一、认识
? ? ? ?奇异值分解(Singular Value Decomposition,以下简称SVD)是在机器学习领域广泛应用的算法,它不光可以用于降维算法中的特征分解,还可以用于推荐系统,以及自然语言处理等领域。是很多机器学习算法的基石
1.1特征值和特征向量
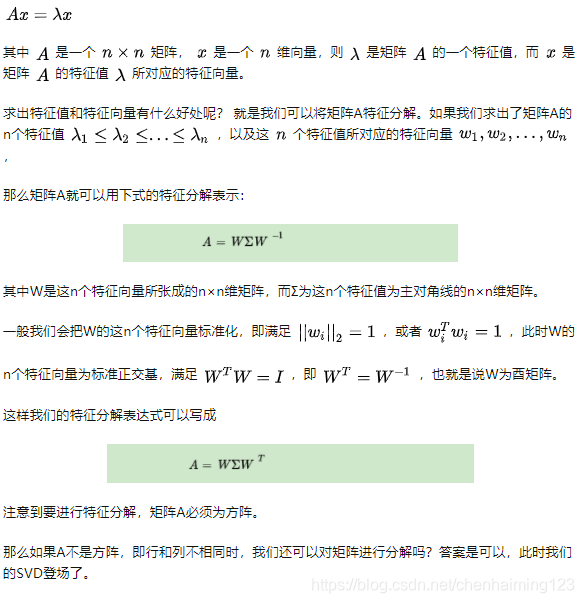
? ? ? ? ? ? ? ? ? ? ? ? ? ? ? ? ? ? ? ? ? (注:特征值和特征向量的图来自网络)
?1.2.SVD的相关内容
? ? ? ?不做解释,需要先认识定义,然后做基本的向量运算,还有内容很多啊!
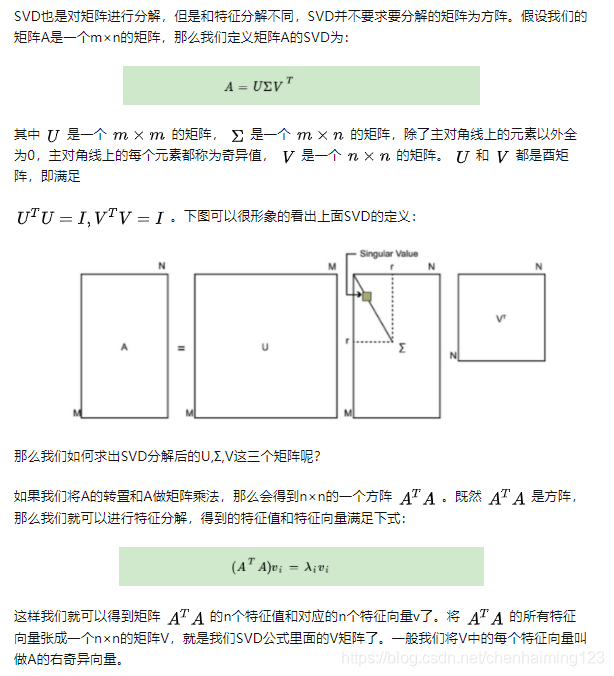
?
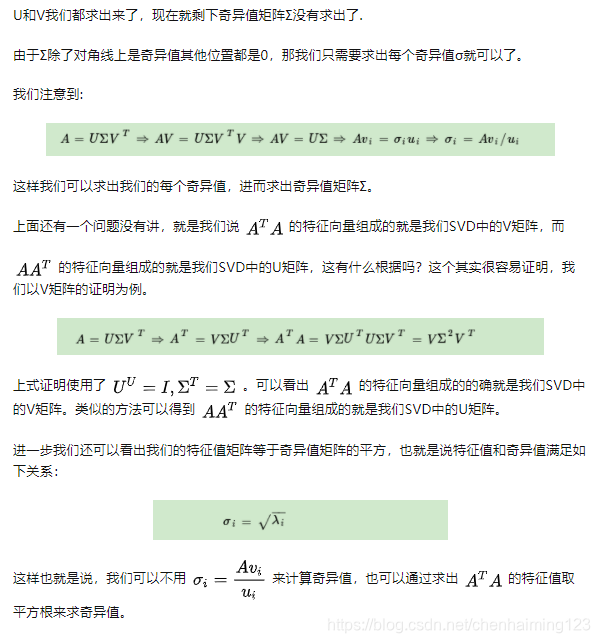
?
? 二 、设计java代码
package no.uib.cipr.matrix;
import com.github.fommil.netlib.LAPACK;
import org.netlib.util.intW;
public class SVD
{
private final double[] work;
private final int[] iwork;
private final int m;
private final int n;
private final boolean vectors;
private final JobSVD job;
private final double[] S;
private final DenseMatrix U;
private final DenseMatrix Vt;
public SVD(int m, int n)
{
this(m, n, true);
}
public SVD(int m, int n, boolean vectors)
{
this.m = m;
this.n = n;
this.vectors = vectors;
this.S = new double[Math.min(m, n)];
if (vectors)
{
this.U = new DenseMatrix(m, m);
this.Vt = new DenseMatrix(n, n);
}
else
{
this.U = (this.Vt = null);
}
this.job = (vectors ? JobSVD.All : JobSVD.None);
this.iwork = new int[8 * Math.min(m, n)];
double[] worksize = new double[1];
intW info = new intW(0);
LAPACK.getInstance().dgesdd(this.job.netlib(), m, n, new double[0],
Matrices.ld(m), new double[0], new double[0], Matrices.ld(m), new double[0],
Matrices.ld(n), worksize, -1, this.iwork, info);
int lwork = -1;
if (info.val != 0)
{
if (vectors) {
lwork = 3 * Math.min(m, n) * Math.min(m, n) + Math.max(
Math.max(m, n), 4 *
Math.min(m, n) * Math.min(m, n) + 4 *
Math.min(m, n));
} else {
lwork = 3 * Math.min(m, n) * Math.min(m, n) + Math.max(
Math.max(m, n), 5 *
Math.min(m, n) * Math.min(m, n) + 4 *
Math.min(m, n));
}
}
else {
lwork = (int)worksize[0];
}
lwork = Math.max(lwork, 1);
this.work = new double[lwork];
}
public static SVD factorize(Matrix A)
throws NotConvergedException
{
return new SVD(A.numRows(), A.numColumns()).factor(new DenseMatrix(A));
}
public SVD factor(DenseMatrix A)
throws NotConvergedException
{
if (A.numRows() != this.m) {
throw new IllegalArgumentException("A.numRows() != m");
}
if (A.numColumns() != this.n) {
throw new IllegalArgumentException("A.numColumns() != n");
}
intW info = new intW(0);
LAPACK.getInstance().dgesdd(this.job.netlib(), this.m, this.n, A.getData(),
Matrices.ld(this.m), this.S, this.vectors ? this.U.getData() : new double[0],
Matrices.ld(this.m), this.vectors ? this.Vt.getData() : new double[0],
Matrices.ld(this.n), this.work, this.work.length, this.iwork, info);
if (info.val > 0) {
throw new NotConvergedException(NotConvergedException.Reason.Iterations);
}
if (info.val < 0) {
throw new IllegalArgumentException();
}
return this;
}
public boolean hasSingularVectors()
{
return this.U != null;
}
public DenseMatrix getU()
{
return this.U;
}
public DenseMatrix getVt()
{
return this.Vt;
}
public double[] getS()
{
return this.S;
}
}
|