开发平台:AI Studio 深度学习框架:paddle
开发流程
第一步:新建项目
打开AI studio,选择创建项目 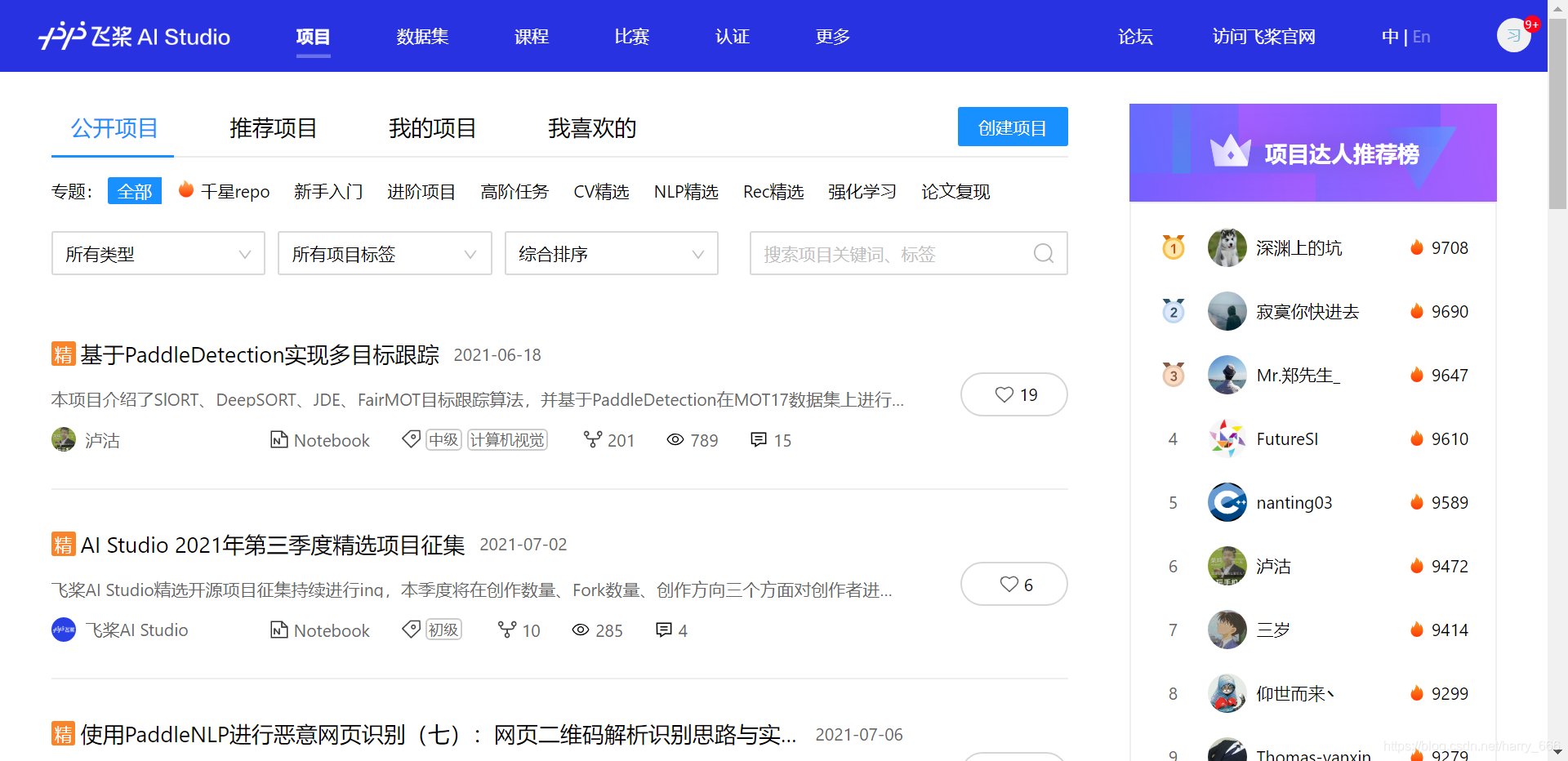 选择notebook,点下一步 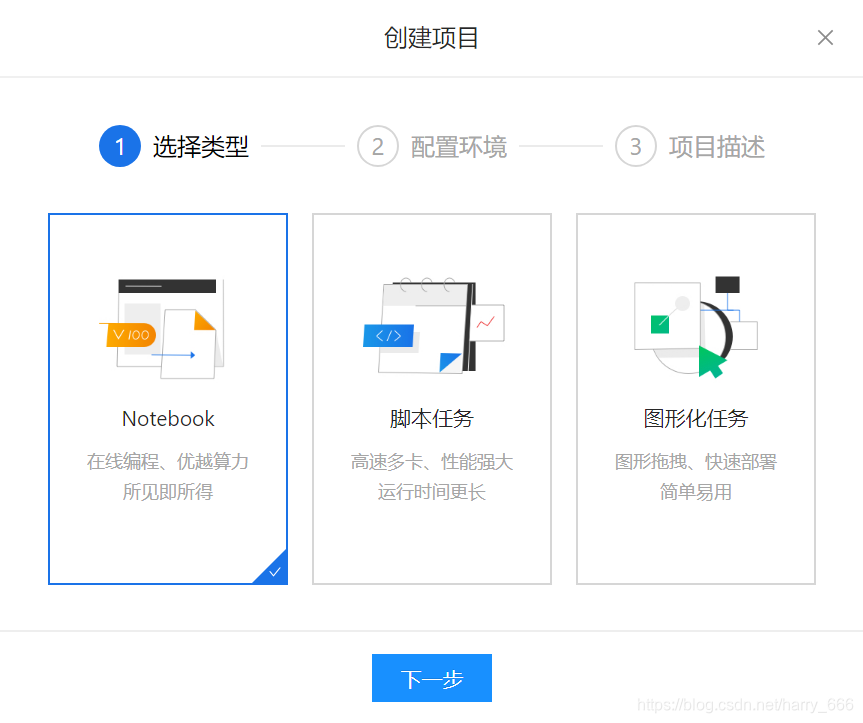 配置环境 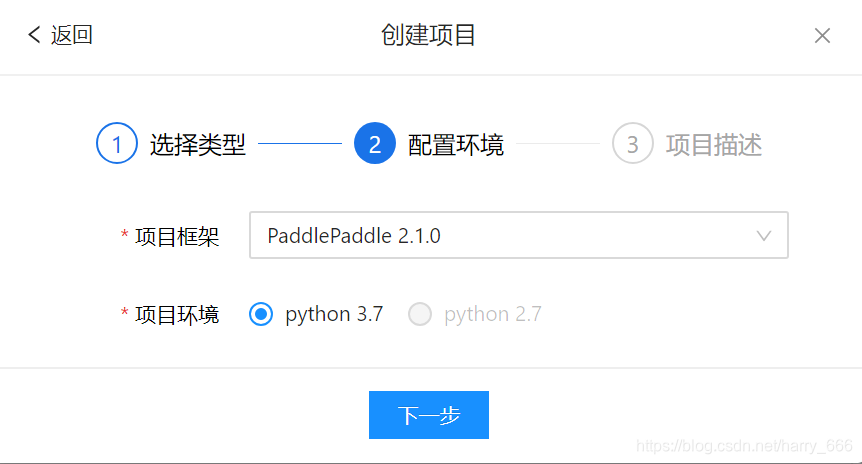 项目描述,大概一写 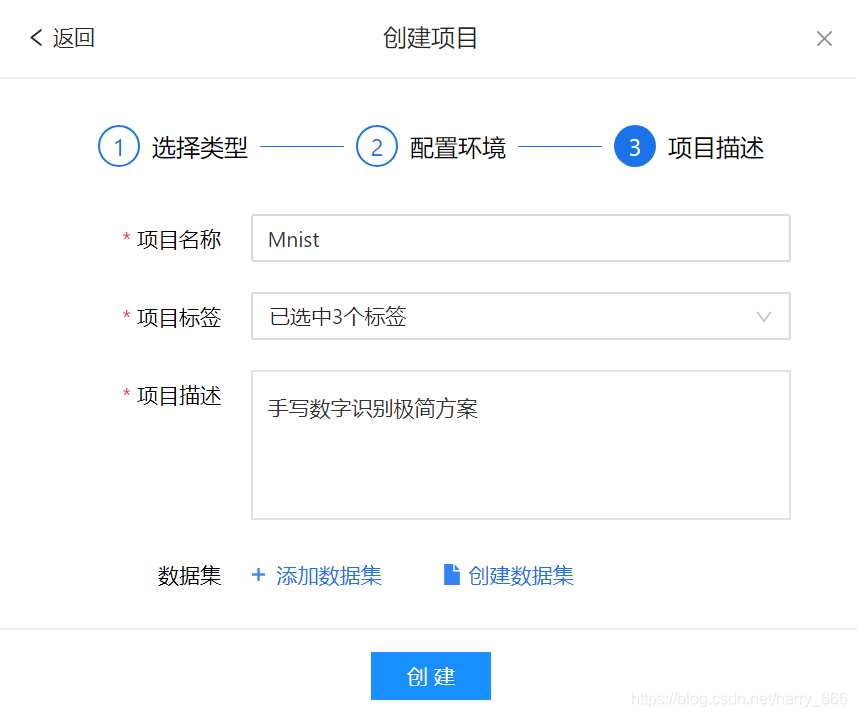 选择数据集,选择公开数据集中的经典Mnist数据集 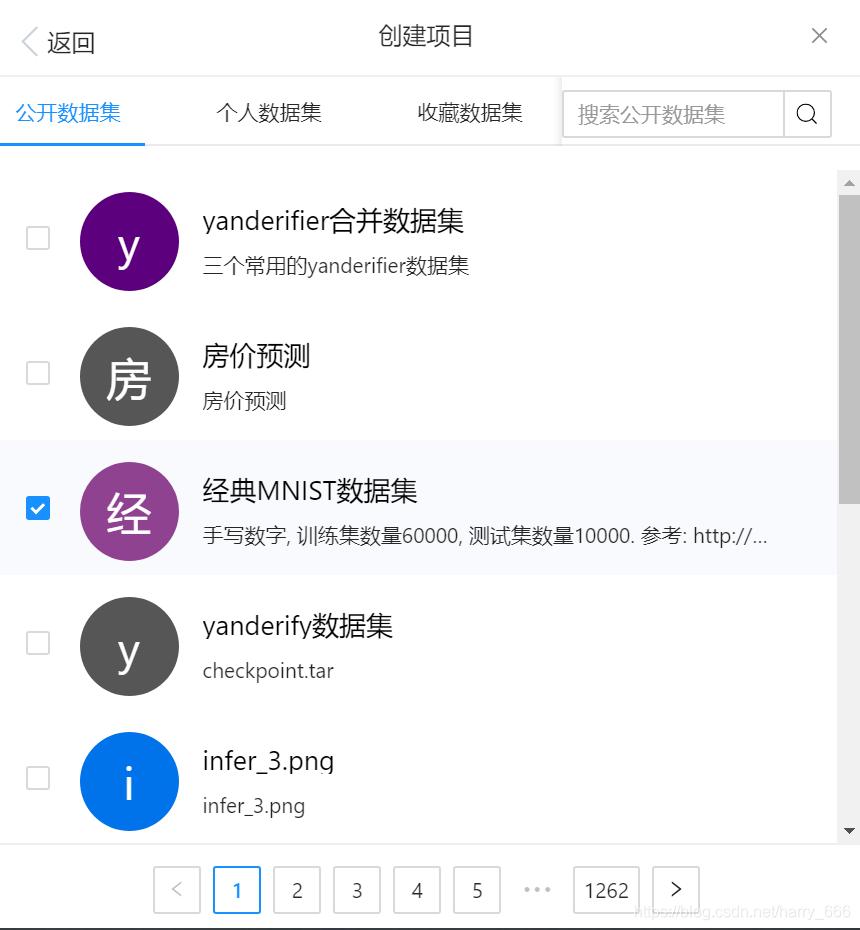 添加后点击创建 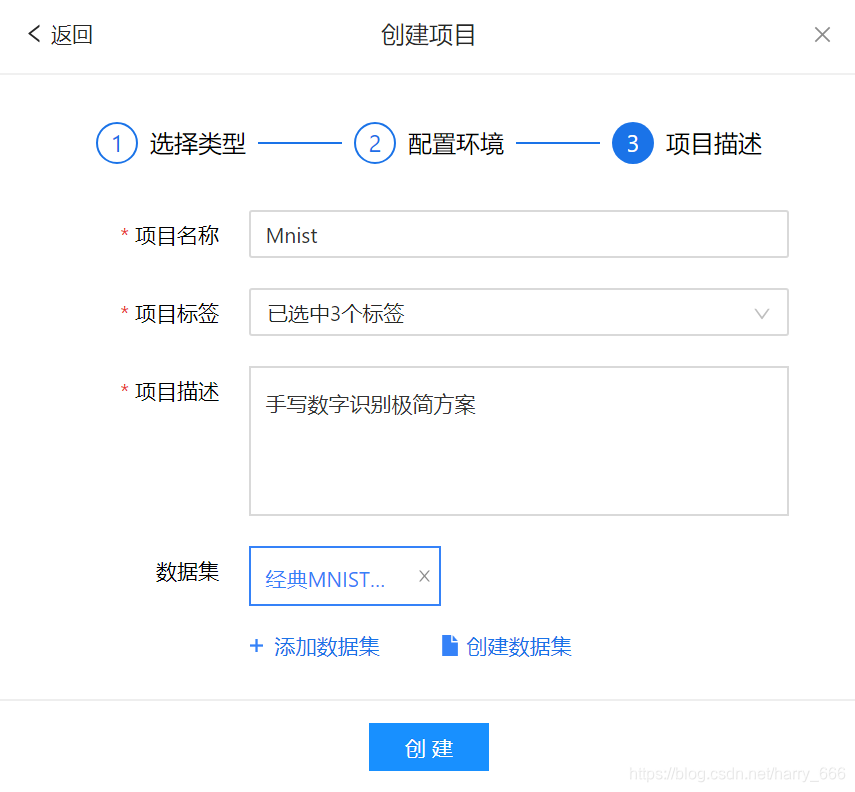 创建之后,点击启动环境 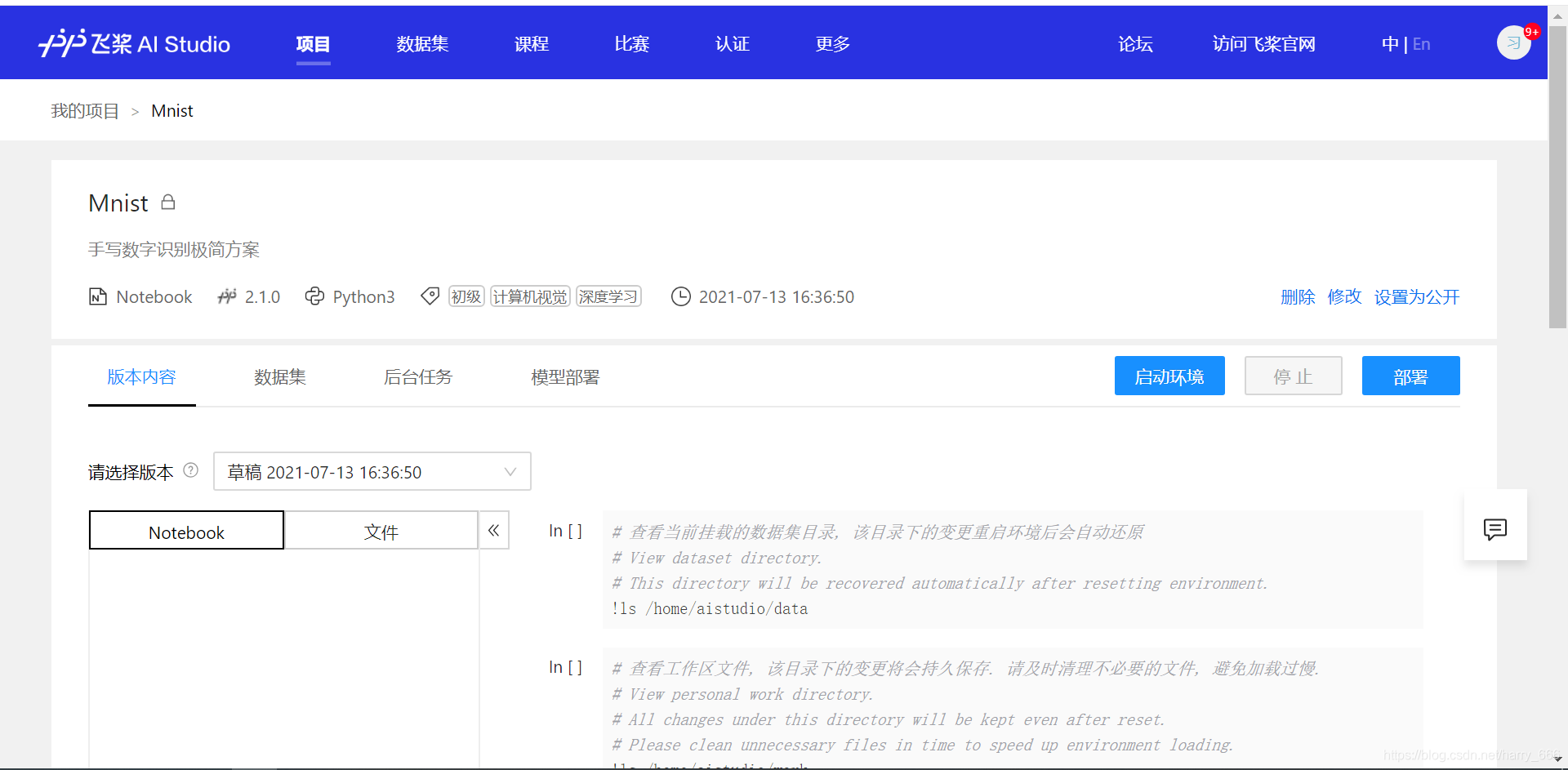 选择基础版 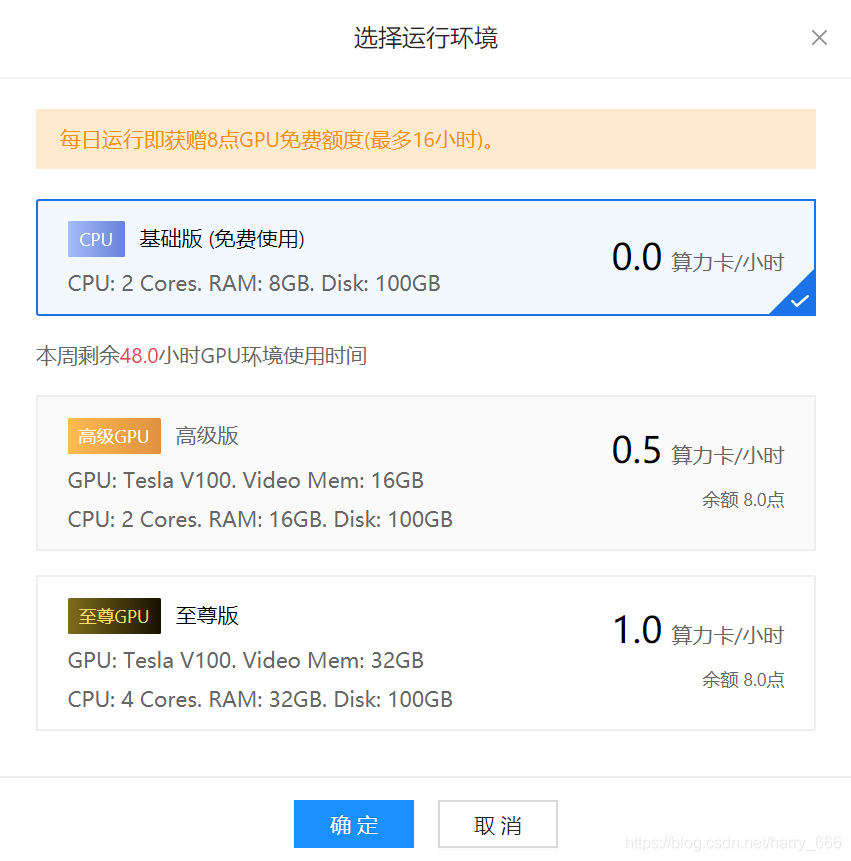 启动之后 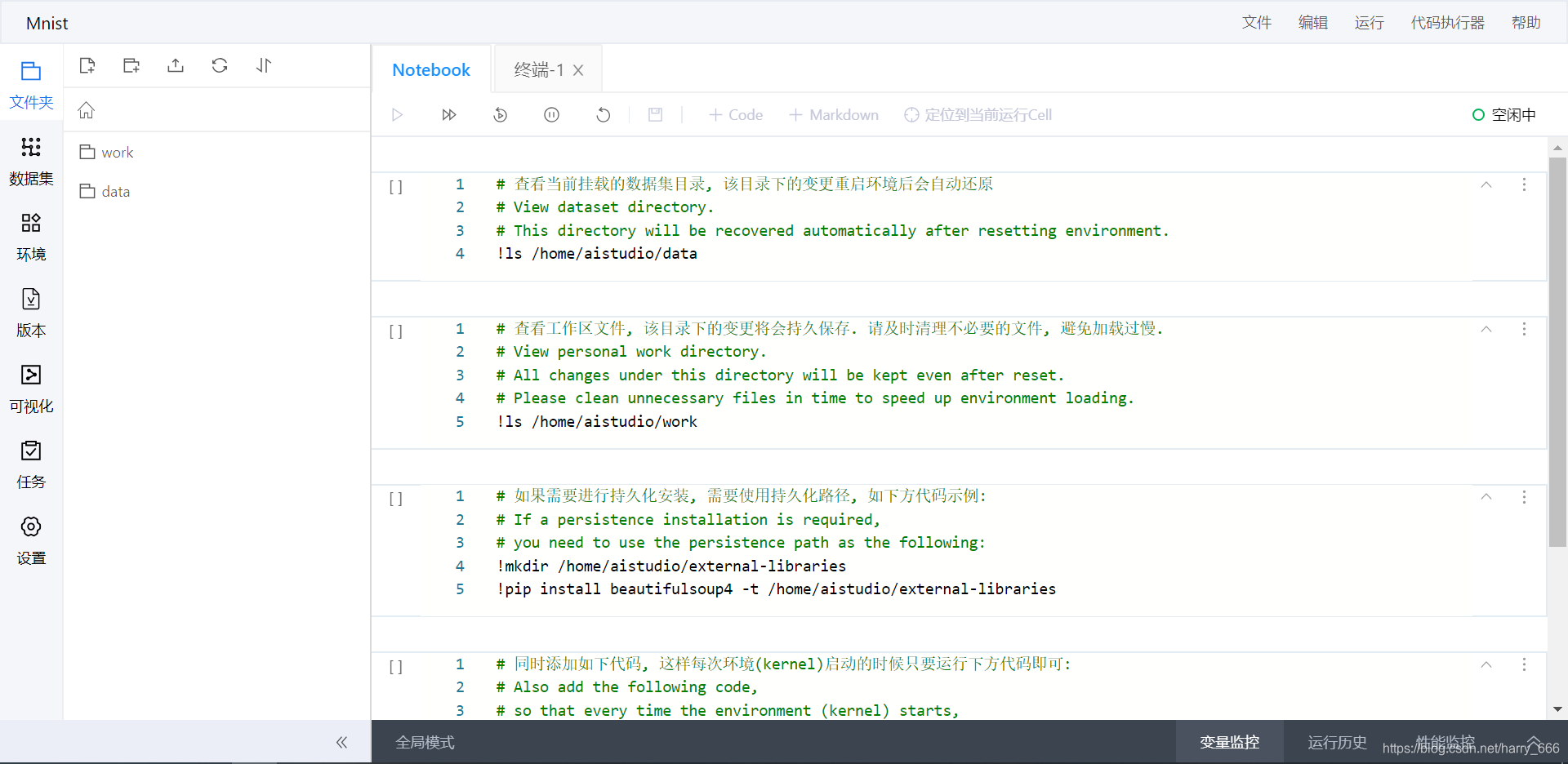
第二步:在notebook中书写代码
