Backbone-ResNet
1.介绍
ResNet太耀眼了,何凯明团队在2015年在论文Deep Residual Learning for Image Recognition中提出后,至今已经有了8w+的被引数,因为是华人学者的成果,ResNet在国内宣传得很好。
到2015年,当时基于卷积的backbone有AlexNet、GoogLenet、VGG等,这些网络都有一个特点:网络层数比较少,最多十几二十层。从直觉上来讲,网络层数深有很多好处,例如,意味着更强的非线性表达能力,模型可以学习更加复杂的变换,从而可以拟合更加复杂的映射关系;深度卷积网络自然的整合了低中高不同层次的特征,特征的层次可以靠加深网络的层次来丰富。因此在构建卷积网络时,网络的深度越高,可抽取的特征层次就越丰富越抽象。
但是问题接踵而至,当网络变 深的时候,网络会出现两个问题。第一个是神经网络退化。作者在Introduction部分提出,When deeper networks are able to start converging, a degradation problem has been exposed: with the network depth increasing, accuracy gets saturated (which might be unsurprising) and then degrades rapidly. Unexpectedly, such degradation is not caused by overfitting, and adding more layers to a suitably deep model leads to higher training error, as reported in and thoroughly verified by our experiments. Fig. 1 shows a typical example. 简单来说,当网络深度太大时,模型的效果反而会变差。第二个是会出现梯度消失和梯度爆炸现象。
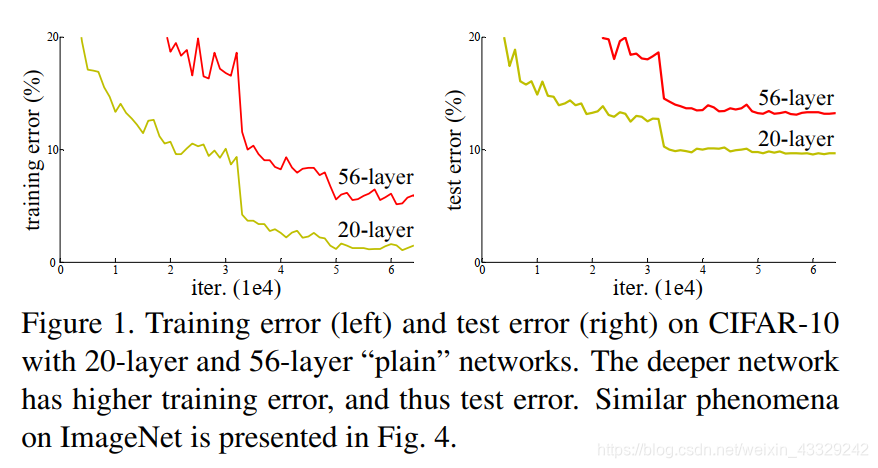
作者解决了这两个问题!新的结构residual模块解决了神经网络退化的问题,Batch Normalization解决了梯度爆炸的问题。如此一来,作者将网络层数直接推到了100多层,这是第一次构造如此之深的神经网络。下面来详细说说ResNet的结构。
2.网络结构
下图就是residual模块了,整个网络大多数是由这样的residual搭建起来的,现在介绍一下这个模块。residual最引人注目的就是从首端直接连接到尾端的那条线,我称之为辅路(图中最右侧)。左侧有三个卷积层的是主路。网络的思想是,将主路的输处和辅路的输出加在一起,作为整个residual模块的输出。
这里给出感性的认识。当网络过深时,梯度下降很难保证网络最前面的参数得到有效地更新,如果在网络的脊背上牵一根线,那么在对前面的网络参数求导的时候,导数为,经过主路的导数,加上经过辅路的导数。而经过辅路的导数相当于无视了后面的许多层网络,这样就相当于更新一个比较浅的网络了。默认来说,residual模块不改变输出入和输出的长和宽。
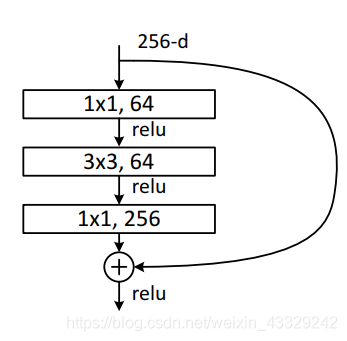
下面这张图是论文中提出的各种深度的网络表格。我这里选用50-layer的ResNet来做说明,看下面过程的时候,可以对照表格中50-layer那一列。
- 输入图像的尺寸是3*224*224,经过conv1卷积层(7*7,有64个卷积层,stride为2),输出尺寸为64*112*112;
- 经过conv2_x中的最大池化层,输出尺寸为64*56*56;
- 现在到了conv2_x中第一个residual模块。考虑主路,第一个卷积层(卷积核大小为1*1,卷积数目为64且stride为1的卷积层)的输出为64*56*56,第二个卷积层输出为64*56*56,第三层卷积层输出为256*56*56。考虑辅路,这里用一个卷积层(卷积核大小为1*1,卷积数目为256且stride为1的卷积层),输出为256*56*56。最后再将这两条路的尺寸为256*56*56的输出相加,输出。
- 现在到了conv2_x中第二个residual模块。考虑主路,第一个卷积层(卷积核大小为1*1,卷积数目为64且stride为1的卷积层)的输出为64*56*56,第二个卷积层输出为64*56*56,第三层卷积层输出为256*56*56。考虑辅路,直接输出原始的值,尺寸为256*56*56。最后再将这两条路的尺寸为256*56*56的输出相加并输出。
- 现在到了conv2_x中第三个residual模块。与conv2_x中第三个residual模块一模一样,输出尺寸为256*56*56。
