- 过程推导 - 了解BP原理
- 数值计算 - 手动计算,掌握细节
- 代码实现 - numpy手推 + pytorch自动
代码实现:
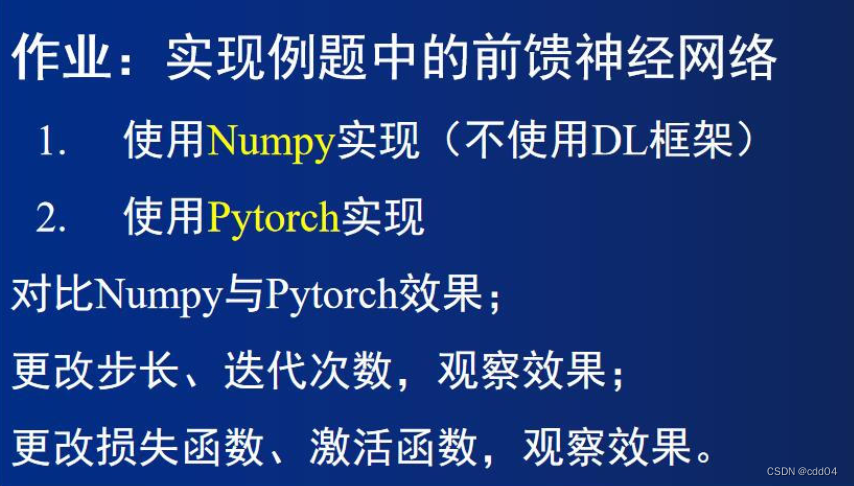
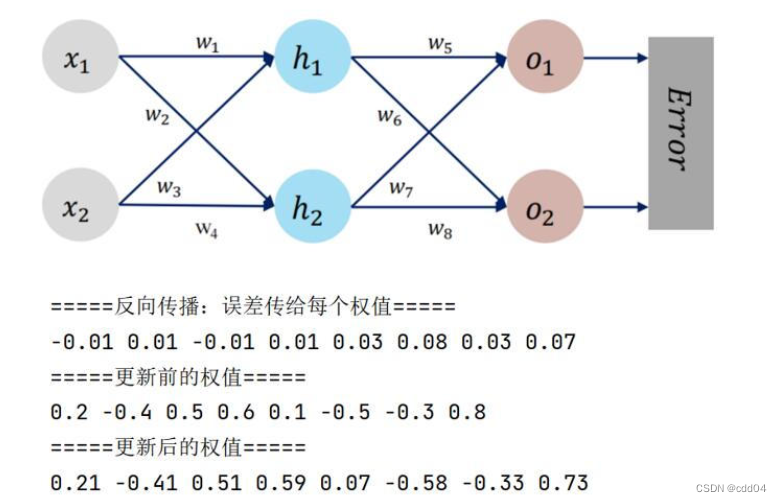
- ?对比【numpy】和【pytorch】程序,总结并陈述。
- 激活函数Sigmoid用PyTorch自带函数torch.sigmoid(),观察、总结并陈述。
- 激活函数Sigmoid改变为Relu,观察、总结并陈述。
- 损失函数MSE用PyTorch自带函数 t.nn.MSELoss()替代,观察、总结并陈述。
- 损失函数MSE改变为交叉熵,观察、总结并陈述。
- 改变步长,训练次数,观察、总结并陈述。
- 权值w1-w8初始值换为随机数,对比“指定权值”的结果,观察、总结并陈述。
- 权值w1-w8初始值换为0,观察、总结并陈述。
- 全面总结反向传播原理和编码实现,认真写心得体会。
?
