用python实现基于PANN的声音事件检测方法
1.PANN 国外论文:《PANNs: Large-Scale Pretrained Audio Neural Networks for Audio Pattern Recognition》
在本文中,我们提出了在大规模音频集数据集上训练的预先训练的音频神经网络(PANNs)。这些面板被转移到其他与音频相关的任务中。我们研究了由各种卷积神经网络建模的粒子的性能和计算复杂度。我们提出了一种称为波图-Logmel-CNN的架构,使用Log-mel谱图和波形作为输入特征。我们最好的PANN系统在音频集标签上实现了最先进的平均平均精度(mAP)为0.439,优于之前最好的0.392系统。我们将PANNs转移到六个音频模式识别任务中,并演示了在其中一些任务中最先进的性能。我们已经发布了PANNs的源代码和预训练的模型:
GitHub代码地址:https://github.com/qiuqiangkong/audioset_tagging_cnn
需要用到训练好的模型:Cnn14_DecisionLevelMax_mAP=0.385.pth
2.模型介绍 我们用的模型是CNN14架构 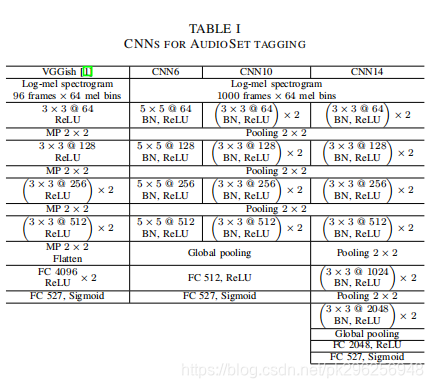 3.具体代码 ① 下载zip压缩文件 https://github.com/qiuqiangkong/audioset_tagging_cnn 创建你的项目保存以下文件 一部分在pytorch文件夹,另一部分utils文件夹 wav文件自己准备或者用压缩包里面的 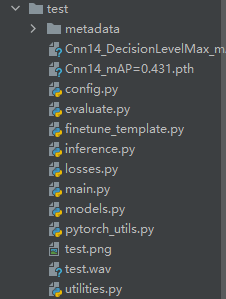 ② 修改inference.py文件,直接复制代码
import os
import sys
sys.path.insert(1, os.path.join(sys.path[0], '../utils'))
import numpy as np
import argparse
import librosa
import matplotlib.pyplot as plt
import torch
from utilities import create_folder, get_filename
from models import *
from pytorch_utils import move_data_to_device
import config
def audio_tagging(args):
"""Inference audio tagging result of an audio clip.
"""
sample_rate = args.sample_rate
window_size = args.window_size
hop_size = args.hop_size
mel_bins = args.mel_bins
fmin = args.fmin
fmax = args.fmax
model_type = args.model_type
checkpoint_path = args.checkpoint_path
audio_path = args.audio_path
device = torch.device('cuda') if args.cuda and torch.cuda.is_available() else torch.device('cpu')
classes_num = config.classes_num
labels = config.labels
Model = eval(model_type)
model = Model(sample_rate=sample_rate, window_size=window_size,
hop_size=hop_size, mel_bins=mel_bins, fmin=fmin, fmax=fmax,
classes_num=classes_num)
checkpoint = torch.load(checkpoint_path, map_location=device)
model.load_state_dict(checkpoint['model'])
if 'cuda' in str(device):
model.to(device)
print('GPU number: {}'.format(torch.cuda.device_count()))
model = torch.nn.DataParallel(model)
else:
print('Using CPU.')
(waveform, _) = librosa.core.load(audio_path, sr=sample_rate, mono=True)
waveform = waveform[None, :]
waveform = move_data_to_device(waveform, device)
with torch.no_grad():
model.eval()
batch_output_dict = model(waveform, None)
clipwise_output = batch_output_dict['clipwise_output'].data.cpu().numpy()[0]
"""(classes_num,)"""
sorted_indexes = np.argsort(clipwise_output)[::-1]
for k in range(10):
print('{}: {:.3f}'.format(np.array(labels)[sorted_indexes[k]],
clipwise_output[sorted_indexes[k]]))
if 'embedding' in batch_output_dict.keys():
embedding = batch_output_dict['embedding'].data.cpu().numpy()[0]
print('embedding: {}'.format(embedding.shape))
return clipwise_output, labels
def sound_event_detection(args):
"""Inference sound event detection result of an audio clip.
"""
sample_rate = args.sample_rate
window_size = args.window_size
hop_size = args.hop_size
mel_bins = args.mel_bins
fmin = args.fmin
fmax = args.fmax
model_type = args.model_type
checkpoint_path = args.checkpoint_path
audio_path = args.audio_path
device = torch.device('cuda') if args.cuda and torch.cuda.is_available() else torch.device('cpu')
classes_num = config.classes_num
labels = config.labels
frames_per_second = sample_rate // hop_size
fig_path = os.path.join('results', '{}.png'.format(get_filename(audio_path)))
create_folder(os.path.dirname(fig_path))
Model = eval(model_type)
model = Model(sample_rate=sample_rate, window_size=window_size,
hop_size=hop_size, mel_bins=mel_bins, fmin=fmin, fmax=fmax,
classes_num=classes_num)
checkpoint = torch.load(checkpoint_path, map_location=device)
model.load_state_dict(checkpoint['model'])
if 'cuda' in str(device):
model.to(device)
print('GPU number: {}'.format(torch.cuda.device_count()))
model = torch.nn.DataParallel(model)
else:
print('Using CPU.')
