NLP系列讲解笔记
本专题是针对NLP的一些常用知识进行记录,主要由于本人接下来的实验需要用到NLP的一些知识点,但是本人非NLP方向学生,对此不是很熟悉,所以打算做个笔记记录一下自己的学习过程,也是为了博士的求学之路做铺垫,希望可以找到一个NLP方向的博导吧😩!希望大家喜欢。如果有哪里写的不对,欢迎大家批评指正,感谢感谢!
传送门:
第一章 细讲:Attention模型的机制原理
前言
最近几年,Attention模型在NLP乃至深度学习、人工智能领域都是一个相当热门的词汇,被学术界和工业界的广大学者放入自己的模型当中,并得到了不错的反馈。再加上BERT的强势表现以及Transformer的霸榜,让大家对Attention变得更加感兴趣,本人在上一篇文章对Attention模型的机制原理进行了详细的介绍分析,有兴趣的可以自行查看哟。
纸上得来终觉浅,绝知此事要躬行。机制原理、理论讲的再好,没有实验证明也白搭。实践是检验真理的唯一途径。上一篇文章出来,有人建议我出个代码讲解、具体实现方式。正好本人也要实验,所以我打算这一篇文章给大家详细讲解一下使用范围最广的Soft Attention以及Self Attention的代码实现,主要包括TensorFlow Addons、Keras封装函数以及自建网络三种形式,其他Attention模型的具体实现其实都差不太多,具体代码可以自行百度。
工具简介
本文主要采用的是TensorFlow、Keras以及tensorflow-addons等python库。
TensorFlow
TensorFlow 是一个端到端开源机器学习(主要是深度学习)平台。它拥有一个全面而灵活的生态系统,其中包含各种工具、库和社区资源,可助力研究人员推动先进机器学习技术的发展,并使开发者能够轻松地构建和部署由机器学习提供支持的应用。截止目前,最新版本为v2.6.0。
官网API:https://tensorflow.google.cn/api_docs/python/tf
Keras
Keras是一个由Python编写的开源人工神经网络库,可以作为Tensorflow、Microsoft-CNTK和Theano的高阶应用程序接口,进行深度学习模型的设计、调试、评估、应用和可视化。在TF2.x中可作为TensorFlow的高层API,并同步更新版本。
官网API:https://keras.io/zh/
tensorflow-addons
在TensorFlow2.x版本引入了 Special Interest Group (SIG),特殊兴趣小组,主要实现新发布论文中的算法。
TensorFlow2.x将tf.contrib移除,许多功能转移到了第三方库,而TensorFlow Addons 是一个符合完善的 API 模式但实现了核心 TensorFlow 中未提供的新功能的贡献仓库。TensorFlow 原生支持大量算子、层、指标、损失和优化器。但是,在像机器学习一样的快速发展领域中,有许多有趣的新开发成果无法集成到核心 TensorFlow 中(因为它们的广泛适用性尚不明确,或者主要由社区的较小子集使用)。
tensorflow-seq2seq在新版的tf中合并到tensorflow-addons中去了,除此之外tensorflow addons还有很多比较新的实现。
安装:pip install tensorflow-addons
官网API: https://tensorflow.google.cn/addons/api_docs/python/tfa
需要注意的是,tfa的版本需要和tf以及python的版本对应,不然运行会报错,具体版本对应信息看下图: 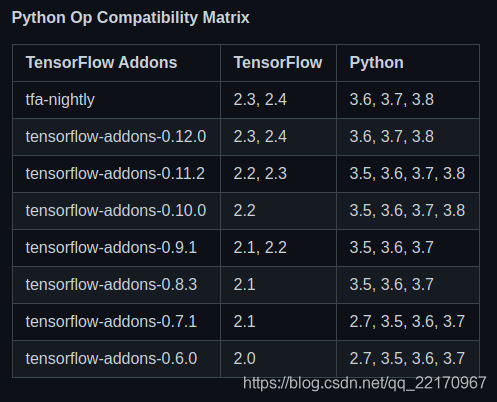
本实验所用库的版本信息
tensorflow-gpu==2.2.0
keras==2.4.3
tensorflow-addons==0.11.2
numpy==1.18.1
pandas==1.0.1
matplotlib==3.1.3
OK,工具介绍完毕,调参🦐上线!
