data, cmap=cmap)
ax = fig.add_subplot(223)
cmap = mpl.cm.winter
im = ax.imshow(data, cmap=my_cmap)
plt.colorbar(im)
ax = fig.add_subplot(224)
cmap = mpl.cm.rainbow
norm = mpl.colors.Normalize(vmin=-1, vmax=1)
im=ax.imshow(data,cmap=cmap)
plt.colorbar(im,cmap=cmap, norm=norm,ticks=[-1,0,1])
plt.show()
![[外链图片转存失败,源站可能有防盗链机制,建议将图片保存下来直接上传(img-tj7FM8rp-1664085596507)(output_67_0.png)]](https://img-blog.csdnimg.cn/bd2dd394dc134bfb9664960f331bca9a.png#pic_center)
|