是在检测图片的基础上进行加工的 详情可看opencv图片倾斜度检(一)对图片进行检测 打开摄像头进行实时检测矩形轮廓,实时画出坐标轴坐标点和倾斜度,并且具有保存图片和利用plot单纯画出矩形的功能
直接上全部代码 注释已经比较详细了 如下:
from matplotlib.cm import register_cmap
import matplotlib.pyplot as plt
import cv2
import numpy as np
import imutils
import time
from scipy.spatial import distance as dist
def read_usb_capture():
cap = cv2.VideoCapture(1, cv2.CAP_DSHOW)
cv2.namedWindow('video', cv2.WINDOW_NORMAL)
while (cap.isOpened()):
ret, frame = cap.read()
lunkuo(frame)
cv2.imshow('video', frame)
k = cv2.waitKey(1) & 0xFF
if k == ord('s'):
cv2.imwrite("1.jpg", frame)
img = cv2.imread('1.jpg')
cv2.imshow('img',img)
if k == ord('q'):
break
cap.release()
cv2.destroyAllWindows()
def midpoint(ptA, ptB):
return ((ptA[0] + ptB[0]) * 0.5, (ptA[1] + ptB[1]) * 0.5)
def show(img):
cv2.imshow('img', img)
cv2.waitKey(0)
cv2.destroyAllWindows()
def lunkuo(img):
start = time.time()
img1_ = cv2.cvtColor(img, cv2.COLOR_BGR2GRAY)
img_ = cv2.GaussianBlur(img1_, (5, 5), 0)
img__ = cv2.Canny(img_, 75, 200)
img__ = cv2.dilate(img__, None, iterations=1)
img__ = cv2.erode(img__, None, iterations=1)
cnts = cv2.findContours(img__.copy(), cv2.RETR_LIST, cv2.CHAIN_APPROX_SIMPLE)
cnts = cnts[1] if imutils.is_cv3() else cnts[0]
cnts = sorted(cnts, key=cv2.contourArea, reverse=True)[:]
global screenCnt,box,rangle
box = 0
rangle = 0
cv2.line(img, (595, 45), (595, 435),
(255, 0, 255), 1)
cv2.line(img, (45, 435), (595, 435),
(255, 0, 255), 1)
cv2.line(img, (45, 45), (45, 435),
(255, 0, 255), 1)
