1.图像着色算法原理
图像着色,通俗讲就是对黑白的照片进行处理,生成为彩色的图像。有点像买的图框画,自己用颜料在图框中进行填色。
算法原理上用到了上一节讲到的Lab颜色空间,具体模型架构如下图所示: 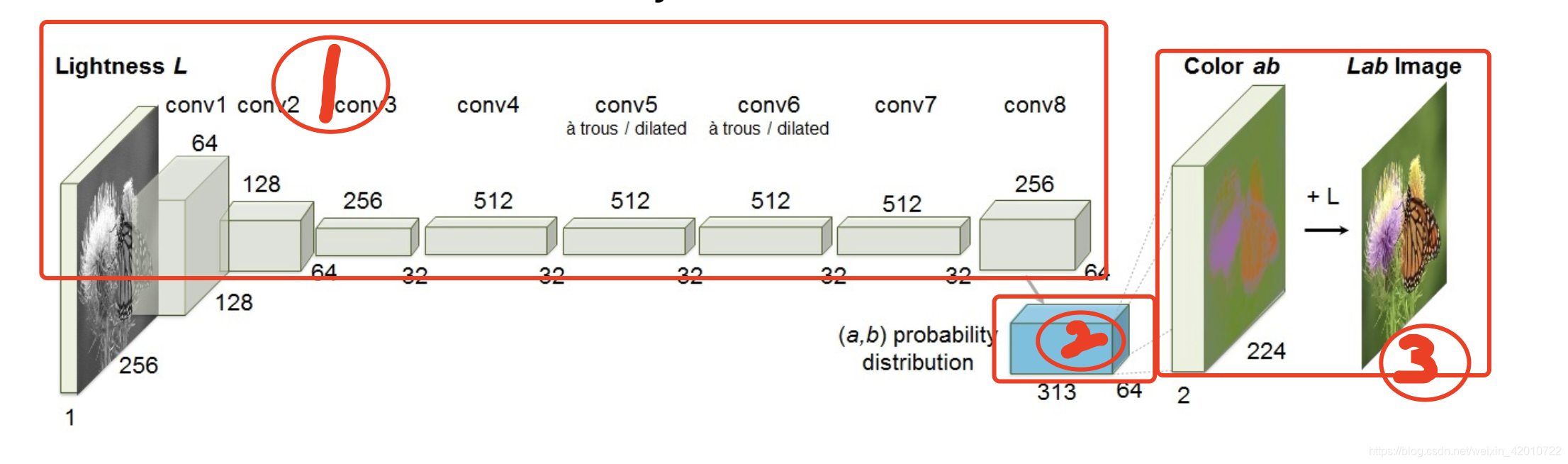
1.1 模型架构
这里我把模型分为三个部分,对这三部分进行详细解释。
第一部分
第一部分实际是一个典型的VGG16模型,只不过去掉了VGG16后面池化层部分,在后面加上如下表的卷积层
卷积层 | 通道数 | 卷积核 | 步长 | 填充padding | 备注 |
---|
Conv7_1 | 512 | 3x3 | 1 | 1 | 卷积后进行ReLU | Conv7_2 | 512 | 3x3 | 1 | 1 | 卷积后进行ReLU | Conv7_3 | 512 | 3x3 | 1 | 1 | 卷积后进行ReLU,再进行一次批次归一化处理nn.BatchNorm2d | Conv8_1 | 256 | 4x4 | 2 | 1 | 卷积后进行ReLU | Conv8_2 | 256 | 3x3 | 1 | 1 | 卷积后进行ReLU | Conv8_3 | 256 | 3x3 | 1 | 1 | 卷积后进行ReLU |
注意这里Conv8_1使用的是反卷积。 反卷积函数如下:
class torch.nn.ConvTranspose2d(in_channels, out_channels,kernel_size, stride=1, padding=0, output_padding=0, groups=1, bias=True, dilation=1)
反卷积公式如下:
o
u
t
p
u
t
=
(
i
n
p
u
t
?
1
)
?
s
t
r
i
d
e
+
o
u
t
p
u
t
p
a
d
d
i
n
g
?
