一、Smooth Inverse Frequency(SIF)
??Smooth Inverse Frequency是一种基于向量的检索。在介绍SIF前,需要先理解平均词向量与TFIDF加权平均词向量。
- 平均词向量就是将句子中所有词的word embedding相加取平均,得到的向量就当做最终的sentence embedding。这种方法的缺点是认为句子中的所有词对于表达句子含义同样重要。
- TFIDF加权平均词向量就是对每个词按照tfidf进行打分,然后进行加权平均,得到最终的句子表示。
SIF加权平均词向量主要是对上述两种方法进行了改进。SIF算法包括两步,第一步是对句子中所有的词向量进行加权平均,得到平均向量
v
s
v_s
vs?;第二步是移出(减去)
v
s
v_s
vs?在所有句子向量组成的矩阵的第一个主成分(principal component / singular vector)上的投影。
- 第一步主要是对TFIDF加权平均词向量表示句子的方法进行改进。论文提出了一种平滑倒词频 (smooth inverse frequency, SIF)方法用于计算每个词的加权系数,具体地,词
w
w
w的权重为
a
/
(
a
+
p
(
w
)
)
a/(a+p(w))
a/(a+p(w)),其中
a
a
a为平滑参数,
p
(
w
)
p(w)
p(w)为(估计的)词频。直观理解SIF,就是说频率越低的词在当前句子出现了,说明它在句子中的重要性更大,也就是加权系数更大。事实上,如果把一个句子认为是一篇文档并且假设该句中不出现重复的词(TF=1),那么TFIDF将演变成SIF,即未平滑的倒词频。但是相较于TFIDF这种经验式公式,论文通过理论证明为SIF提供理论依据。
- 第二步,个人的直观理解是移出所有句子的共有信息,因此保留下来的句子向量更能够表示本身并与其它句子向量产生差距。
算法伪代码如下: 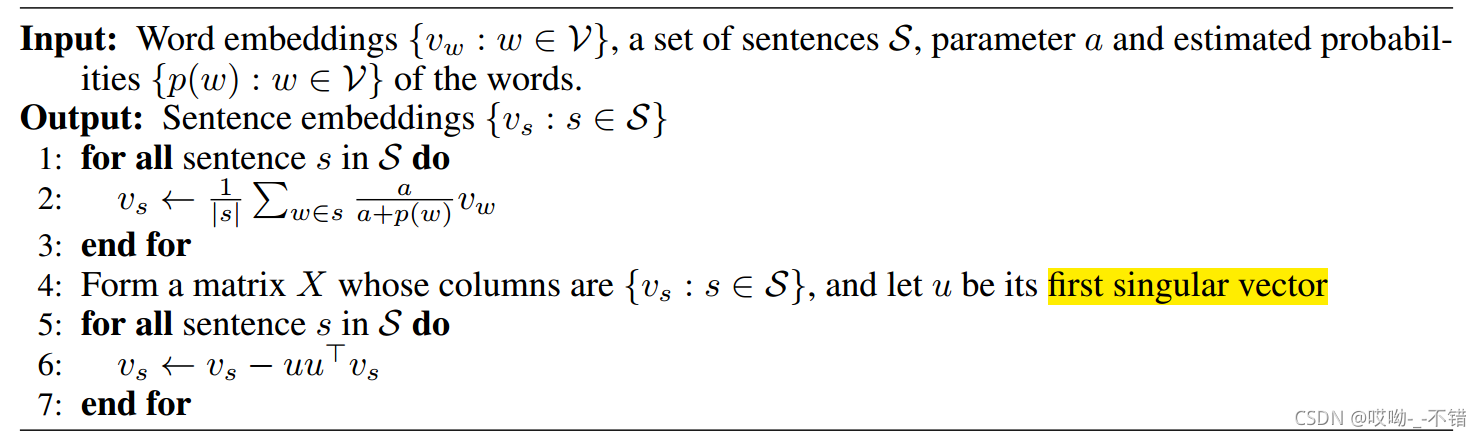 算法源码如下:https://github.com/PrincetonML/SIF 代码实现如下
二、BM25
??BM25是一种用来评价搜索词和文档之间相关性的算法,它是一种基于概率检索模型提出的算法:有一个query和一批文档Ds,现在计算query和每篇文档D之间的相关性,先对query进行切分(分词),得到单词
q
i
q_i
qi?,然后单词由3部分组成:
- 每个单词的权重
N表示所有文档的数目,
n
(
q
i
)
n(q_i)
n(qi?)包含了单词
q
i
q_i
qi?的文档数目。
I
D
F
(
q
i
)
=
l
o
g
(
N
?