过程推导:
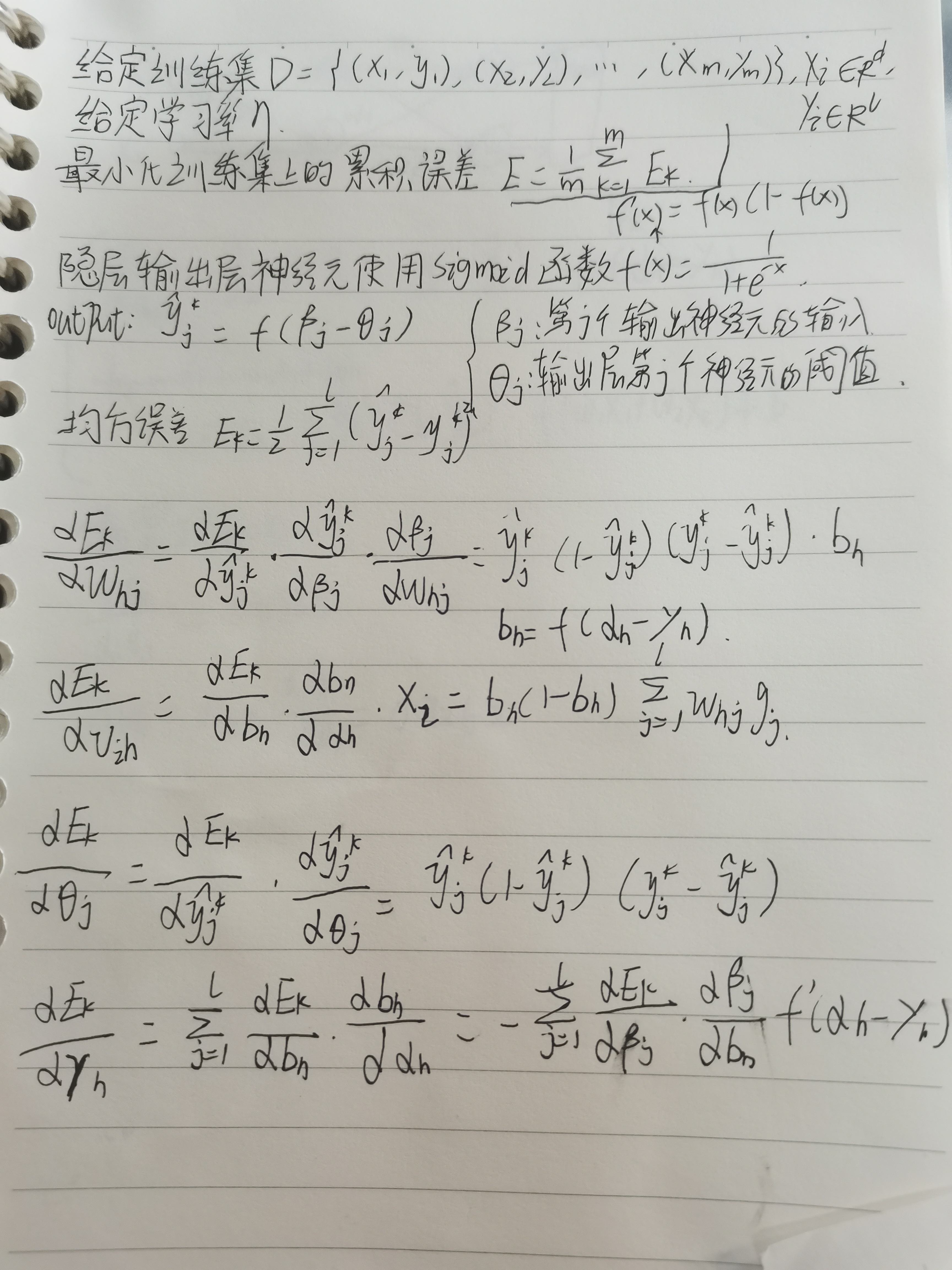
数值计算:
?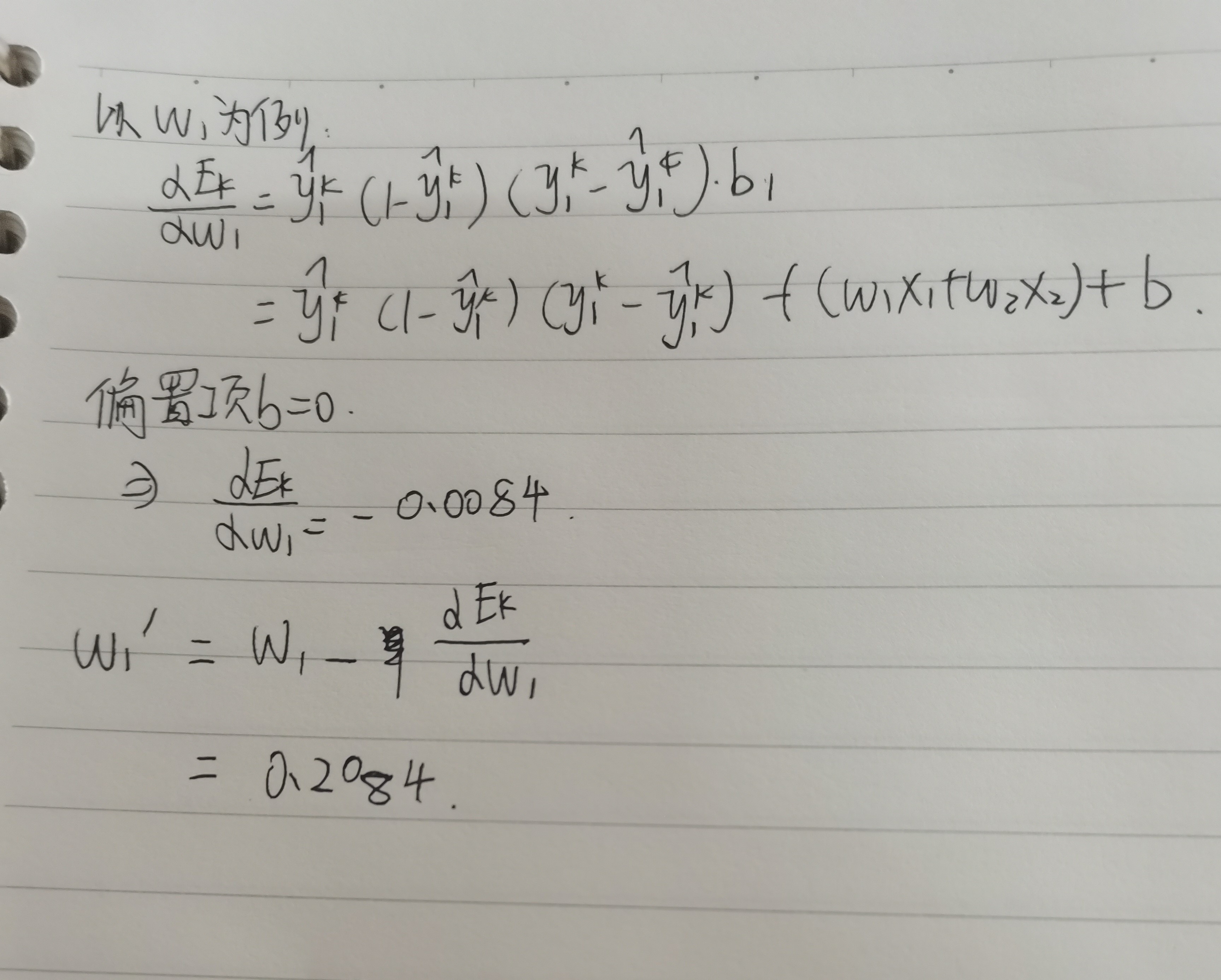
?代码实现:
使用numpy实现
import numpy as np
w1, w2, w3, w4, w5, w6, w7, w8 = 0.2, -0.4, 0.5, 0.6, 0.1, -0.5, -0.3, 0.8
x1, x2 = 0.5, 0.3
y1, y2 = 0.23, -0.07
print("输入值 x0, x1:")
print(x1, x2)
print("输出值 y0, y1:")
print(y1, y2)
def sigmoid(z):
a = 1 / (1 + np.exp(-z))
return a
def forward_propagate(x1, x2, y1, y2, w1, w2, w3, w4, w5, w6, w7, w8):
in_h1 = w1 * x1 + w3 * x2
out_h1 = sigmoid(in_h1)
in_h2 = w2 * x1 + w4 * x2
out_h2 = sigmoid(in_h2)
in_o1 = w5 * out_h1 + w7 * out_h2
out_o1 = sigmoid(in_o1)
in_o2 = w6 * out_h1 + w8 * out_h2
out_o2 = sigmoid(in_o2)
print("正向计算:预测值o1 ,o2为")
print(round(out_o1, 5), round(out_o2, 5))
error = (1 / 2) * (out_o1 - y1) ** 2 + (1 / 2) * (out_o2 - y2) ** 2
print("损失函数(均方误差):",round(error, 5))
return out_o1, out_o2, out_h1, out_h2
def back_propagate(out_o1, out_o2, out_h1, out_h2):
# 反向传播
d_o1 = out_o1 - y1
d_o2 = out_o2 - y2
d_w5 = d_o1 * out_o1 * (1 - out_o1) * out_h1
d_w7 = d_o1 * out_o1 * (1 - out_o1) * out_h2
d_w6 = d_o2 * out_o2 * (1 - out_o2) * out_h1
d_w8 = d_o2 * out_o2 * (1 - out_o2) * out_h2
d_w1 = (d_o1 * out_h1 * (1 - out_h1) * w5 + d_o2 * out_o2 * (1 - out_o2) * w6) * out_h1 * (1 - out_h1) * x1
