迁移学习是什么,为什么要用迁移学习
场景一
我们在训练模型的时候,拿 CNN 网络来举例,CNN 的前几层捕获的是边缘的信息,其实对于任何图片,他们的边缘信息都是有共同点的,这些信息抽象度比较低,重要程度也并不高。但是我们建立神经网络的时候,并不可能只要后几层,这就类似再高的楼,都要有地基。而有了前面的这些层次,神经网络的参数就会在一层层的堆叠中不断变大,那么我们怎么来解决这个问题呢?
场景二
我想训练一个目标跟踪或者图像分割的复杂神经网络,他们的前端无一例外地都要使用很庞大的 CNN 网络来提取图片中的信息。但是如果我从头训练整个网络,势必会消耗大量的时间和资源。
破局之法:迁移学习
- 使用别人构建的标准模型为你的模型或者功能服务;
- 使用别人训练好的模型的参数,减少了自己训练的麻烦;
- 可以在别人模型的基础上进行进一步操作,站在巨人的肩膀上;
- 可以横跨很多领域,使得功能实现更加灵活。
迁移学习主要的三种手段
实现迁移学习有以下三种手段:
- Transfer Learning: 冻结预训练模型的全部卷积层,只训练自己定制的全连接层。
- Extract Feature Vector: 先计算出预训练模型的卷积层对所有训练和测试数据的特征向量,然后抛开预训练模型,只训练自己定制的简配版全连接网络。
- Fine-tuning: 冻结预训练模型的部分卷积层(通常是靠近输入的多数卷积层,因为这些层保留了大量底层信息)甚至不冻结任何网络层,训练剩下的卷积层(通常是靠近输出的部分卷积层)和全连接层。
Keras 可以使用哪些已经训练好的模型
模型选择:Inceptionv3
- Inceptionv3 的网络结构中的基本块就是这种形式,结合了多种大小的卷积核对同一个图片进行多次卷积操作,最终将结果按照通道进行整合。
- 具体的情况不再赘述,可以去找相应的文章看。
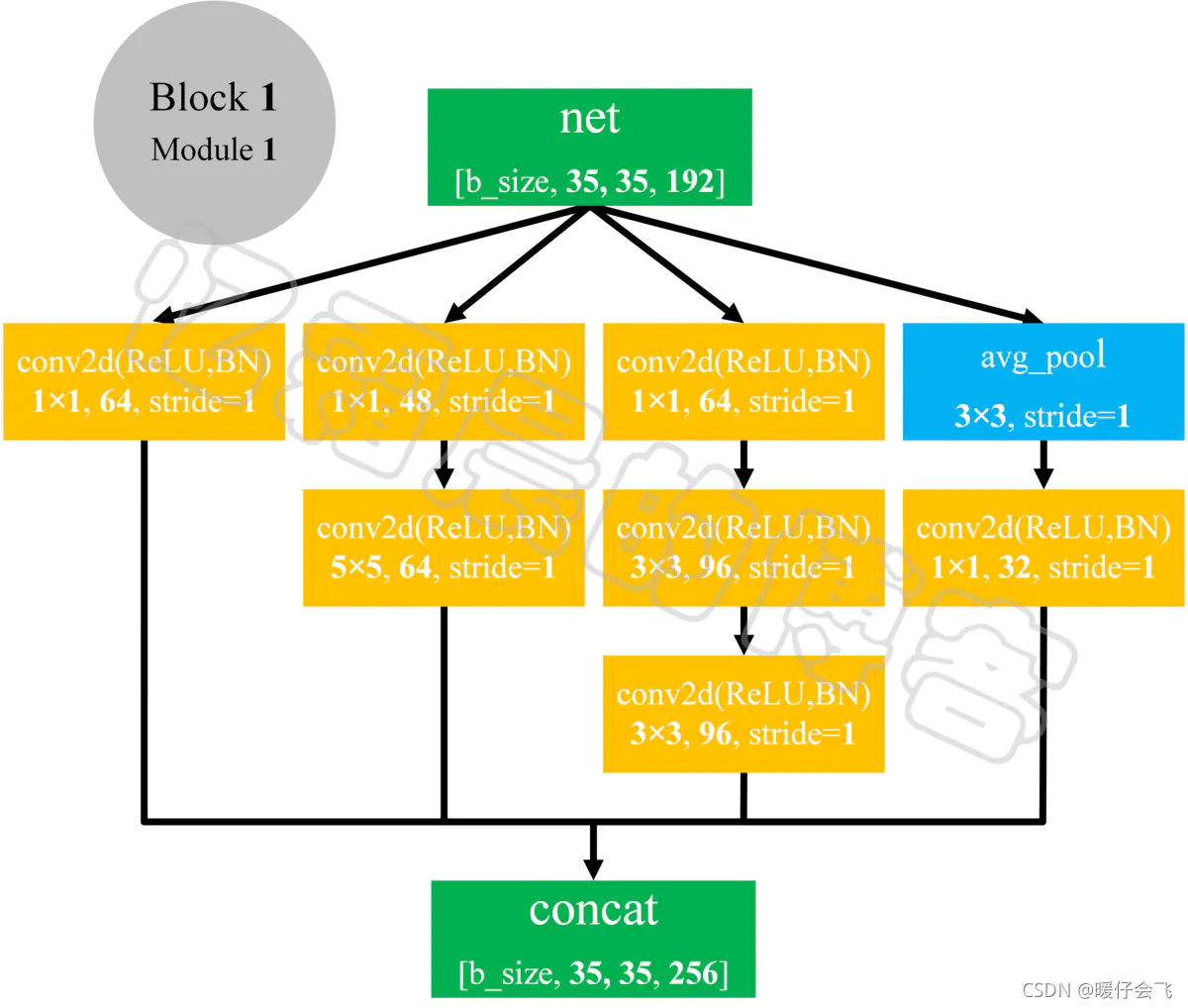
实现步骤
- 加载数据集 cifar10,因为 keras 中使用的 Inceptionv3 的模型的默认输入是 (299,299,3),因此我们需要使用三通道的数据集。这里推荐使用 cifar10 或者 cifar100 进行测试。当然你也可以自己使用别的图片数据集。