cv2.line(img, (45, 45), (595, 45),
(255, 0, 255), 1)
cv2.line(img, (45, 45), (595, 45),
(255, 0, 255), 1)
cv2.circle(img, (45, 45), 2, (255, 0, 0), -1)
cv2.putText(img, "(0,0)",
(45, 435), cv2.FONT_HERSHEY_SIMPLEX,
0.5, (0, 244, 245), 2)
for c in cnts:
peri = cv2.arcLength(c, True)
approx = cv2.approxPolyDP(c, 0.02 * peri, True)
if len(approx) == 4:
screenCnt = approx
rangle = cv2.minAreaRect(screenCnt)[2]
box = cv2.cv.BoxPoints(cv2.minAreaRect(screenCnt)) if imutils.is_cv2() else cv2.boxPoints(
cv2.minAreaRect(screenCnt))
cv2.drawContours(img, [box.astype("int")], -1, (0, 255, 0), 2)
box = np.array(box, dtype="int")
(tl, tr, br, bl) = box
(tltrX, tltrY) = midpoint(tl, tr)
(blbrX, blbrY) = midpoint(bl, br)
(tlblX, tlblY) = midpoint(tl, bl)
(trbrX, trbrY) = midpoint(tr, br)
dA = dist.euclidean((tltrX, tltrY), (blbrX, blbrY))
dB = dist.euclidean((tlblX, tlblY), (trbrX, trbrY))
pixelsPerMetric = 35.15
if pixelsPerMetric != 0:
dimA = dA / pixelsPerMetric
dimB = dB / pixelsPerMetric
cv2.putText(img, "{:.1f}cm".format(dimB),
(int(tltrX - 15), int(tltrY - 10)), cv2.FONT_HERSHEY_SIMPLEX,
0.65, (225, 190, 0), 2)
cv2.putText(img, "{:.1f}cm".format(dimA),
(int(trbrX + 10), int(trbrY)), cv2.FONT_HERSHEY_SIMPLEX,
0.65, (225, 190, 0), 2)
cv2.putText(img, "r:{:.1f}".format(90 - rangle),
(int(tltrX + 20), int(tltrY + 20)), cv2.FONT_HERSHEY_SIMPLEX,
0.65, (225, 190, 0), 2)
cv2.line(img, (int(bl[0]), int(bl[1])), (int(bl[0]), 435),
(0, 244, 245), 1)
cv2.putText(img, "({},{})".format(bl[0], 640 - bl[1]),
