一、KITTI数据集介绍 KITTI数据集是一个用于自动驾驶场景下的计算机视觉算法测评数据集,由德国卡尔斯鲁厄理工学院(KIT)和丰田工业大学芝加哥分校(TTIC)共同创立。 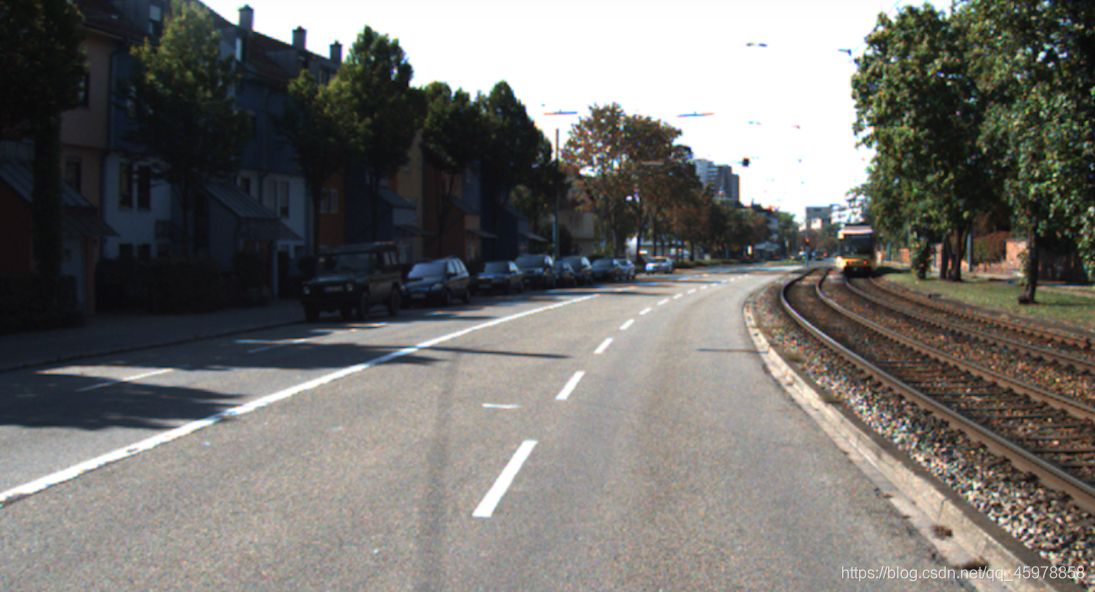
包含场景:市区、乡村和高速公路 在这里,我们只用到它的部分与行人,车辆有关的内容
下载可以转到官网 http://www.cvlibs.net/download.php?file=data_object_image_2.zip http://www.cvlibs.net/download.php?file=data_object_label_2.zip 得到我们的图片和标签
我们再yolov5/dataset下创建文件夹kitti 再kiiti中放入我们的数据
|——kitti
├── imgages
│ ├── val
│ │ └── 000000.png
├── .......
│ └── train
│ │ └── 000000.png
├── .......
