完整代码连接
一、K-means算法
1.1 K-means 介绍
\quad
K-means 算法是一种聚类算法,所谓聚类,即根据相似性原则,将具有较高相似度的数据对象划分至同一类簇,将具有较高相异度的数据对象划分至不同类簇。 聚类与分类最大的区别在于,聚类过程为无监督过程,即待处理数据对象没有任何先验知识,而分类过程为有监督过程,即存在有先验知识的训练数据集。
\quad
K-means 算法中的
K
K
K 代表类簇个数,
m
e
a
n
s
means
means 代表簇内数据对象的均值 (当有部分异常点时,求均值是不合理的,即一个极大异常点,或者极小的异常点,都会影响均值的数值),因此,K-means 算法又称为
k
?
均
值
算
法
k-均值算法
k?均值算法,k-means 算法是一种基于划分的聚类算法,以距离作为数据对象间相似性度量的标准,即数据对象间的距离越小,则它们的相似性越高,则它们越有可能在同一个类簇。数据对象间距离的计算有很多种,k-means 算法通常采用欧氏距离来计算数据对象间的距离。
1.2 K-means 算法原理
\quad
K-means 算法以距离作为数据对象间相似性度量的标准,通常采用欧氏距离来计算数据对象间的距离。下面给出欧式距离的计算公式 (
x
x
x 代表据具有
m
m
m 个属性的行向量):
d
i
s
t
(
x
i
,
x
j
)
=
(
x
i
?
x
j
)
?
(
x
i
?
x
j
)
T
dist(x_i, x_j) = \sqrt{(x_i - x_j)*(x_i - x_j)^T}
dist(xi?,xj?)=(xi??xj?)?(xi??xj?)T
?
\quad
K-means 算法聚类过程中,每次迭代,对应的类簇中心需要重新计算 (更新):簇中所有数据对象的均值,即为更新后该簇的中心。定义第
K
K
K 个簇的中心为
C
e
n
t
e
r
k
Center_k
Centerk? ,则类簇中心更新方式如下:
C
e
n
t
e
r
k
=
1
∣
C
k
∣
∑
x
i
∈
C
k
x
i
Center_k = \frac{1}{|C_k|} \sum_{x_i \in C_k}{x_i}
Centerk?=∣Ck?∣1?xi?∈Ck?∑?xi?
\quad
其中,
C
k
C_k
Ck? 表示第
k
k
k 个类簇,
∣
C
k
∣
|C_k|
∣Ck?∣ 表示第
k
k
k 个类簇中数据对象的个数,这里的求和是指类簇
C
k
C_k
Ck? 中所有元素在每列属性上的和,因此
C
e
n
t
e
r
k
Center_k
Centerk? 也是一个含有
m
m
m 个属性的行向量。
\quad
K-means 算法需要不断地迭代来重新划分类簇,并更新簇中心。 一般情况,有两种方法来终止迭代:(1)一种方法是设定迭代次数
T
T
T,当到达第
T
T
T 次迭代,则终止迭代,此时所得簇即为最终聚类结果;(2)另一种方法是K个中心点不变或是变化较小,函数模型如下:
Δ
J
=
C
e
n
t
e
r
k
n
e
w
?
C
e
n
t
e
r
k
o
l
d
\Delta J = Center_k^{new} - Center_k^{old}
ΔJ=Centerknew??Centerkold? 此公式表示,簇的中心点变化的幅度,其中,
K
K
K 表示簇的个数。当两次迭代
J
J
J 的差值小于某一阈值时,即
Δ
J
<
δ
\Delta J < \delta
ΔJ<δ时,则终止迭代,此时所得簇即为最终聚类结果。即下次类中心都移动距离不大则聚类完毕
1.3 K-means 算法思想:
- 初始化
K
K
K 个簇集中心,然后计算各个数据对象到簇集中心的距离,把数据对象划分至距最近的聚类中心所在簇中,跳转到2
- 根据所得簇,得到新的簇中心;同时计算簇的中心点变化的幅度
Δ
J
\Delta J
ΔJ,跳转到3
- 判断
Δ
J
\Delta J
ΔJ是否小于阈值或者循环次数是否大于
T
T
T,如果小于阈值或者循环次数大于
T
T
T,跳出循环,结束聚类。否则跳转到4
- 根据所得簇,得到新的簇集中心;同时计算簇的中心点变化的幅度
Δ
J
\Delta J
ΔJ。
1.4 K-means 算法实现:
1.4.1 numpy实现 K-means 算法
class K_Means(Cluster):
def __init__(self, k=9):
"""
:param k: 随机的中心点的个数
"""
super(K_Means, self).__init__()
self.k = k
def __CentralPointOfCluster(self, data_indexes, data_matrix):
""" 计算聚类结果的中心点
:param data_indexes: 类中点的索引
:param data_matrix: 类中点的各维度的数据
:return: 聚类数量,聚类结果的中心点
"""
bin_size = len(data_indexes)
c_point = None
min_sum = float("inf")
for cpoint_index in data_indexes:
sum_distance = 0
for point_index in data_indexes:
sum_distance += self.Compute_Euclidean(data_matrix[cpoint_index], data_matrix[point_index])
if sum_distance < min_sum:
min_sum = sum_distance
c_point = data_matrix[cpoint_index]
return bin_size, c_point
def k_means(self, pointsIndex, matrix, iteration=150):
"""K_means的聚类算法
:param pointsIndex: 需要聚类的点的Id的集合
:param matrix: 点的真实位置
:param iteration: 最大迭代次数
:return: [中心点,中心点的聚类结果]
"""