Soft Attention
上一篇文章说到,在传统的Attention模型当中,最重要的就是生成attention score的F函数,目前为止,使用最广泛的是LuongAttention(参考3)以及BahdanauAttention(参考4),公式如下(图片来自参考1): 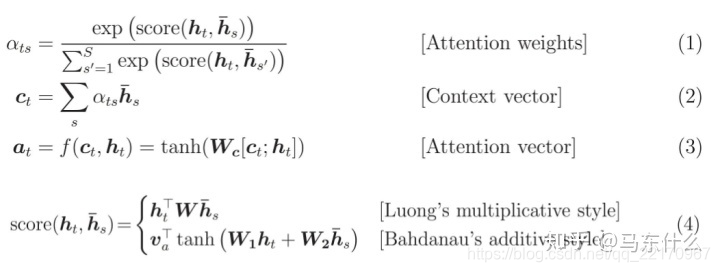 大家看公式知道了这两个的具体实现方式,其实二者的实现理念大致相同,只是实现细节上有很多区别,如图(图片来自参考2): 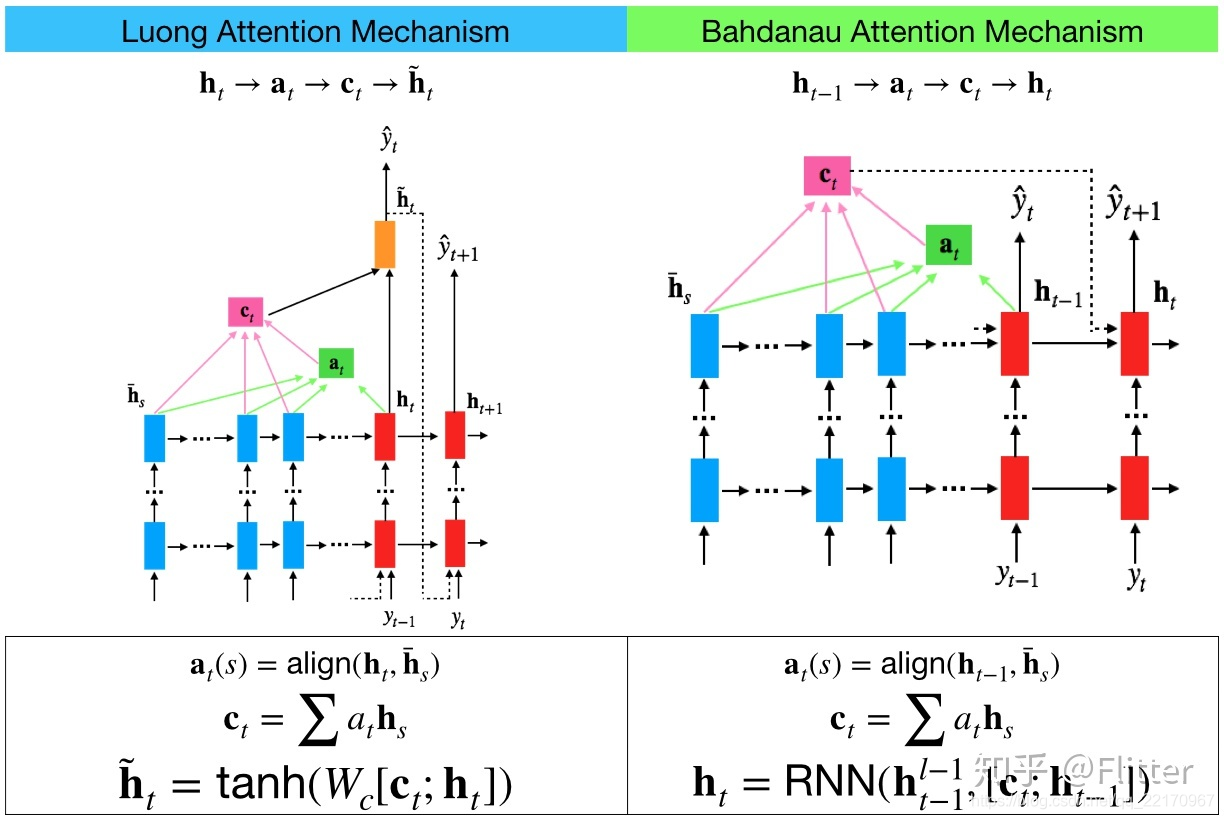 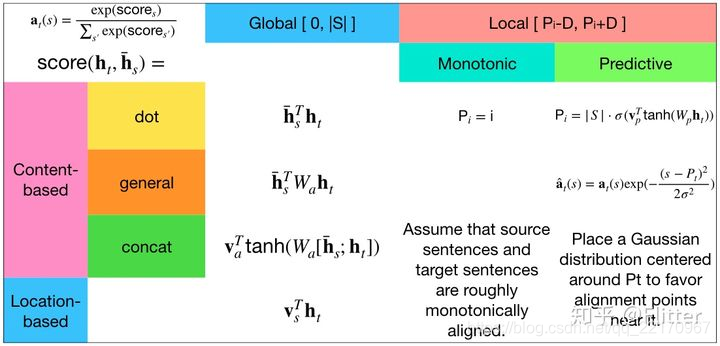
简单来说,Luong Attention 相较 Bahdanau Attention 主要有以下几点区别:
-
注意力的计算方式不同 在 Luong Attention 机制中,第 t 步的注意力
c
t
c_{t}
ct?是由 decoder 第 t 步的 hidden state
h
t
h_{t}
ht?与 encoder 中的每一个 hidden state
h
~
s
\tilde{h}_{s}
h~s? 加权计算得出的。而在 Bahdanau Attention 机制中,第 t 步的注意力
c
t
c_{t}
ct?是由 decoder 第 t-1 步的 hidden state
h
t
?
1
h_{t-1}
ht?1? 与 encoder 中的每一个 hidden state
h
~
s
\tilde{h}_{s}
h~s?加权计算得出的。 -
decoder 的输入输出不同 在 Bahdanau Attention 机制中,decoder 在第 t 步时,输入是由注意力
c
t
c_{t}
ct?与前一步的 hidden state
h
t
?
1
h_{t-1}
ht?1? 拼接(concat方式,如上图)得出的,得到第 t 步的 hidden state
h
t
h_{t}
ht?并直接输出
y
t
+
1
y_{t+1}
yt+1?。而 Luong Attention 机制在 decoder 部分建立了一层额外的网络结构,以注意力
c
t
c_{t}
ct?与原 decoder 第 t 步的 hidden state
h
t
h_{t}
ht? 拼接作为输入,得到第 t 步的 hidden state
h
~
t
\tilde{h}_{t}
h~t? 并输出
y
t
y_{t}
yt? 。
tfa的实现
在tfa.seq2seq中,对这两种常用的attention score已经进行了很好的封装,通过查看源码,我们可知:
LuongAttention
def _luong_score(query, keys, scale):
"""Implements Luong-style (multiplicative) scoring function.
This attention has two forms. The first is standard Luong attention,
as described in:
Minh-Thang Luong, Hieu Pham, Christopher D. Manning.
"Effective Approaches to Attention-based Neural Machine Translation."
EMNLP 2015. https://arxiv.org/abs/1508.04025
The second is the scaled form inspired partly by the normalized form of
Bahdanau attention.
To enable the second form, call this function with `scale=True`.
Args:
query: Tensor, shape `[batch_size, num_units]` to compare to keys.
keys: Processed memory, shape `[batch_size, max_time, num_units]`.
scale: the optional tensor to scale the attention score.
Returns:
A `[batch_size, max_time]` tensor of unnormalized score values.
Raises:
ValueError: If `key` and `query` depths do not match.
"""
depth = query.shape[-1]
key_units = keys.shape[-1]
if depth != key_units:
raise ValueError(
"Incompatible or unknown inner dimensions between query and keys. "
"Query (%s) has units: %s. Keys (%s) have units: %s. "
"Perhaps you need to set num_units to the keys' dimension (%s)?"