n
(
q
i
)
+
0.5
n
(
q
i
)
+
0.5
)
IDF(q_i)=log(\frac{N-n(q_i)+0.5}{n(q_i)+0.5})
IDF(qi?)=log(n(qi?)+0.5N?n(qi?)+0.5?) - 相关性分数R:单词和Doc之间的相关性,最后对于每个query中单词的分数求和,得到query和文档之间的分数
R
(
q
i
,
d
)
=
f
i
(
k
1
+
1
)
f
i
+
K
q
f
i
(
k
2
+
1
)
q
f
i
+
k
2
R(q_i,d)=\frac{f_i(k_1+1)}{f_i+K}\frac{qf_i(k_2+1)}{qf_i+k_2}
R(qi?,d)=fi?+Kfi?(k1?+1)?qfi?+k2?qfi?(k2?+1)?
K
=
k
1
(
1
?
b
+
b
d
l
a
v
g
?
d
l
)
K=k_1(1-b+b\frac{dl}{avg\ dl})
K=k1?(1?b+bavg?dldl?)
k
1
,
k
2
,
b
k_1,k_2,b
k1?,k2?,b均为超参数
f
i
f_i
fi?表示
q
i
q_i
qi?出现在多少个文档中
q
f
i
qf_i
qfi?表示
q
i
q_i
qi?在这个query中的次数
d
l
dl
dl表示的是当前doc的长度
a
v
g
?
d
l
avg\ dl
avg?dl表示的是平均长度
S
c
o
r
e
(
Q
,
d
)
=
∑
n
I
D
F
(
q
i
)
R
(
q
i
,
d
)
Score(Q,d)=\sum^{n}IDF(q_i)R(q_i,d)
Score(Q,d)=∑n?IDF(qi?)R(qi?,d) 返回
t
o
p
K
top K
topK的Doc。
1.bm25源码实现
from gensim.summarization import bm25
class BM25RetrievalModel:
"""BM25 definition: https://en.wikipedia.org/wiki/Okapi_BM25"""
def __init__(self, corpus):
self.model = bm25.BM25(corpus)
def get_top_similarities(self, query, topk=10):
"""query: [word1, word2, ..., wordn]"""
scores = self.model.get_scores(query)
rtn = sorted(enumerate(scores), key=lambda x: x[1], reverse=True)[:topk]
return rtn[0][0], rtn[1][0]
其底层源码为:
import logging
import math
from six import iteritems
from six.moves import range
from functools import partial
from multiprocessing import Pool
from ..utils import effective_n_jobs
PARAM_K1 = 1.5
PARAM_B = 0.75
EPSILON = 0.25
logger = logging.getLogger(__name__)
class BM25(object):
"""Implementation of Best Matching 25 ranking function.
Attributes
----------
corpus_size : int
Size of corpus (number of documents).
avgdl : float
Average length of document in `corpus`.
doc_freqs : list of dicts of int
Dictionary with terms frequencies for each document in `corpus`. Words used as keys and frequencies as values.
idf : dict
Dictionary with inversed documents frequencies for whole `corpus`. Words used as keys and frequencies as values.
doc_len : list of int
List of document lengths.