2
?
p
a
d
d
i
n
g
+
k
e
r
n
e
l
s
i
z
e
output=(input-1)*stride+outputpadding-2*padding+kernelsize
output=(input?1)?stride+outputpadding?2?padding+kernelsize
第二部分
第二部分为一个简单的卷积运算。卷积层通道数为313,1x1,步长为1,padding为0。
第三部分
- 首先将输入的图像进行一次softmax,得到每一个通道下各像素值的概率分布,其中每一个通道下的像素值的和为1。
- 再进行一次卷积,卷积层通道数为2,padding为0,步长为1,并对图像的纹理边缘进行一次加强dilation。
- 在前面卷积后对图像,再进行一次向上采样恢复原始图像尺寸,最后乘以固定参数值110,得到通道ab的图像。
1.2 算法步骤
- 将所有训练图像从RGB颜色空间转换为Lab颜色空间,通过原始图像的ab值
Z
Z
Z和模型训练预测的ab值
Z
^
\hat{Z}
Z^,计算loss
L
(
Z
^
,
Z
)
=
?
1
H
W
∑
h
,
w
∑
q
Z
h
,
w
,
q
l
o
g
(
Z
^
h
,
w
,
q
)
L(\hat{Z},Z)=-\frac{1}{HW}\sum_{h,w}\sum_{q}Z_{h,w,q}log(\hat{Z}_{h,w,q})
L(Z^,Z)=?HW1?∑h,w?∑q?Zh,w,q?log(Z^h,w,q?) - 将想要着色的图像的L通道作为输入,输入到模型网络中,来预测ab通道。
- 将输入的L通道与预测出来的ab通道进行结合
- 将Lab图像转换回RGB
pytorch实现图像着色
由于模型训练太长了 这里选择下载别人已经训练好的模型 训练模型 代码目录
\colorizers
\img
\imgout
\main.py
\model.py
\util.py
其中img存放需要着色的图片,imgout保存生成的图片,util实现图像处理,model.py定义模型代码。
model.py
class model():
def __init__(self, norm_layer=nn.BatchNorm2d):
super(model, self).__init__()
self.ab_norm=110
model1=[nn.Conv2d(1, 64, kernel_size=3, stride=1, padding=1, bias=True),]
model1+=[nn.ReLU(True),]
model1+=[nn.Conv2d(64, 64, kernel_size=3, stride=2, padding=1, bias=True),]
model1+=[nn.ReLU(True),]
model1+=[norm_layer(64),]
model2=[nn.Conv2d(64, 128, kernel_size=3, stride=1, padding=1, bias=True),]
model2+=[nn.ReLU(True),]
model2+=[nn.Conv2d(128, 128, kernel_size=3, stride=2, padding=1, bias=True),]
model2+=[nn.ReLU(True),]
model2+=[norm_layer(128),]
model3=[nn.Conv2d(128, 256, kernel_size=3, stride=1, padding=1, bias=True),]
model3+=[nn.ReLU(True),]
model3+=[nn.Conv2d(256, 256, kernel_size=3, stride=1, padding=1, bias=True),]
model3+=[nn.ReLU(True),]
model3+=[nn.Conv2d(256, 256, kernel_size=3, stride=2, padding=1, bias=True),]
model3+=[nn.ReLU(True),]
model3+=[norm_layer(256),]
model4=[nn.Conv2d(256, 512, kernel_size=3, stride=1, padding=1, bias=True),]
model4+=[nn.ReLU(True),]
