1. partial?
from functools import partial
# A normal function
def f(a, b, c, x):
print("a",a)
print("b",b)
print("c",c)
print("x",x)
return 1000*a + 100*b + 10*c + x
# A partial function that calls f with
g = partial(f, 3, 1, x=4)
# Calling g()
print(g(5))
?a= 3, b=1, c=5, x=4, 3154
2. nn. LayerNorm
torch.nn.LayerNorm(normalized_shape, eps=1e-05,
elementwise_affine=True, device=None, dtype=None)
??????normalized_shape?(int?or?list?or?torch.Size) –input shape from an expected input of size
? ? ? ? ?[?×normalized_shape[0]×normalized_shape[1]×…×normalized_shape[?1]]
-
If a single integer is used, it is treated as a singleton list, and this module will normalize over the last dimension which is expected to be of that specific size. -
eps?– a value added to the denominator for numerical stability. Default: 1e-5 -
elementwise_affine?– a boolean value that when set to?True , this module has learnable per-element affine parameters initialized to ones (for weights) and zeros (for biases). Default:?True .
# NLP Example
batch, sentence_length, embedding_dim = 20, 5, 10
embedding = torch.randn(batch, sentence_length, embedding_dim)
layer_norm = nn.LayerNorm(embedding_dim)
# Activate module
layer_norm(embedding)
# Image Example
N, C, H, W = 20, 5, 10, 10
input = torch.randn(N, C, H, W)
# Normalize over the last three dimensions (i.e. the channel and spatial dimensions)
# as shown in the image below
layer_norm = nn.LayerNorm([C, H, W])
output = layer_norm(input)
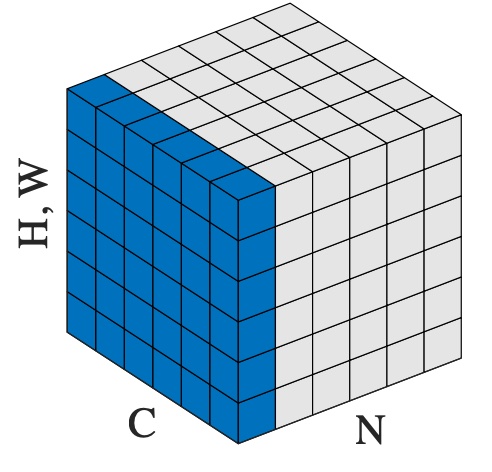
3. a or b
t1=None
t2=2
t1= t1 or t2
print(t1)
Results: 2?
t1=1
t2=2
t1= t1 or t2
print(t1)
Results:1?
4. nn.GELU
torch.nn.GELU(approximate='none')
Applies the Gaussian Error Linear Units functionwhere?\Phi(x)Φ(x)?is the Cumulative Distribution Function for Gaussian Distribution.
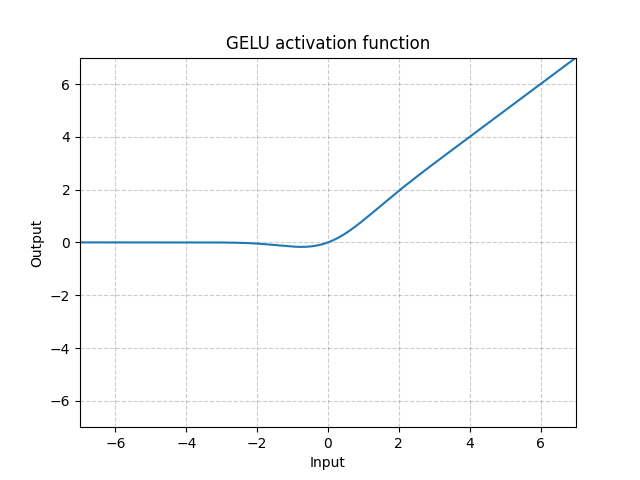
>>> m = nn.GELU()
>>> input = torch.randn(2)
>>> output = m(input)
5. nn.Dropout
torch.nn.Dropout(p=0.5,?inplace=False)
>>> m = nn.Dropout(p=0.2)
>>> input = torch.randn(20, 16)
>>> output = m(input)
6. nn.linspace
# Importing the PyTorch library
import torch
# Applying the linspace function and
# storing the resulting tensor in 't'
a = torch.linspace(3, 10, 5)
print("a = ", a)
b = torch.linspace(start =-10, end = 10, steps = 5)
print("b = ", b)
a = tensor([ 3.0000, 4.7500, 6.5000, 8.2500, 10.0000])
b = tensor([-10., -5., 0., 5., 10.])