"""
def __init__(self, corpus, k1=PARAM_K1, b=PARAM_B, epsilon=EPSILON):
"""
Parameters
----------
corpus : list of list of str
Given corpus.
k1 : float
Constant used for influencing the term frequency saturation. After saturation is reached, additional
presence for the term adds a significantly less additional score. According to [1]_, experiments suggest
that 1.2 < k1 < 2 yields reasonably good results, although the optimal value depends on factors such as
the type of documents or queries.
b : float
Constant used for influencing the effects of different document lengths relative to average document length.
When b is bigger, lengthier documents (compared to average) have more impact on its effect. According to
[1]_, experiments suggest that 0.5 < b < 0.8 yields reasonably good results, although the optimal value
depends on factors such as the type of documents or queries.
epsilon : float
Constant used as floor value for idf of a document in the corpus. When epsilon is positive, it restricts
negative idf values. Negative idf implies that adding a very common term to a document penalize the overall
score (with 'very common' meaning that it is present in more than half of the documents). That can be
undesirable as it means that an identical document would score less than an almost identical one (by
removing the referred term). Increasing epsilon above 0 raises the sense of how rare a word has to be (among
different documents) to receive an extra score.
"""
self.k1 = k1
self.b = b
self.epsilon = epsilon
self.corpus_size = 0
self.avgdl = 0
self.doc_freqs = []
self.idf = {}
self.doc_len = []
self._initialize(corpus)
def _initialize(self, corpus):
"""Calculates frequencies of terms in documents and in corpus. Also computes inverse document frequencies."""
nd = {}
num_doc = 0
for document in corpus:
self.corpus_size += 1
self.doc_len.append(len(document))
num_doc += len(document)
frequencies = {}
for word in document:
if word not in frequencies:
frequencies[word] = 0
frequencies[word] += 1
self.doc_freqs.append(frequencies)
for word, freq in iteritems(frequencies):
if word not in nd:
nd[word] = 0
nd[word] += 1
self.avgdl = float(num_doc) / self.corpus_size
idf_sum = 0
negative_idfs = []
for word, freq in iteritems(nd):
idf = math.log(self.corpus_size - freq + 0.5) - math.log(freq + 0.5)
self.idf[word] = idf
idf_sum += idf
if idf < 0:
negative_idfs.append(word)
self.average_idf = float(idf_sum) / len(self.idf)
if self.average_idf < 0:
logger.warning(
'Average inverse document frequency is less than zero. Your corpus of {} documents'
' is either too small or it does not originate from natural text. BM25 may produce'
' unintuitive results.'.format(self.corpus_size)
)
eps = self.epsilon * self.average_idf
for word in negative_idfs:
self.idf[word] = eps
def get_score(self, document, index):
"""Computes BM25 score of given `document` in relation to item of corpus selected by `index`.
Parameters
----------
document : list of str
Document to be scored.
index : int
Index of document in corpus selected to score with `document`.
Returns
-------
float
BM25 score.
"""
score = 0.0
doc_freqs = self.doc_freqs[index]
numerator_constant = self.k1 + 1
denominator_constant = self.k1 * (1 - self.b + self.b * self.doc_len[index] / self.avgdl)
for word in document:
if word in doc_freqs:
df = self.doc_freqs[index][word]
idf = self.idf[word]
score += (idf * df * numerator_constant) / (df + denominator_constant)
return score
def get_scores(self, document):
"""Computes and returns BM25 scores of given `document` in relation to
every item in corpus.
Parameters
----------
document : list of str
Document to be scored.
Returns
-------
list of float
BM25 scores.
"""
scores = [self.get_score(document, index) for index in range(self.corpus_size)]
return scores
def get_scores_bow(self, document):
"""Computes and returns BM25 scores of given `document` in relation to
every item in corpus.
Parameters
----------
document : list of str
Document to be scored.
Returns
-------
list of float
BM25 scores.
