七、反向传播算法
内容参考来自https://github.com/dragen1860/Deep-Learning-with-TensorFlow-book开源书籍《TensorFlow2深度学习》,这只是我做的简单的学习笔记,方便以后复习。
1.激活函数导数
1.1Sigmoid 函数导数
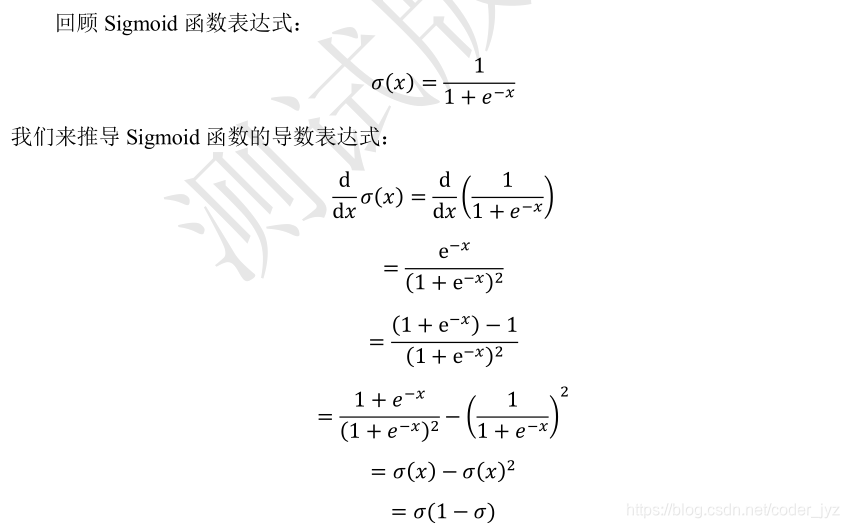 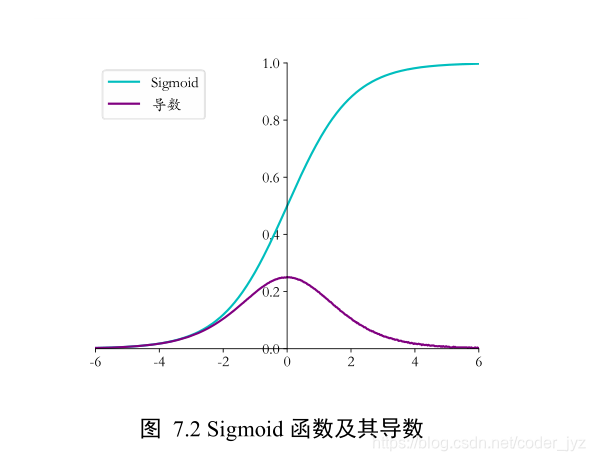
import numpy as np
def sigmoid(x):
return 1 / (1 + np.exp(-x))
def derivative(x):
return sigmoid(x)*(1-sigmoid(x))
1.2ReLU函数导数
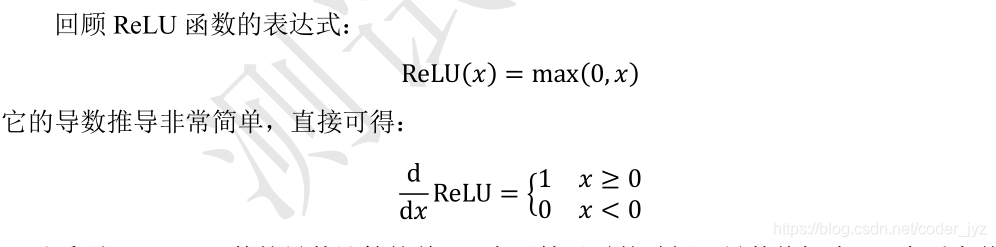 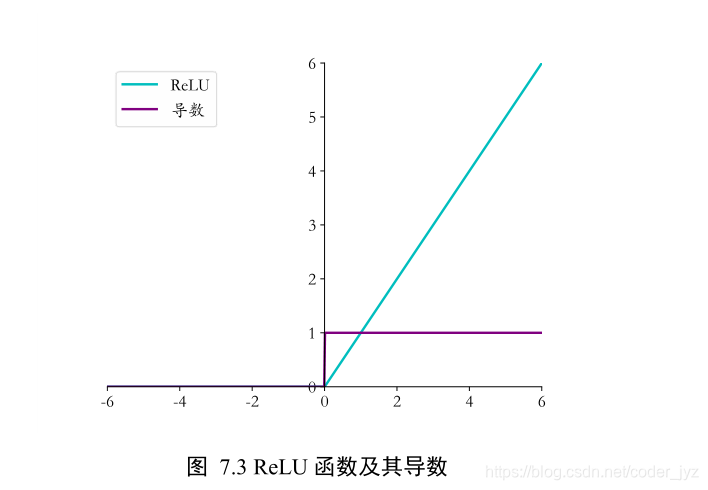
def derivative(x):
d = np.array(x, copy=True)