% (query, depth, keys, key_units, key_units)
)
query = tf.expand_dims(query, 1)
score = tf.matmul(query, keys, transpose_b=True)
score = tf.squeeze(score, [1])
if scale is not None:
score = scale * score
return score
class LuongAttention(AttentionMechanism):
"""Implements Luong-style (multiplicative) attention scoring.
This attention has two forms. The first is standard Luong attention,
as described in:
Minh-Thang Luong, Hieu Pham, Christopher D. Manning.
[Effective Approaches to Attention-based Neural Machine Translation.
EMNLP 2015.](https://arxiv.org/abs/1508.04025)
The second is the scaled form inspired partly by the normalized form of
Bahdanau attention.
To enable the second form, construct the object with parameter
`scale=True`.
"""
@typechecked
def __init__(
self,
units: TensorLike,
memory: Optional[TensorLike] = None,
memory_sequence_length: Optional[TensorLike] = None,
scale: bool = False,
probability_fn: str = "softmax",
dtype: AcceptableDTypes = None,
name: str = "LuongAttention",
**kwargs,
):
"""Construct the AttentionMechanism mechanism.
Args:
units: The depth of the attention mechanism.
memory: The memory to query; usually the output of an RNN encoder.
This tensor should be shaped `[batch_size, max_time, ...]`.
memory_sequence_length: (optional): Sequence lengths for the batch
entries in memory. If provided, the memory tensor rows are masked
with zeros for values past the respective sequence lengths.
scale: Python boolean. Whether to scale the energy term.
probability_fn: (optional) string, the name of function to convert
the attention score to probabilities. The default is `softmax`
which is `tf.nn.softmax`. Other options is `hardmax`, which is
hardmax() within this module. Any other value will result
intovalidation error. Default to use `softmax`.
dtype: The data type for the memory layer of the attention mechanism.
name: Name to use when creating ops.
**kwargs: Dictionary that contains other common arguments for layer
creation.
"""
self.probability_fn_name = probability_fn
probability_fn = self._process_probability_fn(self.probability_fn_name)
def wrapped_probability_fn(score, _):
return probability_fn(score)
memory_layer = kwargs.pop("memory_layer", None)
if not memory_layer:
memory_layer = tf.keras.layers.Dense(
units, name="memory_layer", use_bias=False, dtype=dtype
)
self.units = units
self.scale = scale
self.scale_weight = None
super().__init__(
memory=memory,
memory_sequence_length=memory_sequence_length,
query_layer=None,
memory_layer=memory_layer,
probability_fn=wrapped_probability_fn,
name=name,
dtype=dtype,
**kwargs,
)
def build(self, input_shape):
super().build(input_shape)
if self.scale and self.scale_weight is None:
self.scale_weight = self.add_weight(
"attention_g", initializer=tf.ones_initializer, shape=()
)
self.built = True
def _calculate_attention(self, query, state):
"""Score the query based on the keys and values.
Args:
query: Tensor of dtype matching `self.values` and shape
`[batch_size, query_depth]`.
state: Tensor of dtype matching `self.values` and shape
`[batch_size, alignments_size]`
(`alignments_size` is memory's `max_time`).
Returns:
alignments: Tensor of dtype matching `self.values` and shape
`[batch_size, alignments_size]` (`alignments_size` is memory's
`max_time`).
next_state: Same as the alignments.
"""
score = _luong_score(query, self.keys, self.scale_weight)
alignments = self.probability_fn(score, state)
next_state = alignments
return alignments, next_state
def get_config(self):
config = {
"units": self.units,
"scale": self.scale,
"probability_fn": self.probability_fn_name,
}
base_config = super().get_config()
return {**base_config, **config}
@classmethod
def from_config(cls, config, custom_objects=None):
config = AttentionMechanism.deserialize_inner_layer_from_config(
config, custom_objects=custom_objects
)
return cls(**config)
从源码中我们不难找到score的具体计算方式:
def _luong_score(query, keys, scale):
'''前面代码省略,只看score计算代码'''
score = tf.matmul(query, keys, transpose_b=True)
score = tf.squeeze(score, [1])
if scale is not None:
score = scale * score
return score
class LuongAttention(AttentionMechanism):
'''代码省略'''
score = _luong_score(query, self.keys, self.scale_weight)
alignments = self.probability_fn(score, state)
next_state = alignments
return alignments, next_state
'''代码省略'''
使用方式:
tfa.seq2seq.LuongAttention(
units: tfa.types.TensorLike,
memory: Optional[TensorLike] = None,
memory_sequence_length: Optional[TensorLike] = None,
scale: bool = False,
probability_fn: str = 'softmax',
dtype: tfa.types.AcceptableDTypes = None,
name: str = 'LuongAttention',
**kwargs
)
需要说明几点:
- units参数:
神经元节点数,我们知道在计算score的时候,需要使用 Decoder的
h
t
?
1
h_{t-1}
ht?1? 或
h
t
h_{t}
ht? 和Encoder的
h
~
s
\tilde{h}_{s}
h~s? 来进行计算,而二者的维度可能并不是统一的,需要进行变换和统一,所以这里就有了 Wa 和 Ua 这两个系数,所以在代码中就是用 units 来声明了一个全连接 Dense 网络,用于统一二者的维度,以便于下一步的计算,从代码我们可以看到:
memory_layer = kwargs.pop("memory_layer", None)
if not memory_layer:
memory_layer = tf.keras.layers.Dense(
units, name="memory_layer", use_bias=False, dtype=dtype
)
- scale参数:
源码已经做出了解释:The second is the scaled form inspired partly by the normalized form of Bahdanau attention.To enable the second form, construct the object with parameter scale=True .
if self.scale and self.scale_weight is None:
self.scale_weight = self.add_weight(
"attention_g", initializer=tf.ones_initializer, shape=()
)
Bahdanau Attention
Bahdanau Attention和LuongAttention的区别上面已经说明了,接下来直接上源码:
def _bahdanau_score(
processed_query, keys, attention_v, attention_g=None, attention_b=None
):
"""Implements Bahdanau-style (additive) scoring function.