"""
scores = []
for index in range(self.corpus_size):
score = self.get_score(document, index)
if score > 0:
scores.append((index, score))
return scores
三、基于BM25、tfidf和SIF的检索系统代码实现
主函数main.py 为:
import argparse
from NLP贪心.information_retrieval_demo.utils import get_corpus, word_tokenize, build_word_embedding
from model_zoo.bm25_model import BM25RetrievalModel
from model_zoo.tfidf_model import TFIDFRetrievalModel
from model_zoo.sif_model import SIFRetrievalModel
from model_zoo.bert_model import BertRetrievalModel
parser = argparse.ArgumentParser(description='Information retrieval model hyper-parameter setting.')
parser.add_argument('--input_file_path', type=str, default='./ChangCheng.xls', help='Training data location.')
parser.add_argument('--model_type', type=str, default='sif', help='Different information retrieval models.')
parser.add_argument('--gensim_model_path', type=str, default='./cached/gensim_model.pkl')
parser.add_argument('--pretrained_gensim_embddings_file', type=str, default='./cached/gensim_word_embddings.pkl')
parser.add_argument('--cached_gensim_embedding_file', type=str, default='./cached/embeddings_gensim.pkl')
parser.add_argument('--embedding_dim', type=int, default=100)
parser.add_argument('--bert_model_ckpt', type=str, default='./model_zoo/bert/chinese_L-12_H-768_A-12/bert_model.ckpt')
parser.add_argument('--bert_config_file', type=str, default='./model_zoo/bert/chinese_L-12_H-768_A-12/bert_config.json')
parser.add_argument('--bert_vocab_file', type=str, default='./model_zoo/bert/chinese_L-12_H-768_A-12/vocab.txt')
parser.add_argument('--max_seq_len', type=int, default=30)
parser.add_argument('--pooling_strategy', type=int, default=0)
parser.add_argument('--pooling_layer', type=str, default='-2')
args = parser.parse_args()
questions_src, answers = get_corpus(args.input_file_path)
questions = [word_tokenize(line) for line in questions_src]
answers_corpus = [word_tokenize(line) for line in answers]
print('\nBuild gensim model and word vectors...')
build_word_embedding(questions+answers_corpus, args.gensim_model_path, args.pretrained_gensim_embddings_file)
def predict(model, query):
"""
预测
:param model: 模型
:param query: 输入文本
:return: topK
"""
query = word_tokenize(query)
top_1, top_2 = model.get_top_similarities(query, topk=2)
return questions_src[top_1], answers[top_1], questions_src[top_2], answers[top_2]
if __name__ == '__main__':
query = '上班穿什么'
if args.model_type == 'bm25':
bm25_model = BM25RetrievalModel(questions)
res = predict(bm25_model, query)
elif args.model_type == 'tfidf':
tfidf_model = TFIDFRetrievalModel(questions)
res = predict(tfidf_model, query)
elif args.model_type == 'sif':
sif_model = SIFRetrievalModel(questions, args.pretrained_gensim_embddings_file,
args.cached_gensim_embedding_file, args.embedding_dim)
res = predict(sif_model, query)
elif args.model_type == 'bert':
bert_model = BertRetrievalModel(questions, args.bert_model_ckpt, args.bert_config_file,
args.bert_vocab_file, args.max_seq_len,
args.pooling_strategy, args.pooling_layer)
res = predict(bert_model, query)
else:
raise ValueError('Invalid model type!')