flag = 0
originalCpoints = [matrix[sample_index] for sample_index in random.sample(pointsIndex, self.k)]
cpoints = copy.deepcopy(originalCpoints)
while True:
clusterPoints = [[] for i in range(len(originalCpoints))]
for pointIndex in pointsIndex:
minDistance = float("inf")
selectCpoint = 0
for cpointIndex in range(len(cpoints)):
v1 = cpoints[cpointIndex]
v2 = matrix[pointIndex]
distance = self.Compute_Euclidean(v1, v2)
if minDistance > distance:
minDistance = distance
selectCpoint = cpointIndex
clusterPoints[selectCpoint].append(pointIndex)
cpoints = []
result = [[] for i in range(len(clusterPoints))]
for i in range(len(clusterPoints)):
cluster_pointsIndex = clusterPoints[i]
if len(cluster_pointsIndex) == 0:
continue
_, cpoint = self.__CentralPointOfCluster(cluster_pointsIndex, matrix)
cpoints.append(cpoint)
result[i].append(cpoint)
result[i].append(cluster_pointsIndex)
cpoints = np.asarray(cpoints)
originalCpoints = np.asarray(originalCpoints)
up_bound = cpoints < originalCpoints * 1.05
low_bound = originalCpoints * 0.95 < cpoints
if False not in up_bound:
if False not in low_bound:
print("聚类完成,共聚类{}次".format(flag))
break
flag += 1
if flag > iteration:
print("已经聚类{}次,时间过长,已经终止".format(iteration))
break
print("已经聚类{}次".format(flag))
return result
使用 scikit-learn 调用 K-means 算法
import utils as ut
import numpy as np
from sklearn.cluster import KMeans
data, labels = ut.load_data("dataset/data_1.csv", drop=0.1, error=0.01)
std = ut.Transverter(data, "standardization")
data = std.fit()
pointsIndex = [i for i in range(len(data))]
k_means = KMeans(n_clusters=3,max_iter=100,tol=0.001)
k_means.fit(data)
label_pred = k_means.labels_
centroids = k_means.cluster_centers_
inertia = k_means.inertia_
print(label_pred)
1.5 K-means 优缺点
- 优点:
\quad
算法简单易实现; - 缺点:
\quad
需要用户事先指定类簇个数K;
\quad
对异常点敏感,一个特大都值,或者极小的值,会影响均值的数值
\quad
聚类结果对初始类簇中心的选取较为敏感;
\quad
容易陷入局部最优;
\quad
只能发现球型类簇;
二、DBSCAN算法
\quad
DBSCAN(Density-Based Spatial Clustering of Applications with Noise) 是一基 于密度的聚类算法,DBSCAN将簇定义为密度相连的点的最大集合,能够把具有足够高密度的区域划分为簇,并可在噪声的空间数据库中发现任意形状的聚类。
2.1 DBSCAN 相关定义
\quad
DBSCAN 是基于一组邻域来描述样本集的紧密程度的,参数
(
?
,
M
i
n
P
t
s
)
(\epsilon , MinPts)
(?,MinPts) 用来描述邻域的样本分布紧密程度。其中,
?
\epsilon
? 描述了某一数据点的邻域距离阈值(半径),
M
i
n
P
t
s
MinPts
MinPts 描述了数据点半径为
?
\epsilon
? 的邻域中数据点个数的最小个数。下面是与密度聚类相关的定义(假设我的样本集是
D
=
(
x
1
,
x
2
,
.
.
.
,
x
m
)
D=(x_1,x_2,...,x_m)
D=(x1?,x2?,...,xm?):
-
?
\epsilon
?-邻域: 对于
x
j
∈
D
x_j \in D
xj?∈D x ,其
?
\epsilon
?-邻域包含样本集
D
D
D 中与
x
j
x_j
xj? 的距离不大于
?
\epsilon
? 的子样本集。即
N
?
(
x
j
)
=
{
x
i
∈
D
∣
d
i
s
t
a
n
c
e
(
x
i
,
x
j
)
≤
?
}
N_?(x_j)=\{x_i \in D|distance(x_i,x_j)≤ \epsilon\}
N??(xj?)={xi?∈D∣distance(xi?,xj?)≤?} , 这个子样本集的个数记为
∣
N
?