- 现在到了conv3_x中第一个residual模块。注意,这个residual要做特殊处理,改变输出和输出的长与宽。为了使conv2_x中下面两个residual模块不改变尺寸,同时又要保证整个conv2_x的输出尺寸为512*28*28,所以conv2_x中第一个residual模块要将256*56*56转变为512*28*28。考虑主路,第一个卷积层(卷积核大小为1*1,卷积数目为128且stride为1的卷积层)的输出为128*56*56,第二个卷积层将stride从1改为3,这样尺寸会减半,输出为128*28*28,第三层卷积层输出为512*28*28。考虑辅路,采用卷积层(卷积核大小为1*1,卷积数目为512且stride为2的卷积层),这样得到的输出尺寸也是512*28*28。最后再将这两条路的尺寸为512*28*28的输出相加,输出。
- 现在到了conv3_x中第二个residual模块。和第4步一样,主通道和辅助通道输出都是512*28*28。
- conv3_x中第三个residual模块,和conv3_x中第二个residual模块一样。
- …
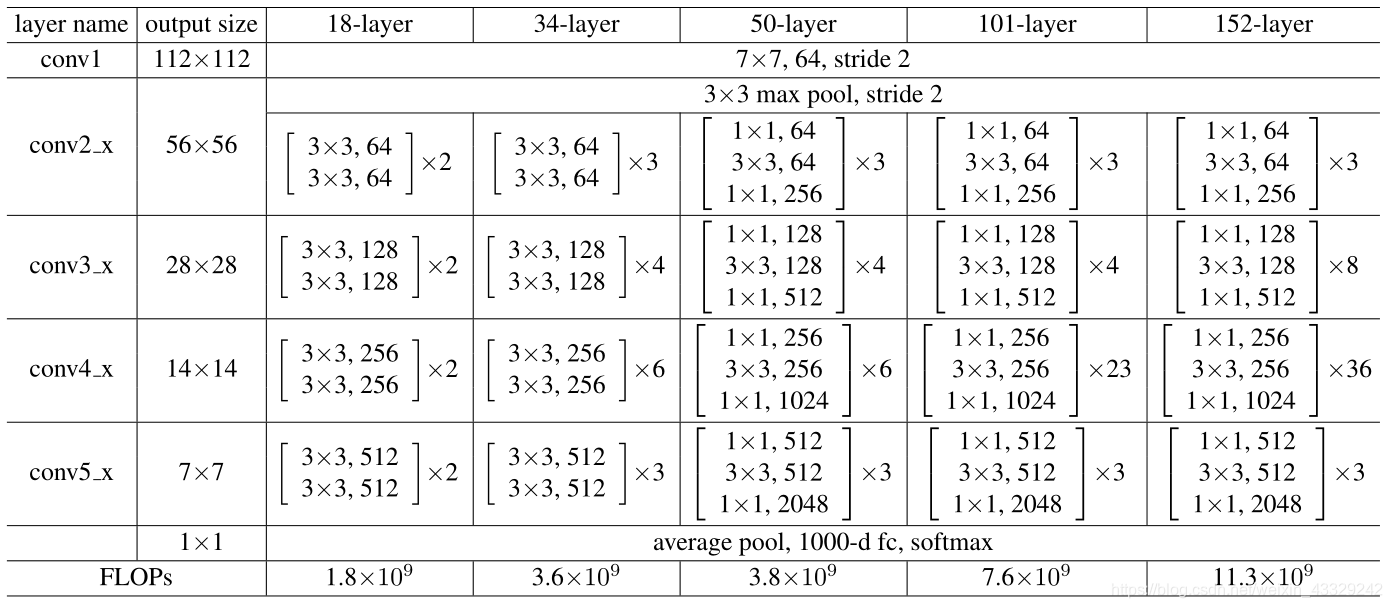
3.实现
下面是ResNet of 50 layers 的pytorch实现。
import torch
import torch.nn as nn
class ResNet50(nn.Module):
def __init__(self):
super(ResNet50, self).__init__()
self.conv1 = nn.Conv2d(in_channels=3, out_channels=64, kernel_size=7, stride=2, padding=3, bias=False)
self.bn1 = nn.BatchNorm2d(64)
self.relu = nn.ReLU(inplace=True)
self.maxpool = nn.MaxPool2d(kernel_size=3, stride=2, padding=1)
self.conv2_x_1 = BasicBlock(in_channels=64, out_channels=64, downsample=False)
self.conv2_x_2 = BasicBlock(in_channels=256, out_channels=64, downsample=False)
self.conv2_x_3 = BasicBlock(in_channels=256, out_channels=64, downsample=False)
self.conv3_x_pre = BasicBlock(in_channels=256, out_channels=128, downsample=True)
self.conv3_x_2 = BasicBlock(in_channels=512, out_channels=128, downsample=False)
self.conv3_x_3 = BasicBlock(in_channels=512, out_channels=128, downsample=False)
self.conv4_x_pre = BasicBlock(in_channels=512, out_channels=256, downsample=True)
self.conv4_x_2 = BasicBlock(in_channels=1024, out_channels=256, downsample=False)
self.conv4_x_3 = BasicBlock(in_channels=1024, out_channels=256, downsample=False)
self.conv5_x_pre = BasicBlock(in_channels=1024, out_channels=512, downsample=True)
self.conv5_x_2 = BasicBlock(in_channels=2048, out_channels=512, downsample=False)
self.conv5_x_3 = BasicBlock(in_channels=2048, out_channels=512, downsample=False)
self.avgpool = nn.AvgPool2d(kernel_size=7, stride=1)
self.classifier = nn.Sequential(
nn.Linear(2048, 1000),
nn.Softmax(dim=1),
)
def forward(self, x):
x = self.conv1(x)
x = self.bn1(x)
x = self.relu(x)
x = self.maxpool(x)
x = self.conv2_x_1(x)
x = self.conv2_x_2(x)
x = self.conv2_x_3(x)