print('Query: ', query)
print('\nQuestion 1: ', res[0])
print('Answer 1: ', res[1])
print('\nQuestion 2: ', res[2])
print('Answer 2: ', res[3])
工具util.py 部分为:
import jieba as jie
import pandas as pd
import numpy as np
import pickle
from gensim.models import Word2Vec
import re
from tqdm import tqdm
def get_corpus(file_path, header_idx=0):
"""
读取问题、答案
:param file_path: 文件路径
:param header_idx: 表头索引
:return:
"""
src_df = pd.read_excel(file_path, header=header_idx)
print('Corpus shape before: ', src_df.shape)
src_df = src_df.dropna(subset=['Response'])
print('Corpus shape after: ', src_df.shape)
return src_df['Question'].tolist(), src_df['Response'].tolist()
def clean_text(text):
"""
对文本进行正则化处理
:param text:
:return:
"""
text = re.sub(
u"([hH]ttp[s]{0,1})://[a-zA-Z0-9\.\-]+\.([a-zA-Z]{2,4})(:\d+)?(/[a-zA-Z0-9\-~!@#$%^&*+?:_/=<>.',;]*)?", '',
text)
text = re.sub(u'#[^#]+#', '', text)
text = re.sub(u'回复@[\u4e00-\u9fa5a-zA-Z0-9_-]{1,30}:', '', text)
text = re.sub(u'@[\u4e00-\u9fa5a-zA-Z0-9_-]{1,30}', '', text)
text = re.sub(r'[0-9]+', 'DIG', text.strip()).lower()
text = ''.join(text.split())
return text
def word_tokenize(line):
"""
对输入文本进行分词
:param line: 输入文本
:return:
"""
content = clean_text(line)
return jie.lcut(content)
def load_embedding(cached_embedding_file):
"""load embeddings"""
with open(cached_embedding_file, mode='rb') as f:
return pickle.load(f)
def save_embedding(word_embeddings, cached_embedding_file):
"""save word embeddings"""
with open(cached_embedding_file, mode='wb') as f:
pickle.dump(word_embeddings, f)
def get_word_embedding_matrix(word2idx, pretrained_embeddings_file, embedding_dim=200):
"""Load pre-trained embeddings"""
pre_trained_embeddings = np.zeros((len(word2idx), embedding_dim))
initialized = 0
exception = 0
num = 0
with open(pretrained_embeddings_file, mode='r') as f:
try:
for line in f:
word_vec = line.split()
idx = word2idx.get(word_vec[0], -1)
if idx != -1:
pre_trained_embeddings[idx] = np.array(word_vec[1:], dtype=np.float)
initialized += 1
num += 1
if num % 10000 == 0:
print(num)
except:
exception += 1
print('Pre-trained embedding initialization proportion: ', (initialized + 0.0) / len(word2idx))
print('exception num: ', exception)
return pre_trained_embeddings
def build_word_embedding(corpus, gensim_model_path, gensim_word_embdding_path):
"""
基于语料训练词向量
:param corpus: 语料
:param gensim_model_path: 模型路径
:param gensim_word_embdding_path: 编码路径
:return:
"""
model = Word2Vec(min_count=1, size=100, window=5, sg=1, negative=5, sample=0.001,
iter=30)
model.build_vocab(sentences=corpus)
model.train(sentences=corpus, total_examples=model.corpus_count, epochs=model.iter)
model.wv.save_word2vec_format(gensim_word_embdding_path, binary=False)
model.save(gensim_model_path)
print('\nGensim model build successfully!')
print('\nTest the performance of word2vec model')
for test_word in ['门禁卡', '食堂', '试用期']:
aa = model.wv.most_similar(test_word)[0:10]
print('\nMost similar word of %s is:' % test_word)
for word, score in aa:
print('{} {}'.format(word, score))
'''
# save word counts
sorted_word_counts = OrderedDict(sorted(model.wv.vocab.items(), key=lambda x: x[1].count, reverse=True))
word_counts_file = codecs.open('./word_counts.txt', mode='w', encoding='utf-8')
for k, v in sorted_word_counts.items():
word_counts_file.write(k + ' ' + str(v.count) + '\n')
'''
BM25模型部分为:
from gensim.summarization import bm25
class BM25RetrievalModel:
"""BM25 definition: https://en.wikipedia.org/wiki/Okapi_BM25"""
def __init__(self, corpus):
self.model = bm25.BM25(corpus)
def get_top_similarities(self, query, topk=10):
"""query: [word1, word2, ..., wordn]"""
scores = self.model.get_scores(query)
rtn = sorted(enumerate(scores), key=lambda x: x[1], reverse=True)[:topk]
return rtn[0][0], rtn[1][0]
TF-IDF模型部分为:
from gensim.corpora import Dictionary
from gensim.models import TfidfModel
from gensim.similarities import MatrixSimilarity
from sklearn.feature_extraction.text import TfidfVectorizer
from annoy import AnnoyIndex
import numpy as np
class TFIDFRetrievalModel:
def __init__(self, corpus):
'''
min_freq = 3
self.dictionary = Dictionary(corpus)
