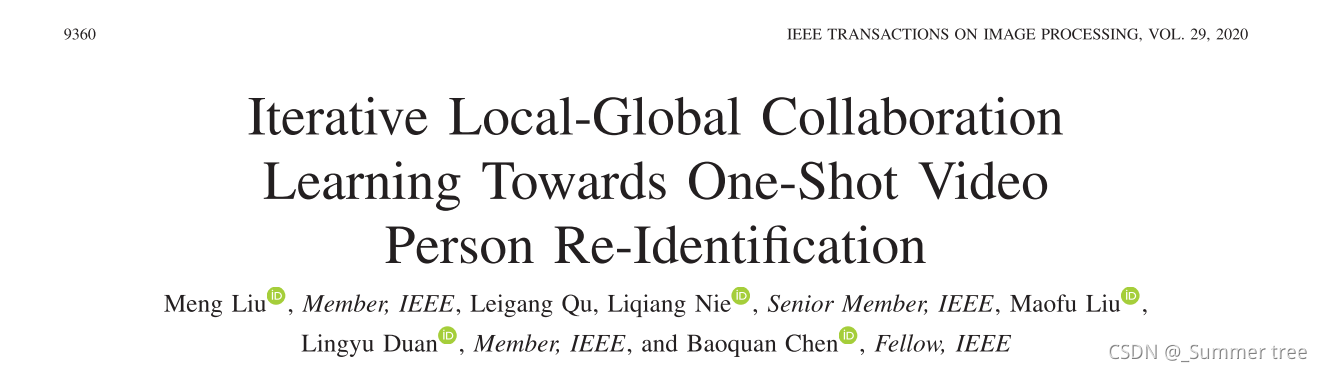
引言
- 提出了local-global 协作学习方法,用来进行标签估计
- 在损失中引入了变量信息瓶颈作为正则项。使得特征提取器能过滤掉无关因素
- 采用了和eug一样的迭代模式。
内容概要
论文名称 | 简称 | 会议/期刊 | 出版年份 | baseline | backbone | 数据集 |
---|
Iterative Local-Global Collaboration Learning Towards One-Shot Video Person Re-Identification | VOLTA | IEEE TIP | 2020 | Y. Wu, Y. Lin, X. Dong, Y. Yan, W. Bian, Y. Yang, Progressive learning for person re-identification with one example, IEEE Transactions on Image Processing 28 (6) (2019) 2872–2881 | ResNet-50 | DukeMTMC-VideoReID、MARS |
在线链接:https://ieeexplore-ieee-org-s.nudtproxy.yitlink.com/document/9211791 源码链接: https://github.com/LgQu/VOLTA
工作概述
- In this article, we focus on one-shot video Re-ID and present an iterative local-global collaboration learning approach to learn robust and discriminative person representations. Specifically, it jointly considers the global video information and local frame sequence information to better capture the diverse appearance of the person for feature learning and pseudo-label estimation.
- Moreover, as the cross-entropy loss may induce the model to focus on identity-irrelevant factors, we introduce the variational information bottleneck as a regularization term to train the model together. It can help filter undesirable information and characterize subtle differences among persons. Since accuracy cannot always be guaranteed for pseudo-labels, we adopt a dynamic selection strategy to select part of pseudo-labeled data with higher confidence to update the training set and re-train the learning model. During
成果概述
Extensive experiments on two public datasets, i.e., DukeMTMC-VideoReID and MARS, have verified the superiority of our model to several cutting-edge competitors.