7. nn.indetity
seed =4
m = nn.Identity()
input = torch.randn(4,4)
output = m(input)
print("m",m)
print("input",input)
print("output",output)
print(output.size())
8. nn.init.trunc_normal_()
torch.nn.init.trunc_normal_(tensor, mean=0.0, std=1.0, a=- 2.0, b=2.0)
?Fills the input Tensor with values drawn from a truncated normal distribution. The values are effectively drawn from the normal distribution
?\mathcal{N}(\text{mean}, \text{std}^2)N(mean,std2)?
with values outside?[a, b][a,b]?redrawn until they are within the bounds. The method used for generating the random values works best when?a
\leq \text{mean} \leq ba≤mean≤b.
9. OrderedDictT
from collections import OrderedDict
print("This is a Dict:\n")
d = {}
d['a'] = 1
d['b'] = 2
d['c'] = 3
d['d'] = 4
for key, value in d.items():
print(key, value)
print("\nThis is an Ordered Dict:\n")
od = OrderedDict()
od['a'] = 1
od['b'] = 2
od['c'] = 3
od['d'] = 4
for key, value in od.items():
print(key, value)
print("\nThis is an *****Ordered Dict:\n")
od = OrderedDict([("a1",1),("b1",2),("c1",3),("d1",4)])
for key, value in od.items():
print(key, value)
?The above three oupts are the same
10. random.seed()
How Seed Function Works ?
Seed function is used to save the state of a random function, so that it can generate same random numbers on multiple executions of the code on the same machine or on different machines (for a specific seed value). The seed value is the previous value number generated by the generator. For the first time when there is no previous value, it uses current system time.?
Using random.seed() function
Here we will see how we can generate the same random number every time with the same seed value.?
# random module is imported
import random
for i in range(5):
# Any number can be used in place of '0'.
random.seed(0)
# Generated random number will be between 1 to 1000.
print(random.randint(1, 1000))
Output:
865
865
865
865
865
# importing random module
import random
random.seed(3)
# print a random number between 1 and 1000.
print(random.randint(1, 1000))
# if you want to get the same random number again then,
random.seed(3)
print(random.randint(1, 1000))
# If seed function is not used
# Gives totally unpredictable responses.
print(random.randint(1, 1000))
Output:
244
244
607
?random.seed(0)? the next random.randint(1) get same int.?
random.seed(1)? ?the next random.randint(1) get different int.
11. Tensor, List? ?Convert each other
a. Tensors concate to a Tensor
import torch
#step: generate a tensor with shape([5,3,3,4])
# we can think B is 5, N_views is 3, Token is 3, Dim is 4
a=torch.arange(5*3*3*4).reshape([5,3,3,4])
print(a.shape)
print("a=",a)
torch.Size([5, 3, 3, 4])
a= tensor([[[[ 0, 1, 2, 3],
[ 4, 5, 6, 7],
[ 8, 9, 10, 11]],
[[ 12, 13, 14, 15],
[ 16, 17, 18, 19],
[ 20, 21, 22, 23]],
[[ 24, 25, 26, 27],
[ 28, 29, 30, 31],