# Filter low frequency words from dictionary.
low_freq_ids = [id_ for id_, freq in
self.dictionary.dfs.items() if freq <= min_freq]
self.dictionary.filter_tokens(low_freq_ids)
self.dictionary.compactify()
self.corpus = [self.dictionary.doc2bow(line) for line in corpus]
self.model = TfidfModel(self.corpus)
self.corpus_mm = self.model[self.corpus]
self.index = MatrixSimilarity(self.corpus_mm)
'''
corpus_str = []
for line in corpus:
corpus_str.append(' '.join(line))
self.tfidf = TfidfVectorizer(token_pattern=r"(?u)\b\w+\b")
sentence_tfidfs = np.asarray(self.tfidf.fit_transform(corpus_str).todense().astype('float'))
self.t = AnnoyIndex(sentence_tfidfs.shape[1])
for i in range(sentence_tfidfs.shape[0]):
self.t.add_item(i, sentence_tfidfs[i, :])
self.t.build(10)
def get_top_similarities(self, query, topk=10):
"""query: [word1, word2, ..., wordn]"""
'''
query_vec = self.model[self.dictionary.doc2bow(query)]
scores = self.index[query_vec]
rtn = sorted(enumerate(scores), key=lambda x: x[1], reverse=True)[:topk]
return rtn
'''
'''
query2tfidf = []
for word in query:
if word in self.word2tfidf:
query2tfidf.append(self.word2tfidf[word])
query2tfidf = np.array(query2tfidf)
'''
query2tfidf = np.asarray(self.tfidf.transform([' '.join(query)]).todense().astype('float'))[0]
top_ids, top_distances = self.t.get_nns_by_vector(query2tfidf, n=topk, include_distances=True)
return top_ids
SIF模型部分为:
from sklearn.feature_extraction.text import CountVectorizer
import numpy as np
from NLP贪心.information_retrieval_demo.utils import get_word_embedding_matrix, save_embedding, load_embedding
import os
from sklearn.decomposition import PCA
from annoy import AnnoyIndex
class SIFRetrievalModel:
"""
A simple but tough-to-beat baseline for sentence embedding.
from https://openreview.net/pdf?id=SyK00v5xx
Principle : Represent the sentence by a weighted average of the word vectors, and then modify them using Principal Component Analysis.
Issue 1: how to deal with big input size ?
randomized SVD version will not be affected by scale of input, see https://github.com/PrincetonML/SIF/issues/4
Issue 2: how to preprocess input data ?
Even if you dont remove stop words SIF will take care, but its generally better to clean the data,
see https://github.com/PrincetonML/SIF/issues/23
Issue 3: how to obtain the embedding of new sentence ?