This attention has two forms. The first is Bahdanau attention,
as described in:
Dzmitry Bahdanau, Kyunghyun Cho, Yoshua Bengio.
"Neural Machine Translation by Jointly Learning to Align and Translate."
ICLR 2015. https://arxiv.org/abs/1409.0473
The second is the normalized form. This form is inspired by the
weight normalization article:
Tim Salimans, Diederik P. Kingma.
"Weight Normalization: A Simple Reparameterization to Accelerate
Training of Deep Neural Networks."
https://arxiv.org/abs/1602.07868
To enable the second form, set please pass in attention_g and attention_b.
Args:
processed_query: Tensor, shape `[batch_size, num_units]` to compare to
keys.
keys: Processed memory, shape `[batch_size, max_time, num_units]`.
attention_v: Tensor, shape `[num_units]`.
attention_g: Optional scalar tensor for normalization.
attention_b: Optional tensor with shape `[num_units]` for normalization.
Returns:
A `[batch_size, max_time]` tensor of unnormalized score values.
"""
processed_query = tf.expand_dims(processed_query, 1)
if attention_g is not None and attention_b is not None:
normed_v = (
attention_g
* attention_v
* tf.math.rsqrt(tf.reduce_sum(tf.square(attention_v)))
)
return tf.reduce_sum(
normed_v * tf.tanh(keys + processed_query + attention_b), [2]
)
else:
return tf.reduce_sum(attention_v * tf.tanh(keys + processed_query), [2])
class BahdanauAttention(AttentionMechanism):
"""Implements Bahdanau-style (additive) attention.
This attention has two forms. The first is Bahdanau attention,
as described in:
Dzmitry Bahdanau, Kyunghyun Cho, Yoshua Bengio.
"Neural Machine Translation by Jointly Learning to Align and Translate."
ICLR 2015. https://arxiv.org/abs/1409.0473
The second is the normalized form. This form is inspired by the
weight normalization article:
Tim Salimans, Diederik P. Kingma.
"Weight Normalization: A Simple Reparameterization to Accelerate
Training of Deep Neural Networks."
https://arxiv.org/abs/1602.07868
To enable the second form, construct the object with parameter
`normalize=True`.
"""
@typechecked
def __init__(
self,
units: TensorLike,
memory: Optional[TensorLike] = None,
memory_sequence_length: Optional[TensorLike] = None,
normalize: bool = False,
probability_fn: str = "softmax",
kernel_initializer: Initializer = "glorot_uniform",
dtype: AcceptableDTypes = None,
name: str = "BahdanauAttention",
**kwargs,
):
"""Construct the Attention mechanism.
Args:
units: The depth of the query mechanism.
memory: The memory to query; usually the output of an RNN encoder.
This tensor should be shaped `[batch_size, max_time, ...]`.
memory_sequence_length: (optional): Sequence lengths for the batch
entries in memory. If provided, the memory tensor rows are masked
with zeros for values past the respective sequence lengths.
normalize: Python boolean. Whether to normalize the energy term.
probability_fn: (optional) string, the name of function to convert
the attention score to probabilities. The default is `softmax`
which is `tf.nn.softmax`. Other options is `hardmax`, which is
hardmax() within this module. Any other value will result into
validation error. Default to use `softmax`.
kernel_initializer: (optional), the name of the initializer for the
attention kernel.
dtype: The data type for the query and memory layers of the attention
mechanism.
name: Name to use when creating ops.
**kwargs: Dictionary that contains other common arguments for layer
creation.
"""
self.probability_fn_name = probability_fn
probability_fn = self._process_probability_fn(self.probability_fn_name)
def wrapped_probability_fn(score, _):
return probability_fn(score)
query_layer = kwargs.pop("query_layer", None)
if not query_layer:
query_layer = tf.keras.layers.Dense(
units, name="query_layer", use_bias=False, dtype=dtype
)
memory_layer = kwargs.pop("memory_layer", None)
if not memory_layer:
memory_layer = tf.keras.layers.Dense(
units, name="memory_layer", use_bias=False, dtype=dtype
)
self.units = units
self.normalize = normalize
self.kernel_initializer = tf.keras.initializers.get(kernel_initializer)
self.attention_v = None
self.attention_g = None
self.attention_b = None
super().__init__(
memory=memory,
memory_sequence_length=memory_sequence_length,
query_layer=query_layer,
memory_layer=memory_layer,
probability_fn=wrapped_probability_fn,
name=name,
dtype=dtype,
**kwargs,
)
def build(self, input_shape):
super().build(input_shape)
if self.attention_v is None:
self.attention_v = self.add_weight(
"attention_v",
[self.units],
dtype=self.dtype,
initializer=self.kernel_initializer,
)
if self.normalize and self.attention_g is None and self.attention_b is None:
self.attention_g = self.add_weight(
"attention_g",
initializer=tf.constant_initializer(math.sqrt(1.0 / self.units)),
shape=(),
)
self.attention_b = self.add_weight(
"attention_b", shape=[self.units], initializer=tf.zeros_initializer()
)
self.built = True
def _calculate_attention(self, query, state):
"""Score the query based on the keys and values.
Args:
query: Tensor of dtype matching `self.values` and shape
`[batch_size, query_depth]`.
state: Tensor of dtype matching `self.values` and shape
`[batch_size, alignments_size]`
(`alignments_size` is memory's `max_time`).
Returns:
alignments: Tensor of dtype matching `self.values` and shape
`[batch_size, alignments_size]` (`alignments_size` is memory's
`max_time`).
next_state: same as alignments.