d[x < 0] = 0
d[x >= 0] = 1
return d
1.3LeakyReLU 函数导数
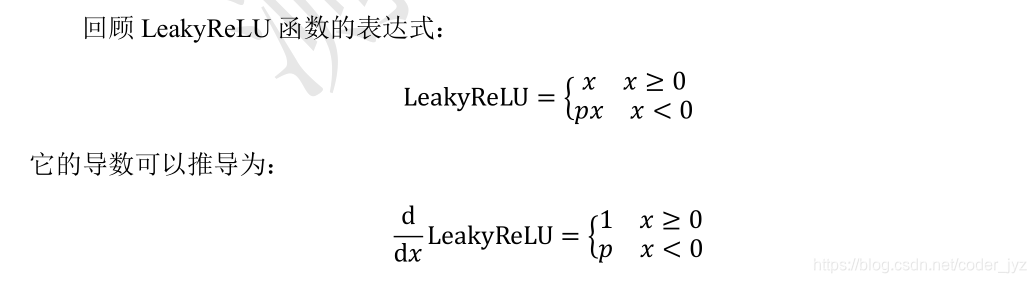
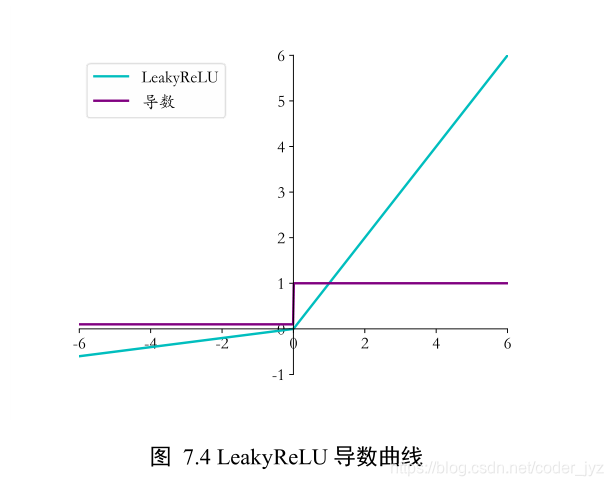
def derivative(x, p):
dx = np.ones_like(x)
dx[x < 0] = p
return dx
1.4Tanh 函数梯度
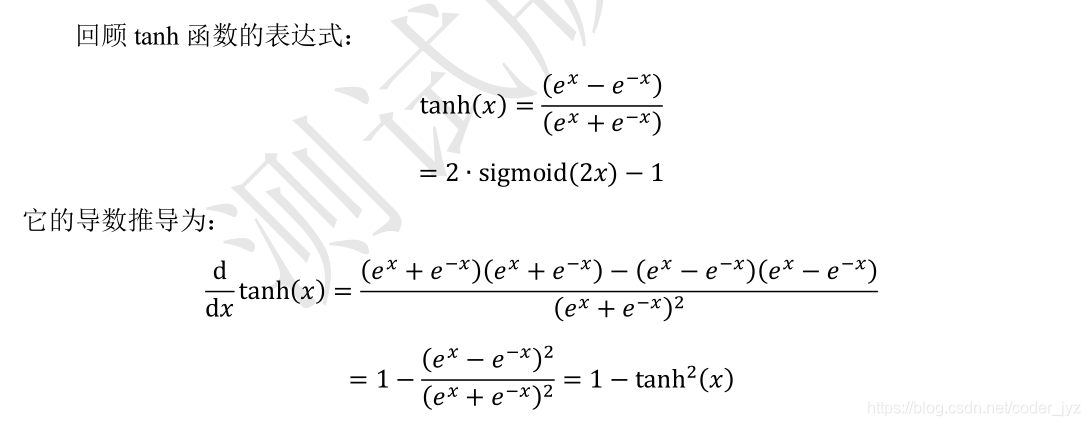 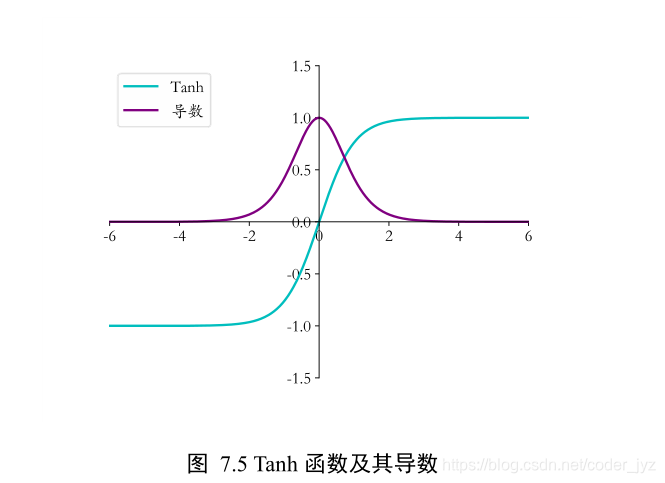
def sigmoid(x):
return 1 / (1 + np.exp(-x))
def tanh(x):
return 2*sigmoid(2*x) - 1
def derivative(x):
return 1-tanh(x)**2
2.链式法则
import tensorflow as tf
x = tf.constant(1.)