(
x
j
)
∣
|N_?(x_j)|
∣N??(xj?)∣ 。
?
\epsilon
?-邻域是一个集合。
- 核心对象: 对于任一样本
x
j
∈
D
x_j \in D
xj?∈D,如果其
?
\epsilon
?-邻域对应的
N
?
(
x
j
)
N_?(x_j)
N??(xj?) 至少包含
M
i
n
P
t
s
MinPts
MinPts 个样本,即如果
∣
N
?
(
x
j
)
∣
≥
M
i
n
P
t
s
|N_?(x_j)| \geq MinPts
∣N??(xj?)∣≥MinPts,则
x
j
x_j
xj? 是核心对象。
- 密度直达: 如果
x
i
x_i
xi? 位于
x
j
x_j
xj? 的
?
\epsilon
?-邻域中,且
x
j
x_j
xj? 是核心对象,则称
x
i
x_i
xi? 由
x
j
x_j
xj? 密度直达。反之不一定成立,即此时不能说
x
j
x_j
xj? 由
x
i
x_i
xi? 密度直达, 除非
x
i
x_i
xi? 也是核心对象,即密度直达不满足对称性。
- 密度可达: 对于
x
i
x_i
xi? 和
x
j
x_j
xj?, 如果存在样本序列
p
1
,
p
2
,
.
.
.
,
p
T
p_1,p_2,...,p_T
p1?,p2?,...,pT? ,满足
p
1
=
x
i
,
p
T
=
x
j
p_1=x_i, p_T=x_j
p1?=xi?,pT?=xj?, 且
p
t
+
1
p_{t+1}
pt+1? 由
p
t
p_t
pt? 密度直达,则称
x
j
x_j
xj? 由
x
i
x_i
xi? 密度可达。也就是说,密度可达满足传递性。此时序列中的传递样本 $ p_1,p_2,…,p_{T?1}$ 均为核心对象,因为只有核心对象才能使其他样本密度直达。密度可达也不满足对称性,这个可以由密度直达的不对称性得出。
- 密度相连: 对于
x
i
x_i
xi? 和
x
j
x_j
xj?,如果存在核心对象样本
x
k
x_k
xk? ,使
x
i
x_i
xi? 和
x
j
x_j
xj? 均由
x
k
x_k
xk? 密度可达,则称
x
i
x_i
xi? 和
x
j
x_j
xj? 密度相连。密度相连关系满足对称性。
\quad
从下图可以很容易看出理解上述定义,图中
M
i
n
P
t
s
=
5
MinPts=5
MinPts=5,红色的点都是核心对象,因为其
?
\epsilon
?-邻域至少有
5
5
5个样本。黑色的样本是非核心对象。所有核心对象密度直达的样本在以红色核心对象为中心的圆内,如果不在圆内,则不能密度直达。图中用绿色箭头连起来的核心对象组成了密度可达的样本序列,此序列是一个簇集。在这些密度可达的样本序列的?-邻域内所有的样本相互都是密度相连的 (注意,此图中有两个簇集)。 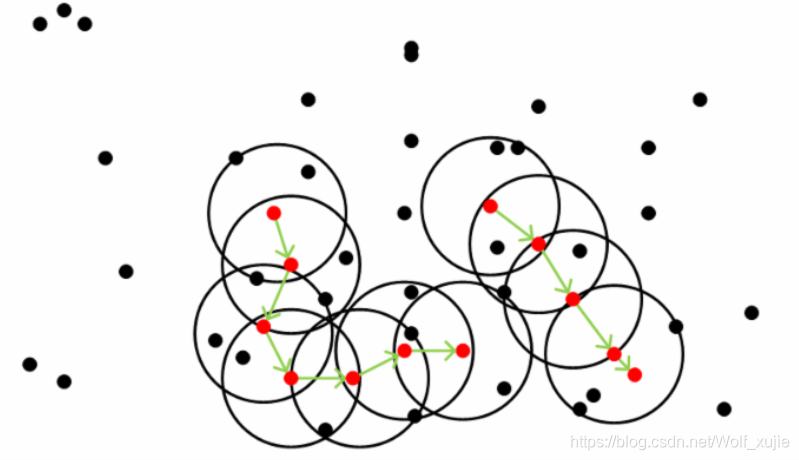
2.2 DBSCAN 密度聚类思想
DBSCAN 算法定义
\quad
DBSCAN 的聚类定义很简单:**由密度可达关系导出的最大密度相连的样本集合,即为我们最终聚类的一个类别,或者说一个簇(注意是密度相连的集合)。**簇里面可以有一个或者多个核心对象。如果只有一个核心对象,则簇里其他的非核心对象样本都在这个核心对象的
?
\epsilon
?-邻域里;如果有多个核心对象,则簇里的任意一个核心对象的
?
\epsilon
?-邻域中一定有一个其他的核心对象,否则这两个核心对象无法密度可达。这些核心对象的
?