model4+=[nn.Conv2d(512, 512, kernel_size=3, stride=1, padding=1, bias=True),]
model4+=[nn.ReLU(True),]
model4+=[nn.Conv2d(512, 512, kernel_size=3, stride=1, padding=1, bias=True),]
model4+=[nn.ReLU(True),]
model4+=[norm_layer(512),]
model5=[nn.Conv2d(512, 512, kernel_size=3, dilation=2, stride=1, padding=2, bias=True),]
model5+=[nn.ReLU(True),]
model5+=[nn.Conv2d(512, 512, kernel_size=3, dilation=2, stride=1, padding=2, bias=True),]
model5+=[nn.ReLU(True),]
model5+=[nn.Conv2d(512, 512, kernel_size=3, dilation=2, stride=1, padding=2, bias=True),]
model5+=[nn.ReLU(True),]
model5+=[norm_layer(512),]
model6=[nn.Conv2d(512, 512, kernel_size=3, dilation=2, stride=1, padding=2, bias=True),]
model6+=[nn.ReLU(True),]
model6+=[nn.Conv2d(512, 512, kernel_size=3, dilation=2, stride=1, padding=2, bias=True),]
model6+=[nn.ReLU(True),]
model6+=[nn.Conv2d(512, 512, kernel_size=3, dilation=2, stride=1, padding=2, bias=True),]
model6+=[nn.ReLU(True),]
model6+=[norm_layer(512),]
model7=[nn.Conv2d(512, 512, kernel_size=3, stride=1, padding=1, bias=True),]
model7+=[nn.ReLU(True),]
model7+=[nn.Conv2d(512, 512, kernel_size=3, stride=1, padding=1, bias=True),]
model7+=[nn.ReLU(True),]
model7+=[nn.Conv2d(512, 512, kernel_size=3, stride=1, padding=1, bias=True),]
model7+=[nn.ReLU(True),]
model7+=[norm_layer(512),]
model8=[nn.ConvTranspose2d(512, 256, kernel_size=4, stride=2, padding=1, bias=True),]
model8+=[nn.ReLU(True),]
model8+=[nn.Conv2d(256, 256, kernel_size=3, stride=1, padding=1, bias=True),]
model8+=[nn.ReLU(True),]
model8+=[nn.Conv2d(256, 256, kernel_size=3, stride=1, padding=1, bias=True),]
model8+=[nn.ReLU(True),]
model8+=[nn.Conv2d(256, 313, kernel_size=1, stride=1, padding=0, bias=True),]
self.model1 = nn.Sequential(*model1)
self.model2 = nn.Sequential(*model2)
self.model3 = nn.Sequential(*model3)
self.model4 = nn.Sequential(*model4)
self.model5 = nn.Sequential(*model5)
self.model6 = nn.Sequential(*model6)
self.model7 = nn.Sequential(*model7)