│
└── labels
└── train
注意此时先不要把标签数据放入,我们需要对标签转换一下
二、KITTI数据集转换 我们打开标签中的一个内容 比如000000.txt
Pedestrian 0.00 0 -0.20 712.40 143.00 810.73 307.92 1.89 0.48 1.20 1.84 1.47 8.41 0.01
这里是kitty独有的数据格式,不适用于我们的yolov5网络,所以我们得转换一下
首先我们把类别归一一下,因为我们只需要用到三个类(代码中的路径自行修改)
import glob
import string
txt_list = glob.glob('你下载的标签文件夹的标签路径/*.txt')
def show_category(txt_list):
category_list= []
for item in txt_list:
try:
with open(item) as tdf:
for each_line in tdf:
labeldata = each_line.strip().split(' ')
category_list.append(labeldata[0])
except IOError as ioerr:
print('File error:'+str(ioerr))
print(set(category_list))
def merge(line):
each_line=''
for i in range(len(line)):
if i!= (len(line)-1):
each_line=each_line+line[i]+' '
else:
each_line=each_line+line[i]
each_line=each_line+'\n'
return (each_line)
print('before modify categories are:\n')
show_category(txt_list)
for item in txt_list:
new_txt=[]
try:
with open(item, 'r') as r_tdf:
for each_line in r_tdf:
labeldata = each_line.strip().split(' ')
if labeldata[0] in ['Truck','Van','Tram']:
labeldata[0] = labeldata[0].replace(labeldata[0],'Car')
if labeldata[0] == 'Person_sitting':
labeldata[0] = labeldata[0].replace(labeldata[0],'Pedestrian')
if labeldata[0] == 'DontCare':
continue
if labeldata[0] == 'Misc':
continue
new_txt.append(merge(labeldata))
with open(item,'w+') as w_tdf:
for temp in new_txt:
w_tdf.write(temp)
except IOError as ioerr:
print('File error:'+str(ioerr))
print('\nafter modify categories are:\n')
show_category(txt_list)
然后我们再把它转换为xml文件 创建一个Annotations文件夹用于存放xml
from xml.dom.minidom import Document
import cv2
import os
def generate_xml(name,split_lines,img_size,class_ind):
doc = Document()