\epsilon
?-邻域里所有的样本的集合组成的一个 DBSCAN 聚类簇。
\quad
那么怎么才能找到这样的簇样本集合呢? DBSCAN 使用的方法很简单,它任意选择一个没有类别的核心对象作为种子,然后找到所有这个核心对象能够密度可达的样本集合,即为一个聚类簇。接着继续选择另一个没有类别的核心对象去寻找密度可达的样本集合,这样就得到另一个聚类簇 (这样的得到都肯定是密度相连的)。一直运行到所有核心对象都有类别为止。
基本上这就是DBSCAN算法的主要内容了,但是我们还是有三个问题没有考虑。
- 异常点问题: 一些异常样本点或者说少量游离于簇外的样本点,这些点不在任何一个核心对象在周围,在DBSCAN中,我们一般将这些样本点标记为噪音点。
- 距离度量问题: 即如何计算某样本和核心对象样本的距离。在DBSCAN中,一般采用最近邻思想,采用某一种距离度量来衡量样本距离,比如欧式距离、曼哈顿距离等。
- 数据点优先级分配问题: 例如某些样本可能到两个核心对象的距离都小于
?
\epsilon
?,但是这两个核心对象由于不是密度直达,又不属于同一个聚类簇,那么如果界定这个样本的类别呢?一般来说,此时
DBSCAN 采用先来后到,先进行聚类的类别簇会标记这个样本为它的类别。也就是说 DBSCAN 的算法不是完全稳定的算法。
DBSCAN 算法流程
输入: 样本集
D
=
(
x
1
,
x
2
,
.
.
.
,
x
m
)
D=(x_1,x_2,...,x_m)
D=(x1?,x2?,...,xm?),邻域参数
(
?
,
M
i
n
P
t
s
)
(\epsilon,MinPts)
(?,MinPts)
- 初始化核心对象集合
Ω
=
?
\Omega = \emptyset
Ω=? ,初始化类别
k
=
0
k=0
k=0
- 遍历
D
D
D 的元素,如果是核心对象,则将其加入到核心对象集合
Ω
\Omega
Ω 中
- 如果核心对象集合
Ω
\Omega
Ω 中元素都已经被访问,则算法结束,否则转入步骤4
- 在核心对象集合
Ω
\Omega
Ω 中,随机选择一个 未访问 的核心对象
o
o
o,首先将
o
o
o 标记为 已访问,然后将
o
o
o 标记类别
k
k
k,最后将
o
o
o 的
?
\epsilon
?-邻域中未访问的数据,存放到种子集合
s
e
e
d
s
seeds
seeds中。
- 如果种子集合
s
e
e
d
s
=
?
seeds= \emptyset
seeds=?,则当前聚类簇
C
k
C_k
Ck?生成完毕, 且
k
=
k
+
1
k=k+1
k=k+1,跳转到3。否则,从种子集合
s
e
e
d
s
seeds
seeds 中挑选一个种子点
s
e
e
d
seed
seed,首先将其标记为 已访问、标记类别
k
k
k,然后判断
s
e
e
d
seed
seed 是否为核心对象,如果是将
s
e
e
d
seed
seed 中 未访问 的种子点加入到种子集合中,跳转到5。
2.3 DBSCAN 算法实现
使用 numpy 实现 DBSCAN 算法
def dbscan(self, pointsIndex, matrix):
dis_matrix = []
candid_matrix = [matrix[index] for index in pointsIndex]
candid_size = np.array(candid_matrix).shape[0]
for point_index in pointsIndex:
different_matrix = np.tile(matrix[point_index], (candid_size, 1)) - candid_matrix
square_matrix = np.power(different_matrix, 2)
sum_matrix = np.sum(square_matrix, axis=1)
sqrt_matrix = np.power(sum_matrix, 0.5)
dis_matrix.append(sqrt_matrix)
dis_matrix = np.asarray(dis_matrix)
