1、数据集
经过前两部分的处理,我们可以得到参与建模的变量,统计类特征的处理包括在mainProcess 中,bert构建的特征包括在get_bert_features 中,数据预处理如下:
import warnings
warnings.simplefilter('ignore')
import re
import gc
import pickle
import numpy as np
import pandas as pd
pd.set_option('max_columns', None)
pd.set_option('max_rows', 100)
from tqdm.notebook import tqdm
%matplotlib inline
import matplotlib.pyplot as plt
import seaborn as sns
import sklearn
from sklearn.model_selection import KFold, StratifiedKFold
from sklearn.preprocessing import LabelEncoder
from sklearn.feature_extraction.text import TfidfVectorizer
from sklearn.decomposition import TruncatedSVD
from sklearn.metrics import f1_score
from lightgbm import LGBMClassifier
from sklearn.decomposition import PCA
import jieba
import jieba.posseg as pseg
import lightgbm as lgb
import codecs
from textrank4zh import TextRank4Keyword, TextRank4Sentence, util
from hyperopt import fmin, tpe, hp
from hyperopt import Trials
train_path = "./data/train.csv"
test_path = "./data/test.csv"
print("数据预处理...")
train, test = mainProcess(train_path, test_path)
train, test = get_bert_features(train, test)
最终得到的数据集如下:
train.shape
test.shape
2、自动调参
通常有两种常用的调参方法:网格搜索和随机搜索。每一种都有自己的优点和缺点。网格搜索速度慢,但在搜索整个搜索空间方面效果很好,而随机搜索很快,但可能会错过搜索空间中的重要点。幸运的是,还有第三种选择:贝叶斯优化。该比赛的调参环节我们使用了贝叶斯优化的一个实现,一个名为hyperopt 的 Python 模块。
hyperopt 的自动调参代码如下:
def hp_param(train_df):
"""基于贝叶斯的自动调参"""
data = train_df
X = data.drop(['id', 'label'], axis=1)
y = data['label']
X_train, X_test, y_train, y_test = sklearn.model_selection.train_test_split(X, y, test_size=0.2, random_state=1)
train_data = lgb.Dataset(data=X_train,label=y_train)
test_data = lgb.Dataset(data=X_test,label=y_test)
space = {"max_depth": hp.randint("max_depth", 15),
"num_trees": hp.randint("num_trees", 300),
"learning_rate": hp.uniform("learning_rate", 1e-3, 5e-1),
"bagging_fraction": hp.randint("bagging_fraction", 5),
"num_leaves": hp.randint("num_leaves", 6),
}
def argsDict_tranform(argsDict, isPrint=False):
argsDict["max_depth"] = argsDict["max_depth"] + 5
argsDict['num_trees'] = argsDict['num_trees'] + 150
argsDict["learning_rate"] = argsDict["learning_rate"] * 0.02 + 0.05
argsDict["bagging_fraction"] = argsDict["bagging_fraction"] * 0.1 + 0.5
argsDict["num_leaves"] = argsDict["num_leaves"] * 3 + 10
if isPrint:
print(argsDict)
else:
pass
return argsDict
def lgb_f1_score(y_hat, data):
y_true = data.get_label()
y_hat = np.round(y_hat)
return 'f1', f1_score(y_true, y_hat), True
def lightgbm_factory(argsDict):
argsDict = argsDict_tranform(argsDict)
params={'nthread': -1,
'max_depth': argsDict['max_depth'],
'num_trees': argsDict['num_trees'],
'eta': argsDict['learning_rate'],
'bagging_fraction': argsDict['bagging_fraction'],
'num_leaves': argsDict['num_leaves'],
'objective': 'binary',
'feature_fraction': 0.8,
'lambda_11': 2,
'lambda_12': 3,
'baggingseed': 100,
}
params['metric'] = ['auc']
model_lgb = lgb.train(params, train_data, valid_sets=[test_data], feval=lgb_f1_score, early_stopping_rounds=10)
return get_tranformer_score(model_lgb)
def get_tranformer_score(tranformer):
model = tranformer
prediction = model.predict(X_test, num_iteration=model.best_iteration)