[ 32, 33, 34, 35]]],
[[[ 36, 37, 38, 39],
[ 40, 41, 42, 43],
[ 44, 45, 46, 47]],
[[ 48, 49, 50, 51],
[ 52, 53, 54, 55],
[ 56, 57, 58, 59]],
[[ 60, 61, 62, 63],
[ 64, 65, 66, 67],
[ 68, 69, 70, 71]]],
[[[ 72, 73, 74, 75],
[ 76, 77, 78, 79],
[ 80, 81, 82, 83]],
[[ 84, 85, 86, 87],
[ 88, 89, 90, 91],
[ 92, 93, 94, 95]],
[[ 96, 97, 98, 99],
[100, 101, 102, 103],
[104, 105, 106, 107]]],
[[[108, 109, 110, 111],
[112, 113, 114, 115],
[116, 117, 118, 119]],
[[120, 121, 122, 123],
[124, 125, 126, 127],
[128, 129, 130, 131]],
[[132, 133, 134, 135],
[136, 137, 138, 139],
[140, 141, 142, 143]]],
[[[144, 145, 146, 147],
[148, 149, 150, 151],
[152, 153, 154, 155]],
[[156, 157, 158, 159],
[160, 161, 162, 163],
[164, 165, 166, 167]],
[[168, 169, 170, 171],
[172, 173, 174, 175],
[176, 177, 178, 179]]]])
a_local2=[]
a=a.reshape(3,5,3,4)
print("a.reshape",a)
a.reshape tensor([[[[ 0, 1, 2, 3],
[ 4, 5, 6, 7],
[ 8, 9, 10, 11]],
[[ 12, 13, 14, 15],
[ 16, 17, 18, 19],
[ 20, 21, 22, 23]],
[[ 24, 25, 26, 27],
[ 28, 29, 30, 31],
[ 32, 33, 34, 35]],
[[ 36, 37, 38, 39],
[ 40, 41, 42, 43],
[ 44, 45, 46, 47]],
[[ 48, 49, 50, 51],
[ 52, 53, 54, 55],
[ 56, 57, 58, 59]]],
[[[ 60, 61, 62, 63],
[ 64, 65, 66, 67],
[ 68, 69, 70, 71]],
[[ 72, 73, 74, 75],
[ 76, 77, 78, 79],
[ 80, 81, 82, 83]],
[[ 84, 85, 86, 87],
[ 88, 89, 90, 91],
[ 92, 93, 94, 95]],
[[ 96, 97, 98, 99],
[100, 101, 102, 103],
[104, 105, 106, 107]],
[[108, 109, 110, 111],
[112, 113, 114, 115],
[116, 117, 118, 119]]],
[[[120, 121, 122, 123],
[124, 125, 126, 127],
[128, 129, 130, 131]],
[[132, 133, 134, 135],
[136, 137, 138, 139],
[140, 141, 142, 143]],
[[144, 145, 146, 147],
[148, 149, 150, 151],
[152, 153, 154, 155]],
[[156, 157, 158, 159],
[160, 161, 162, 163],
[164, 165, 166, 167]],
[[168, 169, 170, 171],
[172, 173, 174, 175],
[176, 177, 178, 179]]]])
for j in range(a.shape[0]):
a_local2.append(a[j,:,:,:])
print(torch.cat(a_local2))
torch.cat(a_local2).shape torch.Size([15, 3, 4])
tensor([[[ 0, 1, 2, 3],
[ 4, 5, 6, 7],
[ 8, 9, 10, 11]],
[[ 12, 13, 14, 15],
[ 16, 17, 18, 19],
[ 20, 21, 22, 23]],
[[ 24, 25, 26, 27],
[ 28, 29, 30, 31],
[ 32, 33, 34, 35]],
[[ 36, 37, 38, 39],
[ 40, 41, 42, 43],
[ 44, 45, 46, 47]],
[[ 48, 49, 50, 51],
[ 52, 53, 54, 55],
[ 56, 57, 58, 59]],
[[ 60, 61, 62, 63],
[ 64, 65, 66, 67],
[ 68, 69, 70, 71]],
[[ 72, 73, 74, 75],
[ 76, 77, 78, 79],
[ 80, 81, 82, 83]],
[[ 84, 85, 86, 87],
[ 88, 89, 90, 91],
[ 92, 93, 94, 95]],
[[ 96, 97, 98, 99],
[100, 101, 102, 103],
[104, 105, 106, 107]],
[[108, 109, 110, 111],
[112, 113, 114, 115],
[116, 117, 118, 119]],
[[120, 121, 122, 123],
[124, 125, 126, 127],
[128, 129, 130, 131]],
[[132, 133, 134, 135],
[136, 137, 138, 139],
[140, 141, 142, 143]],
[[144, 145, 146, 147],
[148, 149, 150, 151],
[152, 153, 154, 155]],
[[156, 157, 158, 159],
[160, 161, 162, 163],
[164, 165, 166, 167]],
[[168, 169, 170, 171],