初始界面已经有四个代码块了,我们直接在最后新增一个代码块(cell),每一个cell点击左上角可以单独运行。
第一个cell:加载paddle和相关库
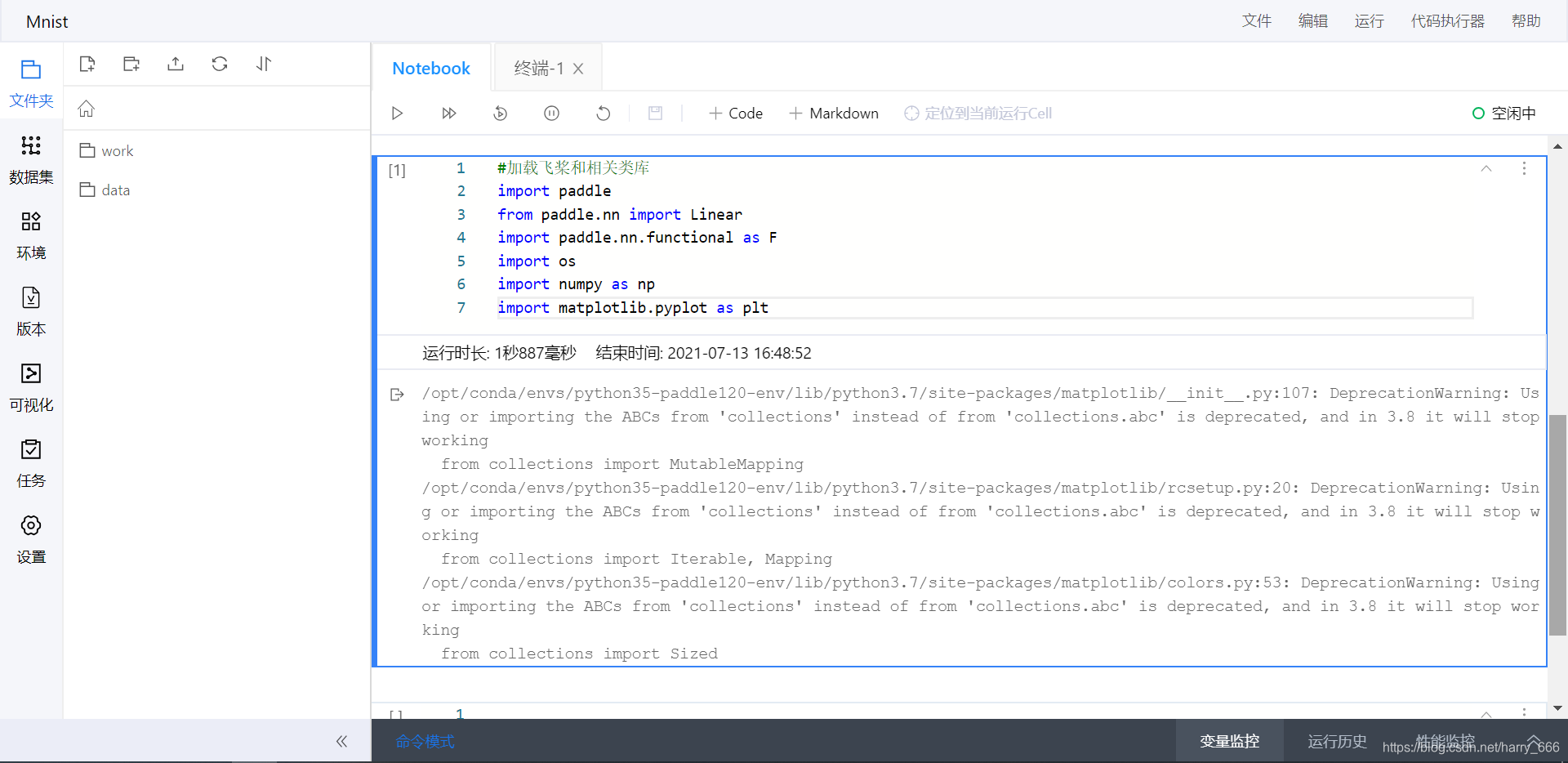
第二个cell:设置数据读取器,API自动读取MNIST数据训练集
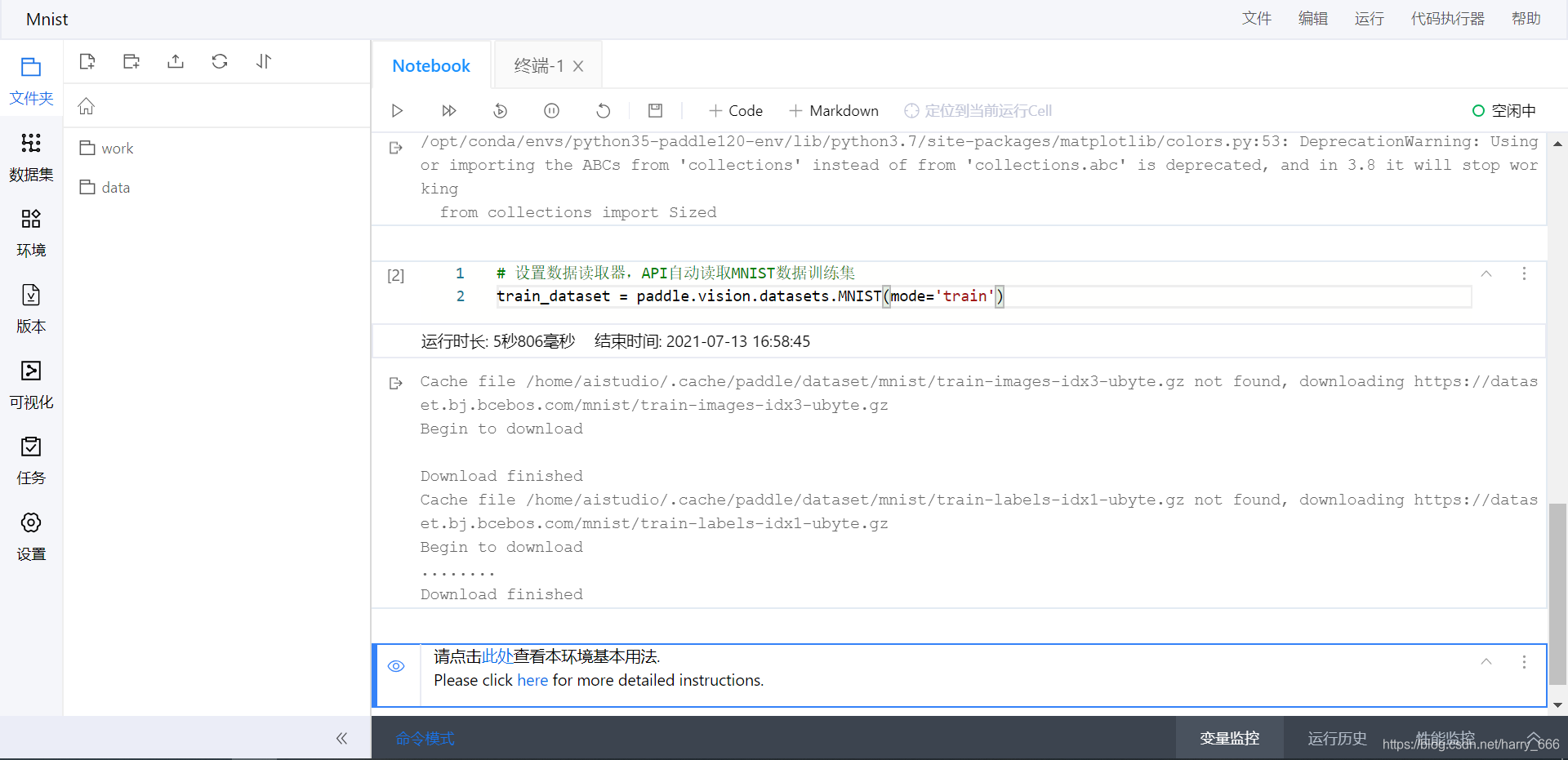
第三个cell:读取任意一个数据内容,观察打印结果。
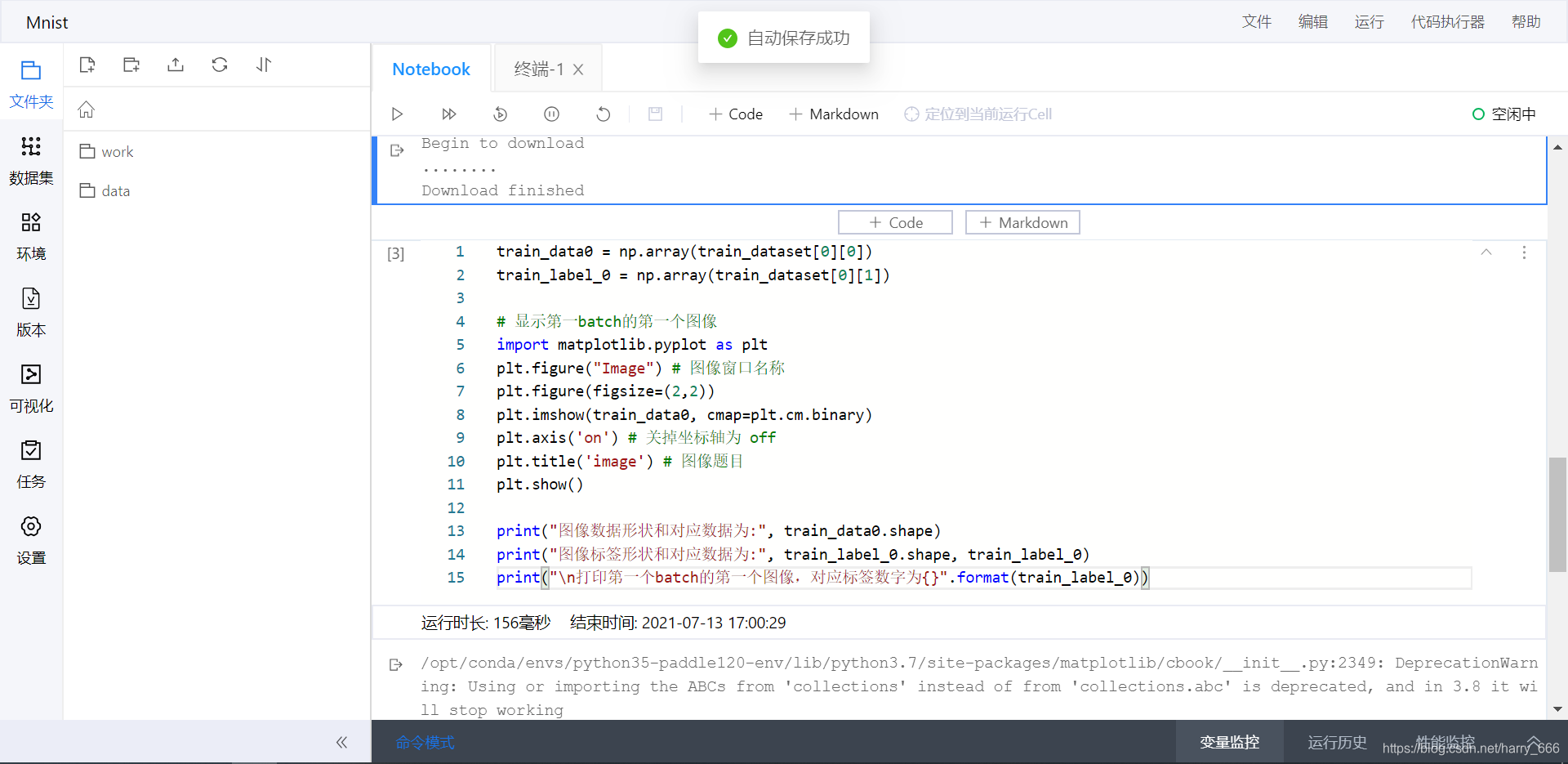 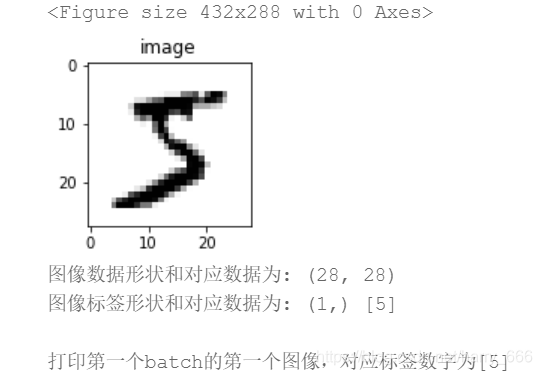
第四个cell:以类的方式组建手写数字识别的网络
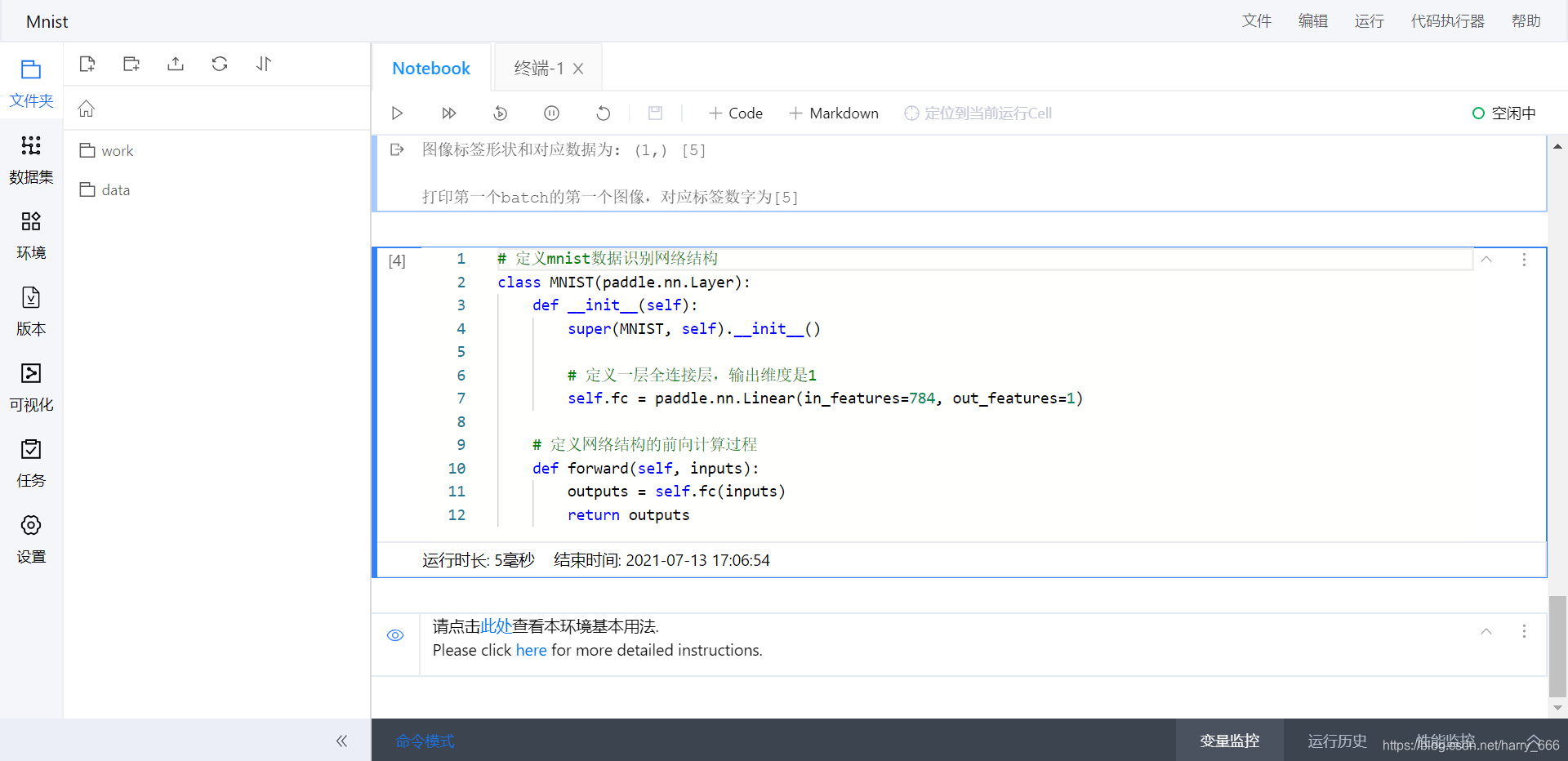
第五个cell:训练配置
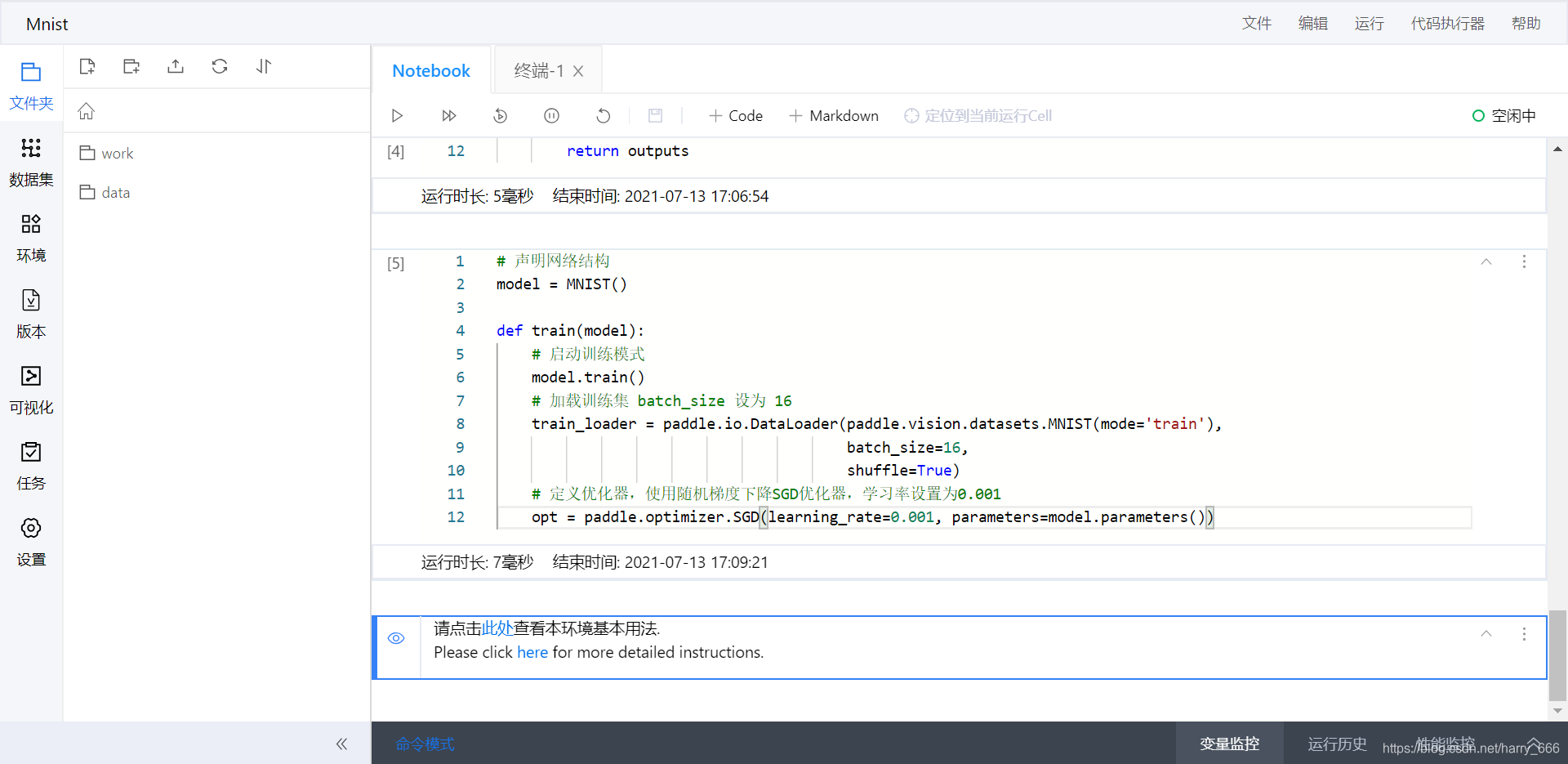
第六个cell:图像归一化
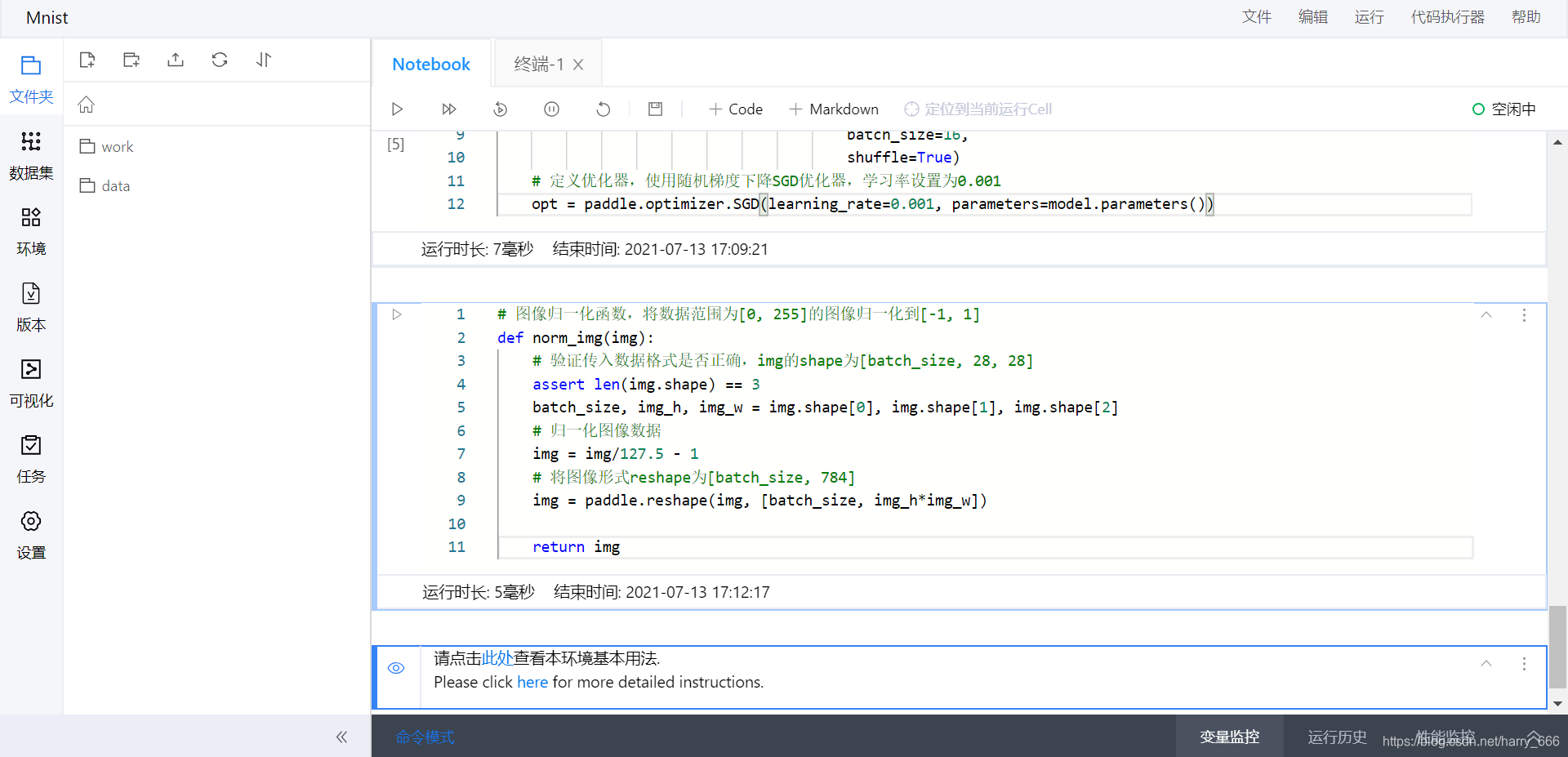
第七个cell:训练过程
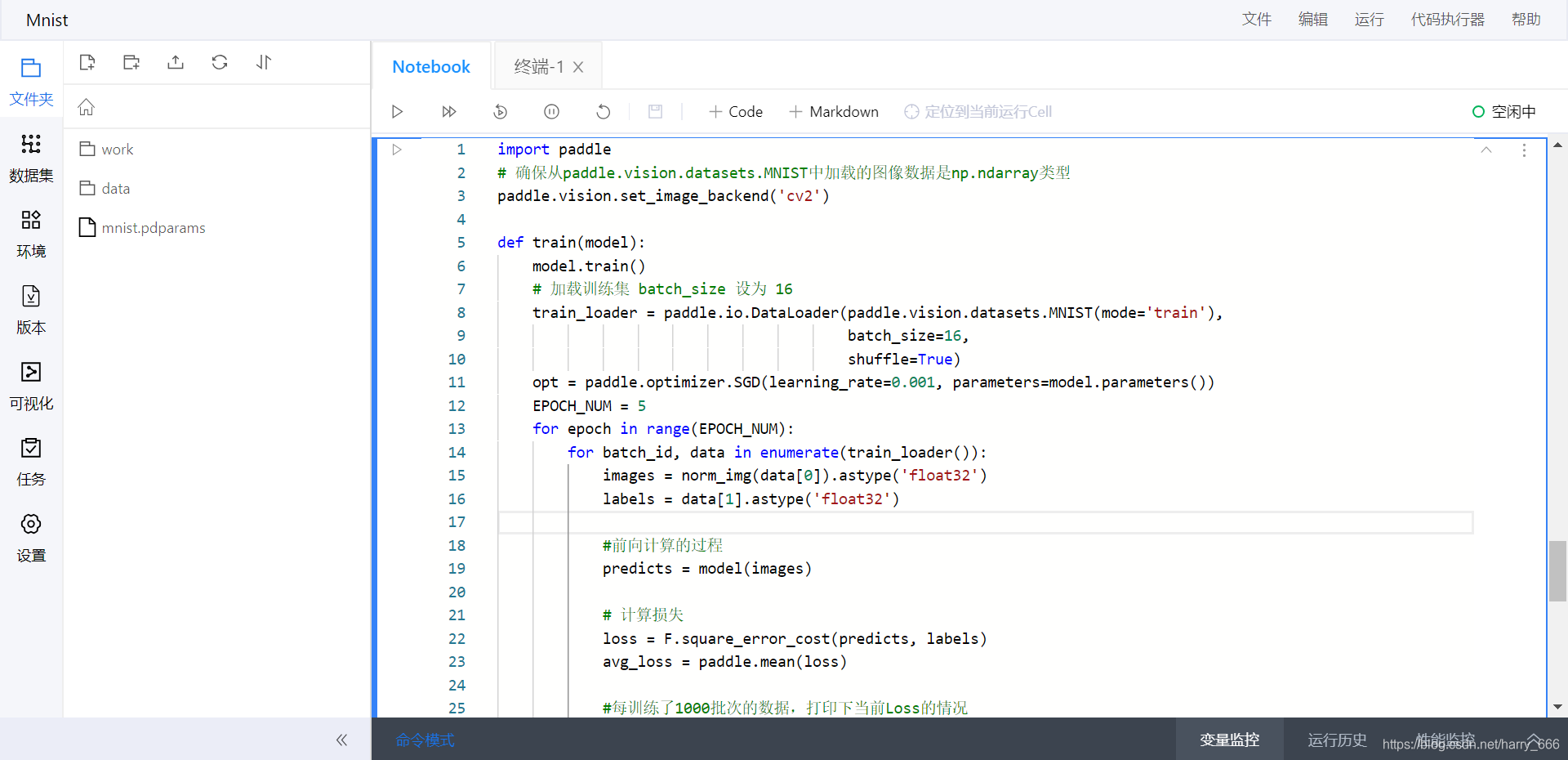 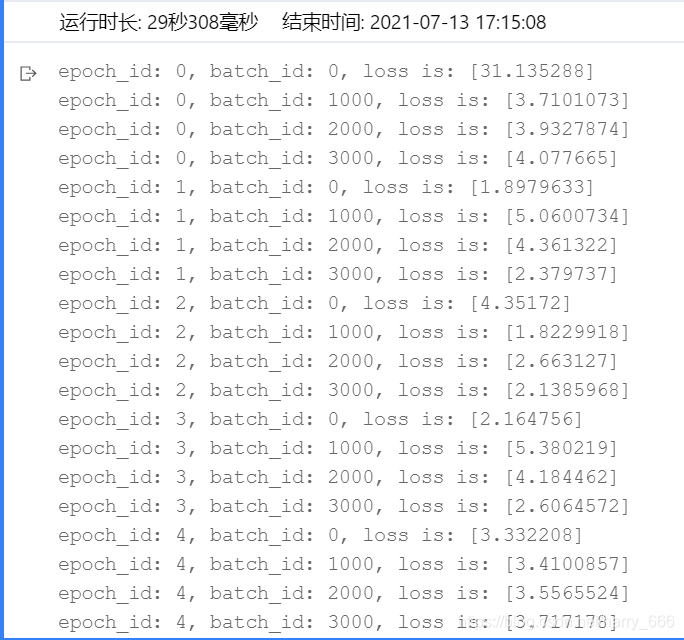 可以看到训练后左侧多了一个文件,就是训练好的模型。