d_w3 = (d_o1 * out_h1 * (1 - out_h1) * w5 + d_o2 * out_o2 * (1 - out_o2) * w6) * out_h1 * (1 - out_h1) * x2
d_w2 = (d_o1 * out_h1 * (1 - out_h1) * w7 + d_o2 * out_o2 * (1 - out_o2) * w8) * out_h2 * (1 - out_h2) * x1
d_w4 = (d_o1 * out_h1 * (1 - out_h1) * w7 + d_o2 * out_o2 * (1 - out_o2) * w8) * out_h2 * (1 - out_h2) * x2
print("反向传播:误差传给每个权值", round(d_w1, 5), round(d_w2, 5), round(d_w3, 5), round(d_w4, 5), round(d_w5, 5), round(d_w6, 5),
round(d_w7, 5), round(d_w8, 5))
return d_w1, d_w2, d_w3, d_w4, d_w5, d_w6, d_w7, d_w8
def update_w(w1, w2, w3, w4, w5, w6, w7, w8):
# 步长
step = 1
w1 = w1 - step * d_w1
w2 = w2 - step * d_w2
w3 = w3 - step * d_w3
w4 = w4 - step * d_w4
w5 = w5 - step * d_w5
w6 = w6 - step * d_w6
w7 = w7 - step * d_w7
w8 = w8 - step * d_w8
return w1, w2, w3, w4, w5, w6, w7, w8
if __name__ == "__main__":
print("更新前的权值:",round(w1, 2), round(w2, 2), round(w3, 2), round(w4, 2), round(w5, 2), round(w6, 2), round(w7, 2),
round(w8, 2))
for i in range(1):
print("第" + str(i+1) + "轮:")
out_o1, out_o2, out_h1, out_h2 = forward_propagate(x1, x2, y1, y2, w1, w2, w3, w4, w5, w6, w7, w8)
d_w1, d_w2, d_w3, d_w4, d_w5, d_w6, d_w7, d_w8 = back_propagate(out_o1, out_o2, out_h1, out_h2)