方法详解
方法框架
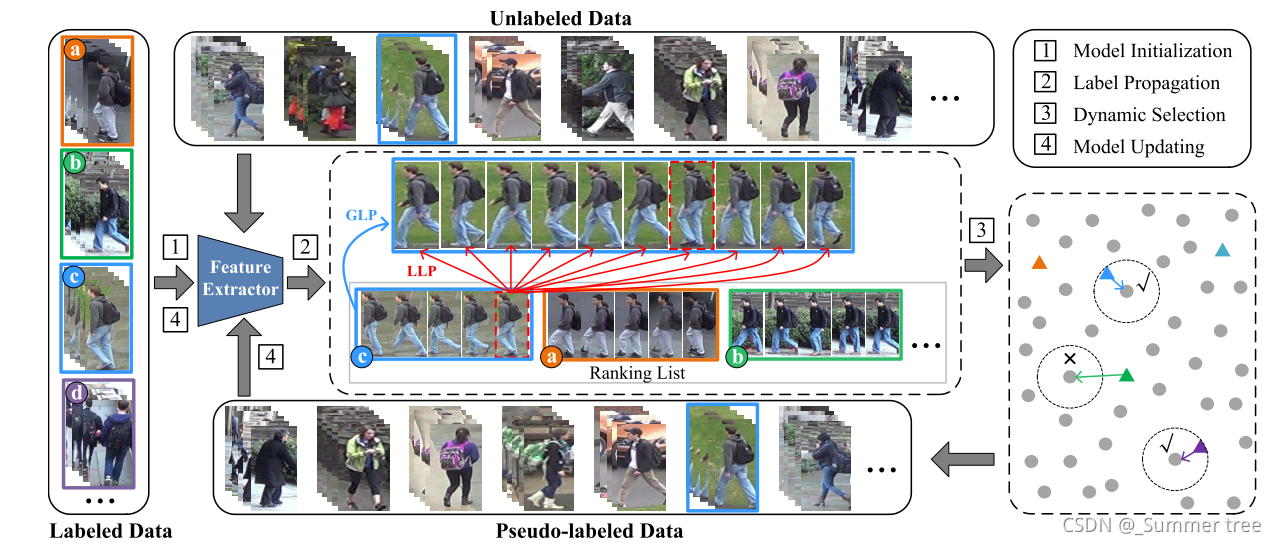
Fig. 2. The pipeline of our proposed one-shot video Re-ID approach VOLTA, comprising the following four processes: 1) Model Initialization. All the one-shot labeled video tracklets are utilized to initialize the learning model such that it has basic discrimination power. 2) Local-Global Label Propagation. We respectively utilize global and local video features to estimate the similarities between labeled and unlabeled samples, and then integrate two similarity values to predict pseudo labels for unlabeled video tracklets. 3) Dynamic Selection. Pseudo-labeled samples with higher confidence are selected to add into the training set via our dynamic threshold selection strategy. And 4) Model Update. Updating our feature learning model with the new training set. The GLP denotes the global label propagation, while the LLP denotes the local one. The bounding boxes and triangles with different colors refer to different identities. Moreover, the two red dotted boxes denote the frame pair with the highest similarity among the sequences.

Fig. 3. The framework of our feature extractor module, including the following two parts: 1) Local-Global Representation. For each video tracklet, the frame- level features are first extracted by a CNN model. The video-level feature is obtained by the global average pooling (GAP) operation. By stacking the two-level features, we could acquire the local-global feature representation. And 2) VIB-based Classification. Each column of the local-global feature is input to the VIB encoder to generate a latent vector. Afterwards, we combine the information and identity loss to train the feature learning model.
具体实现
- Local-Global 表达。取了一个T帧的平均池化,再拼接上T帧,构成T+1维的 特征表示M。
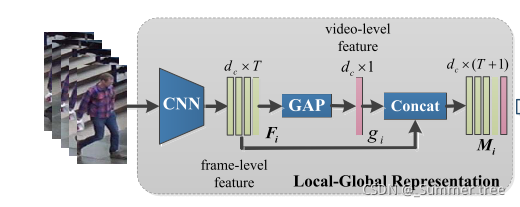
局部特征: 
全局特征:
 局部-全局特征: 
- 信息过滤 VIB(variational information bottleneck) 分类。 由身份损失Lid 和信息损失Lin两个部分组成,其中Lin是文章独特设计的,计算的是 特征Z的标注正态分布和多元高斯分布之间的KL散度。而Z是根据原始特征的均值和方差计算得出的。最后再利用Z来求交叉熵损失作为身份损失Lid。
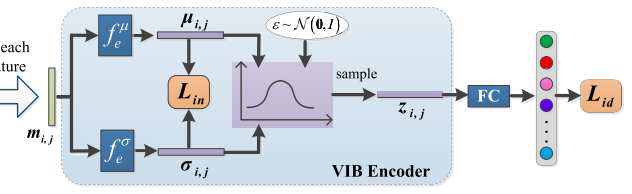

信息损失: 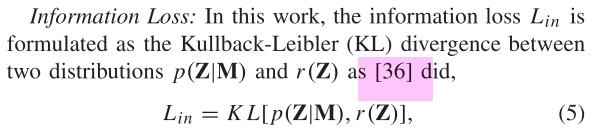 其中:   
m
i
,
j
m_{i,j}
mi,j? the j-th column of the
M
i
M_i
Mi?