第八个cell:上传本地图片
从本地上传一个数字图片,可以看到左侧多了一张jpg格式的图片
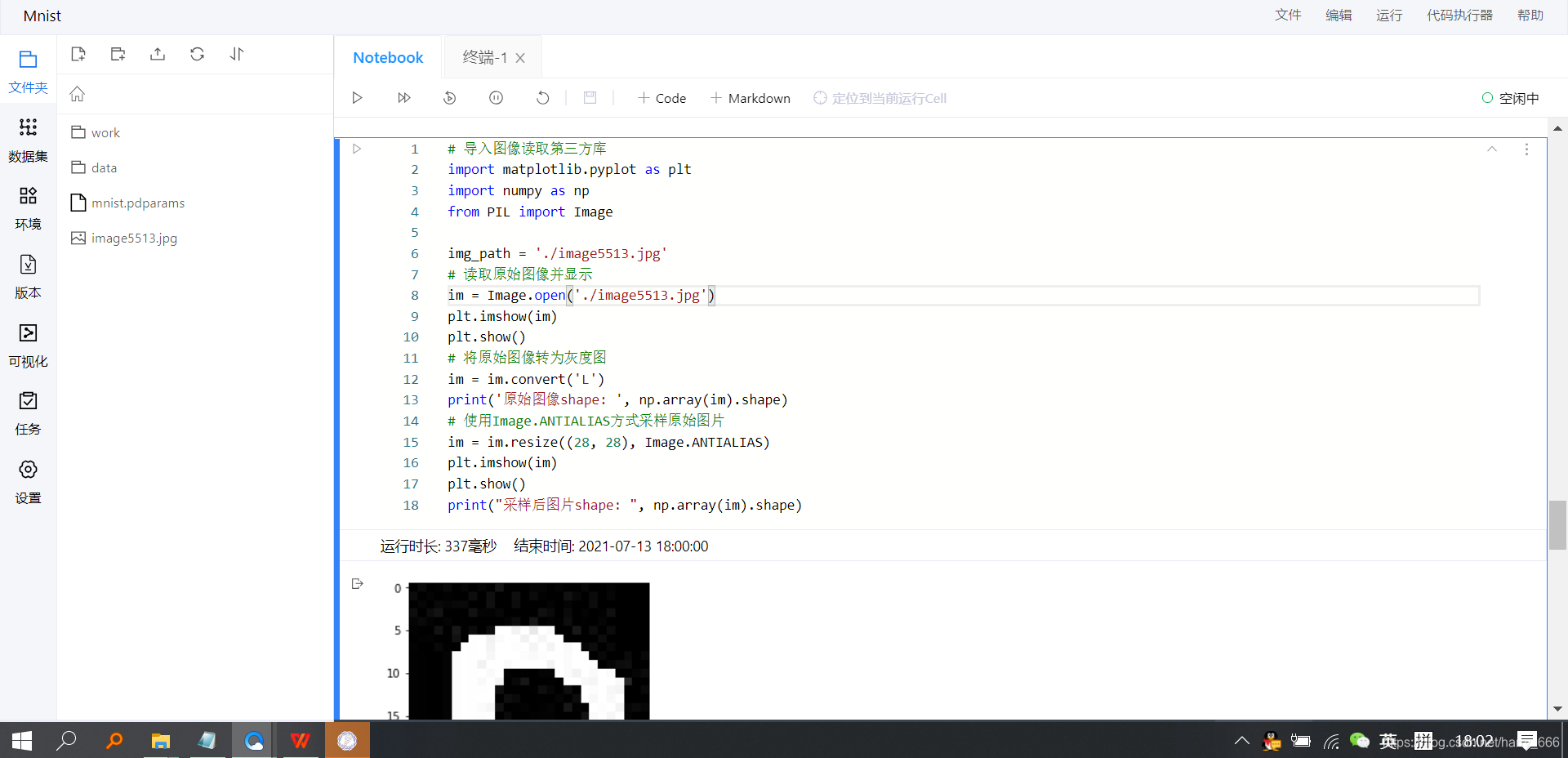 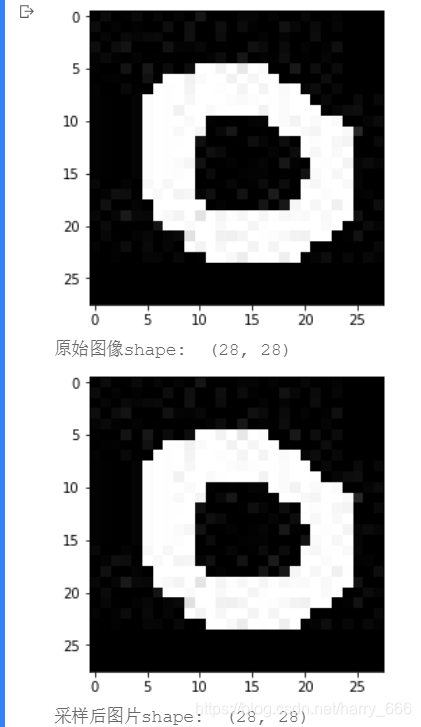 我放入的图片本来就是Mnist数据集里的图片,所以大小是一样的
第九个cell:测试过程
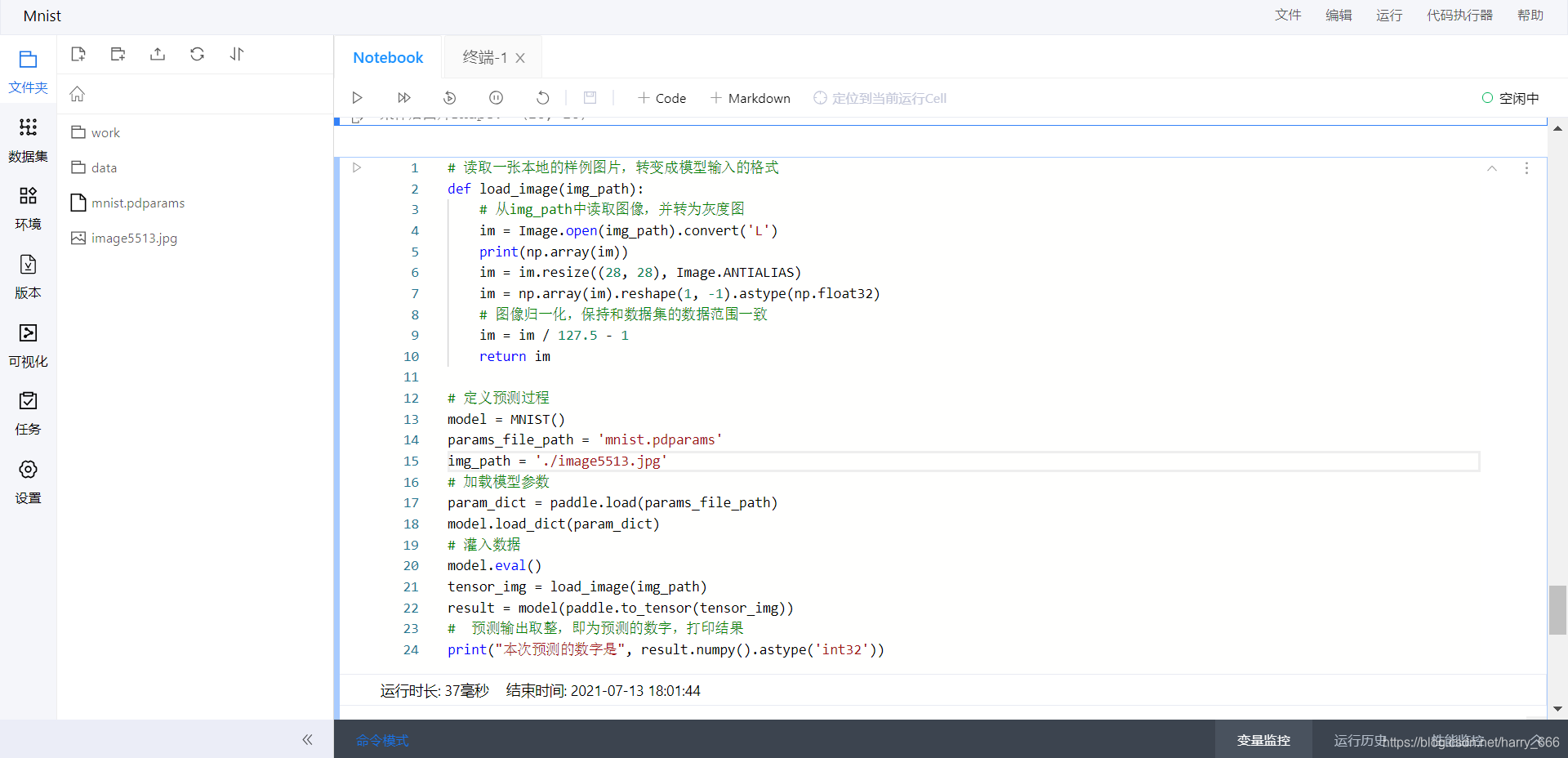 可以看到测试结果为0 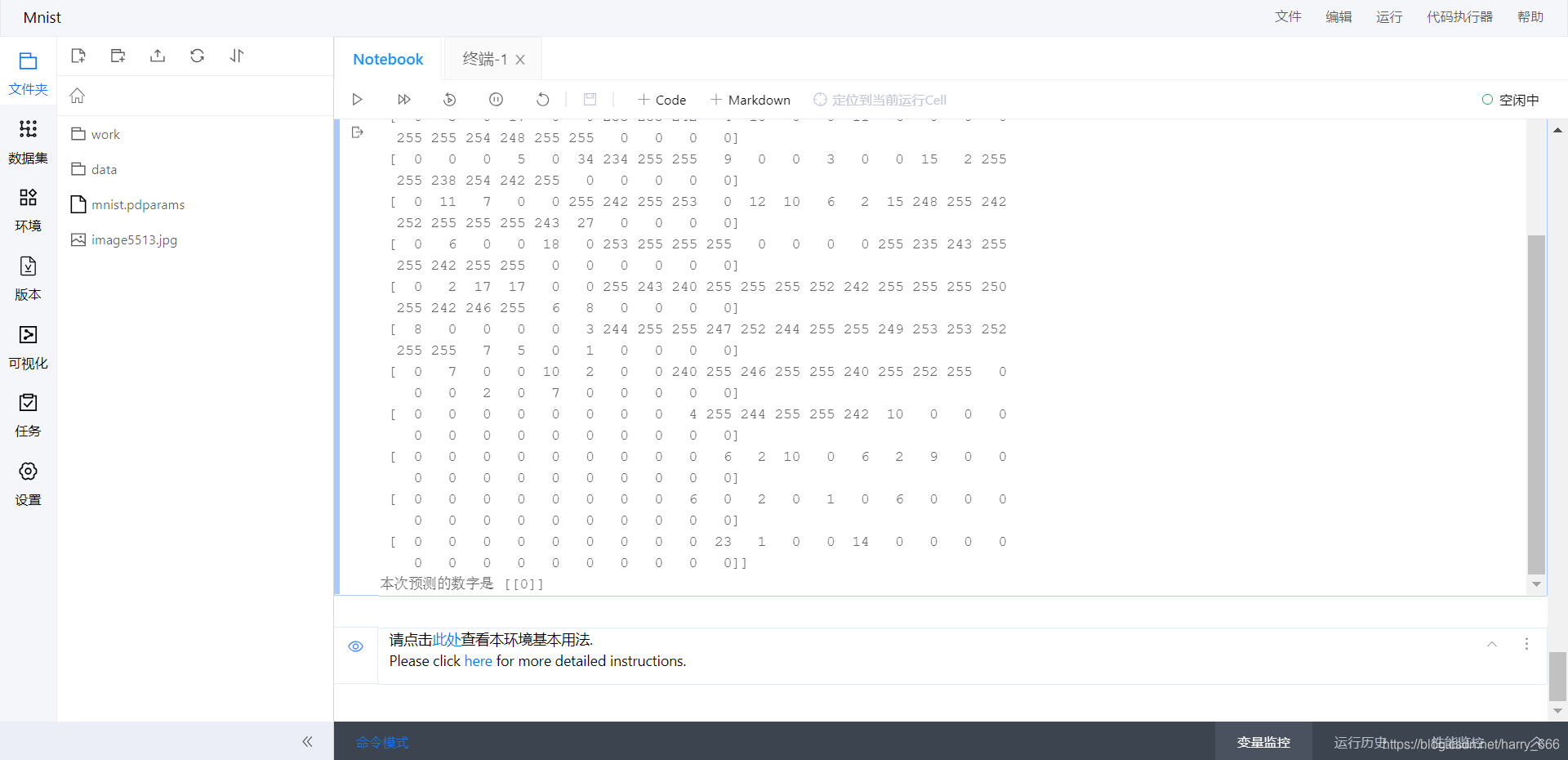
总代码:
cell1
import paddle
from paddle.nn import Linear
import paddle.nn.functional as F
import os
import numpy as np
import matplotlib.pyplot as plt
cell2
train_dataset = paddle.vision.datasets.MNIST(mode='train')
cell3
train_data0 = np.array(train_dataset[0][0])
