写在前面
做为我的第一个机器学习的练手项目,我选择了较为简单的MNIST手写字符集识别,因为MNIST在网上是开源的,但是我们需要将其处理成模型可使用的格式,因此这篇文章我就分享我的MNIST字符集下载以及数据集成过程。
conda下载tensorflow
在pycharm的这个地方可以通过命令行下载tensorflow:
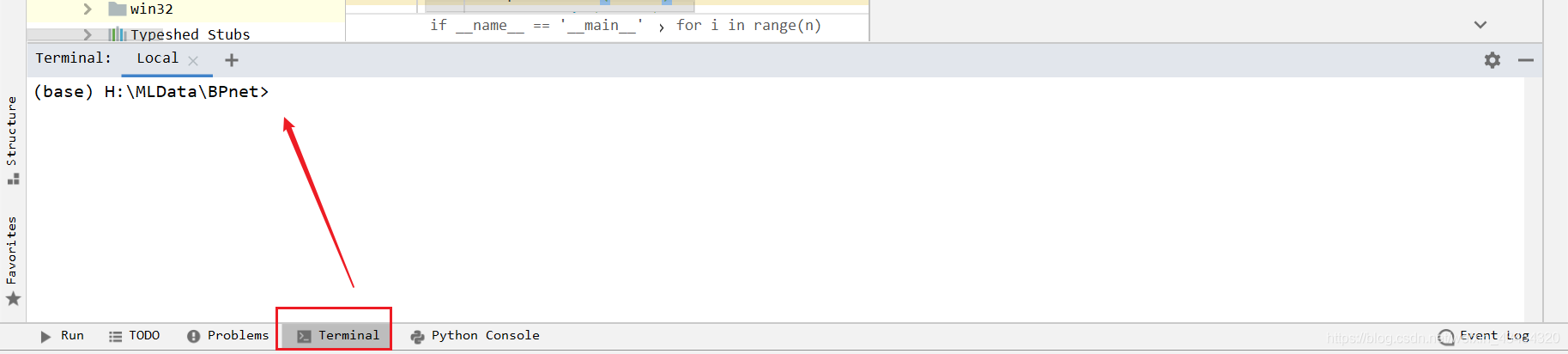 通过命令conda install tensorflow 之后,可能会报下面这个错误:
Collecting package metadata (current_repodata.json): failed
ProxyError: Conda cannot proceed due to an error in your proxy configuration.
Check for typos and other configuration errors in any '.netrc' file in your home directory,
any environment variables ending in '_PROXY', and any other system-wide proxy
configuration settings.
关掉当前开的VPN软件即可解决这个错误。
MNIST下载
言归正传,首先我们需要去MNIST的官网上下载手写字符集,地址在这里: http://yann.lecun.com/exdb/mnist/ 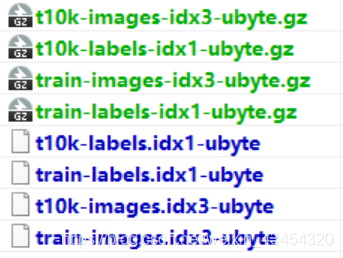 下载并且进行解压之后得到如下这些文件。
MNIST数据读取
在MNIST官网里下载下来的文件中由60k个数据的数据集(包含训练集和验证集),还有10k个数据的测试集,各个数据集分别由图片和标签组成,因此读取的时候我们需要分别进行读取:
def readFile(type=0):
if (type == 0):
with open('./dataSet/train-images.idx3-ubyte','rb') as ti:
train_image = ti.read()
with open('./dataSet/train-labels.idx1-ubyte', 'rb') as tl:
train_labels = tl.read()
return train_image,train_labels
elif (type == 1):
with open('./dataSet/t10k-images-idx3-ubyte', 'rb') as t_i:
test_image = t_i.read()
with open('./dataSet/t10k-labels-idx1-ubyte', 'rb') as t_l:
test_labels = t_l.read()
return test_image,test_labels
image, label = readFile()
我将数据读取都写在了一个函数中,由type 参数区别读取的文件类型,读取出来的数据存储在image, label 变量中,image变量中存储的是图像数值,MNIST字符集存储的手写字符集是28X28的大小,label变量存储的是图像对应的标签,也就是1~9。
将图片和标签读取出来之后是所有数据都合在一起的,但是在训练的时候我们需要将数据单个取出并且与标签对应起来,而且我们需要在数据流里面的任意位置开始读取任意长度的数据,所以我写了下面的程序:
img_size_bit = struct.calcsize('>784B')
lab_size_bit = struct.calcsize('>1B')
def getImages(image,n,startidx=0):
img = []
index = struct.calcsize('>IIII') + img_size_bit * startidx
for i in range(n):
temp = struct.unpack_from('>784B', image, index)
img.append(np.reshape(temp, (28, 28)))
index += img_size_bit
return img
def getLabels(label,n,startidx=0):
lab = []
index = struct.calcsize('>II') + lab_size_bit * startidx