导入模块
from keras.applications.inception_v3 import InceptionV3
import matplotlib.pyplot as plt
from keras.layers import *
from keras.models import *
from keras.optimizers import *
from keras.losses import categorical_crossentropy
from keras_preprocessing.image import ImageDataGenerator
from keras.datasets import cifar10,cifar100
from keras.utils import to_categorical
from sklearn.model_selection import train_test_split
import numpy as np
import cv2
加载数据集
(x_train,y_train),(x_test,y_test)= cifar10.load_data()
y_test = to_categorical(y_test)
y_train = to_categorical(y_train)
print(x_train.shape)
print(y_train.shape)
调整数据集中图片的尺寸
temp = [cv2.resize(i,(299,299)) for i in x_train]
temp_test = [cv2.resize(i,(299,299)) for i in x_test]
temp = np.array(temp)
temp_test = np.array(temp_test)
print(temp.shape)
print(temp_test.shape)
x_train = temp.reshape(50000,299,299,3)
x_test = temp_test.reshape(10000,299,299,3)
数据增强 / 使用简单的原始数据
'''
构建增强数据的生成器
可用可不用,最好使用
在本文中因为只是对迁移学习的效果进行验证;
因此不适用这个数据增强器增强我们的数据
使用原始数据的话,这里就不用看了。
'''
train_datagen = ImageDataGenerator(
rotation_range=30,
width_shift_range=0.2,
height_shift_range=0.2,
shear_range=0.2,
zoom_range=0.2,
horizontal_flip=True,
)
加载 Keras 中的 Inceptionv3 模型
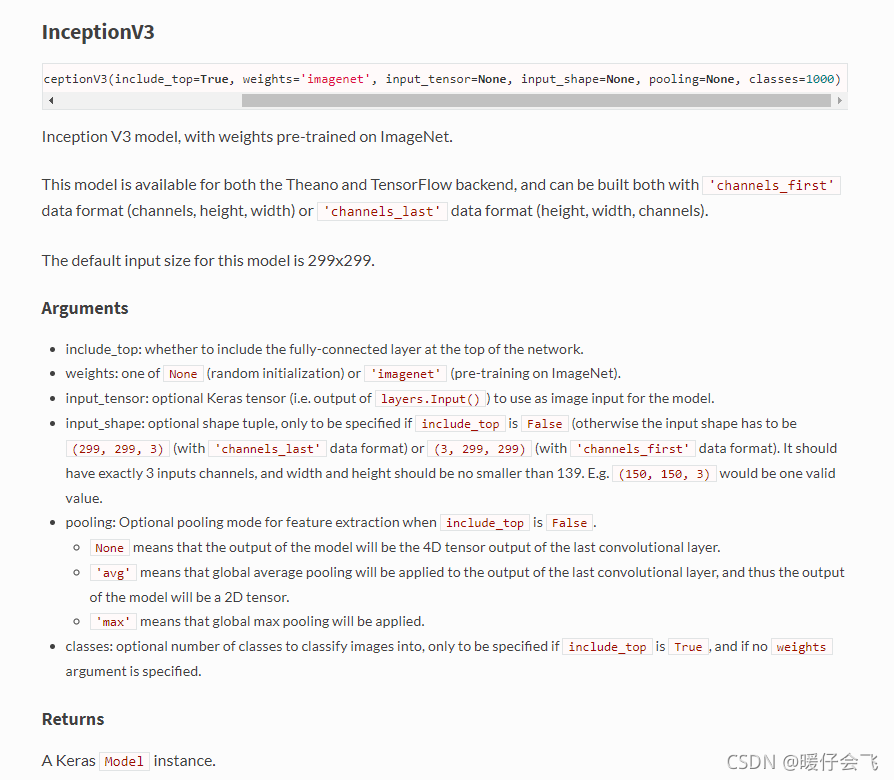
- weights 设置为 “imagenet” 的话,他就会自动进行参数下载了。
- 建议 include_top 设置为 false,如果设置为 true 的话,模型参数大的不得了
base_model = InceptionV3(weights="imagenet",include_top=False)
模型删改
'''
在原有基础上构建进一步的模型
'''
x = base_model.output
x = GlobalAveragePooling2D()(x)
x = Dense(64,activation='relu')(x)
output = Dense(10,activation='softmax')(x)
model = Model(inputs=base_model.input,outputs=output)
- 打印此时的模型信息,就会发现,需要训练的参数还是巨大的,有
21900138 。 - 红色框里面是我们构建的模块
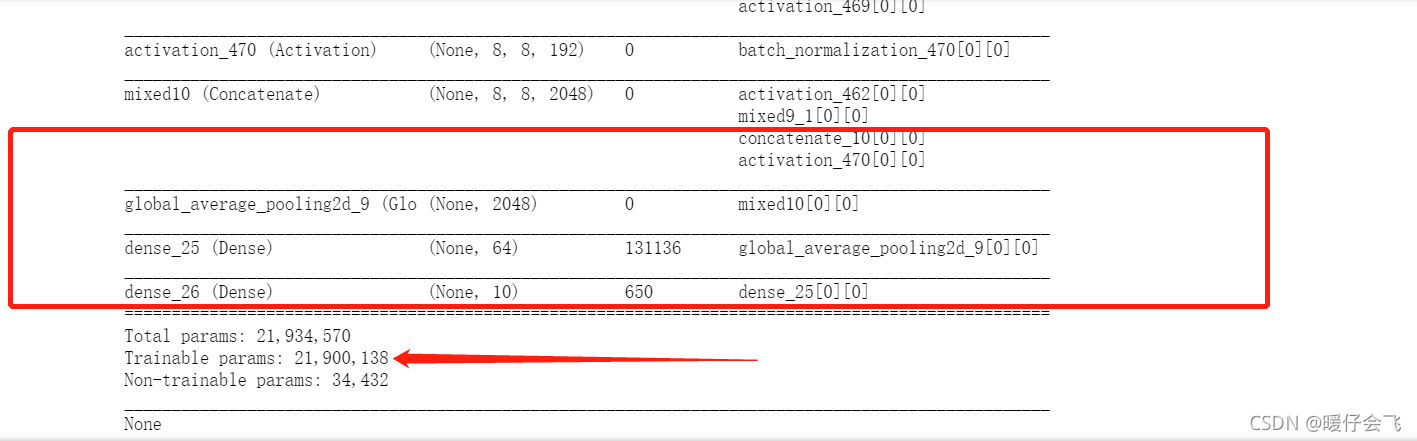
知识迁移
回顾一下上面的知识: 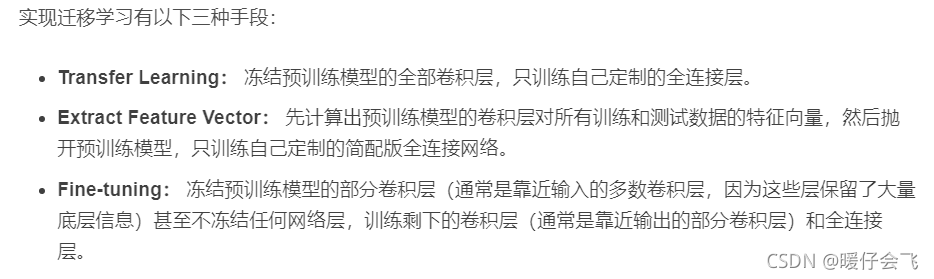
第一种手段:Transfer Learning
- 不要忘记,冻结所有原来的层之后,一定要 compile 才会生效哦~
'''
遍历所有的 Inceptionv3 自己的层,
把这些层的 trainable 都设置为 false;
!!!!