w1, w2, w3, w4, w5, w6, w7, w8 = update_w(w1, w2, w3, w4, w5, w6, w7, w8)
print("更新后的权值w:", round(w1, 2), round(w2, 2), round(w3, 2), round(w4, 2), round(w5, 2), round(w6, 2), round(w7, 2),
round(w8, 2))
结果:
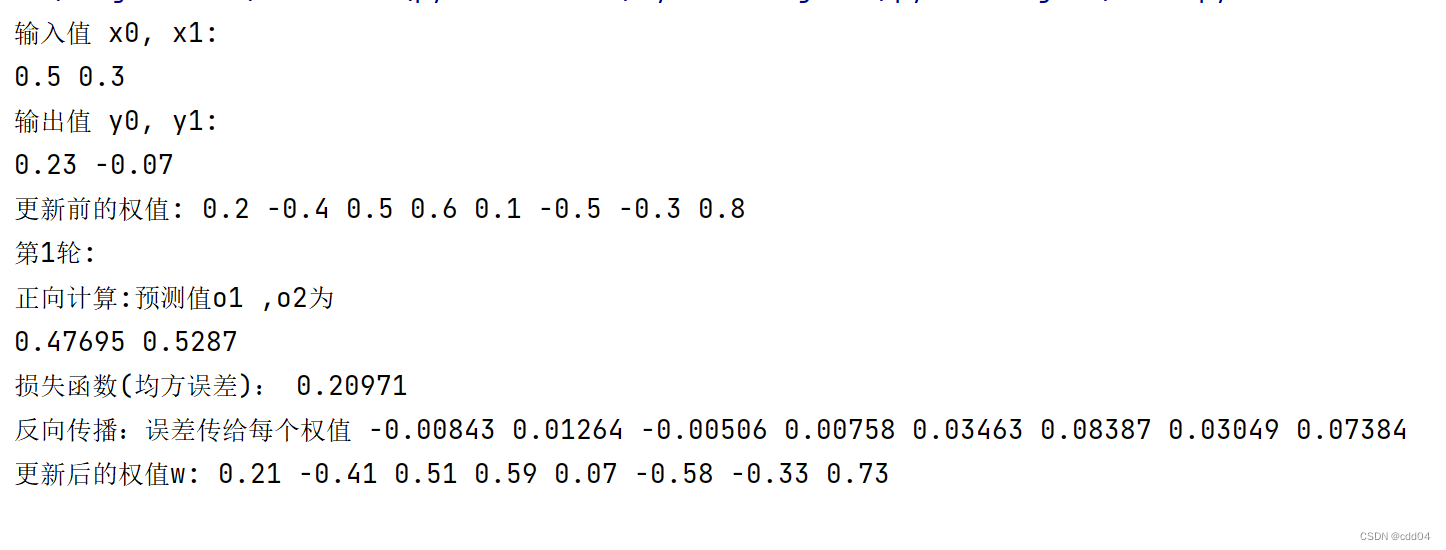
?使用pytorch实现
import torch
x1, x2 = torch.Tensor([0.5]), torch.Tensor([0.3])
y1, y2 = torch.Tensor([0.23]), torch.Tensor([-0.07])
print("=====输入值:x1, x2;真实输出值:y1, y2=====")
print(x1, x2, y1, y2)
w1, w2, w3, w4, w5, w6, w7, w8 = torch.Tensor([0.2]), torch.Tensor([-0.4]), torch.Tensor([0.5]), torch.Tensor(
[0.6]), torch.Tensor([0.1]), torch.Tensor([-0.5]), torch.Tensor([-0.3]), torch.Tensor([0.8]) # 权重初始值
w1.requires_grad = True
w2.requires_grad = True
w3.requires_grad = True
w4.requires_grad = True
w5.requires_grad = True
w6.requires_grad = True
w7.requires_grad = True
w8.requires_grad = True
def sigmoid(z):
a = 1 / (1 + torch.exp(-z))
return a
def forward_propagate(x1, x2):
in_h1 = w1 * x1 + w3 * x2
out_h1 = sigmoid(in_h1) # out_h1 = torch.sigmoid(in_h1)
in_h2 = w2 * x1 + w4 * x2
out_h2 = sigmoid(in_h2) # out_h2 = torch.sigmoid(in_h2)
in_o1 = w5 * out_h1 + w7 * out_h2
out_o1 = sigmoid(in_o1) # out_o1 = torch.sigmoid(in_o1)