for i in range(n):
temp = struct.unpack_from('>1B', label, index)
lab.append(temp[0])
index += lab_size_bit
return lab
因为图片是28X28=784大小的,在刚开始读取图像文件的时候是需要后移4个byte的,之后每次图片读取的索引都需要后移784byte。
在刚开始的时候标签文件需要后移2个byte,因为图像标签是1byte大小的,因此之后每次标签索引都需要后移1byte。
最后在main函数里面通过循环读取图像和标签文件,演示程序中取了9张图片和9个标签,从索引0开始读取的结果如下图所示: 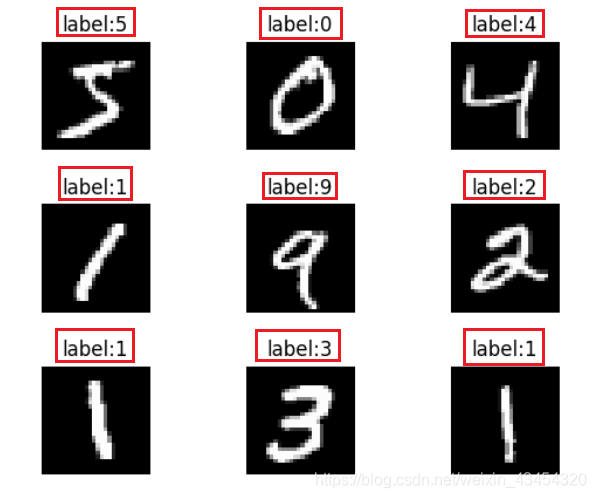 从索引4开始读取的结果如下图所示: 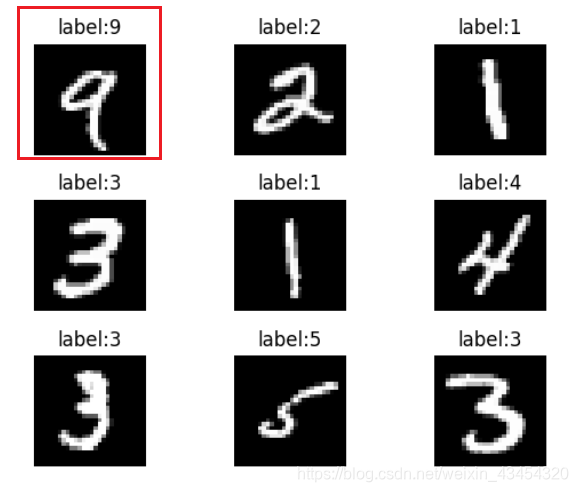 可以看到图片和标签一一对应,因此程序逻辑没有问题,明天就开始建立神经网络模型了。
源码:
import pandas
import struct
import numpy as np
import matplotlib.pyplot as plt
img_size_bit = struct.calcsize('>784B')
lab_size_bit = struct.calcsize('>1B')
def readFile(type=0):
if (type == 0):
with open('./dataSet/train-images.idx3-ubyte','rb') as ti:
train_image = ti.read()
with open('./dataSet/train-labels.idx1-ubyte', 'rb') as tl:
train_labels = tl.read()
return train_image,train_labels
elif (type == 1):
with open('./dataSet/t10k-images.idx3-ubyte', 'rb') as t_i:
test_image = t_i.read()
with open('./dataSet/t10k-labels.idx1-ubyte', 'rb') as t_l:
test_labels = t_l.read()
return test_image,test_labels
def getImages(image,n,startidx=0):
img = []
index = struct.calcsize('>IIII') + img_size_bit * startidx
for i in range(n):
temp = struct.unpack_from('>784B', image, index)
img.append(np.reshape(temp, (28, 28)))
index += img_size_bit
return img
def getLabels(label,n,startidx=0):
lab = []
index = struct.calcsize('>II') + lab_size_bit * startidx
for i in range(n):
temp = struct.unpack_from('>1B', label, index)
lab.append(temp[0])
index += lab_size_bit
return lab
if __name__ == '__main__':
n = 9
image, label = readFile(1)
train_img = getImages(image, n)
train_lab = getLabels(label, n)
for i in range(n):
plt.subplot(int(np.sqrt(n)), int(np.sqrt(n)), 1 + i)
title = "label:" + str(train_lab[i])
plt.title(title)
plt.axis('off')
plt.subplots_adjust(hspace=0.4)
plt.imshow(train_img[i], cmap='gray')
plt.show()
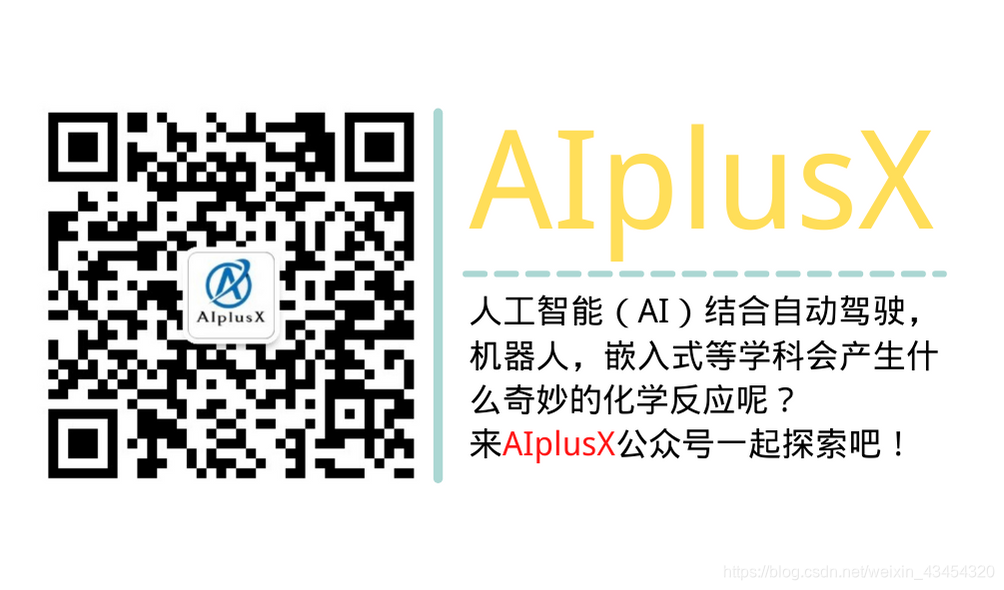
|