return -sklearn.metrics.roc_auc_score(y_test, prediction)
best = fmin(lightgbm_factory, space, algo=tpe.suggest, max_evals=6)
print('best:')
print(best)
print('best param')
params = argsDict_tranform(best, isPrint=True)
return params
def model_lgb_hp(train_df, params):
data = train_df
X = data.drop(['id', 'label'], axis=1)
y = data['label']
X_train, X_test, y_train, y_test = sklearn.model_selection.train_test_split(X, y, test_size=0.2, random_state=1)
train_data = lgb.Dataset(data=X_train,label=y_train)
test_data = lgb.Dataset(data=X_test,label=y_test)
gbm_model = LGBMClassifier(boosting_type='gbdt', **params)
gbm_model.fit(X_train, y_train, eval_set=[(X_train, y_train), (X_test, y_test)], eval_metric='AUC', early_stopping_rounds=10)
pickle.dump(gbm_model, open("model", "wb"))
print("lgb已保存成文件model")
调用如上实现可以获得最优参数best_params:
best_params = hp_param(train)
model_lgb_hp(train, best_params)
3、训练LGB模型
采用五折交叉验证训练模型,最终的预测结果是五个模型预测结果的平均值。
def train_model(train, test):
ycol = 'label'
feature_names = list(filter(lambda x: x not in [ycol, 'id'], train.columns))
model = lgb.LGBMClassifier(objective='binary',
boosting_type='gbdt',
learning_rate=0.05,
n_estimators=1000,
max_depth=15,
num_leaves=13,
subsample=0.6,
feature_fraction=0.8,
reg_alpha=2,
reg_lambda=3,
random_state=2021,
is_unbalance=True,
metric='auc')
oof = []
prediction = test[['id']]
prediction[ycol] = 0
df_importance_list = []
kfold = StratifiedKFold(n_splits=5, shuffle=True, random_state=2021)
for fold_id, (trn_idx, val_idx) in enumerate(kfold.split(train[feature_names], train[ycol])):
X_train = train.iloc[trn_idx][feature_names]
Y_train = train.iloc[trn_idx][ycol]
X_val = train.iloc[val_idx][feature_names]
Y_val = train.iloc[val_idx][ycol]
print('\nFold_{} Training ================================\n'.format(fold_id+1))
lgb_model = model.fit(X_train,
Y_train,
eval_names=['train', 'valid'],
eval_set=[(X_train, Y_train), (X_val, Y_val)],
verbose=100,
eval_metric='auc',
early_stopping_rounds=50)
pred_val = lgb_model.predict_proba(X_val, num_iteration=lgb_model.best_iteration_)
df_oof = train.iloc[val_idx][['id', ycol]].copy()
df_oof['pred'] = pred_val[:,1]
oof.append(df_oof)
pred_test = lgb_model.predict_proba(test[feature_names], num_iteration=lgb_model.best_iteration_)
prediction[ycol] += pred_test[:,1] / kfold.n_splits
df_importance = pd.DataFrame({
'column': feature_names,
'importance': lgb_model.feature_importances_,
})
df_importance_list.append(df_importance)
del lgb_model, pred_val, pred_test, X_train, Y_train, X_val, Y_val
gc.collect()
df_oof = pd.concat(oof)
df_importance = pd.concat(df_importance_list)
df_importance = df_importance.groupby(['column'])['importance'].agg(
'mean').sort_values(ascending=False).reset_index()
print(df_importance)
return df_oof, prediction
模型的输出结果:
df_oof, prediction, df_importance = train_model(train, test)
'''
Fold_1 Training ================================
Training until validation scores don't improve for 50 rounds
[100] train's auc: 0.998856 valid's auc: 0.992418
Early stopping, best iteration is:
[135] train's auc: 0.999204 valid's auc: 0.992803
Fold_2 Training ================================
Training until validation scores don't improve for 50 rounds