w1 = tf.constant(2.)
b1 = tf.constant(1.)
w2 = tf.constant(2.)
b2 = tf.constant(1.)
with tf.GradientTape(persistent=True) as tape:
tape.watch([w1, b1, w2, b2])
y1 = x * w1 + b1
y2 = y1 * w2 + b2
dy2_dy1 = tape.gradient(y2, [y1])[0]
dy1_dw1 = tape.gradient(y1, [w1])[0]
dy2_dw1 = tape.gradient(y2, [w1])[0]
print(dy2_dy1 * dy1_dw1)
print(dy2_dw1)
3.Himmelblau 函数优化实战
Himmelblau 函数是用来测试优化算法的常用样例函数之一,它包含了两个自变量𝑦和𝑧,数学表达式是
f
(
x
,
y
)
=
(
x
2
+
y
?
11
)
2
+
(
x
+
y
2
?
7
)
2
f(x,y)=(x^2+y-11)^2+(x+y^2-7)^2
f(x,y)=(x2+y?11)2+(x+y2?7)2
Himmelblau 函数的等高线图,大致可以看出,它共有 4 个局部极小值点,并且局部极小值都是 0,所以这 4 个局部极小值也是全局最小值。我们可以通过解析的方法计算出局部极小值的精确坐标,它们分别是:(3,2),(?2 805,3 131),(?3 779,?3 283),(3 584,?1 848)
import numpy as np
from mpl_toolkits.mplot3d import Axes3D
from matplotlib import pyplot as plt
import tensorflow as tf
def himmelblau(x):
return (x[0] ** 2 + x[1] - 11) ** 2 + (x[0] + x[1] ** 2 - 7) ** 2
x = np.arange(-6, 6, 0.1)
y = np.arange(-6, 6, 0.1)
print('x,y range:', x.shape, y.shape)
X, Y = np.meshgrid(x, y)
print('X,Y maps:', X.shape, Y.shape)
Z = himmelblau([X, Y])
fig = plt.figure('himmelblau')
ax = fig.gca(projection='3d')
ax.plot_surface(X, Y, Z)
ax.view_init(60, -30)
ax.set_xlabel('x')
ax.set_ylabel('y')
plt.show()
x = tf.constant([-2., 2.])
for step in range(200):
with tf.GradientTape() as tape:
tape.watch([x])
y = himmelblau(x)
grads = tape.gradient(y, [x])[0]
x -= 0.01*grads
if step % 20 == 19:
print ('step {}: x = {}, f(x) = {}'
.format(step, x.numpy(), y.numpy()))
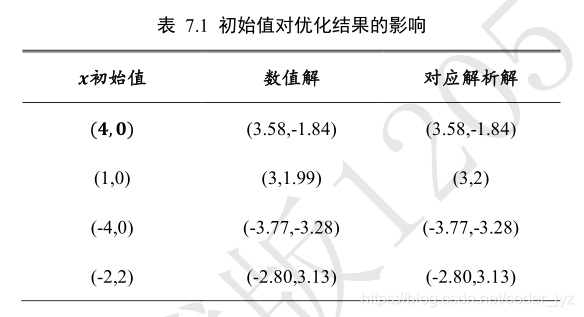 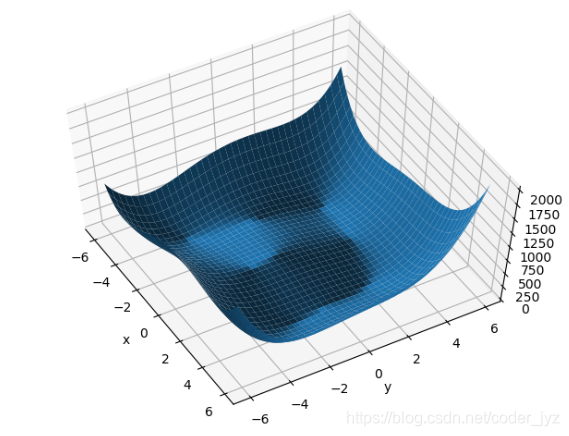
4.反向传播算法实战
"""
@author: HuRuiFeng
@file: 7.9-backward-prop.py
@time: 2020/2/24 17:32
@desc: 7.9 反向传播算法实战的代码
"""
import matplotlib.pyplot as plt
import numpy as np
import seaborn as sns
from sklearn.datasets import make_moons
from sklearn.model_selection import train_test_split
plt.rcParams['font.size'] = 16
plt.rcParams['font.family'] = ['STKaiti']
plt.rcParams['axes.unicode_minus'] = False
def load_dataset():
N_SAMPLES = 2000
TEST_SIZE = 0.3
