_ init _()函数
参数: self, config, pretrained_word_embedding, pretrained_entity_embedding, pretrained_context_embedding config: 设置的固定的参数! pretrained_word_embedding: 根据下面的使用是一个bool类型,表示是不是单词被预训练过! pretrained_entity_embedding、pretrained_context_embedding: 同理也是
super(KCNN, self).__init__()
self.config = config
if pretrained_word_embedding is None:
self.word_embedding = nn.Embedding(config.num_words,
config.word_embedding_dim,
padding_idx=0)
else:
self.word_embedding = nn.Embedding.from_pretrained(
pretrained_word_embedding, freeze=False, padding_idx=0)
if pretrained_entity_embedding is None:
self.entity_embedding = nn.Embedding(config.num_entities,
config.entity_embedding_dim,
padding_idx=0)
else:
self.entity_embedding = nn.Embedding.from_pretrained(
pretrained_entity_embedding, freeze=False, padding_idx=0)
if config.use_context:
if pretrained_context_embedding is None:
self.context_embedding = nn.Embedding(
config.num_entities,
config.entity_embedding_dim,
padding_idx=0)
else:
self.context_embedding = nn.Embedding.from_pretrained(
pretrained_context_embedding, freeze=False, padding_idx=0)
self.transform_matrix = nn.Parameter(
torch.empty(self.config.entity_embedding_dim,
self.config.word_embedding_dim).uniform_(-0.1, 0.1))
self.transform_bias = nn.Parameter(
torch.empty(self.config.word_embedding_dim).uniform_(-0.1, 0.1))
self.conv_filters = nn.ModuleDict({
str(x): nn.Conv2d(3 if self.config.use_context else 2,
self.config.num_filters,
(x, self.config.word_embedding_dim))
for x in self.config.window_sizes
})
self.additive_attention = AdditiveAttention(
self.config.query_vector_dim, self.config.num_filters)
def forward(self, news):
"""
Args:
news: #输入的news参数是个字典! (title, title_entities) 个数
{
"title": batch_size * num_words_title,
"title_entities": batch_size * num_words_title
}
Returns:
final_vector: batch_size, len(window_sizes) * num_filters
"""
word_vector = self.word_embedding(news["title"].to(device))
entity_vector = self.entity_embedding(
news["title_entities"].to(device))
if self.config.use_context:
context_vector = self.context_embedding(
news["title_entities"].to(device))
transformed_entity_vector = torch.tanh(
torch.add(torch.matmul(entity_vector, self.transform_matrix),
self.transform_bias))
if self.config.use_context:
transformed_context_vector = torch.tanh(
torch.add(torch.matmul(context_vector, self.transform_matrix),
self.transform_bias))
multi_channel_vector = torch.stack([
word_vector, transformed_entity_vector,
transformed_context_vector
],
dim=1)
else:
multi_channel_vector = torch.stack(
[word_vector, transformed_entity_vector], dim=1)
pooled_vectors = []
for x in self.config.window_sizes:
convoluted = self.conv_filters[str(x)](
multi_channel_vector).squeeze(dim=3)
activated = F.relu(convoluted)
pooled = self.additive_attention(activated.transpose(1, 2))
pooled_vectors.append(pooled)
final_vector = torch.cat(pooled_vectors, dim=1)
return final_vector
补充:
1、 python中的继承!
python2.7中的继承:
super是superclass的缩写,而且在super()中要包含两个实参,子类名和对象self ! 这些必不可少!
同时父类中必须含有object这个原始父类!
2、 torch.nn.Module()
如果自己想研究,官方文档
它是所有的神经网络的根父类! 你的神经网络必然要继承! 模块也可以包含其他模块,允许将它们嵌套在树结构中。所以呢,你可以将子模块指定为常规属性。常规定义子模块的方法如下: 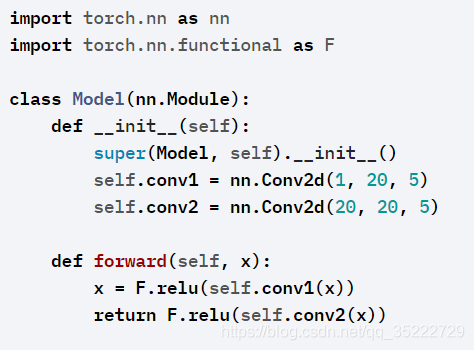
以这种方式分配的子模块将被注册(也就是成为你的该分支下的子类),当你调用to()等方法的时候时,它们的参数也将被转换,等等。 当然子模块就可以包含各种线性or卷积等操作了! 也就是模型
该模型的方法: 参考博文
3、 torch.nn.Embedding(num_embeddings, embedding_dim, padding_idx=None, max_norm=None, norm_type=2.0, scale_grad_by_freq=False, sparse=False, _weight=None)
参考博文 是torch.nn中集成的进行词嵌入的方法!
4. torch.nn
|