core_points_index = np.where(np.sum(np.where(dis_matrix <= self.eps, 1, 0), axis=1) >= self.min_pts)[0]
point_labels = np.asarray([-1 for i in range(len(pointsIndex))])
cluster_id = 0
for core_index in core_points_index:
if(point_labels[core_index] == -1):
point_labels[core_index] = cluster_id
neighbour_index = np.where((dis_matrix[core_index] <= self.eps) & (point_labels == -1))[0]
seeds_index = set(neighbour_index)
while seeds_index:
new_point = seeds_index.pop()
point_labels[new_point] = cluster_id
if new_point in core_points_index:
point_field = np.where(dis_matrix[new_point] <= self.eps)[0]
for point in point_field:
if point_labels[point] == -1:
seeds_index.add(point)
cluster_id += 1
return point_labels
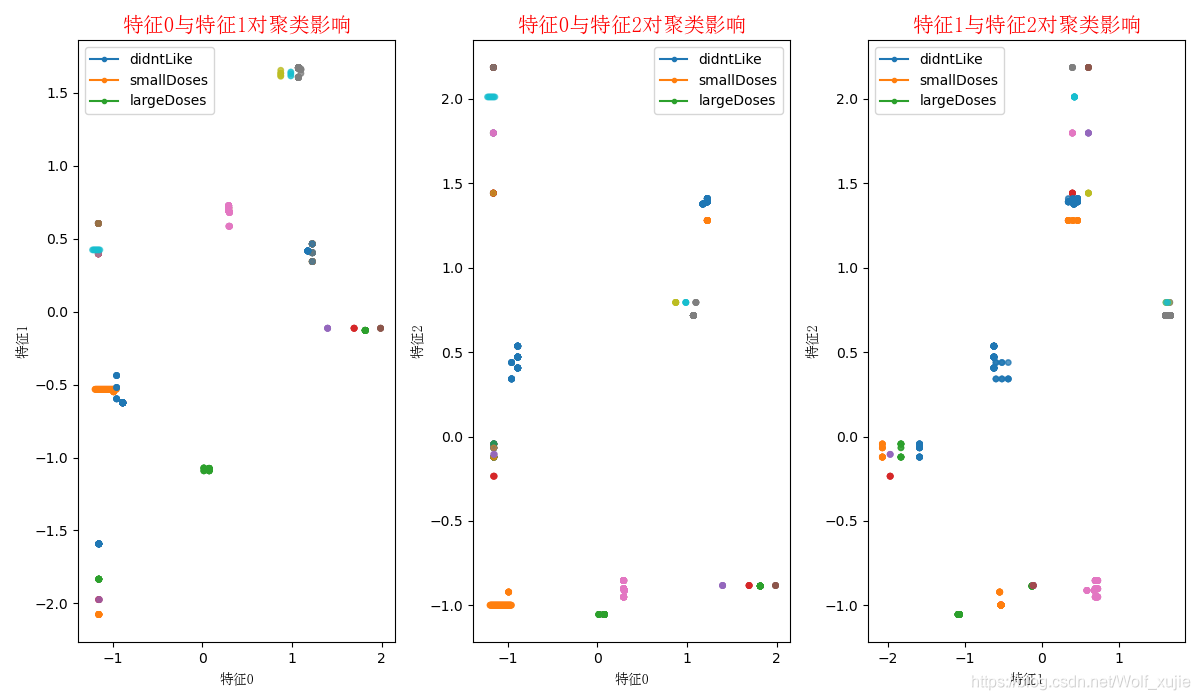
使用 scikit-learn 调用 DBSCAN 算法
from sklearn.cluster import DBSCAN
data, labels = ut.load_data("dataset/data_1.csv")
estimator=DBSCAN(eps=0.1,min_samples=3,metric='euclidean')
estimator.fit(data)
print(estimator.labels_)
2.4 DBSCAN 优缺点
DBSCAN 的主要优点有:
- 可以对任意形状的稠密数据集进行聚类,相对的,
K-Means 之类的聚类算法一般只适用于凸数据集。 - 可以在聚类的同时发现异常点,对数据集中的异常点不敏感。
- 聚类结果没有偏倚,相对的,
K-Means 之类的聚类算法初始值对聚类结果有很大影响。
DBSCAN 的主要缺点有:
- 如果样本集的密度不均匀、聚类间距差相差很大时,聚类质量较差,这时用DBSCAN聚类一般不适合。
- 如果样本集较大时,聚类收敛时间较长
- 调参相对于传统的
K-Means 之类的聚类算法稍复杂,主要需要对距离阈值
?
\epsilon
?,邻域样本阈值
M
i
n
P
t
s
MinPts
MinPts 联合调参,不同的参数组合对最后的聚类效果有较大影响。
三、OPTICS算法
\quad
OPTICS(Ordering points to identify the clustering structure) 是一基于密度的聚类算法,是 DBSCAN 的改进版本。在DBCSAN算法中需要输入两个参数:
?
\epsilon
? 和
M
i
n
P
t
s
MinPts
MinPts,选择不同的参数会导致最终聚类的结果千差万别,因此 DBCSAN 对于输入参数过于敏感。OPTICS 算法的提出就是为了帮助 DBSCAN 算法选择合适的参数,降低输入参数的敏感度。OPTICS 主要针对输入参数
?
\epsilon
? 过敏感做的改进,虽然 OPTICS 算法中也需要两个输入参数,但该算法对
?
\epsilon
? 输入不敏感(一般将
?
\epsilon
? 固定为无穷大),同时该算法中并不显式的生成数据聚类,而是为聚类分析生成一个增广的簇排序(比如,以可达距离为纵轴,样本点输出次序为横轴的坐标图),这个排序代表了各样本点基于密度的聚类结构。它包含的信息等价于从一个广泛的参数设置所获得的基于密度的聚类,换句话说,从这个排序中可以得到基于任何参数
?
