目标检测 YOLOv5 - 在多类别中应用NMS(非极大值抑制)
flyfish
非极大值抑制(Non-maximum Suppression (NMS))的作用简单说就是模型检测出了很多框,我应该留哪些。 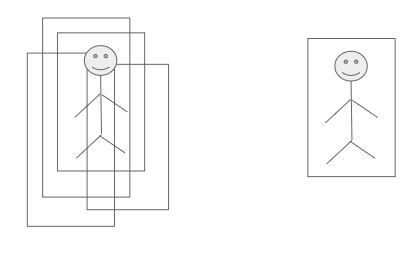
根据参数执行多个类一起应用NMS还是执行按照不同的类分别应用NMS 不同的类分别应用NMS(非极大值抑制),即每个索引值对应一个类别,不同类别的元素之间不会应用NMS。 实现方法一句话 多类别NMS(非极大值抑制)的处理策略是为了让每个类都能独立执行NMS,在所有的边框上添加一个偏移量。偏移量仅取决于类IDX,并且足够大,以便来自不同类的框不会重叠。
YOLOv5的处理方式
就是上面的一句话。 实现代码在utils/general.py
c = x[:, 5:6] * (0 if agnostic else max_wh)
boxes, scores = x[:, :4] + c, x[:, 4]
i = torchvision.ops.nms(boxes, scores, iou_thres)
agnostic参数 True表示多个类一起计算nms,False表示按照不同的类分别进行计算nms 代码重点是在 '+c’这里的c就是偏移量 x[:, :4]表示box(从二维看第0,1,2,3列) x[:, 4] 表示分数(从二维看第4列) x[:, 5:6]表示类IDX(从二维看第5列) max_wh这里是4096,这样偏移量仅取决于类IDX,并且足够大。
在终端执行命令行的时候,可以传参决定执行多个类一起应用NMS还是执行按照不同的类分别应用NMS
detect.py
pred = non_max_suppression(pred, conf_thres, iou_thres, classes, agnostic_nms, max_det=max_det)
parser.add_argument('--agnostic-nms', action='store_true', help='class-agnostic NMS')
不同的类分别应用NMS,普通方式的实现
最普通的的方式就是一个for循环,分别计算每一个类的NMS for循环for class_id in torch.unique(idxs)
def _batched_nms_vanilla(
boxes: Tensor,
scores: Tensor,
idxs: Tensor,
iou_threshold: float,
) -> Tensor:
keep_mask = torch.zeros_like(scores, dtype=torch.bool)
for class_id in torch.unique(idxs):
curr_indices = torch.where(idxs == class_id)[0]
curr_keep_indices = nms(boxes[curr_indices], scores[curr_indices], iou_threshold)
keep_mask[curr_indices[curr_keep_indices]] = True
keep_indices = torch.where(keep_mask)[0]
return keep_indices[scores[keep_indices].sort(descending=True)[1]]
不同的类分别应用NMS,使用加偏移量的实现
def _batched_nms_coordinate_trick(
boxes: Tensor,
scores: Tensor,
idxs: Tensor,
iou_threshold: float,
) -> Tensor:
if boxes.numel() == 0:
return torch.empty((0,), dtype=torch.int64, device=boxes.device)
max_coordinate = boxes.max()
offsets = idxs.to(boxes) * (max_coordinate + torch.tensor(1).to(boxes))
boxes_for_nms = boxes + offsets[:, None]
keep = nms(boxes_for_nms, scores, iou_threshold)
return keep
YOLOv5也可以不用自己实现,调用PyTorch的torchvision.ops.batched_nms
为什么参数用class-agnostic这个词
目标检测器有类别不可知检测器(class-agnostic detector)和类别可知检测器(class-aware detector)。 类别不可知检测器(class-agnostic detector)在不知道它们属于哪个类别的情况下检测到一堆对象。简单地说,他们只探测“前景”物体。类似前景={猫,狗,车,飞机,…。}。因为它不知道它检测到的对象的类别,所以我们称之为class-agnostic(类不可知性)。 类别可知检测器(class-aware detector)在检测出框时就检测出了类别,class与box已经做了关联。 所以当不知道类别只有边框或者所有类的所有边框一起应用NMS时,class-agnostic就设置为True
|