(waveform, _) = librosa.core.load(audio_path, sr=sample_rate, mono=True)
waveform = waveform[None, :]
waveform = move_data_to_device(waveform, device)
with torch.no_grad():
model.eval()
batch_output_dict = model(waveform, None)
framewise_output = batch_output_dict['framewise_output'].data.cpu().numpy()[0]
"""(time_steps, classes_num)"""
print('Sound event detection result (time_steps x classes_num): {}'.format(
framewise_output.shape))
sorted_indexes = np.argsort(np.max(framewise_output, axis=0))[::-1]
top_k = 10
top_result_mat = framewise_output[:, sorted_indexes[0: top_k]]
"""(time_steps, top_k)"""
stft = librosa.core.stft(y=waveform[0].data.cpu().numpy(), n_fft=window_size,
hop_length=hop_size, window='hann', center=True)
frames_num = stft.shape[-1]
fig, axs = plt.subplots(2, 1, sharex=True, figsize=(10, 4))
axs[0].matshow(np.log(np.abs(stft)), origin='lower', aspect='auto', cmap='jet')
axs[0].set_ylabel('Frequency bins')
axs[0].set_title('Log spectrogram')
axs[1].matshow(top_result_mat.T, origin='upper', aspect='auto', cmap='jet', vmin=0, vmax=1)
axs[1].xaxis.set_ticks(np.arange(0, frames_num, frames_per_second))
axs[1].xaxis.set_ticklabels(np.arange(0, frames_num / frames_per_second))
axs[1].yaxis.set_ticks(np.arange(0, top_k))
axs[1].yaxis.set_ticklabels(np.array(labels)[sorted_indexes[0: top_k]])
axs[1].yaxis.grid(color='k', linestyle='solid', linewidth=0.3, alpha=0.3)
axs[1].set_xlabel('Seconds')
axs[1].xaxis.set_ticks_position('bottom')
plt.tight_layout()
plt.savefig(fig_path)
print('Save sound event detection visualization to {}'.format(fig_path))
return framewise_output, labels
class Parser:
def _init_(self, sample_rate, window_size, hop_size, mel_bins, fmin, fmax, model_type, checkpoint_path, audio_path,
cuda):
self.sample_rate = sample_rate
self.window_size = window_size
self.hop_size = hop_size
self.mel_bins = mel_bins
self.fmin = fmin
self.fmax = fmax
self.model_type = model_type
self.checkpoint_path = checkpoint_path
self.audio_path = audio_path
self.cuda = cuda
if __name__ == '__main__':
parser_at = Parser()
parser_at.sample_rate = 32000
parser_at.window_size = 1024
parser_at.hop_size = 320
parser_at.mel_bins = 64
parser_at.fmin = 50
parser_at.fmax = 14000
parser_at.model_type = "Cnn14"
parser_at.checkpoint_path = "Cnn14_mAP=0.431.pth"
parser_at.audio_path = "test.wav"
parser_at.cuda = False
print("audio_tagging")
audio_tagging(parser_at)
parser_sed = Parser()
parser_sed.sample_rate = 32000
parser_sed.window_size = 1024
parser_sed.hop_size = 320
parser_sed.mel_bins = 64
parser_sed.fmin = 50
parser_sed.fmax = 14000
parser_sed.model_type = "Cnn14_DecisionLevelMax"
parser_sed.checkpoint_path = "Cnn14_DecisionLevelMax_mAP=0.385.pth"
parser_sed.audio_path = "test.wav"
parser_sed.cuda = False
print("sound_event_detection")
sound_event_detection(parser_sed)
③实现效果 功能1实现效果 小数:可信度 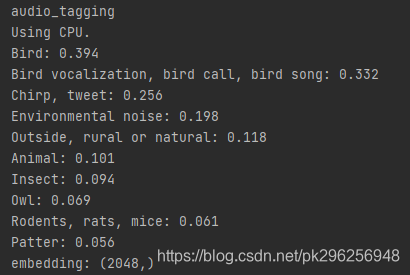 功能2实现效果 如图所示,蓝色越浅,可信度越高 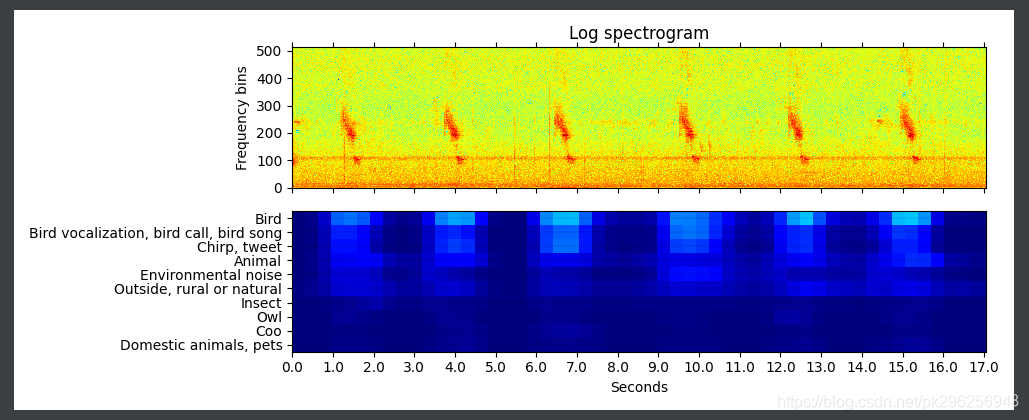 4.拓展实现 ①提取需要的声音,来做语音识别、声纹识别之类的 ②提取需要的声音,重新训练,训练检测单一种类:人声,鸟声,猫叫声等,用cnn10或者cnn6模型训练,提高检测速率。
|