身份损失:
 其中: 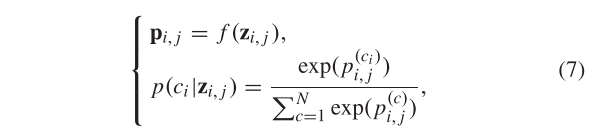
- 标签估计。在这里环节里面也用到了local-global的思想,利用的是全局特征的余弦相似和局部特征余弦相似的最大相似的加权值 来评估相似性。置信度与相似性等同。
全局相似:  局部相似:

最后的相似性:  标签估计:
 标签置信度:

- 标签采样。还是采用的和EUG一样的迭代渐进采样,但不同的是没有设置固定的采样数量,而是通过设定相似性阈值来控制,并且阈值在迭代过程中进行调整,总体原则是放宽限度,使得更多的伪标签样本被选择。
阈值设定:  1 则入选。 阈值动态更新: 
实验结果
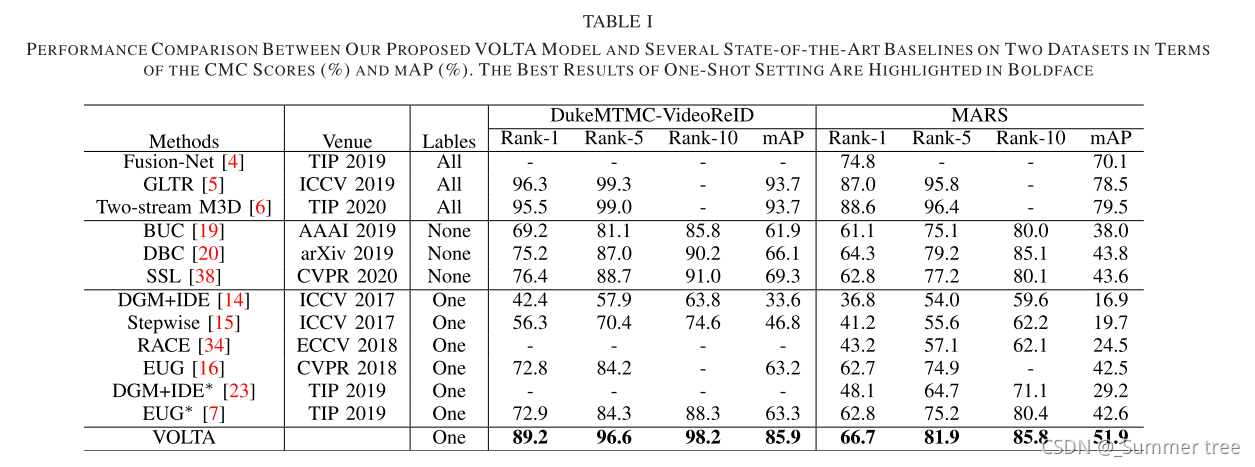 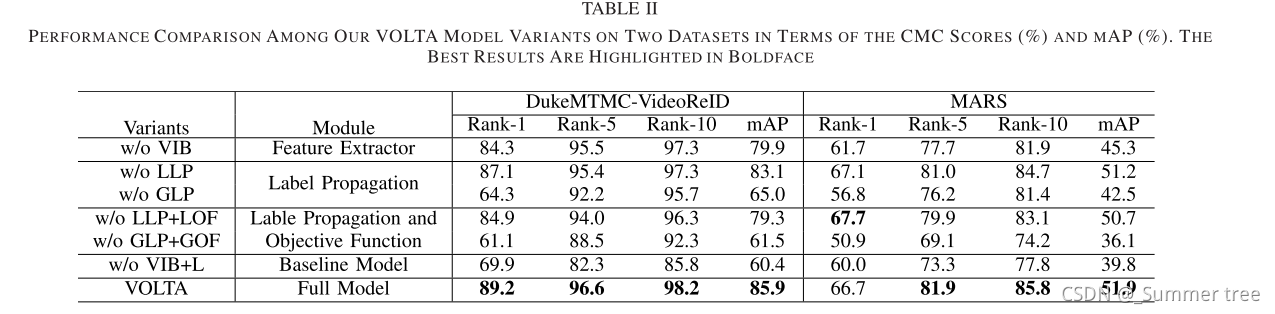
总体评价
- local-global的特征表达这个点比较简单,这种思想在其他文章中也有用到。
- 从ablation study可以看出,没有局部信息对文章的信息很小。看来主要贡献还是在于信息过滤VIB。
- VIB看起来有点复杂了,不知道是不是借鉴了其他文章中的方法。
- 通过设定相似性阈值才进行采样在其他文章中也有见到。
- 这里采用的是余弦相似性,用欧氏距离也可以,并且在以前的文章里面,欧式距离的效果通常比余弦要好。
- 总的来说,是篇好文章,不管是方式,还是写作,都有诸多值得学习的地方。
- 其实觉得它的图画得不是那么漂亮哈
引用格式
@ARTICLE{9211791, author={Liu, Meng and Qu, Leigang and Nie, Liqiang and Liu, Maofu and Duan, Lingyu and Chen, Baoquan}, journal={IEEE Transactions on Image Processing}, title={Iterative Local-Global Collaboration Learning Towards One-Shot Video Person Re-Identification}, year={2020}, volume={29}, number={}, pages={9360-9372}, doi={10.1109/TIP.2020.3026625}}
参考文献
[1] D. Chung, K. Tahboub, and E. J. Delp, “A two stream Siamese convolutional neural network for person re-identification,” in Proc. IEEE Int. Conf. Comput. Vis., Oct. 2017, pp. 1983–1991. [2] Y. Yan, B. Ni, Z. Song, C. Ma, Y. Yan, and X. Yang, “Person re- identification via recurrent feature aggregation,” in Proc. Eur. Conf. Comput. Vis., 2016, pp. 701–716. [3] L. Wu, Y. Wang, J. Gao, and X. Li, “Where-and-when to look: Deep Siamese attention networks for video-based person re- identification,” IEEE Trans. Multimedia, vol. 21, no. 6, pp. 1412–1424, Jun. 2019. [4] S. Kan, Y. Cen, Z. He, Z. Zhang, L. Zhang, and Y. Wang, “Supervised deep feature embedding with handcrafted feature,” IEEE Trans. Image Process., vol. 28, no. 12, pp. 5809–5823, Dec. 2019. [5] J. Li, S. Zhang, J. Wang, W. Gao, and Q. Tian, “Global-local temporal representations for video person re-identification,” in Proc. IEEE Int. Conf. Comput. Vis., Oct. 2019, pp. 3958–3967. [6] J. Li, S. Zhang, and T. Huang, “Multi-scale temporal cues learning for video person re-identification,” IEEE Trans. Image Process., vol. 29, pp. 4461–4473, 2020. [7] Y. Wu, Y. Lin, X. Dong, Y. Yan, W. Bian, and Y. Yang, “Progressive learning for person re-identification with one example,” IEEE Trans. Image Process., vol. 28, no. 6, pp. 2872–2881, Jun. 2019. [8] P. F. Felzenszwalb, R. B. Girshick, D. McAllester, and D. Ramanan, “Object detection with discriminatively trained part-based models,” IEEE Trans. Pattern