annotation = doc.createElement('annotation')
doc.appendChild(annotation)
title = doc.createElement('folder')
title_text = doc.createTextNode('KITTI')
title.appendChild(title_text)
annotation.appendChild(title)
img_name=name+'.png'
title = doc.createElement('filename')
title_text = doc.createTextNode(img_name)
title.appendChild(title_text)
annotation.appendChild(title)
source = doc.createElement('source')
annotation.appendChild(source)
title = doc.createElement('database')
title_text = doc.createTextNode('The KITTI Database')
title.appendChild(title_text)
source.appendChild(title)
title = doc.createElement('annotation')
title_text = doc.createTextNode('KITTI')
title.appendChild(title_text)
source.appendChild(title)
size = doc.createElement('size')
annotation.appendChild(size)
title = doc.createElement('width')
title_text = doc.createTextNode(str(img_size[1]))
title.appendChild(title_text)
size.appendChild(title)
title = doc.createElement('height')
title_text = doc.createTextNode(str(img_size[0]))
title.appendChild(title_text)
size.appendChild(title)
title = doc.createElement('depth')
title_text = doc.createTextNode(str(img_size[2]))
title.appendChild(title_text)
size.appendChild(title)
for split_line in split_lines:
line=split_line.strip().split()
if line[0] in class_ind:
object = doc.createElement('object')
annotation.appendChild(object)
title = doc.createElement('name')
title_text = doc.createTextNode(line[0])
title.appendChild(title_text)
object.appendChild(title)
bndbox = doc.createElement('bndbox')
object.appendChild(bndbox)
title = doc.createElement('xmin')
title_text = doc.createTextNode(str(int(float(line[4]))))
title.appendChild(title_text)
bndbox.appendChild(title)