注意,设置完之后,一定要 compile 才会生效,
所以不要忘记最后一行代码!!!
'''
for layer in base_model.layers:
layer.trainable = False
model.compile(optimizer='adam',loss='categorical_crossentropy',metrics=['accuracy'])
- 冻结了之后,可以再看一遍要训练的参数量,是不是小 case,只有
131786
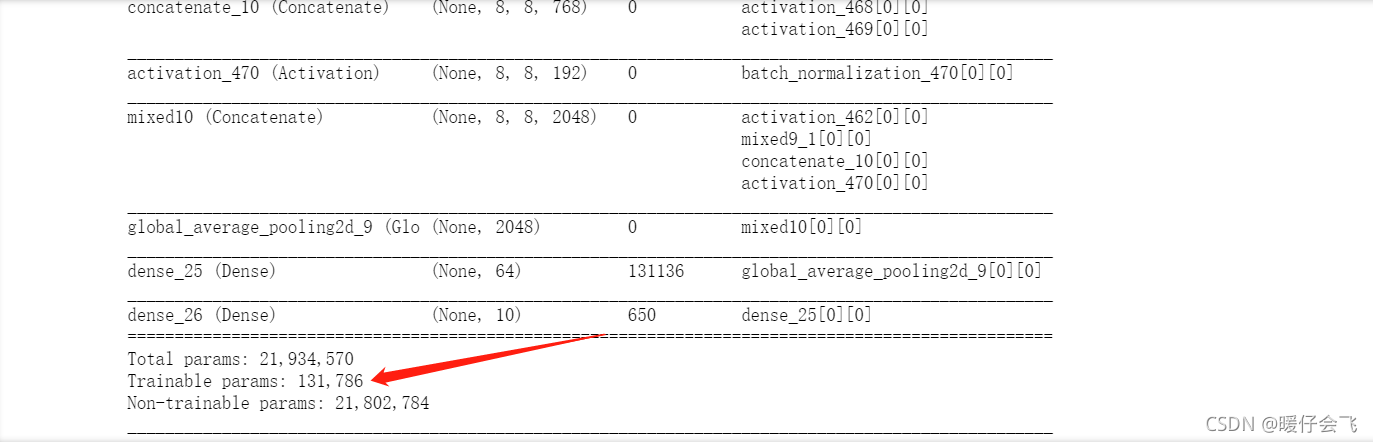
第三种手段:Fine-tune
'''第三种手段在本质上和第一种是一样的,只是冻结的层数不同
训练的参数越多,当然对任务的拟合效果大概率会更好。
'''
frozen_layer_number = 250
for layer in base_model.layers[:frozen_layer_number+1]:
layer.trainable = False
model.compile(optimizer=Adagrad(lr=0.0001),loss='categorical_crossentropy',metrics=['accuracy'])
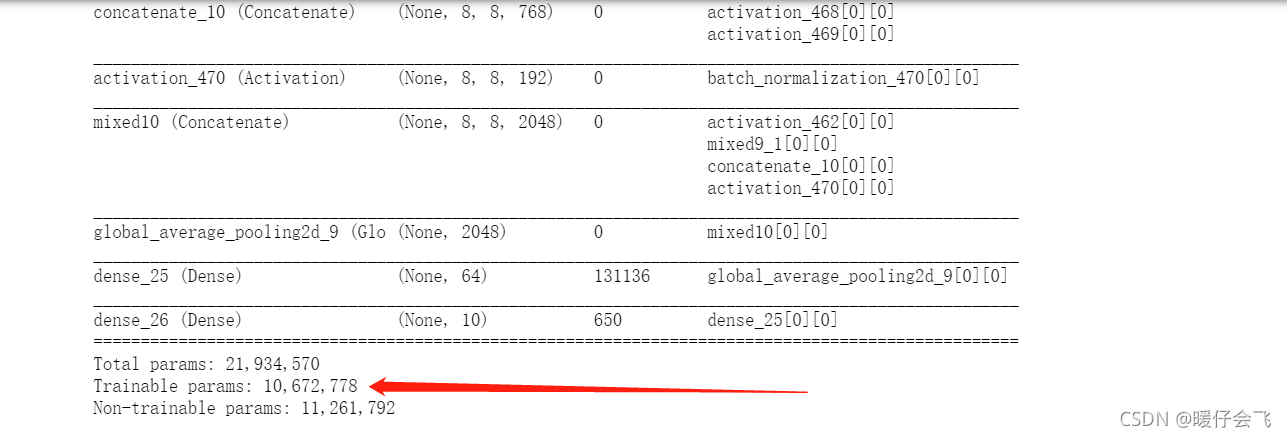
冻结之后的训练
'''
冻结完毕之后就可以开始训练了。
为了简便,这里没有用一些 checkpoint ,步长递减,回调
之类的方法,大家可以参考我的其他关于 keras 的文章
'''
history = model.fit(x_train,
y_train,
batch_size=64,
epochs=10,
validation_data=(x_test,y_test))
history_tl = model.fit_generator(generator=train_datagen.flow(x_train,y_train,batch_size=64),
steps_per_epoch=800,
epochs=10,
validation_data=(x_test,y_test))
为什么不讲第二种手段? 别急,原理是相同的,但操作步骤略有不同,而且训练速度要快很多。放在最后压轴。
让我们先把第一种和第三种的步骤进行完毕。
第二种手段:Extract Feature Vector
- 由于这种手段是新建一个小规模的网络,因此和大网络之间并不存在交集
- 所以训练和收敛的速度都比较快。
- 为了方便演示,这里只是转换了训练和测试集的前 100 个样本,如果你要自己做测试的话,请务必保证原始的数据集大小。
'''
首先将 base model 辛辛苦苦提取出来的特征图拿过来
毕竟这是个好东西
为了做测试方便,我们不使用全部的数据集,而是使用前100个图片
的特征图做一下代码上的演示
'''
x_train_features = base_model.predict(x_train[:100])
x_test_features = base_model.predict(x_test[:100])
'''
通过 x_train_feature 的维度和尺寸来新建一个后续的网络模块
直接接受在 base model 中训练好的 x_train_feature 进行二次训练
从而适应自己的网络功能
'''
print(x_train_features.shape)
'''开始构建小规模的第二阶段网络'''
model_= Sequential()
model_.add(Flatten())
model_.add(Dense(256,activation='relu',input_dim=2048*8*8))
model_.add(Dropout(0.5))
model_.add(Dense(10,activation='softmax'))
model_.compile(optimizer=Adam(lr=0.001),loss='categorical_crossentropy',metrics=['accuracy'])