in_o2 = w6 * out_h1 + w8 * out_h2
out_o2 = sigmoid(in_o2) # out_o2 = torch.sigmoid(in_o2)
print("正向计算:o1 ,o2")
print(out_o1.data, out_o2.data)
return out_o1, out_o2
def loss_fuction(x1, x2, y1, y2): # 损失函数
y1_pred, y2_pred = forward_propagate(x1, x2) # 前向传播
loss = (1 / 2) * (y1_pred - y1) ** 2 + (1 / 2) * (y2_pred - y2) ** 2 # 考虑 : t.nn.MSELoss()
print("损失函数(均方误差):", loss.item())
return loss
def update_w(w1, w2, w3, w4, w5, w6, w7, w8):
# 步长
step = 1
w1.data = w1.data - step * w1.grad.data
w2.data = w2.data - step * w2.grad.data
w3.data = w3.data - step * w3.grad.data
w4.data = w4.data - step * w4.grad.data
w5.data = w5.data - step * w5.grad.data
w6.data = w6.data - step * w6.grad.data
w7.data = w7.data - step * w7.grad.data
w8.data = w8.data - step * w8.grad.data
w1.grad.data.zero_() # 注意:将w中所有梯度清零
w2.grad.data.zero_()
w3.grad.data.zero_()
w4.grad.data.zero_()
w5.grad.data.zero_()
w6.grad.data.zero_()
w7.grad.data.zero_()
w8.grad.data.zero_()
return w1, w2, w3, w4, w5, w6, w7, w8
if __name__ == "__main__":
print("=====更新前的权值=====")
print(w1.data, w2.data, w3.data, w4.data, w5.data, w6.data, w7.data, w8.data)
for i in range(1):
print("=====第" + str(i+1) + "轮=====")
L = loss_fuction(x1, x2, y1, y2) # 前向传播,求 Loss,构建计算图