\epsilon
? 和
M
i
n
P
t
s
MinPts
MinPts 的 DBSCAN 算法的聚类结果。
3.1 OPTICS 相关定义
\quad
由于 OPTICS 算法是 DBSCAN 算法的一种改进,因此有些概念是共用的,比如:
?
\epsilon
? -邻域,核心对象,密度直达,密度可达,密度相连 等,下面是与 OPTICS 相关的定义(假设我的样本集是
X
=
(
x
1
,
x
2
,
.
.
.
,
x
m
)
X=(x_1, x_2, ..., x_m)
X=(x1?,x2?,...,xm?):
- 核心距离(core-distance): 样本
x
∈
X
x \in X
x∈X ,对于给定的
?
\epsilon
? 和
M
i
n
P
t
s
MinPts
MinPts,使得
x
x
x 成为核心点的最小邻域半径称为
x
x
x 的核心距离。其数学表达如下,
N
?
i
(
x
)
N_\epsilon^{i}(x)
N?i?(x) 代表集合
N
?
(
x
)
N_\epsilon(x)
N??(x) 中与节点
x
x
x 第
i
i
i 近邻的节点,如
N
?
1
(
x
)
N_\epsilon^{1}(x)
N?1?(x) 表示
N
?
(
x
)
N_\epsilon(x)
N??(x) 中与
x
x
x 最近的节点
c
o
r
e
?
d
i
s
t
a
n
c
e
(
x
)
=
{
u
n
d
e
f
i
n
e
d
∣
N
?
(
x
)
∣
<
M
i
n
P
t
s
d
i
s
t
a
n
c
e
(
x
,
N
?
M
i
n
P
t
s
(
x
)
)
∣
N
?
(
x
)
∣
≥
M
i
n
P
t
s
core-distance(x)= \begin{cases} undefined& |N_\epsilon(x)| < MinPts\\ distance(x, N_\epsilon^{MinPts}(x))& |N_\epsilon(x)| \geq MinPts \end{cases}
core?distance(x)={undefineddistance(x,N?MinPts?(x))?∣N??(x)∣<MinPts∣N??(x)∣≥MinPts? - 可达距离(reachability-distance): 设
x
,
y
∈
X
x,y \in X
x,y∈X ,对于给定的
?
\epsilon
? 和
M
i
n
P
t
s
MinPts
MinPts,
y
y
y 关于
x
x
x 的可达距离定义为:
r
e
a
c
h
a
b
i
l
i
t
y
?
d
i
s
t
a
n
c
e
(
y
,
x
)
=
{
u
n
d
e
f
i
n
e
d
∣
N
?
(
x
)
∣
<
M
i
n
P
t
s
m
a
x
{
c
o
r
e
?
d
i
s
t
a
n
c
e
(
x
)
,
d
i
s
t
a
n
c
e
(
x
,
y
)
}
∣
N
?
(
x
)
∣
≥
M
i
n
P
t
s
reachability-distance(y, x)= \begin{cases} undefined& |N_\epsilon(x)| < MinPts\\ max\{core-distance(x), distance(x, y)\}& |N_\epsilon(x)| \geq MinPts \end{cases}
reachability?distance(y,x)={undefinedmax{core?distance(x),distance(x,y)}?∣N??(x)∣<MinPts∣N??(x)∣≥MinPts?
\quad
特别的,当
x
x
x 为核心点时(相应的参数为
?
\epsilon
? 和
M
i
n
P
t
s
MinPts
MinPts),可按照下式来理解
r
d
(
y
,
x
)
rd(y,x)
rd(y,x):
r
d
(
y
,
x
)
=
m
i
n
{
η
:
y
∈
N
η
(
x
)
且
∣
N
η
(
x
)
∣
≥
M
i
n
P
t
s
}
rd(y, x) = min\{ \eta : y \in N_{\eta}(x) 且 |N_{\eta}(x)| \geq MinPts\}
rd(y,x)=min{η:y∈Nη?(x)且∣Nη?(x)∣≥MinPts}
\quad
即
r
d
(
y
,
x
)
rd(y,x)
rd(y,x) 表示 使得“
x
x
x 成为核心点”,“
y
y
y 可以从
x
x
x 直接密度可达” 同时成立的最小邻域半径。
\quad
这样每一个点都有两个新属性:可达距离,核心距离。
3.2 OPTICS 聚类思想
\quad
假设我们的数据集为
X
=
(
x
1
,
x
2
,
.
.
.
,
x
m
)
X=(x_1,x_2,...,x_m)
X=(x1?,x2?,...,xm?),OPTICS 算法的目标是输出一个有序排列,以及每个元素的两个属性值:核心距离,可达距离。
OPTICS 算法定义
-
p
i
,
i
=
1
,
2
,
.
.
.
,
N
p_i,i=1,2,...,N
pi?,i=1,2,...,N:
OPTICS 算法的输出有序列表,例如
p
=
{
10
,
100
,
4
,
.
.
.
