BYOL 论文阅读
介绍
Bootstrap Your Own Latent(BYOL),是一种自监督学习方法,主要结构由两层神经网络构成Online和Target,两层网络相互学习。
-
为什么需要自监督学习
- 随着模型不断复杂化,训练过程困难,需要大量的带标签的数据
-
创新点
- 不同于SimCLR,MOCO等对比学习方法,BYOL没有使用负样本
方法与实现
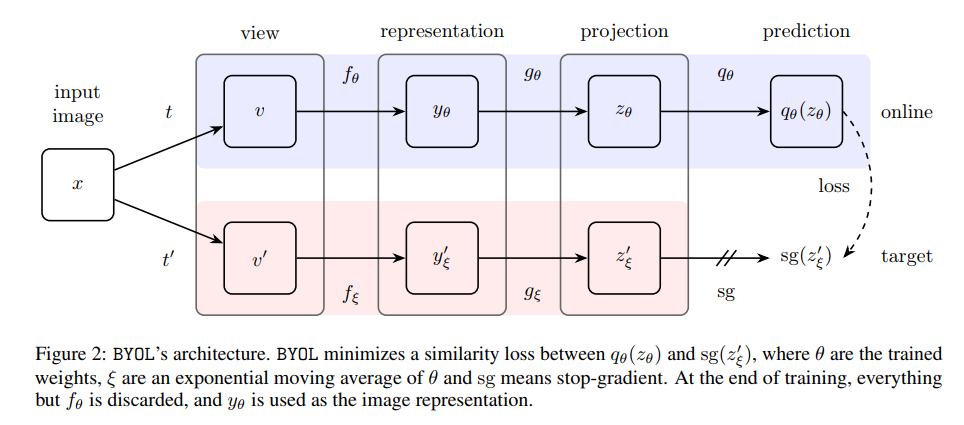
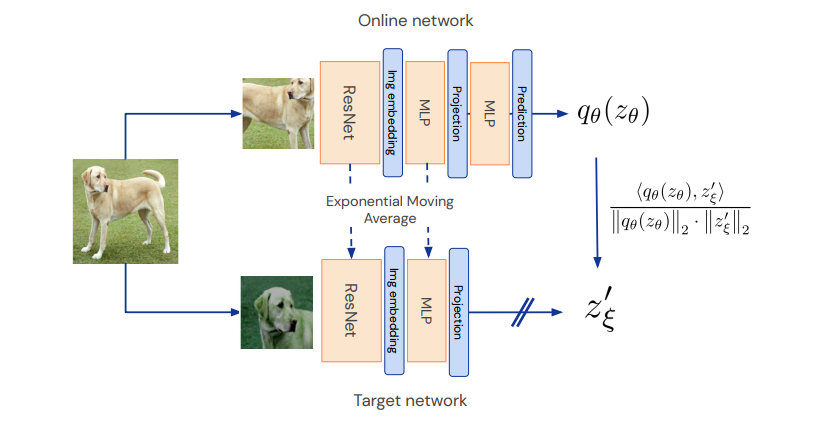
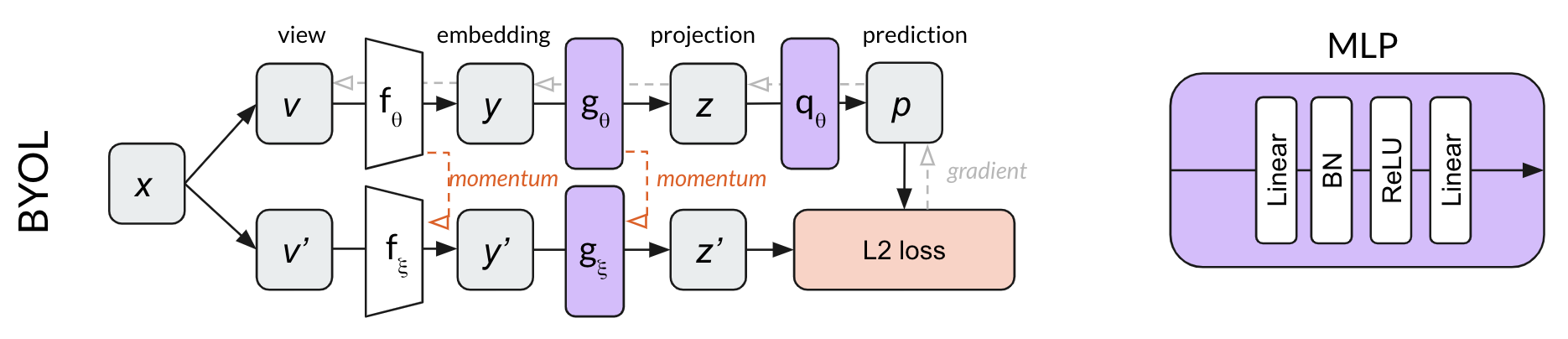
-
结构
- 两个基本框架相同的network,online和target,但参数不同
-
f
f
f??为一个encoder结构,论文中使用ResNet,将image转换为embedding
-
g
g
g???为一个MLP结构,Linear+BN+ReLU+Linear,将img embedding 投影到新纬度
-
q
q
q????????为一个MLP结构,将online的vector
z
z
z????,向target的vector
z
′
z^{'}
z′??靠近?