[100] train's auc: 0.99881 valid's auc: 0.994931
Early stopping, best iteration is:
[97] train's auc: 0.998795 valid's auc: 0.995108
Fold_3 Training ================================
Training until validation scores don't improve for 50 rounds
[100] train's auc: 0.998976 valid's auc: 0.985797
Early stopping, best iteration is:
[51] train's auc: 0.998004 valid's auc: 0.986633
Fold_4 Training ================================
Training until validation scores don't improve for 50 rounds
[100] train's auc: 0.999213 valid's auc: 0.988753
Early stopping, best iteration is:
[82] train's auc: 0.999027 valid's auc: 0.989578
Fold_5 Training ================================
Training until validation scores don't improve for 50 rounds
[100] train's auc: 0.998795 valid's auc: 0.994695
Early stopping, best iteration is:
[76] train's auc: 0.998359 valid's auc: 0.995042
'''
特征重要性:
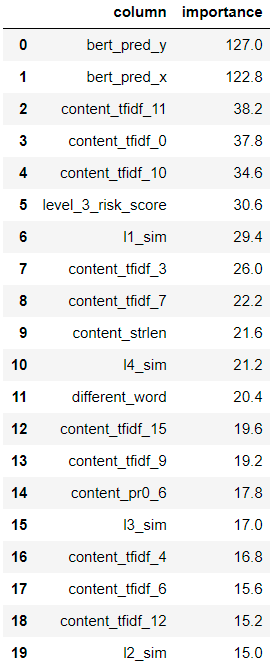
4、搜索最优划分阈值
由于数据的正负样本并不平衡,直接使用0.5作为正样本的划分条件不够合理,这里结合五折交叉验证在训练集上的预测结果,搜索使得f1 score达到最大的划分阈值,搜索过程如下,测试集使用最优划分阈值来得到预测标签:
def search_best_split(df_oof, prediction):
i_bst = 0
bst = 0
for i in np.arange(0.1, 1, 0.05):
df_oof['pred_label'] = df_oof['pred'].apply(lambda x: 1 if x >= i else 0)
score = f1_score(df_oof['label'], df_oof['pred_label'])
print(i, 'f1_score:', score)
if score> bst:
i_bst = i
bst = score
print('best split point: {}, best f1-score: {}'.format(i_bst, bst))
prediction['label'] = prediction['label'].apply(lambda x: 1 if x >= i_bst else 0)
print(prediction['label'].value_counts())
return prediction[['id', 'label']]
输出结果如下:
result = search_best_split(df_oof, prediction)
'''
0.1 f1_score: 0.7603853100541843
0.15000000000000002 f1_score: 0.8142394822006473
0.20000000000000004 f1_score: 0.8392498325519089
0.25000000000000006 f1_score: 0.8588114050154587
0.30000000000000004 f1_score: 0.8713286713286713
0.3500000000000001 f1_score: 0.8830616583982991
0.40000000000000013 f1_score: 0.8921533500537442
0.45000000000000007 f1_score: 0.8991291727140784
0.5000000000000001 f1_score: 0.907957462412908
0.5500000000000002 f1_score: 0.9113082039911308
0.6000000000000002 f1_score: 0.9134328358208955
0.6500000000000001 f1_score: 0.9170731707317074
0.7000000000000002 f1_score: 0.919000757002271
0.7500000000000002 f1_score: 0.9223115193264447
0.8000000000000002 f1_score: 0.9242718446601942
0.8500000000000002 f1_score: 0.9224714679260133
0.9000000000000002 f1_score: 0.9174385823600484
0.9500000000000003 f1_score: 0.797088262056415
best split point: 0.8000000000000002, best f1-score: 0.9242718446601942
0 16082
1 1918
'''
最终的预测结果如下:
result.head()
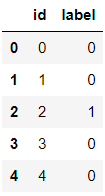 至此,完成比赛。
此外,比赛过程中还有一些发现可以记录下来:
- 适当提高训练bert的epoch可以提高预测结果,但epoch较多时反而变得不好,考虑可能的原因是:适当的epoch可以使得模型得到充分的训练,但是过多epoch会使模型在训练集上拟合的太好,导致泛化性能下降,应该还是bias and variance的问题。
- 通过模型集成和调参确实可以一定程度上提升预测效果。
- 比赛中构造特征是基于Wide&Deep的思路,统计类特征可视为Wide部分,bert输出的特征可视为Deep部分,Wide部分的主要作用是让模型具有较强的“记忆能力”,Deep部分的主要作用是让模型具有“泛化能力”。不过Wide部分和Deep部分没有一起训练,而是先将Deep部分训练好,最后通过LGB融合后作分类。
- bert处理文本分类任务,效果确实非常好(sentence pair bert的结果直接用于预测在线上可以达到0.9528,LGB模型在线上的最好成绩是0.9588),不过对计算资源要求也很高,单机基本跑不动,白嫖了google的GPU才把结果跑出来。
如有更好的处理方式,请不吝指教,非常感谢。
|