1.YOLTv4构建于YOLT和SIMRDWN之上,并更新这些框架以使用YOLO的最高效版本YOLOv4。YOLTv4被设计用于在任意大的图像中检测航空或卫星图像中的目标,这些图像远远超过深度学习目标检测框架通常接收到的~600×600像素大小。
这个存储库是建立在AlexeyAB的YOLOv4实现令人印象深刻的工作之上的,与YOLOv3(在SIMRDWN中实现)相比,YOLOv4提高了速度和检测性能。我们使用YOLOv4
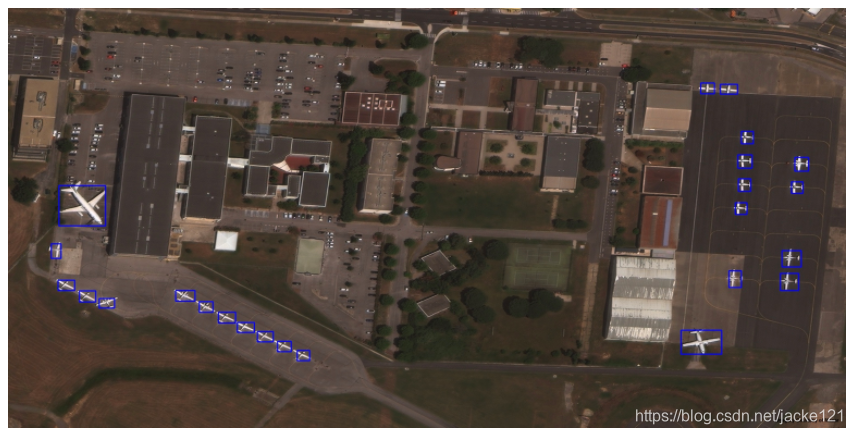
YOLTv4 builds upon?YOLT?and?SIMRDWN, and updates these frameworks to use the most performant version of?YOLO,?YOLOv4. YOLTv4 is designed to detect objects in aerial or satellite imagery in arbitrarily large images that far exceed the ~600×600 pixel size typically ingested by deep learning object detection frameworks.
This repository is built upon the impressive work of AlexeyAB's?YOLOv4?implementation, which improves both speed and detection performance compared to YOLOv3 (which is implemented in SIMRDWN). We use YOLOv4 insead of "YOLOv5", since YOLOv4 is endorsed by the original creators of YOLO, whereas "YOLOv5" is not; furthermore YOLOv4 appears to have superior performance.
Below, we provide examples of how to use this repository with the open-source?Rareplanes?dataset.
https://github.com/avanetten/yoltv4
2018年YOLT算法You only look twice,改变一下思维,对大分辨率图片先进行分割,变成一张张小图,再进行检测。
为了避免两张小图之间,一些目标正好被分割截断,所以两个小图之间设置overlap重叠区域,比如分割的小图是960*960像素大小,则overlap可以设置为960*20%=192像素。
每个小图检测完成后,再将所有的框放到大图上,对大图整体做一次nms操作,将重叠区域的很多重复框去除。(按照在大图上裁剪的位置,直接回归到大图即可。) 坏处就是增加了计算量,我们选用更轻的网络来balance一下吧~
2.
https://blog.csdn.net/moxibingdao/article/details/105852664
|