X, y = make_moons(n_samples=N_SAMPLES, noise=0.2, random_state=100)
X_train, X_test, y_train, y_test = train_test_split(X, y, test_size=TEST_SIZE, random_state=42)
return X, y, X_train, X_test, y_train, y_test
def make_plot(X, y, plot_name, XX=None, YY=None, preds=None, dark=False):
if (dark):
plt.style.use('dark_background')
else:
sns.set_style("whitegrid")
plt.figure(figsize=(16, 12))
axes = plt.gca()
axes.set(xlabel="$x_1$", ylabel="$x_2$")
plt.title(plot_name, fontsize=30)
plt.subplots_adjust(left=0.20)
plt.subplots_adjust(right=0.80)
if XX is not None and YY is not None and preds is not None:
plt.contourf(XX, YY, preds.reshape(XX.shape), 25, alpha=1, cmap=plt.cm.Spectral)
plt.contour(XX, YY, preds.reshape(XX.shape), levels=[.5], cmap="Greys", vmin=0, vmax=.6)
plt.scatter(X[:, 0], X[:, 1], c=y.ravel(), s=40, cmap=plt.cm.Spectral, edgecolors='none')
plt.savefig('数据集分布.svg')
plt.close()
class Layer:
def __init__(self, n_input, n_neurons, activation=None, weights=None,
bias=None):
"""
:param int n_input: 输入节点数
:param int n_neurons: 输出节点数
:param str activation: 激活函数类型
:param weights: 权值张量,默认类内部生成
:param bias: 偏置,默认类内部生成
"""
self.weights = weights if weights is not None else np.random.randn(n_input, n_neurons) * np.sqrt(1 / n_neurons)
self.bias = bias if bias is not None else np.random.rand(n_neurons) * 0.1
self.activation = activation
self.last_activation = None
self.error = None
self.delta = None
def activate(self, x):
r = np.dot(x, self.weights) + self.bias
self.last_activation = self._apply_activation(r)
return self.last_activation
def _apply_activation(self, r):
if self.activation is None:
return r
elif self.activation == 'relu':
return np.maximum(r, 0)
elif self.activation == 'tanh':
return np.tanh(r)
elif self.activation == 'sigmoid':
return 1 / (1 + np.exp(-r))
return r
def apply_activation_derivative(self, r):
if self.activation is None:
return np.ones_like(r)
elif self.activation == 'relu':
grad = np.array(r, copy=True)
grad[r > 0] = 1.
grad[r <= 0] = 0.