train_label_0 = np.array(train_dataset[0][1])
import matplotlib.pyplot as plt
plt.figure("Image")
plt.figure(figsize=(2,2))
plt.imshow(train_data0, cmap=plt.cm.binary)
plt.axis('on')
plt.title('image')
plt.show()
print("图像数据形状和对应数据为:", train_data0.shape)
print("图像标签形状和对应数据为:", train_label_0.shape, train_label_0)
print("\n打印第一个batch的第一个图像,对应标签数字为{}".format(train_label_0))
cell4
class MNIST(paddle.nn.Layer):
def __init__(self):
super(MNIST, self).__init__()
self.fc = paddle.nn.Linear(in_features=784, out_features=1)
def forward(self, inputs):
outputs = self.fc(inputs)
return outputs
cell5
model = MNIST()
def train(model):
model.train()
train_loader = paddle.io.DataLoader(paddle.vision.datasets.MNIST(mode='train'),
batch_size=16,
shuffle=True)
opt = paddle.optimizer.SGD(learning_rate=0.001, parameters=model.parameters())
cell6
def norm_img(img):
assert len(img.shape) == 3
batch_size, img_h, img_w = img.shape[0], img.shape[1], img.shape[2]
img = img/127.5 - 1
img = paddle.reshape(img, [batch_size, img_h*img_w])
return img
cell7
import paddle
paddle.vision.set_image_backend('cv2')
def train(model):
model.train()