(int(bl[0] - 20), 415), cv2.FONT_HERSHEY_SIMPLEX,
0.4, (225, 190, 0), 1)
cv2.line(img, (int(br[0]), int(br[1])), (int(br[0]), 435),
(0, 244, 245), 1)
cv2.putText(img, "({},{})".format(br[0], 640 - br[1]),
(int(br[0]), 435), cv2.FONT_HERSHEY_SIMPLEX,
0.4, (225, 190, 0), 1)
cv2.line(img, (int(bl[0]), int(bl[1])), (int(595), int(bl[1])),
(0, 244, 245), 1)
end = time.time()
print("轮廓检测所用时间:{:.3f}ms".format((end - start) * 1000))
return img
read_usb_capture()
代码结果如下: 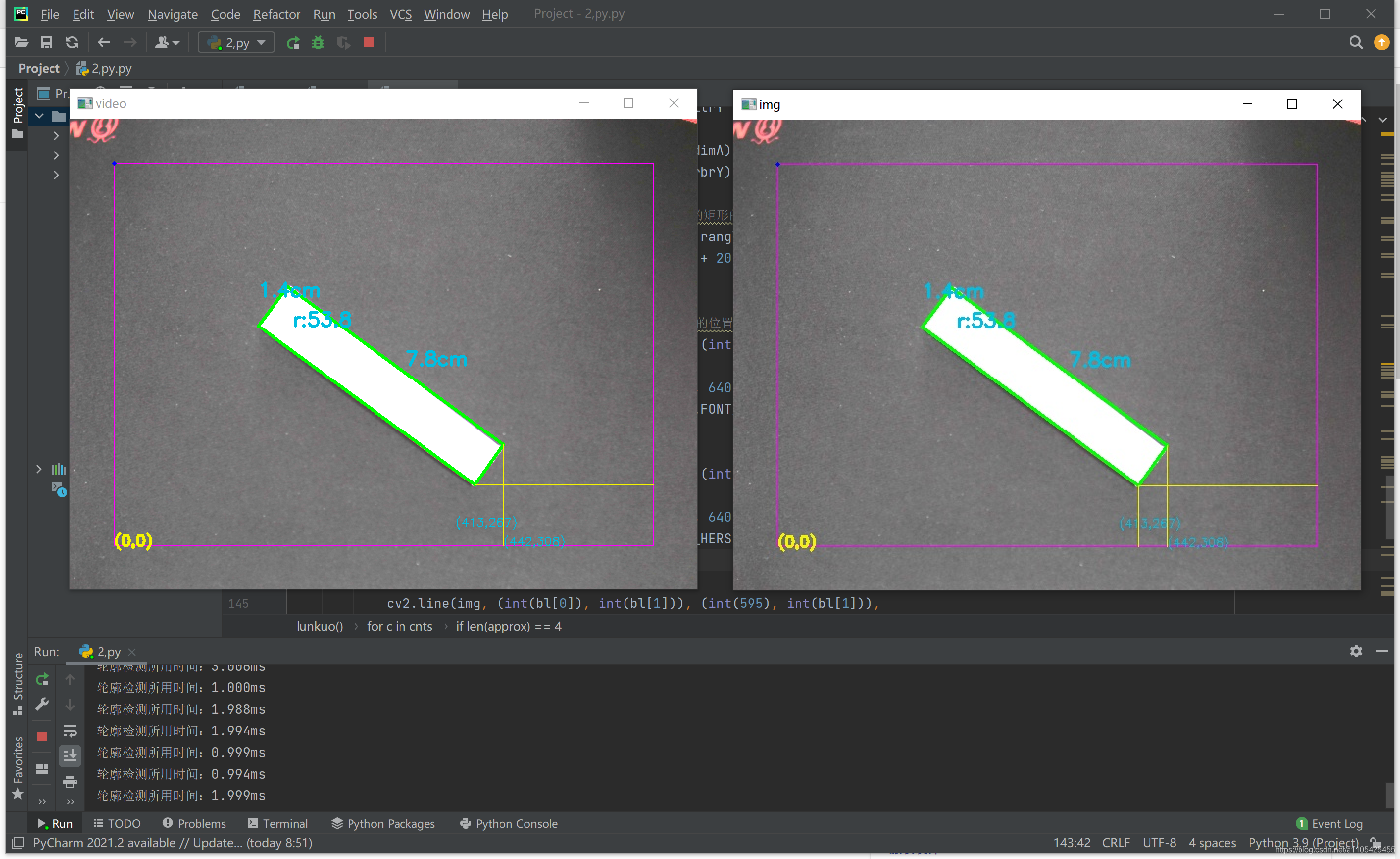 新增一个按下d键打开轮廓的坐标图功能 将画图部分整合成函数,使用全局变量作为触发标志 代码如下:
from matplotlib.cm import register_cmap
import matplotlib.pyplot as plt
import cv2
import numpy as np
import imutils
import time
from scipy.spatial import distance as dist
from PIL import Image
global d
d = 0
def read_usb_capture():
cap = cv2.VideoCapture(1, cv2.CAP_DSHOW)
cv2.namedWindow('video', cv2.WINDOW_AUTOSIZE)
cv2.resizeWindow("video", 640, 480)
while (cap.isOpened()):
ret, frame = cap.read()
lunkuo(frame)
cv2.imshow('video', frame)
k = cv2.waitKey(1) & 0xFF
if k == ord('s'):
cv2.imwrite("1.jpg", frame)
img = cv2.imread('1.jpg')
cv2.imshow('img',img)
if k == ord('q'):
break
if k == ord('d'):
global d
d = 1
cap.release()
cv2.destroyAllWindows()
def plot(tl,tr,bl,br):
x = [tl[0], tr[0], br[0], bl[0], tl[0]]
y = [640 - tl[1], 640 - tr[1], 640 - br[1], 640 - bl[1], 640 - tl[1]]
plt.plot(x, y)
plt.axhline(y=min(y), c="r", ls="--", lw=2)
plt.title("rangel = {}".format(90 - rangle))
plt.axis("equal")