title = doc.createElement('ymin')
title_text = doc.createTextNode(str(int(float(line[5]))))
title.appendChild(title_text)
bndbox.appendChild(title)
title = doc.createElement('xmax')
title_text = doc.createTextNode(str(int(float(line[6]))))
title.appendChild(title_text)
bndbox.appendChild(title)
title = doc.createElement('ymax')
title_text = doc.createTextNode(str(int(float(line[7]))))
title.appendChild(title_text)
bndbox.appendChild(title)
f = open('Annotations/trian'+name+'.xml','w')
f.write(doc.toprettyxml(indent = ''))
f.close()
if __name__ == '__main__':
class_ind=('Pedestrian', 'Car', 'Cyclist')
cur_dir=os.getcwd()
labels_dir=os.path.join(cur_dir,'Labels')
for parent, dirnames, filenames in os.walk(labels_dir):
for file_name in filenames:
full_path=os.path.join(parent, file_name)
f=open(full_path)
split_lines = f.readlines()
name= file_name[:-4]
img_name=name+'.png'
img_path=os.path.join('./JPEGImages/trian',img_name)
img_size=cv2.imread(img_path).shape
generate_xml(name,split_lines,img_size,class_ind)
print('all txts has converted into xmls')
这个时候我们已经将.txt转化为.xml并存放在Annotations下了
最后我们再把.xml转化为适合于yolo训练的标签模式 也就是darknet的txt格式 例如:
0 0.9074074074074074 0.7413333333333333 0.09178743961352658 0.256
0 0.3635265700483092 0.6386666666666667 0.0785024154589372 0.14533333333333334
2 0.6996779388083736 0.5066666666666667 0.008051529790660225 0.08266666666666667
0 0.7024959742351047 0.572 0.0430756843800322 0.09733333333333333
0 0.6755233494363929 0.544 0.03140096618357488 0.06933333333333333
0 0.48027375201288247 0.5453333333333333 0.030998389694041867 0.068
0 0.5032206119162641 0.528 0.021739130434782608 0.05333333333333334
0 0.6533816425120773 0.5306666666666666 0.02214170692431562 0.056