return grad
elif self.activation == 'tanh':
return 1 - r ** 2
elif self.activation == 'sigmoid':
return r * (1 - r)
return r
class NeuralNetwork:
def __init__(self):
self._layers = []
def add_layer(self, layer):
self._layers.append(layer)
def feed_forward(self, X):
for layer in self._layers:
X = layer.activate(X)
return X
def backpropagation(self, X, y, learning_rate):
output = self.feed_forward(X)
for i in reversed(range(len(self._layers))):
layer = self._layers[i]
if layer == self._layers[-1]:
layer.error = y - output
layer.delta = layer.error * layer.apply_activation_derivative(output)
else:
next_layer = self._layers[i + 1]
layer.error = np.dot(next_layer.weights, next_layer.delta)
layer.delta = layer.error * layer.apply_activation_derivative(layer.last_activation)
for i in range(len(self._layers)):
layer = self._layers[i]
o_i = np.atleast_2d(X if i == 0 else self._layers[i - 1].last_activation)
layer.weights += layer.delta * o_i.T * learning_rate
def train(self, X_train, X_test, y_train, y_test, learning_rate, max_epochs):
y_onehot = np.zeros((y_train.shape[0], 2))
y_onehot[np.arange(y_train.shape[0]), y_train] = 1
mses = []
accuracys = []
for i in range(max_epochs + 1):
for j in range(len(X_train)):
self.backpropagation(X_train[j], y_onehot[j], learning_rate)
if i % 10 == 0:
mse = np.mean(np.square(y_onehot - self.feed_forward(X_train)))
mses.append(mse)
accuracy = self.accuracy(self.predict(X_test), y_test.flatten())
accuracys.append(accuracy)
print('Epoch: #%s, MSE: %f' % (i, float(mse)))
print('Accuracy: %.2f%%' % (accuracy * 100))
return mses, accuracys
def predict(self, X):
return self.feed_forward(X)
def accuracy(self, X, y):
return np.sum(np.equal(np.argmax(X, axis=1), y)) / y.shape[0]
def main():
X, y, X_train, X_test, y_train, y_test = load_dataset()
make_plot(X, y, "Classification Dataset Visualization ")
plt.show()
nn = NeuralNetwork()
nn.add_layer(Layer(2, 25, 'sigmoid'))
nn.add_layer(Layer(25, 50, 'sigmoid'))
nn.add_layer(Layer(50, 25, 'sigmoid'))
nn.add_layer(Layer(25, 2, 'sigmoid'))
mses, accuracys = nn.train(X_train, X_test, y_train, y_test, 0.01, 1000)
x = [i for i in range(0, 101, 10)]
plt.title("MES Loss")
plt.plot(x, mses[:11], color='blue')
plt.xlabel('Epoch')
plt.ylabel('MSE')
plt.savefig('训练误差曲线.svg')
plt.close()
plt.title("Accuracy")
plt.plot(x, accuracys[:11], color='blue')
plt.xlabel('Epoch')
plt.ylabel('Accuracy')
plt.savefig('网络测试准确率.svg')
plt.close()
if __name__ == '__main__':
main()
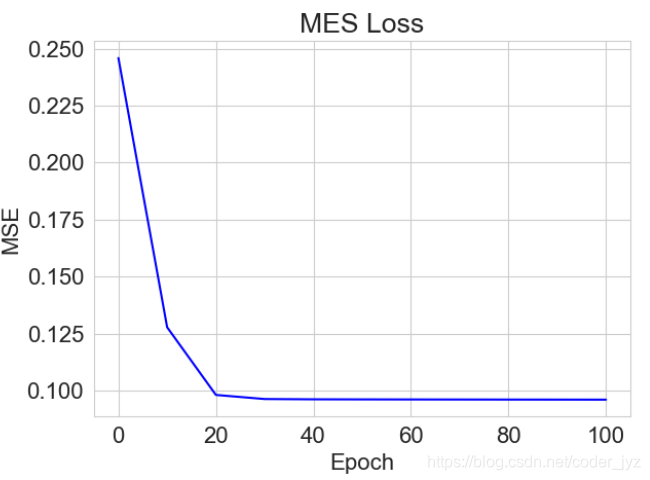 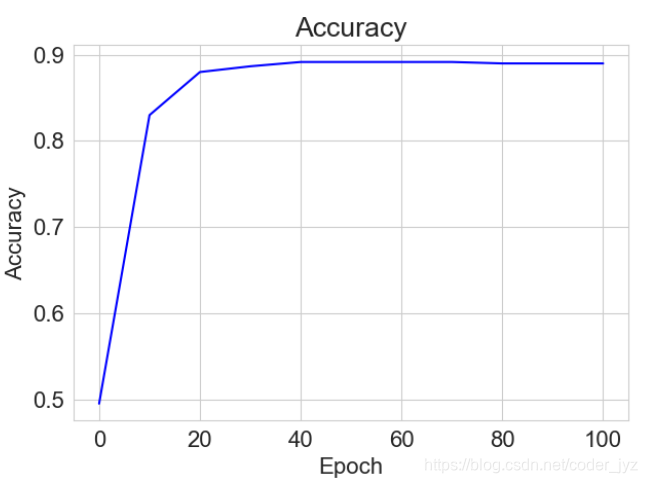
5.题外话
这章相对于书的内容我减少了很多,感觉好多都是常识性东西,还有就是比较难做笔记,就懒得弄了。我还是太懒了…
我的微信公众号,同步更新,求关注,嘻嘻 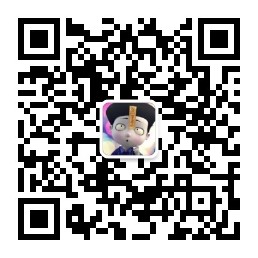
|