for a, b in zip(x, y):
plt.text(a, b, (a, b), ha='center', va='bottom', fontsize=10)
plt.savefig('zuobiao.png')
plt.clf()
plt.cla()
fp = open('zuobiao.png', 'rb')
img = Image.open(fp)
img.show()
def midpoint(ptA, ptB):
return ((ptA[0] + ptB[0]) * 0.5, (ptA[1] + ptB[1]) * 0.5)
def lunkuo(img):
start = time.time()
img1_ = cv2.cvtColor(img, cv2.COLOR_BGR2GRAY)
img_ = cv2.GaussianBlur(img1_, (5, 5), 0)
img__ = cv2.Canny(img_, 75, 200)
img__ = cv2.dilate(img__, None, iterations=1)
img__ = cv2.erode(img__, None, iterations=1)
cnts = cv2.findContours(img__.copy(), cv2.RETR_LIST, cv2.CHAIN_APPROX_SIMPLE)
cnts = cnts[1] if imutils.is_cv3() else cnts[0]
cnts = sorted(cnts, key=cv2.contourArea, reverse=True)[:]
global screenCnt,box,rangle
box = 0
rangle = 0
cv2.line(img, (595, 45), (595, 435),
(255, 0, 255), 1)
cv2.line(img, (45, 435), (595, 435),
(255, 0, 255), 1)
cv2.line(img, (45, 45), (45, 435),
(255, 0, 255), 1)
cv2.line(img, (45, 45), (595, 45),
(255, 0, 255), 1)
cv2.line(img, (45, 45), (595, 45),
(255, 0, 255), 1)
cv2.circle(img, (45, 45), 2, (255, 0, 0), -1)
cv2.putText(img, "(0,0)",
(45, 435), cv2.FONT_HERSHEY_SIMPLEX,
0.5, (0, 244, 245), 2)
for c in cnts:
peri = cv2.arcLength(c, True)
approx = cv2.approxPolyDP(c, 0.02 * peri, True)
if len(approx) == 4:
screenCnt = approx
rangle = cv2.minAreaRect(screenCnt)[2]
box = cv2.cv.BoxPoints(cv2.minAreaRect(screenCnt)) if imutils.is_cv2() else cv2.boxPoints(
cv2.minAreaRect(screenCnt))
cv2.drawContours(img, [box.astype("int")], -1, (0, 255, 0), 2)