}
p=\{10,100,4,...\}
p={10,100,4,...} 表示:在集合
X
X
X 中的数据,第10号节点首先被处理,然后第100号节点被处理,然后第4号节点被处理(即节点被处理的顺序列表) -
c
i
,
i
=
1
,
2
,
.
.
.
,
N
c_i,i=1,2,...,N
ci?,i=1,2,...,N: 第
i
i
i 号节点的核心距离,例如
c
=
{
1.2
,
1.4
,
4.5
,
.
.
.
}
c=\{1.2, 1.4, 4.5,...\}
c={1.2,1.4,4.5,...} 表示:在集合
X
X
X 中的数据,第2号节点的核心距离为1.2,第1号节点的核心距离为1.4,第3号节点的核心距离为4.5
-
r
i
,
i
=
1
,
2
,
.
.
.
,
N
r_i,i=1,2,...,N
ri?,i=1,2,...,N: 第
i
i
i 号节点的可达距离,例如
r
=
{
3.4
,
3.1
,
4.5
,
.
.
.
}
r=\{3.4, 3.1, 4.5,...\}
r={3.4,3.1,4.5,...} 表示:在集合
X
X
X 中的数据,第1号节点的可达距离为3.4,第2号节点的可达距离为3.1,第3号节点的可达距离为4.5
OPTICS 算法流程
\quad
输入: 样本集
X
=
(
x
1
,
x
2
,
.
.
.
,
x
m
)
X=(x_1,x_2,...,x_m)
X=(x1?,x2?,...,xm?),邻域参数
(
?
,
M
i
n
P
t
s
)
(\epsilon, MinPts)
(?,MinPts)
- 初始化核心对象集合
Ω
=
?
\Omega = \emptyset
Ω=?
- 遍历
X
X
X 的元素,如果是核心对象,则将其加入到核心对象集合
Ω
\Omega
Ω中
- 如果核心对象集合
Ω
\Omega
Ω 中元素都已经被处理,则算法结束,否则转入步骤4.
- 在核心对象集合
Ω
\Omega
Ω 中,随机选择一个未处理的核心对象
o
o
o,首先将
o
o
o 标记为已处理,同时将
o
o
o 压入到有序列表
p
p
p 中,最后将
o
o
o 的
e
p
s
i
l
o
n
epsilon
epsilon-邻域中未访问的点,根据可达距离的大小(计算未访问的邻居点到
o
o
o 点的可达距离)依次存放到种子集合
s
e
e
d
s
seeds
seeds 中。
- 如果种子集合
s
e
e
d
s
=
?
seeds=\emptyset
seeds=?,跳转到3,否则,从种子集合
s
e
e
d
s
seeds
seeds 中挑选可达距离最近的种子点
s
e
e
d
seed
seed,首先将
s
e
e
d
seed
seed 标记为已处理,同时将
s
e
e
d
seed
seed 压入到有序列表
p
p
p 中,然后判断
s
e
e
d
seed
seed 是否为核心对象,如果是,将
s
e
e
d
seed
seed 中未访问的邻居点加入到种子集合中,重新计算可达距离(计算种子集合中距离
s
e
e
d
seed
seed 点的可达距离)跳转到5。
说明:
\quad
第一点,第一个被处理的对象是不存在可达距离的 (因为没有被计算过),只有进入过
s
e
e
d
s
seeds
seeds 的点才能计算可达距离
3.3 OPTICS 算法实现
使用 numpy 实现 OPTICS 算法
def optics(self, pointsIndex, matrix):
orders = []
self.dis_matrix = super().computer_distance(pointsIndex, matrix)
temp_core_distance = np.asarray([self.dis_matrix[i][num_index]