0 0.5515297906602254 0.5053333333333333 0.017713365539452495 0.03866666666666667
import glob
import xml.etree.ElementTree as ET
class_names = ['Car', 'Cyclist', 'Pedestrian']
path = './Annotations/'
def single_xml_to_txt(xml_file):
tree = ET.parse(xml_file)
root = tree.getroot()
txt_file = xml_file.split('.')[0]+'.'+xml_file.split('.')[1]+'.txt'
with open(txt_file, 'w') as txt_file:
for member in root.findall('object'):
picture_width = int(root.find('size')[0].text)
picture_height = int(root.find('size')[1].text)
class_name = member[0].text
class_num = class_names.index(class_name)
box_x_min = int(member[1][0].text)
box_y_min = int(member[1][1].text)
box_x_max = int(member[1][2].text)
box_y_max = int(member[1][3].text)
print(box_x_max,box_x_min,box_y_max,box_y_min)
x_center = float(box_x_min + box_x_max) / (2 * picture_width)
y_center = float(box_y_min + box_y_max) / (2 * picture_height)
width = float(box_x_max - box_x_min) / picture_width
height = float(box_y_max - box_y_min) / picture_height
print(class_num, x_center, y_center, width, height)
txt_file.write(str(class_num) + ' ' + str(x_center) + ' ' + str(y_center) + ' ' + str(width) + ' ' + str(height) + '\n')
def dir_xml_to_txt(path):
for xml_file in glob.glob(path + '*.xml'):
single_xml_to_txt(xml_file)
dir_xml_to_txt(path)
最后我们将得到的Annotations/下的所有txt文件放入我们之前的dataset/labels中
|——kitti
├── imgages
│ ├── val
│ │ └── 000000.png
├── .......
│ └── train
│ │ └── 000000.png
├── .......
│
└── labels
└── train
└── 000000.txt
├── .......
这样我们的数据集就准备好了 接下来我们可以训练了,跟我上一篇的教程一样,你们可以先了解怎么训练yolov5的步骤 https://blog.csdn.net/qq_45978858/article/details/119686255?spm=1001.2014.3001.5501