self.model8 = nn.Sequential(*model8)
self.softmax = nn.Softmax(dim=1)
self.model_out = nn.Conv2d(313, 2, kernel_size=1, padding=0, dilation=1, stride=1, bias=False)
self.upsample4 = nn.Upsample(scale_factor=4, mode='bilinear')
def forward(self, x):
conv1_2 = self.model1(self.normalize_l(x))
conv2_2 = self.model2(conv1_2)
conv3_3 = self.model3(conv2_2)
conv4_3 = self.model4(conv3_3)
conv5_3 = self.model5(conv4_3)
conv6_3 = self.model6(conv5_3)
conv7_3 = self.model7(conv6_3)
conv8_3 = self.model8(conv7_3)
out_reg = self.model_out(self.softmax(conv8_3))
return self.upsample4(out_reg)*self.ab_norm
def model(pretrained=True):
model = model()
if(pretrained):
import torch.utils.model_zoo as model_zoo
model.load_state_dict(model_zoo.load_url('https://colorizers.s3.us-east-2.amazonaws.com/colorization_release_v2-9b330a0b.pth',map_location='cpu',check_hash=True))
return model
这里解释几个函数:
nn.BatchNorm2d
模型训练之前,需对数据做归一化处理,使其分布一致
class torch.nn.BatchNorm2d(num_features, eps=1e-05, momentum=0.1, affine=True)
- num_features: 一般输入参数为batch_sizenum_featuresheight*width,即为其中特征的数量,channel数。
- eps: 为保证数值稳定性(分母不能趋近或取0),给分母加上的值。默认为1e-5。
- momentum: 动态均值和动态方差所使用的动量,即一个用于运行过程中均值和方差的一个估计参数,默认值为0.1。
- affine: 一个布尔值,当设为true,给该层添加可学习的仿射变换参数,即给定可以学习的系数矩阵 (gamma)和 (beta)。
nn.Softmax(dim)
dim值一般有0,1,2,分别对应的就是三维数组的0,1,2。
import torch.nn as nn
m = nn.Softmax(dim=0)
n = nn.Softmax(dim=1)
k = nn.Softmax(dim=2)
input = torch.randn(2, 2, 3)
print(input)
print(m(input))
print(n(input))
print(k(input))
对dim=0时,m[0][0][0]+m[1][0][0]=1 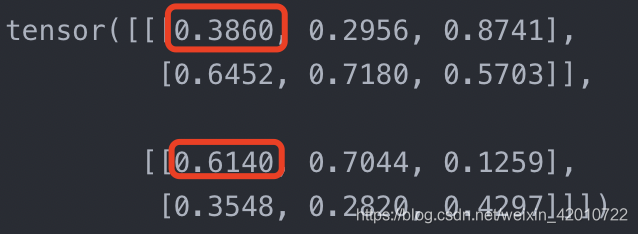 对dim=1时,n[0][1][0]+n[0][0][0]=1 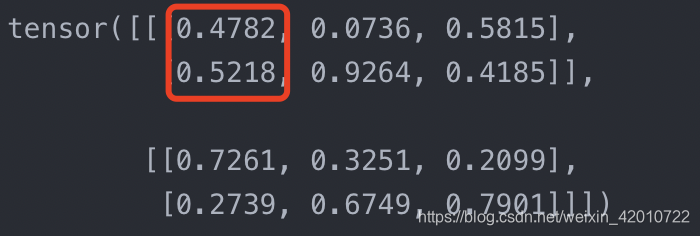 对dim=2时,k[0][0][0]+k[0][0][1]+k[0][0][2]=1 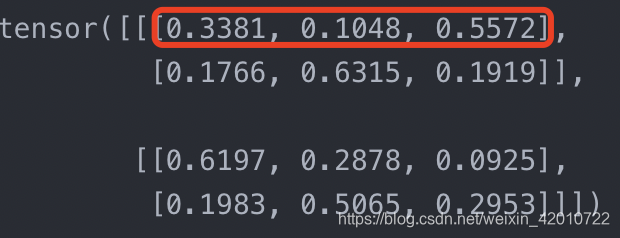
nn.Upsample
实现向上采样
Class nn.Upsample(size=None, scale_factor=None, mode='nearest', align_corners=None)
- size:根据不同的输入类型制定的输出大小。
- scale_factor:指定输出为输入的多少倍数。如果输入为tuple,其也要制定为tuple类型
- mode:可使用的上采样算法,有’nearest’, ‘linear’, ‘bilinear’, ‘bicubic’ and ‘trilinear’. 默认使用’nearest’