L.backward() # 自动求梯度,不需要人工编程实现。反向传播,求出计算图中所有梯度存入w中
print("\tgrad W: ", round(w1.grad.item(), 2), round(w2.grad.item(), 2), round(w3.grad.item(), 2),
round(w4.grad.item(), 2), round(w5.grad.item(), 2), round(w6.grad.item(), 2), round(w7.grad.item(), 2),
round(w8.grad.item(), 2))
w1, w2, w3, w4, w5, w6, w7, w8 = update_w(w1, w2, w3, w4, w5, w6, w7, w8)
print("更新后的权值")
print(w1.data, w2.data, w3.data, w4.data, w5.data, w6.data, w7.data, w8.data)
结果:
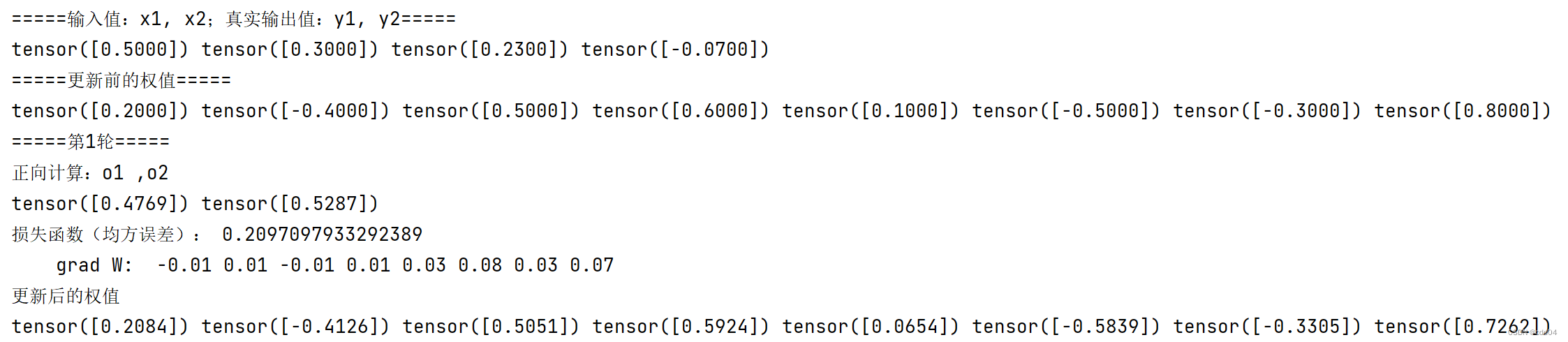
?1.对比【numpy】和【pytorch】程序,总结并陈述。
pytorch在神经网络中可以代替numpy,在程序中改变训练轮数两种实验结果依然接近,但pytorch部分结果精确度更高。
训练轮数为10:
numpy:
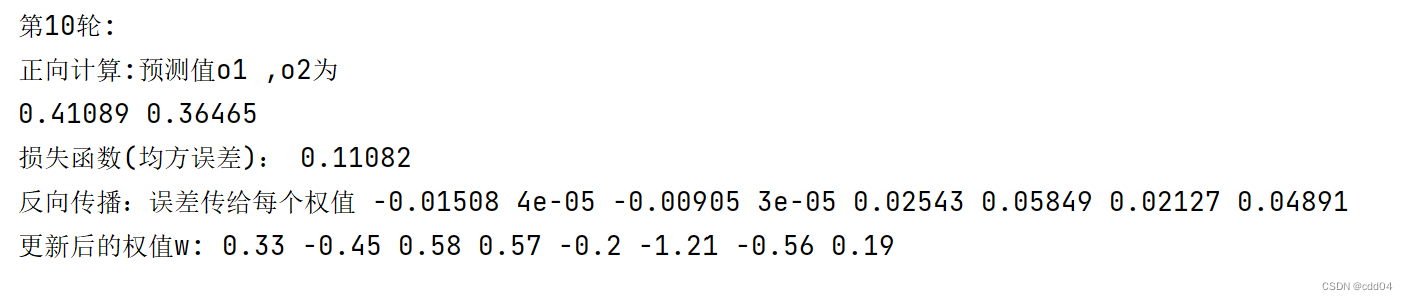
?pytorch:

2.激活函数Sigmoid用PyTorch自带函数torch.sigmoid(),观察、总结并陈述。
使用Sigmoid函数和使用Pytorch自带函数torch.sigmoid()没有什么明显差距。
3.激活函数Sigmoid改变为Relu,观察、总结并陈述。
def relu(z):
return np.maximum(0, z)

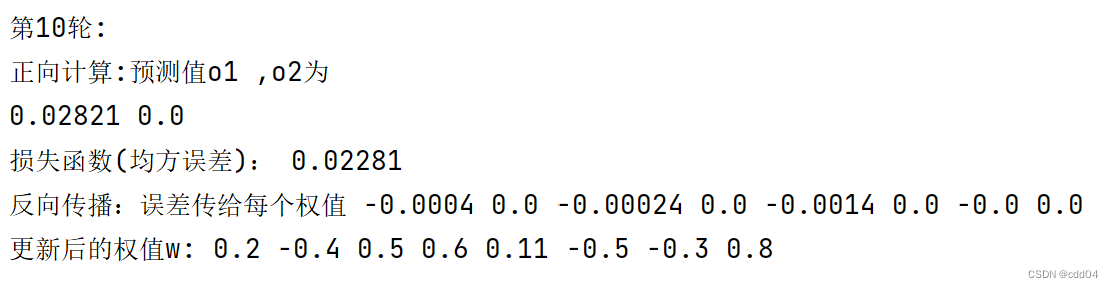
(1)Relu函数均方误差降低速度快,收敛速度更优。
(2)Relu函数可以防止梯度弥散。
(3)? Relu函数会输出零值,导致网络稀疏,即稀疏激活性,减少了参数的相互依存关系,缓解了过拟合问题的发生。
4.损失函数MSE用PyTorch自带函数 t.nn.MSELoss()替代,观察、总结并陈述。