for i, num_index in enumerate(np.argsort(self.dis_matrix)[:, self.min_pts - 1])])
self.core_distances = np.where(temp_core_distance < self.eps, temp_core_distance, -1)
self.reach_distances = np.asarray([np.nan for i in range(len(pointsIndex))])
core_points_index = np.where(np.sum(np.where(self.dis_matrix <= self.eps, 1, 0), axis=1) >= self.min_pts)[0]
self.is_process = np.asarray([-1 for i in range(len(pointsIndex))])
for core_index in core_points_index:
if (self.is_process[core_index] == -1):
self.is_process[core_index] = 1
orders.append(core_index)
neighbour_index = np.where((self.dis_matrix[core_index] <= self.eps) & (self.is_process == -1))[0]
seeds = dict()
seeds = self.__update_seeds(seeds, core_index, neighbour_index)
while seeds:
next_id = sorted(seeds.items(), key=operator.itemgetter(1))[0][0]
del seeds[next_id]
self.is_process[next_id] = 1
orders.append(next_id)
if next_id in core_points_index:
neighbour_index = np.where((self.dis_matrix[next_id] <= self.eps) & (self.is_process == -1))[0]
seeds = self.__update_seeds(seeds, next_id, neighbour_index)
self.reach_distances[0] = 0
return orders, self.reach_distances
- 可达图
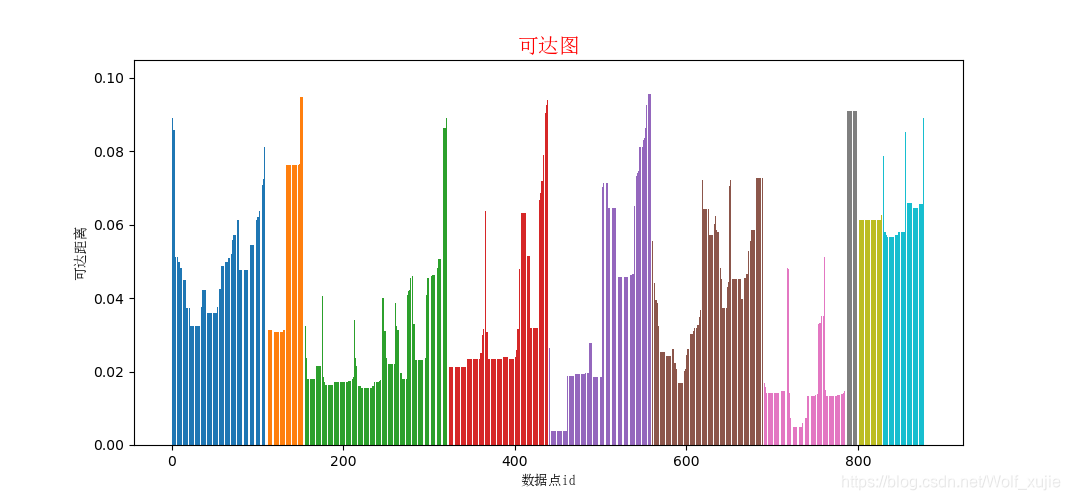 - 聚类结果可视化图(棕色是离群点)
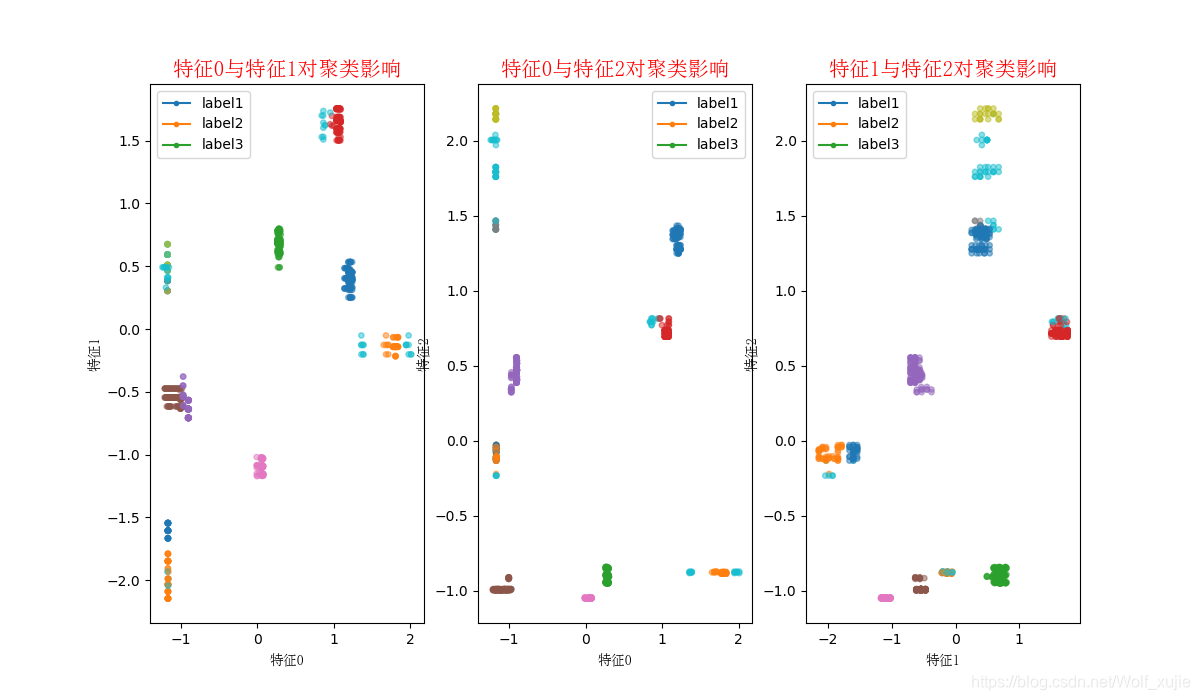
使用 scikit-learn 调用 OPTICS 算法
import utils as ut
import numpy as np
from sklearn.cluster import OPTICS
data, labels = ut.load_data("dataset/data_1.csv", drop=0.1, error=0.01)
std = ut.Transverter(data, "standardization")
data = std.fit()
pointsIndex = [i for i in range(len(data))]
optics = OPTICS(min_samples=10)
optics.fit(data, labels)
print(optics.fit_predict(data, labels))
|