Anal. Mach. Intell., vol. 32, no. 9, pp. 1627–1645, Sep. 2010. [9] A. Dehghan, S. M. Assari, and M. Shah, “GMMCP tracker: Globally optimal generalized maximum multi clique problem for multiple object tracking,” in Proc. IEEE Conf. Comput. Vis. Pattern Recognit., Jun. 2015, pp. 4091–4099. [10] L. Qi, L. Wang, J. Huo, L. Zhou, Y. Shi, and Y. Gao, “A novel unsupervised camera-aware domain adaptation framework for person re-identification,” in Proc. IEEE Int. Conf. Comput. Vis., Oct. 2019, pp. 8080–8089. [11] H.-X. Yu, W.-S. Zheng, A. Wu, X. Guo, S. Gong, and J.-H. Lai, “Unsupervised person re-identification by soft multilabel learning,” in Proc. IEEE Conf. Comput. Vis. Pattern Recognit., Jun. 2019, pp. 2148–2157. [12] N. McLaughlin, J. Martinez del Rincon, and P. Miller, “Recurrent convolutional network for video-based person re-identification,” in Proc. IEEE Conf. Comput. Vis. Pattern Recognit., Jun. 2016, pp. 1325–1334. [13] S. Xu, Y. Cheng, K. Gu, Y. Yang, S. Chang, and P. Zhou, “Jointly attentive spatial-temporal pooling networks for video-based person re- identification,” in Proc. IEEE Int. Conf. Comput. Vis., Oct. 2017, pp. 4733–4742. [14] M. Ye, A. J. Ma, L. Zheng, J. Li, and P. C. Yuen, “Dynamic label graph matching for unsupervised video re-identification,” in Proc. IEEE Int. Conf. Comput. Vis., Oct. 2017, pp. 5142–5150. [15] Z. Liu, D. Wang, and H. Lu, “Stepwise metric promotion for unsuper- vised video person re-identification,” in Proc. IEEE Int. Conf. Comput. Vis., Oct. 2017, pp. 2429–2438. [16] Y. Wu, Y. Lin, X. Dong, Y. Yan, W. Ouyang, and Y. Yang, “Exploit the unknown gradually: One-shot video-based person re-identification by stepwise learning,” in Proc. IEEE Conf. Comput. Vis. Pattern Recognit., Jun. 2018, pp. 5177–5186. [17] H.-X. Yu, A. Wu, and W.-S. Zheng, “Unsupervised person re- identification by deep asymmetric metric embedding,” IEEE Trans. Pattern Anal. Mach. Intell., vol. 42, no. 4, pp. 956–973, Apr. 2020. [18] M. Li, X. Zhu, and S. Gong, “Unsupervised tracklet person re- identification,” IEEE Trans. Pattern Anal. Mach. Intell., vol. 42, no. 7, pp. 1770–1782, Jul. 2020. [19] Y. Lin, X. Dong, L. Zheng, Y. Yan, and Y. Yang, “A bottom-up clustering approach to unsupervised person re-identification,” in Proc. AAAI Conf. Artif. Intell., 2019, pp. 8738–8745. [20] G. Ding, S. Khan, Z. Tang, J. Zhang, and F. Porikli, “Towards bet- ter validity: Dispersion based clustering for unsupervised person re- identification,” 