Weighted average is enough, see https://www.quora.com/What-are-some-interesting-techniques-for-learning-sentence-embeddings
"""
def __init__(self, corpus, pretrained_embedding_file, cached_embedding_file, embedding_dim):
self.embedding_dim = embedding_dim
self.max_seq_len = 0
corpus_str = []
for line in corpus:
corpus_str.append(' '.join(line))
self.max_seq_len = max(self.max_seq_len, len(line))
counter = CountVectorizer(token_pattern=r"(?u)\b\w+\b")
bow = counter.fit_transform(corpus_str).todense().astype('float')
word_count = np.sum(bow, axis=0)
word_freq = word_count / np.sum(word_count)
SIF_weight = 1e-3
self.word2weight = np.asarray(SIF_weight / (SIF_weight + word_freq))
self.SIF_npc = 1
self.word2id = counter.vocabulary_
seq_matrix_id = np.zeros(shape=(len(corpus_str), self.max_seq_len), dtype=np.int64)
seq_matrix_weight = np.zeros((len(corpus_str), self.max_seq_len), dtype=np.float64)
for idx, seq in enumerate(corpus):
seq_id = []
for word in seq:
if word in self.word2id:
seq_id.append(self.word2id[word])
seq_len = len(seq_id)
seq_matrix_id[idx, :seq_len] = seq_id
seq_weight = [self.word2weight[0][id] for id in seq_id]
seq_matrix_weight[idx, :seq_len] = seq_weight
if os.path.exists(cached_embedding_file):
self.word_embeddings = load_embedding(cached_embedding_file)
else:
self.word_embeddings = get_word_embedding_matrix(counter.vocabulary_, pretrained_embedding_file, embedding_dim=self.embedding_dim)
save_embedding(self.word_embeddings, cached_embedding_file)
self.sentence_embeddings = self.SIF_embedding(seq_matrix_id, seq_matrix_weight)
self.t = AnnoyIndex(self.embedding_dim)
for i in range(self.sentence_embeddings.shape[0]):
self.t.add_item(i, self.sentence_embeddings[i, :])
self.t.build(10)
def SIF_embedding(self, x, w):
"""句向量计算"""
n_samples = x.shape[0]
emb = np.zeros((n_samples, self.word_embeddings.shape[1]))
for i in range(n_samples):
emb[i, :] = w[i, :].dot(self.word_embeddings[x[i, :], :]) / np.count_nonzero(w[i, :])
svd = PCA(n_components=self.SIF_npc, svd_solver='randomized')
svd.fit(emb)
self.pc = svd.components_
if self.SIF_npc == 1:
common_component_removal = emb - emb.dot(self.pc.transpose()) * self.pc
else:
common_component_removal = emb - emb.dot(self.pc.transpose()).dot(self.pc)
return common_component_removal
def get_top_similarities(self, query, topk=10):
"""query: [word1, word2, ..., wordn]"""
query2id = []
for word in query:
if word in self.word2id:
query2id.append(self.word2id[word])
query2id = np.array(query2id)
id2weight = np.array([self.word2weight[0][id] for id in query2id])
query_embedding = id2weight.dot(self.word_embeddings[query2id, :]) / query2id.shape[0]
top_ids, top_distances = self.t.get_nns_by_vector(query_embedding, n=topk, include_distances=True)
return top_ids
运行结果为:
Query: 上班穿什么
Question 1: 公司有着装要求吗?
Answer 1: 公司要求周一至周四着正装,佩戴工牌和司徽(司徽可在六楼综合处贾洋洋处领取),男士需打领带。并且保证桌面整洁,座位处不放杂物。
Question 2: 公司有着装要求么?
Answer 2: 公司要求周一至周四着正装,佩戴工牌和司徽(司徽可在六楼综合处贾洋洋处领取),男士需打领带。并且保证桌面整洁,座位处不放杂物。
如果对您有帮助,麻烦点赞关注,这真的对我很重要!!!如果需要互关,请评论或者私信! 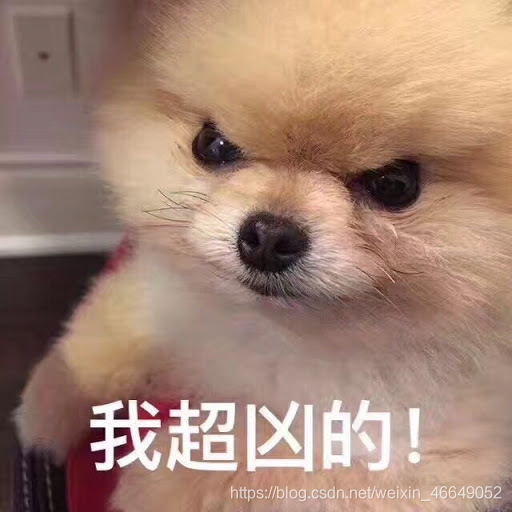
|