- align_corners:如果为True,输入的角像素将与输出张量对齐,因此将保存下来这些像素的值。仅当使用的算法为’linear’, 'bilinear’or’trilinear’时可以使用。默认设置为False
util.py
import cv2
import numpy as np
from skimage import color
import torch
import torch.nn.functional as F
from IPython import embed
def load_img(img_path):
out_np = np.asarray(cv2.imread(img_path))
if(out_np.ndim==2):
out_np = np.tile(out_np[:,:,None],3)
return out_np
def resize_img(img, HW=(256,256), resample=3):
return np.asarray(cv2.resize(img,(HW[1],HW[0])))
def preprocess_img(img_rgb_orig, HW=(256,256), resample=3):
img_rgb_rs = resize_img(img_rgb_orig, HW=HW, resample=resample)
img_lab_orig = color.rgb2lab(img_rgb_orig)
img_lab_rs = color.rgb2lab(img_rgb_rs)
img_l_orig = img_lab_orig[:,:,0]
img_l_rs = img_lab_rs[:,:,0]
tens_orig_l = torch.Tensor(img_l_orig)[None,None,:,:]
tens_rs_l = torch.Tensor(img_l_rs)[None,None,:,:]
return (tens_orig_l, tens_rs_l)
def postprocess_tens(tens_orig_l, out_ab, mode='bilinear'):
HW_orig = tens_orig_l.shape[2:]
HW = out_ab.shape[2:]
if(HW_orig[0]!=HW[0] or HW_orig[1]!=HW[1]):
out_ab_orig = F.interpolate(out_ab, size=HW_orig, mode='bilinear')
else:
out_ab_orig = out_ab
out_lab_orig = torch.cat((tens_orig_l, out_ab_orig), dim=1)
return color.lab2rgb(out_lab_orig.data.cpu().numpy()[0,...].transpose((1,2,0)))
main.py
import argparse
from model import *
from util import *
parser = argparse.ArgumentParser()
parser.add_argument('-i','--img_path', type=str, default='img/10.jpg')
parser.add_argument('-o','--save_path', type=str, default='imgout')
opt = parser.parse_args()
model = model(pretrained=True).eval()
img = load_img(opt.img_path)
(tens_l_orig, tens_l_rs) = preprocess_img(img, HW=(256,256))
img_bw = postprocess_tens(tens_l_orig, torch.cat((0*tens_l_orig,0*tens_l_orig),dim=1))
out_img_model = postprocess_tens(tens_l_orig, model(tens_l_rs))
plt.imsave('%s/img.png'%opt.save_path, out_img_model)
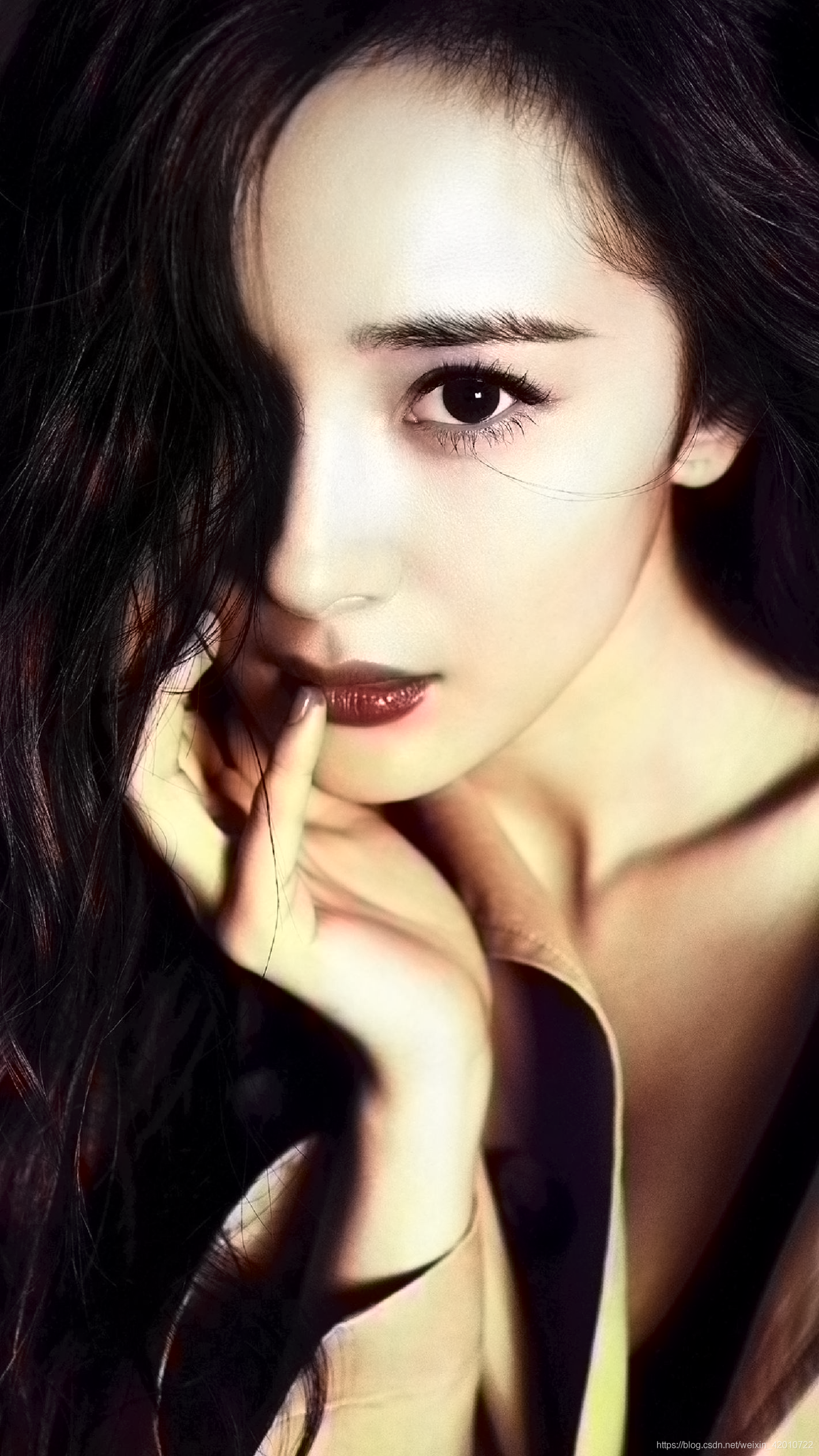
|