2019, arXiv:1906.01308. [Online]. Available: http://arxiv. org/abs/1906.01308 [21] L. Wu, Y. Wang, H. Yin, M. Wang, and L. Shao, “Few-shot deep adversarial learning for video-based person re-identification,” IEEE Trans. Image Process., vol. 29, pp. 1233–1245, 2020. [22] Y. Kim, S. Choi, T. Kim, S. Lee, and C. Kim, “Learning to align multi- camera domains using part-aware clustering for unsupervised video person re-identification,” 2019, arXiv:1909.13248. [Online]. Available: http://arxiv.org/abs/1909.13248 [23] M. Ye, J. Li, A. J. Ma, L. Zheng, and P. C. Yuen, “Dynamic graph co- matching for unsupervised video-based person re-identification,” IEEE Trans. Image Process., vol. 28, no. 6, pp. 2976–2990, Jun. 2019. [24] K. Liu, B. Ma, W. Zhang, and R. Huang, “A spatio-temporal appearance representation for video-based pedestrian re-identification,” in Proc. IEEE Int. Conf. Comput. Vis., Dec. 2015, pp. 3810–3818. [25] H. Liu et al., “Video-based person re-identification with accumulative motion context,” IEEE Trans. Circuits Syst. Video Technol., vol. 28, no. 10, pp. 2788–2802, Oct. 2018. [26] M. Liu, L. Nie, X. Wang, Q. Tian, and B. Chen, “Online data organizer: Micro-video categorization by structure-guided multimodal dictionary learning,” IEEE Trans. Image Process., vol. 28, no. 3, pp. 1235–1247, Mar. 2019. [27] Y. Liu, J. Yan, and W. Ouyang, “Quality aware network for set to set recognition,” in Proc. IEEE Conf. Comput. Vis. Pattern Recognit., Jul. 2017, pp. 5790–5799. [28] Z. Zhou, Y. Huang, W. Wang, L. Wang, and T. Tan, “See the forest for the trees: Joint spatial and temporal recurrent neural networks for video-based person re-identification,” in Proc. IEEE Conf. Comput. Vis. Pattern Recognit., Jul. 2017, pp. 4747–4756. [29] D. Chen, H. Li, T. Xiao, S. Yi, and X. Wang, “Video person re- identification with competitive snippet-similarity aggregation and co- attentive snippet embedding,” in Proc. IEEE Conf. Comput. Vis. Pattern Recognit., Jun. 2018, pp. 1169–1178. [30] S. Li, S. Bak, P. Carr, and X. Wang, “Diversity regularized spatiotem- poral attention for video-based person re-identification,” in Proc. IEEE Conf. Comput. Vis. Pattern Recognit., Jun. 2018, pp. 369–378. [31] M. Liu, X. Wang, L. Nie, Q. Tian, B. Chen, and T.-S. Chua, “Cross- modal moment localization in videos,” in Proc. 26th ACM Int. Conf. Multimedia, 2018, pp. 843–851. [32] J. Li, S. Zhang, and T. Huang, “Multi-scale 3d convolution network for video based person re-identification,” in Proc. AAAI Conf. Artif. Intell., 2019, pp. 8618–8625. [33] J. Liu, Z.