-
s
g
sg
sg??,阻止target network的梯度反向传播,故target采用momentum的形式用online的参数更新
-
基本训练流程
- input image 通过两种不同的图像增强方式,生成两张不同的image,
v
v
v?和
v
′
v^{'}
v′?
- 将
v
v
v和
v
′
v^{'}
v′?分别传进两个网络中
- 使online不断逼近target,target缓慢更新,向online靠拢
- 然后将
v
v
v和
v
′
v^{'}
v′?调换,再次传进两个网络中
- 最终得到一个好的encoder
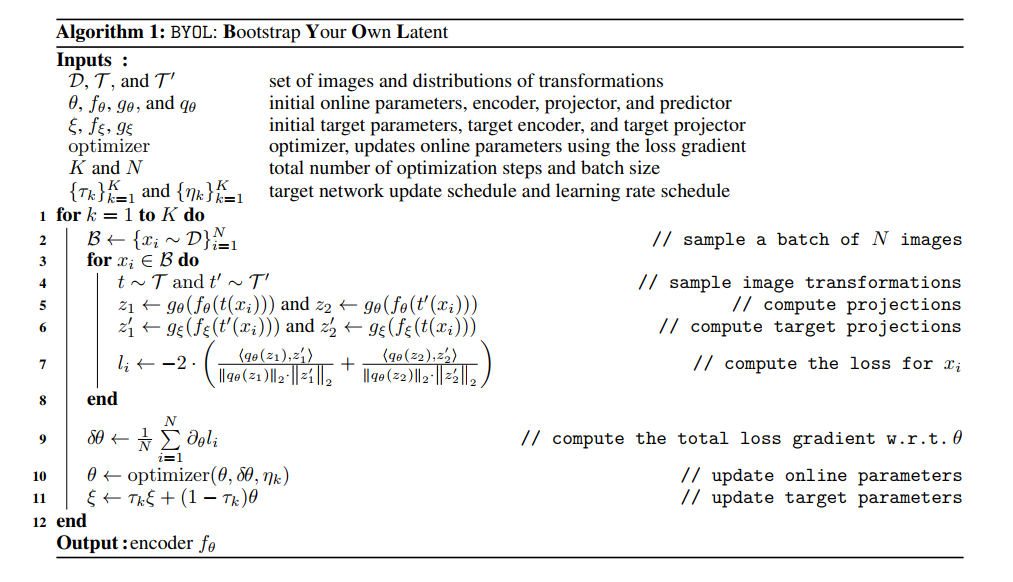
LOSS


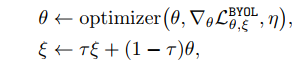
缺少负样本,为什么可以避免坍塌
target采用momentum的形式更新,速度缓慢
实验结果
Linear evaluation on ImageNet
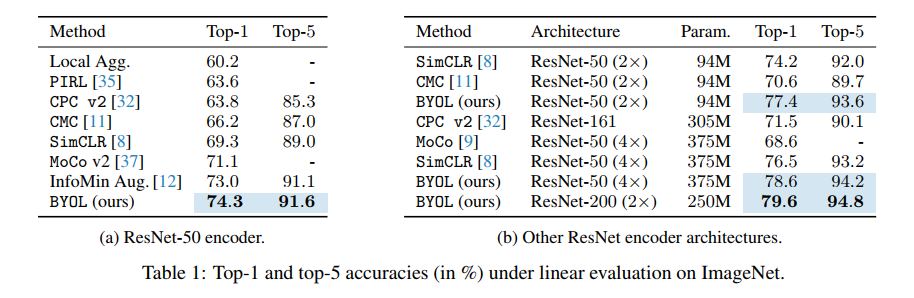
Semi-supervised training on ImageNet
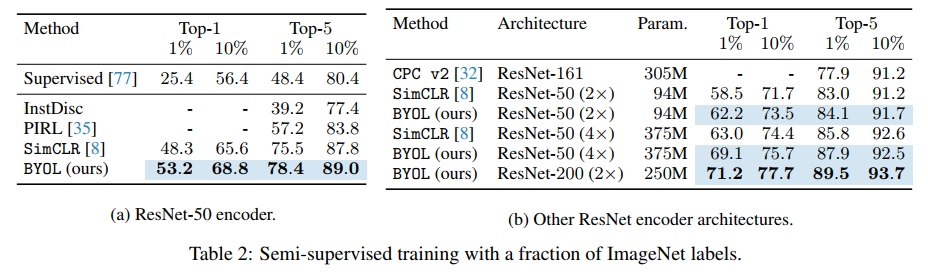
|