train_loader = paddle.io.DataLoader(paddle.vision.datasets.MNIST(mode='train'),
batch_size=16,
shuffle=True)
opt = paddle.optimizer.SGD(learning_rate=0.001, parameters=model.parameters())
EPOCH_NUM = 5
for epoch in range(EPOCH_NUM):
for batch_id, data in enumerate(train_loader()):
images = norm_img(data[0]).astype('float32')
labels = data[1].astype('float32')
predicts = model(images)
loss = F.square_error_cost(predicts, labels)
avg_loss = paddle.mean(loss)
if batch_id % 1000 == 0:
print("epoch_id: {}, batch_id: {}, loss is: {}".format(epoch, batch_id, avg_loss.numpy()))
avg_loss.backward()
opt.step()
opt.clear_grad()
train(model)
paddle.save(model.state_dict(), './mnist.pdparams')
cell8
import matplotlib.pyplot as plt
import numpy as np
from PIL import Image
img_path = './image5513.jpg'
im = Image.open('./image5513.jpg')
plt.imshow(im)
plt.show()
im = im.convert('L')
print('原始图像shape: ', np.array(im).shape)
im = im.resize((28, 28), Image.ANTIALIAS)
plt.imshow(im)
plt.show()
print("采样后图片shape: ", np.array(im).shape)
cell9
def load_image(img_path):
im = Image.open(img_path).convert('L')
print(np.array(im))
im = im.resize((28, 28), Image.ANTIALIAS)
im = np.array(im).reshape(1, -1).astype(np.float32)
im = im / 127.5 - 1
return im
model = MNIST()
params_file_path = 'mnist.pdparams'
img_path = './image5513.jpg'
param_dict = paddle.load(params_file_path)
model.load_dict(param_dict)
model.eval()
tensor_img = load_image(img_path)
result = model(paddle.to_tensor(tensor_img))
print("本次预测的数字是", result.numpy().astype('int32'))
总结
Mnist手写数字识别系统的开发流程大致如此,但是极简毕竟是极简,只是为了让大家熟悉,还有许多需要改进和优化的地方,比如这个0我换了好几张图片它才识别出来…可见准确率并不高。在明白了大致框架之后,我们再去填充和改进它的内容。需要学习和深入的地方还有很多,继续加油ing…
|