box = np.array(box, dtype="int")
(tl, tr, br, bl) = box
(tltrX, tltrY) = midpoint(tl, tr)
(blbrX, blbrY) = midpoint(bl, br)
(tlblX, tlblY) = midpoint(tl, bl)
(trbrX, trbrY) = midpoint(tr, br)
dA = dist.euclidean((tltrX, tltrY), (blbrX, blbrY))
dB = dist.euclidean((tlblX, tlblY), (trbrX, trbrY))
pixelsPerMetric = 35.15
if pixelsPerMetric != 0:
dimA = dA / pixelsPerMetric
dimB = dB / pixelsPerMetric
cv2.putText(img, "{:.1f}cm".format(dimB),
(int(tltrX - 15), int(tltrY - 10)), cv2.FONT_HERSHEY_SIMPLEX,
0.65, (225, 190, 0), 2)
cv2.putText(img, "{:.1f}cm".format(dimA),
(int(trbrX + 10), int(trbrY)), cv2.FONT_HERSHEY_SIMPLEX,
0.65, (225, 190, 0), 2)
cv2.putText(img, "r:{:.1f}".format(90 - rangle),
(int(tltrX + 20), int(tltrY + 20)), cv2.FONT_HERSHEY_SIMPLEX,
0.65, (225, 190, 0), 2)
cv2.line(img, (int(bl[0]), int(bl[1])), (int(bl[0]), 435),
(0, 244, 245), 1)
cv2.putText(img, "({},{})".format(bl[0], 640 - bl[1]),
(int(bl[0] - 20), 415), cv2.FONT_HERSHEY_SIMPLEX,
0.4, (225, 190, 0), 1)
cv2.line(img, (int(br[0]), int(br[1])), (int(br[0]), 435),
(0, 244, 245), 1)
cv2.putText(img, "({},{})".format(br[0], 640 - br[1]),
(int(br[0]), 435), cv2.FONT_HERSHEY_SIMPLEX,
0.4, (225, 190, 0), 1)
cv2.line(img, (int(bl[0]), int(bl[1])), (int(595), int(bl[1])),
(0, 244, 245), 1)
end = time.time()
print("轮廓检测所用时间:{:.3f}ms".format((end - start) * 1000))
global d
if d == 1:
plot(tl, tr, bl, br)
d = 0
return img
read_usb_capture()
按下 d 结果如下: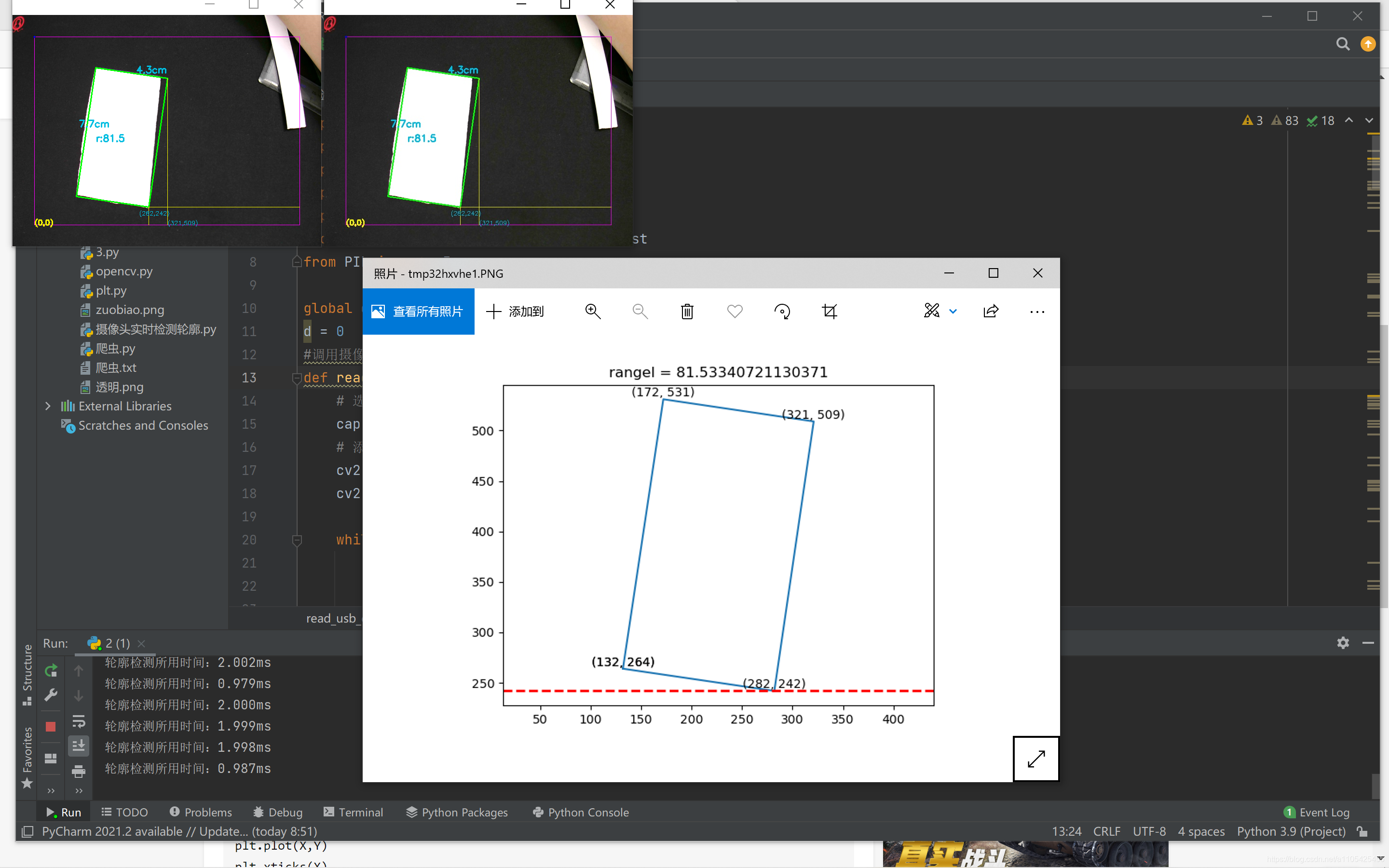
|