x = self.conv3_x_pre(x)
x = self.conv3_x_2(x)
x = self.conv3_x_3(x)
x = self.conv4_x_pre(x)
x = self.conv4_x_2(x)
x = self.conv4_x_3(x)
x = self.conv5_x_pre(x)
x = self.conv5_x_2(x)
x = self.conv5_x_3(x)
x = self.avgpool(x)
x = torch.flatten(x, 1)
x = self.classifier(x)
return x
class BasicBlock(nn.Module):
def __init__(self, in_channels, out_channels, downsample=False):
super(BasicBlock, self).__init__()
self.downsample = downsample
extension = 4
self.cov1 = nn.Conv2d(in_channels=in_channels, out_channels=out_channels, kernel_size=1, stride=1, bias=False)
self.bn1 = nn.BatchNorm2d(out_channels)
self.cov2 = nn.Conv2d(in_channels=out_channels, out_channels=out_channels, kernel_size=3, stride=1, padding=1, bias=False)
self.cov2_downsample = nn.Conv2d(in_channels=out_channels, out_channels=out_channels, kernel_size=3, stride=2,
padding=1, bias=False)
self.bn2 = nn.BatchNorm2d(out_channels)
self.cov3 = nn.Conv2d(in_channels=out_channels, out_channels=4 * out_channels, kernel_size=1, stride=1, bias=False)
self.bn3 = nn.BatchNorm2d(4 * out_channels)
self.side = nn.Conv2d(in_channels=in_channels, out_channels=4 * out_channels, kernel_size=1, stride=1,
bias=False)
self.side_downside = nn.Conv2d(in_channels=in_channels, out_channels=4 * out_channels, kernel_size=1, stride=2, bias=False)
self.bn3 = nn.BatchNorm2d(4 * out_channels)
self.relu = nn.ReLU(inplace=True)
def forward(self, x):
identity = x
x = self.cov1(x)
x = self.bn1(x)
x = self.relu(x)
if self.downsample:
x = self.cov2_downsample(x)
else:
x = self.cov2(x)
x = self.bn2(x)
x = self.relu(x)
x = self.cov3(x)
x = self.bn3(x)
x = self.relu(x)
if self.downsample:
identity = self.side_downside(identity)
else:
identity = self.side(identity)
x = self.bn3(x)
return x + identity
if __name__ == '__main__':
net = ResNet50()
x = torch.rand(1, 3, 224, 224)
out = net.forward(x)
print(out.size())
4.注意
- residual模块最后是相加运算(而不是拼接),需要保证代码中的x和identity尺寸完全相同。
- mobileNet,ResNet等等网络,只能处理图片分辨率是正方形224*224的吗?比如128*135输入图像可以处理吗?答:如果不想reszie,空间金字塔可以有效解决输入尺寸问题。我还没学,以后遇到了再补上。
- 网络在conv2_x、conv3_x、conv4_x、conv5_x中的第一个residual模块都需要调整网络的尺寸。
- 有BN层了,则不在需要卷积的偏置。因为有偏置和没有偏置,BN层的输出是一样的。不信你可以算一算。
|