三、KITTI数据集训练 这里我们直接开始 1.在data文件夹中复制一份coco.yaml然后改名kitti.yaml修改内容
train: ../yolov5-kitty/dataset/kitti/images/train
val: ../yolov5-kitty/dataset/kitti/images/train
nc: 3
names: ['Car','Pedestrian','Cyclist']
2.在models文件夹修改yolov5s.yaml内容
nc: 3
depth_multiple: 0.33
width_multiple: 0.50
anchors:
- [10,13, 16,30, 33,23]
- [30,61, 62,45, 59,119]
- [116,90, 156,198, 373,326]
backbone:
[[-1, 1, Focus, [64, 3]],
[-1, 1, Conv, [128, 3, 2]],
[-1, 3, C3, [128]],
[-1, 1, Conv, [256, 3, 2]],
[-1, 9, C3, [256]],
[-1, 1, Conv, [512, 3, 2]],
[-1, 9, C3, [512]],
[-1, 1, Conv, [1024, 3, 2]],
[-1, 1, SPP, [1024, [5, 9, 13]]],
[-1, 3, C3, [1024, False]],
]
head:
[[-1, 1, Conv, [512, 1, 1]],
[-1, 1, nn.Upsample, [None, 2, 'nearest']],
[[-1, 6], 1, Concat, [1]],
[-1, 3, C3, [512, False]],
[-1, 1, Conv, [256, 1, 1]],
[-1, 1, nn.Upsample, [None, 2, 'nearest']],
[[-1, 4], 1, Concat, [1]],
[-1, 3, C3, [256, False]],
[-1, 1, Conv, [256, 3, 2]],
[[-1, 14], 1, Concat, [1]],
[-1, 3, C3, [512, False]],
[-1, 1, Conv, [512, 3, 2]],
[[-1, 10], 1, Concat, [1]],
[-1, 3, C3, [1024, False]],
[[17, 20, 23], 1, Detect, [nc, anchors]],
]
当然你也要有个一yolo5s.pt权重文件放在yolov5文件夹中,在我的前面的博客也有下载地址 3.开始训练
不用空行,空格间隔就可以
python train.py --img 640 --batch-size 16
--epochs 10 --data data/kitti.yaml
--cfg models/yolov5s.yaml --weights yolov5s.pt
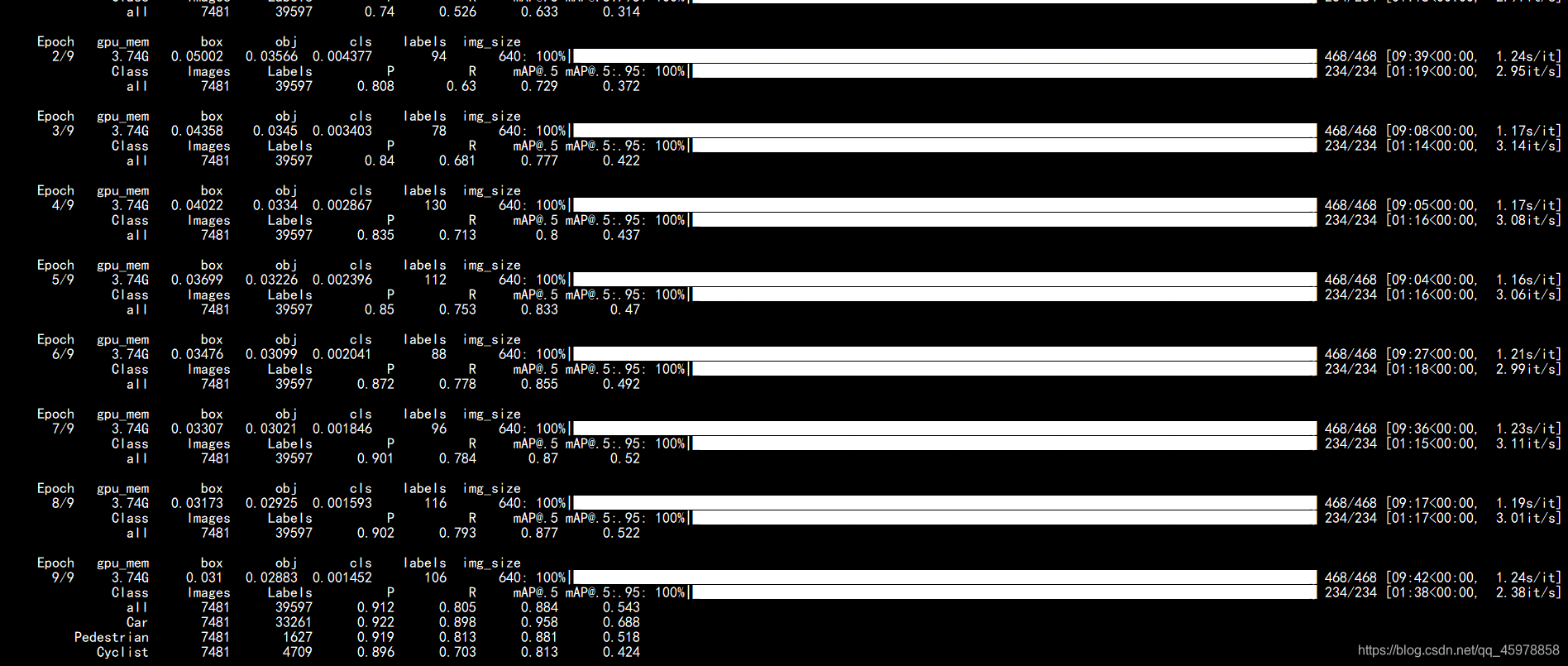 我这里虽然只训练了十个周期,但是还是花了一个多小时,准确率也非常不错,达到了0.9以上
现在我们在runs/train/exp下可以看到我们的训练的结果 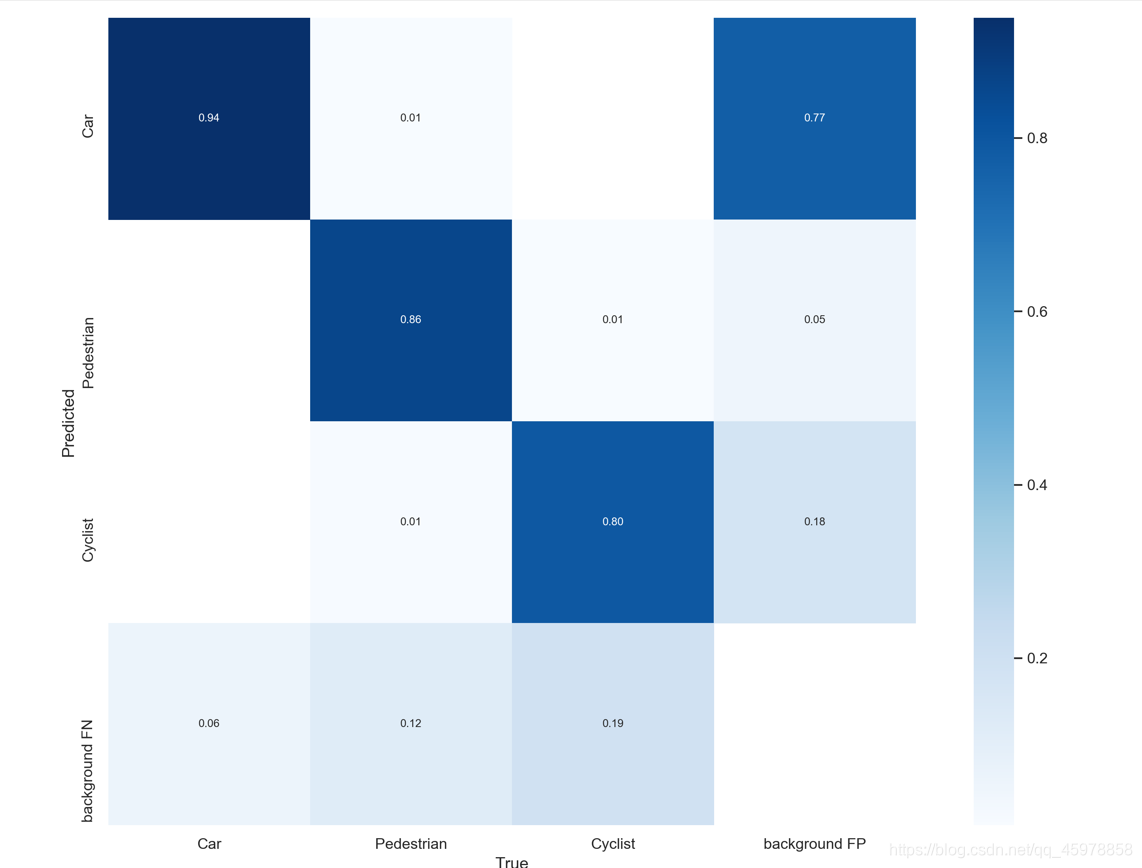 准确率都可以
我们可以拿着训练完的最好的权重试一试
python detect.py --weights runs/train/exp/weights/best.pt
--source Road_traffic_video2.mp4 这里可以是图片 也可以是视频 也可以是0(摄像头)
--device 0
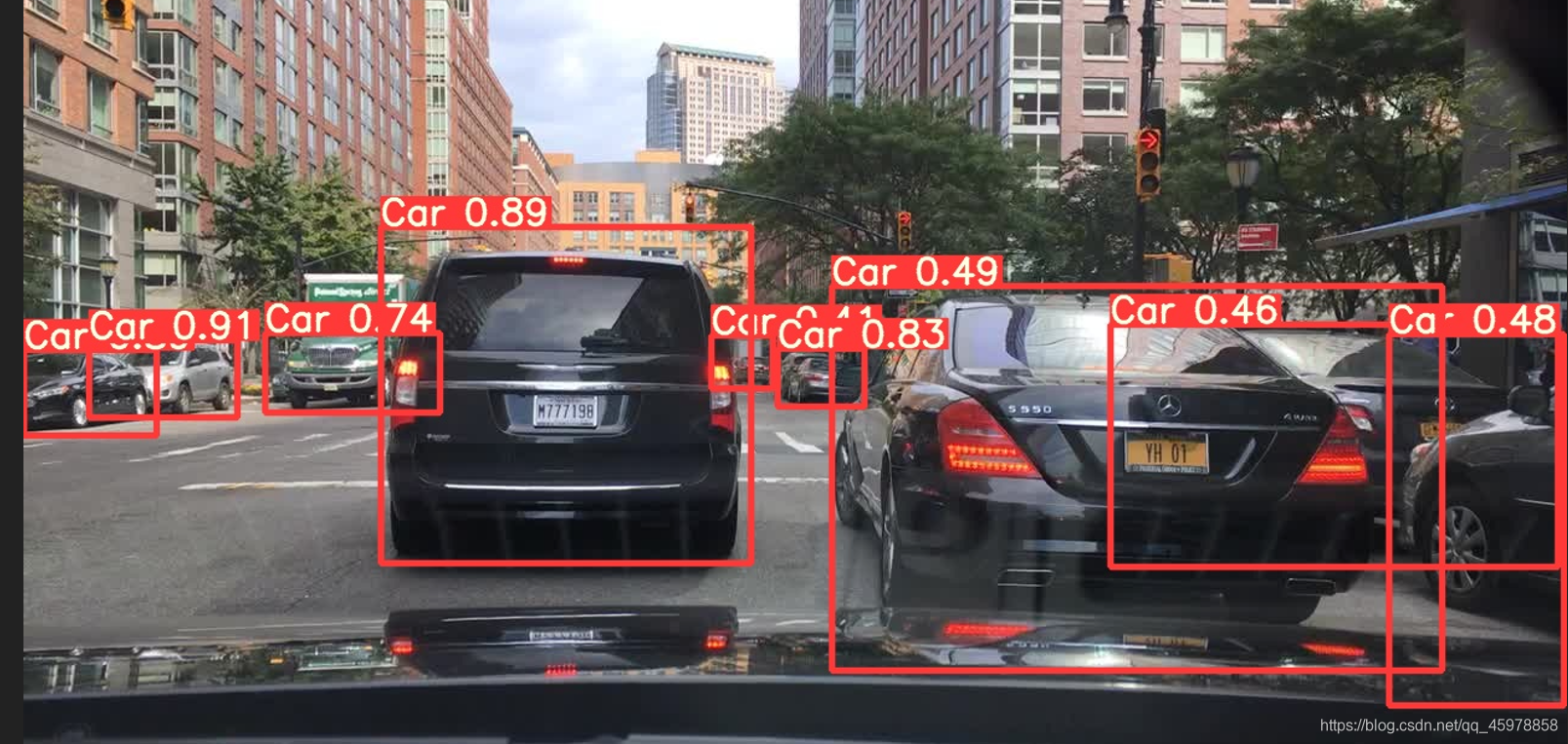 可以看到效果还是可以的,我这只训练了10个epoch,条件好的可以训练300个甚至更久
|