-J. Zha, X. Chen, Z. Wang, and Y. Zhang, “Dense 3D- convolutional neural network for person re-identification in videos,” ACM Trans. Multimedia Comput., Commun., Appl., vol. 15, no. 1s, pp. 1–19, Feb. 2019. [34] M. Ye, X. Lan, and P. C. Yuen, “Robust anchor embedding for unsupervised video person re-identification in the wild,” in Proc. Eur. Conf. Comput. Vis., 2018, pp. 170–186. [35] Y. Zou, Z. Yu, X. Liu, B. V. K. V. Kumar, and J. Wang, “Confidence reg- ularized self-training,” in Proc. IEEE Int. Conf. Comput. Vis., Oct. 2019, pp. 5982–5991. [36] A. A. Alemi, I. Fischer, J. V. Dillon, and K. Murphy, “Deep variational information bottleneck,” in Proc. Int. Conf. Learn. Represent., 2017, pp. 1–19. [37] J. Wu, S. Liao, Z. Lei, X. Wang, Y. Yang, and S. Z. Li, “Clustering and dynamic sampling based unsupervised domain adaptation for person re- identification,” in Proc. IEEE Int. Conf. Multimedia Expo, Jul. 2019, pp. 886–891. [38] Y. Lin, L. Xie, Y. Wu, C. Yan, and Q. Tian, “Unsupervised person re-identification via softened similarity learning,” in Proc. IEEE Conf. Comput. Vis. Pattern Recognit., Jun. 2020, pp. 3390–3399. [39] E. Ristani, F. Solera, R. Zou, R. Cucchiara, and C. Tomasi, “Performance measures and a data set for multi-target, multi-camera tracking,” in Proc. Eur. Conf. Comput. Vis., vol. 2016, pp. 17–35. [40] L. Zheng et al., “Mars: A video benchmark for large-scale person re- identification,” in Proc. Eur. Conf. Comput. Vis., 2016, pp. 868–884. [41] L. Zheng, L. Shen, L. Tian, S. Wang, J. Wang, and Q. Tian, “Scal- able person re-identification: A benchmark,” in Proc. IEEE Int. Conf. Comput. Vis., Dec. 2015, pp. 1116–1124. [42] S. Bak, E. Corvee, F. Bremond, and M. Thonnat, “Person re- identification using spatial covariance regions of human body parts,” in Proc. 7th IEEE Int. Conf. Adv. Video Signal Based Surveill., Aug. 2010, pp. 435–440. [43] A. Krizhevsky, I. Sutskever, and G. E. Hinton, “ImageNet classification with deep convolutional neural networks,” in Proc. Adv. Neural Inf. Process. Syst., 2012, pp. 1097–1105. [44] K. He, X. Zhang, S. Ren, and J. Sun, “Deep residual learning for image recognition,” in Proc. IEEE Conf. Comput. Vis. Pattern Recognit., Jun. 2016, pp. 770–778. [45] B. Zhou, A. Khosla, A. Lapedriza, A. Oliva, and A. Torralba, “Learning deep features for discriminative localization,” in Proc. IEEE Conf. Comput. Vis. Pattern Recognit., Jun. 2016, pp. 2921–2929
|