"""
processed_query = self.query_layer(query) if self.query_layer else query
score = _bahdanau_score(
processed_query,
self.keys,
self.attention_v,
attention_g=self.attention_g,
attention_b=self.attention_b,
)
alignments = self.probability_fn(score, state)
next_state = alignments
return alignments, next_state
def get_config(self):
config = {
"units": self.units,
"normalize": self.normalize,
"probability_fn": self.probability_fn_name,
"kernel_initializer": tf.keras.initializers.serialize(
self.kernel_initializer)
}
base_config = super().get_config()
return {**base_config, **config}
@classmethod
def from_config(cls, config, custom_objects=None):
config = AttentionMechanism.deserialize_inner_layer_from_config(
config, custom_objects=custom_objects
)
return cls(**config)
区别主要在于score的求解方式不一样:
processed_query = tf.expand_dims(processed_query, 1)
if attention_g is not None and attention_b is not None:
normed_v = (
attention_g
* attention_v
* tf.math.rsqrt(tf.reduce_sum(tf.square(attention_v)))
)
return tf.reduce_sum(
normed_v * tf.tanh(keys + processed_query + attention_b), [2]
)
else:
return tf.reduce_sum(attention_v * tf.tanh(keys + processed_query),
方法参数大致差不多,在这里就不多说了。
那么该怎么用呢?tf官网还是相当体贴的,给了大家一个完整的案例分析,大家看看就知道啦!
官网使用示例
https://tensorflow.google.cn/addons/tutorials/networks_seq2seq_nmt
class Encoder(tf.keras.Model):
def __init__(self, vocab_size, embedding_dim, enc_units, batch_sz):
super(Encoder, self).__init__()
self.batch_sz = batch_sz
self.enc_units = enc_units
self.embedding = tf.keras.layers.Embedding(vocab_size, embedding_dim)
self.lstm_layer = tf.keras.layers.LSTM(self.enc_units,
return_sequences=True,
return_state=True,
recurrent_initializer='glorot_uniform')
def call(self, x, hidden):
x = self.embedding(x)
output, h, c = self.lstm_layer(x, initial_state = hidden)
return output, h, c
def initialize_hidden_state(self):
return [tf.zeros((self.batch_sz, self.enc_units)), tf.zeros((self.batch_sz, self.enc_units))]
Encoder代码不难理解,就是搭建了一层词嵌入层和LSTM网络层。重点是Decoder的预测输出,官网案例是这么写的:
class Decoder(tf.keras.Model):
def __init__(self, vocab_size, embedding_dim, dec_units, batch_sz, attention_type='luong'):
super(Decoder, self).__init__()
self.batch_sz = batch_sz
self.dec_units = dec_units
self.attention_type = attention_type
self.embedding = tf.keras.layers.Embedding(vocab_size, embedding_dim)
self.fc = tf.keras.layers.Dense(vocab_size)
self.decoder_rnn_cell = tf.keras.layers.LSTMCell(self.dec_units)
self.sampler = tfa.seq2seq.sampler.TrainingSampler()
self.attention_mechanism = self.build_attention_mechanism(self.dec_units,
None, self.batch_sz*[max_length_input], self.attention_type)
self.rnn_cell = self.build_rnn_cell(batch_sz)
self.decoder = tfa.seq2seq.BasicDecoder(self.rnn_cell, sampler=self.sampler, output_layer=self.fc)
def build_rnn_cell(self, batch_sz):
rnn_cell = tfa.seq2seq.AttentionWrapper(self.decoder_rnn_cell,
self.attention_mechanism, attention_layer_size=self.dec_units)
return rnn_cell
def build_attention_mechanism(self, dec_units, memory, memory_sequence_length, attention_type='luong'):
if(attention_type=='bahdanau'):
return tfa.seq2seq.BahdanauAttention(units=dec_units, memory=memory, memory_sequence_length=memory_sequence_length)
else:
return tfa.seq2seq.LuongAttention(units=dec_units, memory=memory, memory_sequence_length=memory_sequence_length)
def build_initial_state(self, batch_sz, encoder_state, Dtype):
decoder_initial_state = self.rnn_cell.get_initial_state(batch_size=batch_sz, dtype=Dtype)
decoder_initial_state = decoder_initial_state.clone(cell_state=encoder_state)
return decoder_initial_state
def call(self, inputs, initial_state):
x = self.embedding(inputs)
outputs, _, _ = self.decoder(x, initial_state=initial_state, sequence_length=self.batch_sz*[max_length_output-1])
return outputs
这代码看的是不是有点头疼,哈哈哈,反正我一开始看的是云里雾里的,所以我又好好分析了下源码,发现里面竟然已经提供了一个简单案例(大佬们真为我们着想啊,爱了爱了)。
下面是我自己从源码找的一个简单例子:
>>> batch_size = 4
>>> max_time = 7
>>> hidden_size = 32
>>>
>>> memory = tf.random.uniform([batch_size, max_time, hidden_size])
>>> memory_sequence_length = tf.fill([batch_size], max_time)
>>>
>>> attention_mechanism = tfa.seq2seq.LuongAttention(hidden_size)
>>> attention_mechanism.setup_memory(memory, memory_sequence_length)
>>>
>>> cell = tf.keras.layers.LSTMCell(hidden_size)
>>> cell = tfa.seq2seq.AttentionWrapper(
... cell, attention_mechanism, attention_layer_size=hidden_size)
>>>
>>> inputs = tf.random.uniform([batch_size, hidden_size])
>>> state = cell.get_initial_state(inputs)
>>>
>>> outputs, state = cell(inputs, state)
>>> outputs.shape
TensorShape([4, 32])
看完这个顿时通了,再转回去看官网提供的Decoder案例,大师,我悟了!