def loss_fuction(x1, x2, y1, y2): ?# 损失函数 ? ? y1_pred, y2_pred = forward_propagate(x1, x2) ?# 前向传播 ? ? loss_func = torch.nn.MSELoss() # 创建损失函数 ? ? y_pred = torch.cat((y1_pred, y2_pred), dim=0) # 将y1_pred, y2_pred合并成一个向量 ? ? y = torch.cat((y1, y2), dim=0) # 将y1, y2合并成一个向量 ? ? loss = loss_func(y_pred, y) # 计算损失 ? ? print("损失函数(均方误差):", loss.item()) ? ? return loss
训练轮数较低时自带函数和原先函数运行结果相同。
5.损失函数MSE改变为交叉熵,观察、总结并陈述。
def loss_fuction(x1, x2, y1, y2): ? ? y1_pred, y2_pred = forward_propagate(x1, x2) ? ? loss_func = torch.nn.CrossEntropyLoss() # 创建交叉熵损失函数 ? ? y_pred = torch.stack([y1_pred, y2_pred], dim=1) ? ? y = torch.stack([y1, y2], dim=1) ? ? loss = loss_func(y_pred, y) # 计算 ? ? print("损失函数(交叉熵损失):", loss.item()) ? ? return loss


?出现负数,可以看出交叉熵损失函数更实用于分类,而不是预测。
6.改变步长,训练次数,观察、总结并陈述。
步长为1:

?
?步长为2:


?步长为1比步长为2训练效果更好。
7.权值w1-w8初始值换为随机数,对比“指定权值”的结果,观察、总结并陈述。
?w1, w2, w3, w4, w5, w6, w7, w8 = torch.randn(1), torch.randn(1), torch.randn(1), torch.randn(1), torch.randn(1), torch.randn(1), torch.randn(1), torch.randn(1)
训练轮数为1:
训练轮数为10:

可以看出权值的改变,而收敛速度几乎无影响 。
8.权值w1-w8初始值换为0,观察、总结并陈述。
w1, w2, w3, w4, w5, w6, w7, w8 = torch.Tensor([0]), torch.Tensor([0]), torch.Tensor([0]), torch.Tensor([0]), torch.Tensor([0]), torch.Tensor([0]), torch.Tensor([0]), torch.Tensor([0])

?
心得体会:
通过本次实验,我加深了对反向传播算法的理解,以及激活函数,损失函数,步长,训练次数,权值不同对实验结果的影响及其在前馈神经网络中的作用。在神经网络中,pytorch框架可以代替numpy。
|