目标检测现况
两阶段、单阶段。 新:对象关键点: 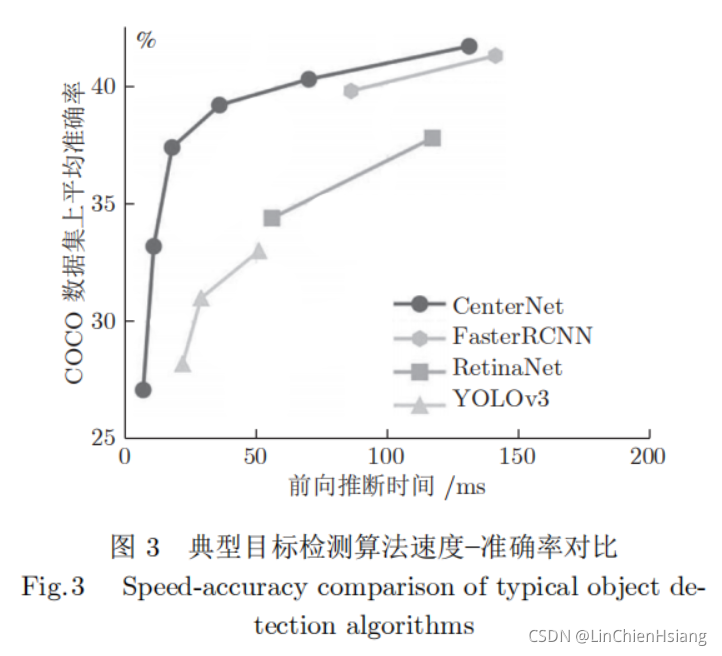
[11] Law H, Deng J. CornerNet: Detecting objects as paired keypoints. In: Proceedings of the 2018 European Conference on Computer Vision (ECCV). Munich, Germany: Springer, 2018. 765?781 [12] Zhou X Y, Zhuo J C, Kr?henbühl P. Bottom-up object detection by grouping extreme and center points. In: Proceedings of the 2019 IEEE/CVF Conference on Computer Vision and Pattern Recognition (CVPR). Long Beach, CA, USA: IEEE, 2019. 850?859 [13] Zhou X Y, Wang D Q, Kr?henbühl P. Objects as points. arXiv: 1904.07850, 2019 [14] Yang Z, Liu S H, Hu H, Wang L W, Lin S. RepPoints: Point set representation for object detection. In: Proceedings of the 2019 IEEE/CVF International Conference on Computer Vision (ICCV). Seoul, Korea: IEEE, 2019. 9656?9665
目标检测模块
主干网络(浅层卷积、深层卷积)、颈部连接层、锚点、区域特征编码、分类与定位头部和损失函数
优化
主干网络:
提取且传递能力有限——残差网络+Inception(残差网络的参数共享,宽度) 特征图与通道 特征图上,任一点与全局的关系——图像注意力机制的非局部网络(增强目标特征)
颈部连接:多特征融合技术:特征金字塔模型——FPN 特征融合支路——PAnet自下向上结构 沙漏式网络结构: **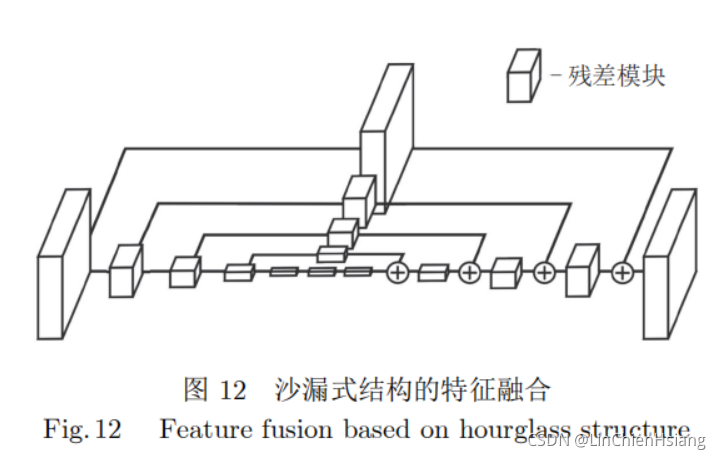 高分辨率网络
锚点
非极大值抑制算法的优化
交并比算法
正负样本采样
分类与定位
上下文信息
多尺度预测方法——不同分辨率的特征图
损失函数——量化错误 分类与定位(置信度
《自动化学报》 目标检测模型及其优化方法综述 蒋弘毅 王永娟 康锦煜
|