Keras的实现
啊啊啊啊,没想到一个tfa讲解就占了我这么大的篇幅。
keras其实也有自己的一套soft attention实现方式。Keras主要提供了两个方法:
- Luong-style attention
tf.keras.layers.Attention(use_scale=False, **kwargs ) - Bahdanau-style attention
tf.keras.layers.AdditiveAttention(use_scale=True, **kwargs )
(你说你都归tf所有了,咋还自立门户呢?这就是所谓的得到了你的人却得不到你的心吧,哈哈哈哈)
keras也很贴心的给出了实现案例:
query_input = tf.keras.Input(shape=(None,), dtype='int32')
value_input = tf.keras.Input(shape=(None,), dtype='int32')
token_embedding = tf.keras.layers.Embedding(max_tokens, dimension)
query_embeddings = token_embedding(query_input)
value_embeddings = token_embedding(value_input)
cnn_layer = tf.keras.layers.Conv1D(
filters=100,
kernel_size=4,
padding='same')
query_seq_encoding = cnn_layer(query_embeddings)
value_seq_encoding = cnn_layer(value_embeddings)
query_value_attention_seq = tf.keras.layers.AdditiveAttention()(
[query_seq_encoding, value_seq_encoding])
query_encoding = tf.keras.layers.GlobalAveragePooling1D()(
query_seq_encoding)
query_value_attention = tf.keras.layers.GlobalAveragePooling1D()(
query_value_attention_seq)
input_layer = tf.keras.layers.Concatenate()(
[query_encoding, query_value_attention])
还有一个我从网上偶然翻到的代码(参考6),不过是截图,源码地址:链接 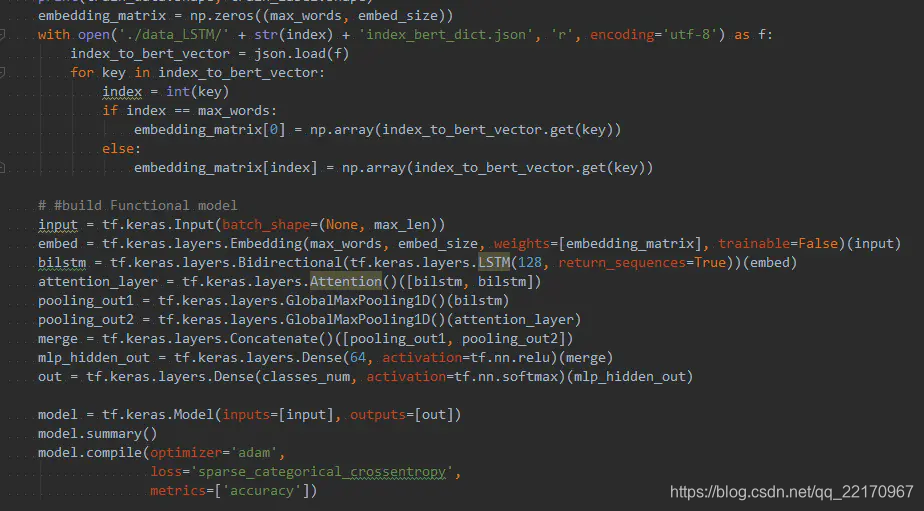
Self Attention
翻遍了tfa和keras的的API文档,我都没有找到self attention的实现。难道是因为这个还没入tf的法眼?还是因为过于简单就没封装?搞不懂这些大佬的脑回路了,也可能是在别的地方实现了,只是我不知道而已?🤔🤔
希望知道的朋友可以在评论区说下,感谢🙏!
既然这样,那我就给大家分享一下我从博客上看的一个self attention自建网络的实验吧(参考7),在大佬的基础上,我自己又稍微修改了一下。
关于如何自定义Keras可以参看这里:链接
Keras实现自定义网络层。需要实现以下三个方法:(注意input_shape是包含batch_size项的)
- build(input_shape): 这是你定义权重的地方。这个方法必须设 self.built = True,可以通过调用 super([Layer], self).build() 完成。
- call(x): 这里是编写层的功能逻辑的地方。你只需要关注传入 call 的第一个参数:输入张量,除非你希望你的层支持masking。
- compute_output_shape(input_shape): 如果你的层更改了输入张量的形状,你应该在这里定义形状变化的逻辑,这让Keras能够自动推断各层的形状。
本实验使用的是Keras自带的电影评论情感分类数据集imdb数据集,库版本在一开始已经说了,上代码:
from keras.preprocessing import sequence
from keras.datasets import imdb
from matplotlib import pyplot as plt
import pandas as pd
import numpy as np
from keras import backend as K
from keras.engine.topology import Layer
Self Attention的构建:
class Self_Attention(Layer):
def __init__(self, output_dim, **kwargs):
self.output_dim = output_dim
super(Self_Attention, self).__init__(**kwargs)
def build(self, input_shape):
self.kernel = self.add_weight(name='kernel',
shape=(3,input_shape[2], self.output_dim),
initializer='uniform',
trainable=True)
print("attention input:",input_shape[0],input_shape[1],input_shape[2])
super(Self_Attention, self).build(input_shape)
def call(self, x):
WQ = K.dot(x, self.kernel[0])
WK = K.dot(x, self.kernel[1])
WV = K.dot(x, self.kernel[2])
print("WQ.shape",WQ.shape)
print("K.permute_dimensions(WK, [0, 2, 1]).shape", K.permute_dimensions(WK, [0,2,1]).shape)
QK = K.batch_dot(WQ, K.permute_dimensions(WK, [0, 2, 1]))
QK = QK / (self.output_dim**0.5)
QK = K.softmax(QK)
print("QK.shape", QK.shape)
V = K.batch_dot(QK, WV)
return V
def compute_output_shape(self, input_shape):
return (input_shape[0], input_shape[1], self.output_dim)
上面代码的原理在上一篇我都有说明,也不难理解,需要的可以看我上一篇文章。
多说一句,dot、matmul和multiply的区别,可参考链接:
- multiply:如果两个维度完全一样的矩阵用multiply做乘法,那么它们只是进行对应位置元素之间的乘法,得到一个同样维度的矩阵输出。如果3x3的矩阵和3x1的矩阵用multiply相乘,相当于用b依次乘以a的每一行。记住,multiply是满足交换律的。(a和b互换位置结果不变)。对于3x3的矩阵a,可以用3x1的矩阵与它相乘,也可以用1x3的矩阵与它相乘。还可以用它乘以一个常数。总结:multiply需要输入矩阵x和y的shape相同或者可broadcast。
- dot:dot就是矩阵叉乘,MxN矩阵乘以NxC矩阵会得到一个MxC的矩阵。对于2D情况下的dot,等同于matmul,也等同于运算符@,
- matmul:matmul不支持标量乘法,在2D矩阵乘法中,其效果与dot一样。在N维矩阵乘法中(N>=3),体现出与dot不一样的算法。
>>> a = np.ones([9, 5, 7, 4])
>>> c = np.ones([9, 5, 4, 3])
>>> np.dot(a, c).shape
(9, 5, 7, 9, 5, 3)
>>> np.matmul(a, c).shape
(9, 5, 7, 3)
扯远了扯远了,继续,self attention写好了,那么我们就开始实验吧!
max_features = 2000
print('Loading data...')