[172, 173, 174, 175],
[176, 177, 178, 179]]])
c=torch.cat(a_local2).reshape(5,3,3,4)
print("c=",c)
c= tensor([[[[ 0, 1, 2, 3],
[ 4, 5, 6, 7],
[ 8, 9, 10, 11]],
[[ 12, 13, 14, 15],
[ 16, 17, 18, 19],
[ 20, 21, 22, 23]],
[[ 24, 25, 26, 27],
[ 28, 29, 30, 31],
[ 32, 33, 34, 35]]],
[[[ 36, 37, 38, 39],
[ 40, 41, 42, 43],
[ 44, 45, 46, 47]],
[[ 48, 49, 50, 51],
[ 52, 53, 54, 55],
[ 56, 57, 58, 59]],
[[ 60, 61, 62, 63],
[ 64, 65, 66, 67],
[ 68, 69, 70, 71]]],
[[[ 72, 73, 74, 75],
[ 76, 77, 78, 79],
[ 80, 81, 82, 83]],
[[ 84, 85, 86, 87],
[ 88, 89, 90, 91],
[ 92, 93, 94, 95]],
[[ 96, 97, 98, 99],
[100, 101, 102, 103],
[104, 105, 106, 107]]],
[[[108, 109, 110, 111],
[112, 113, 114, 115],
[116, 117, 118, 119]],
[[120, 121, 122, 123],
[124, 125, 126, 127],
[128, 129, 130, 131]],
[[132, 133, 134, 135],
[136, 137, 138, 139],
[140, 141, 142, 143]]],
[[[144, 145, 146, 147],
[148, 149, 150, 151],
[152, 153, 154, 155]],
[[156, 157, 158, 159],
[160, 161, 162, 163],
[164, 165, 166, 167]],
[[168, 169, 170, 171],
[172, 173, 174, 175],
[176, 177, 178, 179]]]])
a_local2=torch.cat(a_local2).reshape(5,9,4)
print("torch_a.shape",a_local2.shape)
print("torch_a",a_local2)
torch_a.shape torch.Size([5, 9, 4])
torch_a tensor([[[ 0, 1, 2, 3],
[ 4, 5, 6, 7],
[ 8, 9, 10, 11],
[ 12, 13, 14, 15],
[ 16, 17, 18, 19],
[ 20, 21, 22, 23],
[ 24, 25, 26, 27],
[ 28, 29, 30, 31],
[ 32, 33, 34, 35]],
[[ 36, 37, 38, 39],
[ 40, 41, 42, 43],
[ 44, 45, 46, 47],
[ 48, 49, 50, 51],
[ 52, 53, 54, 55],
[ 56, 57, 58, 59],
[ 60, 61, 62, 63],
[ 64, 65, 66, 67],
[ 68, 69, 70, 71]],
[[ 72, 73, 74, 75],
[ 76, 77, 78, 79],
[ 80, 81, 82, 83],
[ 84, 85, 86, 87],
[ 88, 89, 90, 91],
[ 92, 93, 94, 95],
[ 96, 97, 98, 99],
[100, 101, 102, 103],
[104, 105, 106, 107]],
[[108, 109, 110, 111],
[112, 113, 114, 115],
[116, 117, 118, 119],
[120, 121, 122, 123],
[124, 125, 126, 127],
[128, 129, 130, 131],
[132, 133, 134, 135],
[136, 137, 138, 139],
[140, 141, 142, 143]],
[[144, 145, 146, 147],
[148, 149, 150, 151],
[152, 153, 154, 155],
[156, 157, 158, 159],
[160, 161, 162, 163],
[164, 165, 166, 167],
[168, 169, 170, 171],
[172, 173, 174, 175],
[176, 177, 178, 179]]])
15. Choose a Dimension in Multi-Dimension Tensors?
a=torch.arange(3*2*10).reshape([3,2,10])
print("a.shape",a.shape)
print("a=",a)
print("a[:,0]",a[:,0])
print("a[0,:]",a[0,:])
Results:
a.shape torch.Size([3, 2, 10])
a= tensor([[[ 0, 1, 2, 3, 4, 5, 6, 7, 8, 9],
[10, 11, 12, 13, 14, 15, 16, 17, 18, 19]],
[[20, 21, 22, 23, 24, 25, 26, 27, 28, 29],
[30, 31, 32, 33, 34, 35, 36, 37, 38, 39]],
[[40, 41, 42, 43, 44, 45, 46, 47, 48, 49],
[50, 51, 52, 53, 54, 55, 56, 57, 58, 59]]])
a[:,0] tensor([[ 0, 1, 2, 3, 4, 5, 6, 7, 8, 9],
[20, 21, 22, 23, 24, 25, 26, 27, 28, 29],
[40, 41, 42, 43, 44, 45, 46, 47, 48, 49]])
a[0,:] tensor([[ 0, 1, 2, 3, 4, 5, 6, 7, 8, 9],
[10, 11, 12, 13, 14, 15, 16, 17, 18, 19]])
?
|