箱线图(Box-plot)在高光谱图像处理中的应用
欢迎学习交流! 个人网站:https://zephyrhours.github.io/ 邮箱:zengf.hou@bit.edu.cn
前言
在高光谱异常探测、目标探测和变化检测中,除了使用ROC曲线做定性评价外,箱线图也是被广泛的使用。在很多文章中都可以看到它的身影,箱线图来可以有效地评价算法对背景的抑制和对目标的分离情况,下面分享笔者基于Matlab编写的 箱线图(背景分离性分析图) 给搞科研的各位朋友,希望对您的工作有所帮助!如果您使用该代码,或这些代码对您有所帮助,请添加文章引用!
一、箱线图
箱线图(Box-plot)又称为盒须图、盒式图或箱形图,是一种用作显示一组数据分散情况资料的统计图。因形状如箱子而得名。在各种领域也经常被使用。它主要用于反映原始数据分布的特征,还可以进行多组数据分布特征的比 较。箱线图的绘制方法是:先找出一组数据的上边缘、下边缘、中位数和两个四分位数;然后, 连接两个四分位数画出箱体;再将上边缘和下边缘与箱体相连接,中位数在箱体中间,具体如下: 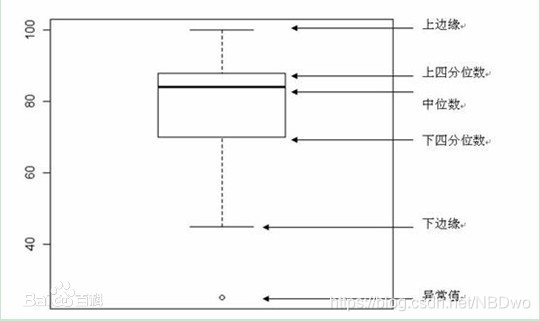
二、箱线图在高光谱影像中的应用
下面是箱线图在高光谱影像异常探测中的一个应用实例,在文章中,我们又称箱线图(Box-plot)为背景异常分离性分析,或者统计性分离分析(Statistical separability analysis),具体如下图所示: 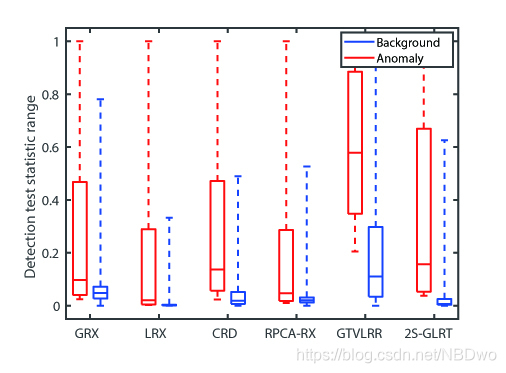 在图中可以看到,不同的检测算法表现不同,其中该图中我们采用蓝色和红色来分别表示背景地物和异常地物,箱体的取值范围,我们截取原始数据的10%~90%进行显示;蓝色箱子和红色箱子的高低,表示不同算法对背景和异常的抑制情况(背景和异常的取值)。在高光谱异常检测或者目标探测中,蓝色箱子越低,背景就会被抑制的越严重;红色箱子跟蓝色箱子之间的距离表示算法对背景和异常的分离情况,间隔越大,就越有利于目标或异常的分离。也就说明算法的分离性比较好。如图所示,LRX算法表现出来了对背景的抑制情况很好,但是蓝色箱子被抑制的同时,红色箱子跟蓝色箱子的高度差很小,也就是说该算法虽然可以有效的抑制背景,但是分离性不好,不能很好的区分背景和异常。而在GTVRR和2S-GLRT中则表现出来了比较好的分离情况,但是GTVLRR对背景的抑制没有2S-GLRT算法好,因此综上比较可知,这些算法中2S-GLRT 体现出来了比较好的背景异常分离性。具体描述请参考下面的文献。
三、相关参考文献
关于箱线图在高光谱异常探测、变化检测和目标探测中的应用以及具体描述请参考下面文献!
版权所有,如果该代码对您有所帮助,使用时请注明出处,并引用下面文献!
- Liu, J., Hou, Z., Li, W., Tao, R., Orlando, D. and Li, H., 2021. Multipixel Anomaly Detection With Unknown Patterns for Hyperspectral Imagery. IEEE Transactions on Neural Networks and Learning Systems.
- Hou, Z., Wei, L., Tao, R. and SHI, W., Collaborative Representation with Background Purification and Saliency Weight for Hyperspectral Anomaly Detection. SCIENCE CHINA Information Sciences.
- Hou, Z., Li, W., Li, L., Tao, R. and Du, Q., 2021. Hyperspectral Change Detection Based on Multiple Morphological Profiles. IEEE Transactions on Geoscience and Remote Sensing.
- Hou, Z., Li, W., Tao, R. and Du, Q., 2021. Three-Order Tucker Decomposition and Reconstruction Detector for Unsupervised Hyperspectral Change Detection. IEEE Journal of Selected Topics in Applied Earth Observations and Remote Sensing, 14, pp.6194-6205.
- Zhao, X., Hou, Z., Wu, X., Li, W., Ma, P. and Tao, R., 2021. Hyperspectral target detection based on transform domain adaptive constrained energy minimization. International Journal of Applied Earth Observation and Geoinformation, 103, p.102461.
BibTex 格式:
@article{liu2021multipixel,
title={Multipixel Anomaly Detection With Unknown Patterns for Hyperspectral Imagery},
author={Liu, Jun and Hou, Zengfu and Li, Wei and Tao, Ran and Orlando, Danilo and Li, Hongbin},
journal={IEEE Transactions on Neural Networks and Learning Systems},
year={2021},
publisher={IEEE}
}
@article{houcollaborative,
title={Collaborative Representation with Background Purification and Saliency Weight for Hyperspectral Anomaly Detection},
author={Hou, Zengfu and Wei, Li and Tao, Ran and SHI, Weihua},
journal={SCIENCE CHINA Information Sciences},
publisher={Science China Press}
}
@ARTICLE{9469924,
author={Hou, Zengfu and Li, Wei and Li, Lu and Tao, Ran and Du, Qian},
journal={IEEE Transactions on Geoscience and Remote Sensing},
title={Hyperspectral Change Detection Based on Multiple Morphological Profiles},
year={2021},
volume={},
number={},
pages={1-12},
doi={10.1109/TGRS.2021.3090802}}
@article{hou2021three,
title={Three-Order Tucker Decomposition and Reconstruction Detector for Unsupervised Hyperspectral Change Detection},
author={Hou, Zengfu and Li, Wei and Tao, Ran and Du, Qian},
journal={IEEE Journal of Selected Topics in Applied Earth Observations and Remote Sensing},
volume={14},
pages={6194--6205},
year={2021},
publisher={IEEE}
}
@article{zhao2021hyperspectral,
title={Hyperspectral target detection based on transform domain adaptive constrained energy minimization},
author={Zhao, Xiaobin and Hou, Zengfu and Wu, Xin and Li, Wei and Ma, Pengge and Tao, Ran},
journal={International Journal of Applied Earth Observation and Geoinformation},
volume={103},
pages={102461},
year={2021},
publisher={Elsevier}
}
四、具体代码
具体代码和相关测试数据请从下面链接下载!
- Github Repository
- 百度网盘【提取码:v006】
注意: 如果您有任何疑问可以随时邮件进行交流。初次联系请注明详细信息,包括您的:姓名,高校,导师,研究方向等!
|