(x_train, y_train), (x_test, y_test) = imdb.load_data(num_words=max_features)
print('Loading data finish!')
y_train, y_test = pd.get_dummies(y_train),pd.get_dummies(y_test)
print(x_train.shape, 'train sequences')
print(x_test.shape, 'test sequences')
maxlen = 128
batch_size = 32
x_train = sequence.pad_sequences(x_train, maxlen=maxlen)
x_test = sequence.pad_sequences(x_test, maxlen=maxlen)
print('x_train shape:', x_train.shape)
print('x_test shape:', x_test.shape)
'''
output:
(25000,) train sequences
(25000,) test sequences
x_train shape: (25000, 128)
x_test shape: (25000, 128)
'''
from keras.models import Model
from keras.optimizers import SGD,Adam
from keras.layers import *
S_inputs = Input(shape=(maxlen,), dtype='int32')
embeddings = Embedding(max_features, 128)(S_inputs)
O_seq = Self_Attention(128)(embeddings)
O_seq = GlobalAveragePooling1D()(O_seq)
O_seq = Dropout(0.5)(O_seq)
outputs = Dense(2, activation='softmax')(O_seq)
model = Model(inputs=S_inputs, outputs=outputs)
print(model.summary())
opt = Adam(lr=0.0002,decay=0.00001)
loss = 'categorical_crossentropy'
model.compile(loss=loss,optimizer=opt,metrics=['accuracy'])
print('Train start......')
h = model.fit(x_train, y_train,
batch_size=batch_size,
epochs=5,
validation_data=(x_test, y_test))
plt.plot(h.history["loss"],label="train_loss")
plt.plot(h.history["val_loss"],label="val_loss")
plt.plot(h.history["accuracy"],label="train_acc")
plt.plot(h.history["val_accuracy"],label="val_acc")
plt.legend()
plt.show()
代码不难理解,我就不多废话了,先看看执行过程。 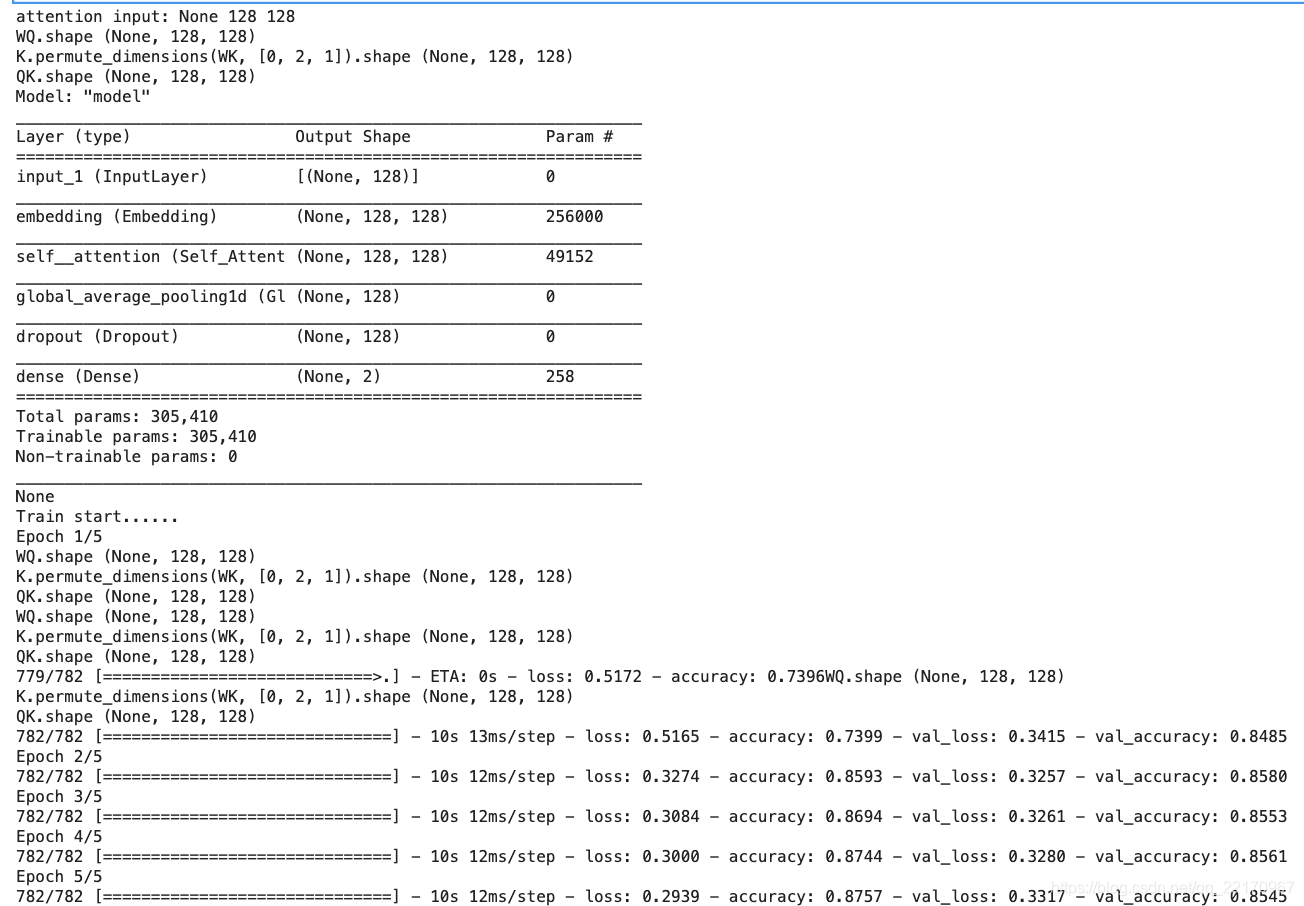 最后给大家看一下误差,准确率的趋势图。 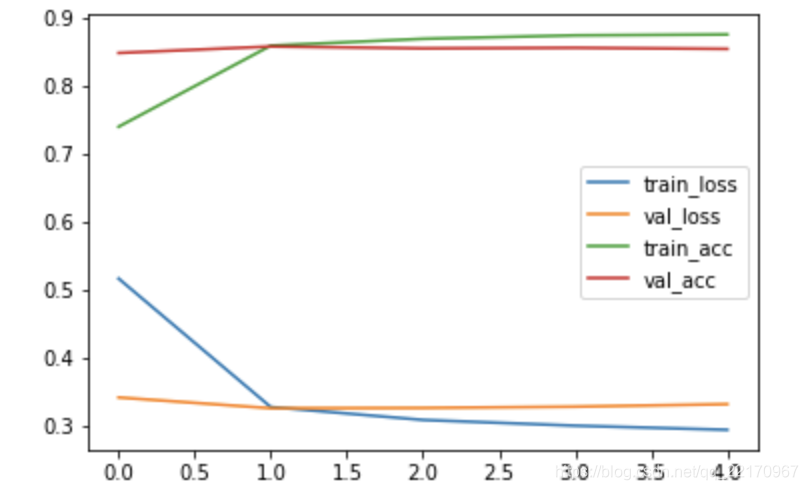 这也就可以当成练习了,起不到什么实际作用😂,大家看看就好啦!
