参数类型
- 一般参数:模型通过最小化损失函数自动求解的参数
- 超参数:不能通过模型对数据进行学习而求解的参数,比如神经网络的层数、正则系数的alpha值等
参数搜索
- 超参数的搜索:提前设置好参数可以选择的后选择,然后根据不同参数组合对于模型泛化能力的贡献
,选取最佳的超参数组合
参数搜索的方法
GridSearchCV,基于交叉验证的网格搜索法:将要搜索的参数候选值输入搜索器内,搜索器遍历每一种参数组合,使用交叉验证法对比每种参数组合下模型的表现,返回表现最好模型的参数值
- 优点:自动调参,参数准确性高
- 缺点:需要耗费巨大的算力和计算时间(如:搜索100颗树的随机森林模型的两种参数各三个候选值,选择k等于10的交叉验证,则需要训练验证9000颗决策树才能返回最佳参数)
RandomizedSearchCV,基于交叉验证的随机搜索法:基本原理与GridSearchCV一致,但为了提高搜索效率,搜索器会从参数组合中随机搜索一些参数进行训练和验证,返回其中表现最好的参数值
- 优点:运行效率高,适合大数据量样本
- 缺点:参数的准确性有所牺牲
sklearn实现
import pandas as pd
import matplotlib.pyplot as plt
df = pd.read_excel('realestate_sample_preprocessed.xlsx')
def age_percent(row):
if row['nightpop'] == 0:
return 0
else:
return row['night20-39']/row['nightpop']
df['per_a20_39'] = df.apply(age_percent,axis=1)
df = df.drop(columns=['nightpop','night20-39'])
price_median = df['average_price'].median()
print(price_median)
df['is_high'] = df['average_price'].map(lambda x: True if x>= price_median else False)
print(df['is_high'].value_counts())
print(df.shape)
print(df.dtypes)
print(df.isnull().sum())
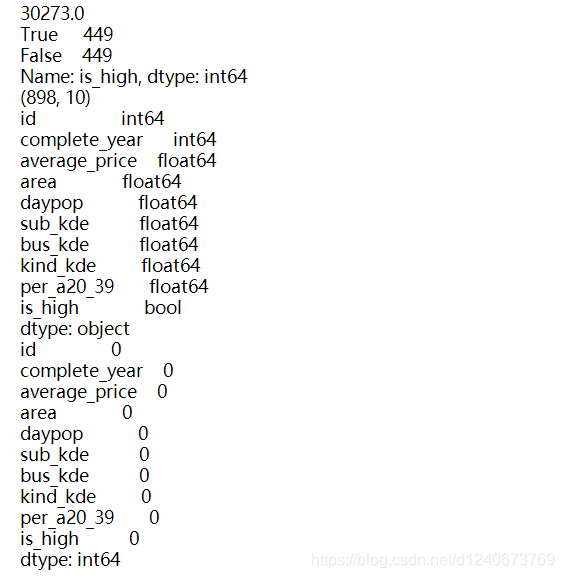
留出法进行数据集划分
from sklearn.model_selection import train_test_split
training, testing = train_test_split(df,test_size=0.25, random_state=1)
x_train = training.copy()[['complete_year','area', 'daypop', 'sub_kde',
'bus_kde', 'kind_kde','per_a20_39']]
y_train = training.copy()['is_high']
x_test = testing.copy()[['complete_year','area', 'daypop', 'sub_kde',
'bus_kde', 'kind_kde','per_a20_39']]
y_test = testing.copy()['is_high']
print(f'the shape of training set is: {training.shape}')
print(f'the shape of testing set is: {testing.shape}')

构建模型
from sklearn.preprocessing import PowerTransformer, StandardScaler, PolynomialFeatures
from sklearn.pipeline import Pipeline
from sklearn.ensemble import RandomForestClassifier
from sklearn.model_selection import StratifiedKFold, GridSearchCV
from sklearn.model_selection import RandomizedSearchCV
rf_model = RandomForestClassifier(criterion='gini',
n_jobs=16,
n_estimators = 100,
random_state=133)
parameters = {'max_features': ['auto',5, 0.8, None],
'max_depth': [None, 3, 9]}
cv = StratifiedKFold(n_splits=3, shuffle=True)
rf_gridsearch = GridSearchCV(rf_model, parameters, n_jobs=16, cv=cv, scoring='roc_auc',
verbose=2, refit=True)
pipe_clf = Pipeline([
('sc',StandardScaler()),
('power_trans',PowerTransformer()),
('polynom_trans',PolynomialFeatures(degree=2)),
('rf_clf',rf_gridsearch)
])
print(pipe_clf)
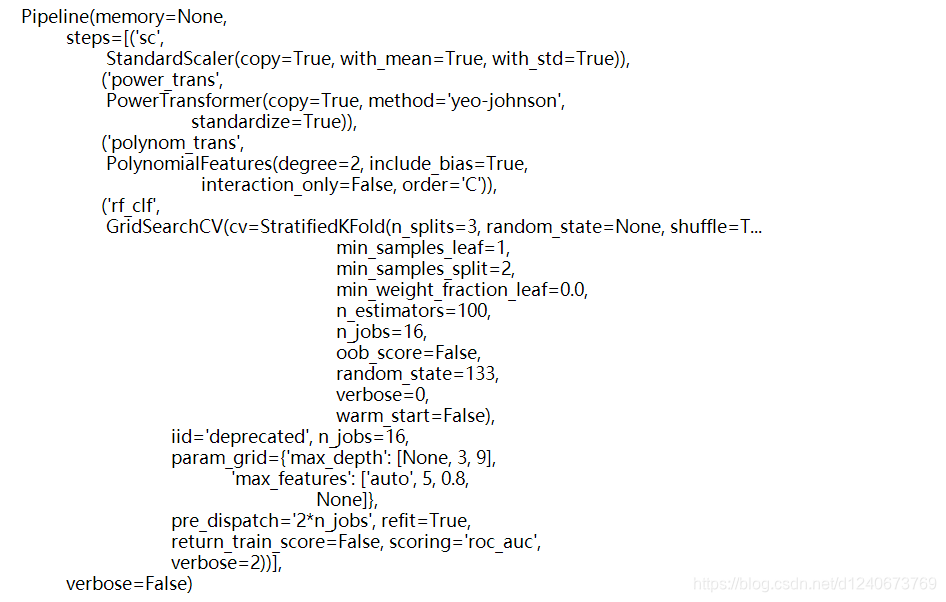
模型表现
import warnings
from sklearn.metrics import accuracy_score, precision_score, recall_score, roc_auc_score
warnings.filterwarnings('ignore')
pipe_clf.fit(x_train,y_train)
y_predict = pipe_clf.predict(x_test)
print(f'accuracy score is: {accuracy_score(y_test,y_predict)}')
print(f'precision score is: {precision_score(y_test,y_predict)}')
print(f'recall score is: {recall_score(y_test,y_predict)}')
print(f'auc: {roc_auc_score(y_test,y_predict)}')

print(pipe_clf.named_steps['rf_clf'].best_params_)

|