如果大家知道更好的self attention模型或者tf已经封装了,麻烦告诉我一下,感谢!
为什么self attention要scaled?
对了,上一篇忘了说了,大家有没有想过,为什么self attention在求attention权重占比的时候需要scaled?为什么使用维度的根号来放缩?为什么一般的NN最后一层输出函数中的softmax不需要scaled呢?我大概说一下,具体请查看参考8。主要在以下几点:
- 比较大的输入会使得softmax的梯度变得很小,数量级对softmax得到的分布影响非常大。在数量级较大时,softmax将几乎全部的概率分布都分配给了最大值对应的标签,如图(其实原理和批标准化(Batch Normalization,BN)差不多)
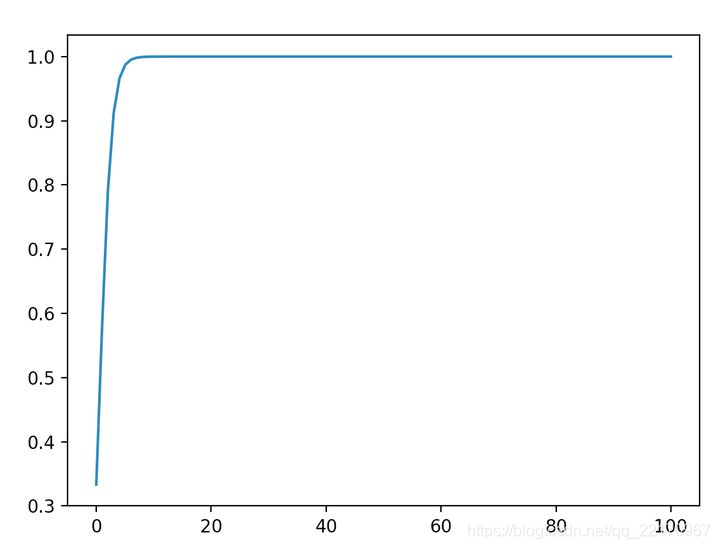 - 使用维度的根号来放缩主要是为了将方差控制为1,也就有效地控制了梯度消失的问题。
- 为什么在分类层(最后一层),使用非 scaled 的 softmax?
分类层的 softmax 也没有两个随机变量相乘的情况。此外,这一层的 softmax 通常和交叉熵联合求导,在某个目标类别 上的整体梯度变为 预测值和真值的差。当出现某个极大的元素值,softmax 的输出概率会集中在该类别上。如果是预测正确,整体梯度接近于 0,抑制参数更新;如果是错误类别,则整体梯度接近于1,给出最大程度的负反馈。 也就是说,这个时候的梯度形式改变,不会出现极大值导致梯度消失的情况了。还有人说是因为离监督信号(即输出结果)很近,不必过多关照;而且只有这一层,不像transformer block 一样堆很多层,引起累积效应。
总结
啊啊啊啊,码字不易,长亮叹气😮?💨😮?💨😮?💨,不知不觉快3w字了,肝了一天,发现自己也是够拼的😂,终于到最后了。Attention作为目前非常流行的一个模型,如果不知道怎么用,那就真的太可惜了,希望我这篇文章可以为大家接下来的各自实验提供一个思路和解决方案😊!本人水平有限,有不对的地方欢迎大家批评指正!
对了对了,吃水不忘挖井人,感谢各位大佬分享的精彩文章,链接我均附在参考当中,再次感谢🙏!
彩蛋
有人评论说想知道Word2Vec具体是什么,该怎么用,👌,No problem!拿捏!下一篇写什么,不用我明说了吧!😉😉😉
参考
- https://zhuanlan.zhihu.com/p/336659232
- https://zhuanlan.zhihu.com/p/129316415
- Minh-Thang Luong, Hieu Pham, Christopher D. Manning.Effective Approaches to Attention-based Neural Machine Translation. EMNLP 2015.(https://arxiv.org/abs/1508.04025)
- Dzmitry Bahdanau, Kyunghyun Cho, Yoshua Bengio. “Neural Machine Translation by Jointly Learning to Align and Translate.” ICLR 2015. https://arxiv.org/abs/1409.0473
- https://blog.csdn.net/hustqb/article/details/104321552
- https://www.jianshu.com/p/674865b5e31c
- https://blog.csdn.net/fkyyly/article/details/104881616?utm_source=app&app_version=4.13.0&code=app_1562916241&uLinkId=usr1mkqgl919blen
- https://www.zhihu.com/question/339723385/answer/782509914
|