history_ = model_.fit(x_train_features,
y_train[0:100],
batch_size=64,
epochs=50,
validation_data=(x_test_features,y_test[0:100]))
重点总结
- 迁移学习有三种方法
- 载入 keras 自带模型的时候要注意 input_shape 要符合官方规定的 (299,299,3) 才能加载 imagenet 的预训练参数
- 使用训练集之前,一定要把训练数据的尺寸调整为合适的尺寸,然后才能输入到网络中
- 以上就是用 keras 做简单的迁移学习的全部小方法。
代码重构
from keras.applications.inception_v3 import InceptionV3
import matplotlib.pyplot as plt
from keras.layers import *
from keras.models import *
from keras.optimizers import *
from keras.losses import categorical_crossentropy
from keras_preprocessing.image import ImageDataGenerator
from keras.datasets import cifar10,cifar100
from keras.utils import to_categorical
import numpy as np
import cv2
INPUT_SHAPE = (299,299,3)
BATCH_SIZE = 64
EPOCHS = 100
TRAIN_SIZE = 50000
TEST_SIZE = 10000
class InceptionV3_Network():
def __init__(self, base_model, classes, transfer_mean):
self.base_model = base_model
self.classes = classes
self.transfer_mean = transfer_mean
self.model = self.make_network()
self.input_shape = INPUT_SHAPE
def get_model_summary(self):
return self.model.summary()
def make_network(self):
if self.transfer_mean == "second":
model = Sequential()
model.add(Flatten())
model.add(Dense(256,activation='relu',input_dim=2048*8*8))
model.add(Dropout(0.5))
model.add(Dense(self.classes,activation='softmax'))
model.compile(optimizer=Adam(lr=0.001), loss='categorical_crossentropy', metrics=['accuracy'])
return model
else:
x = self.base_model.output
x = GlobalAvgPool2D()(x)
x = Dense(64, activation='relu')(x)
output = Dense(self.classes, activation='softmax')(x)
model = Model(inputs=base_model.input, outputs=output)
if self.transfer_mean == "first" :
for layer in base_model.layers:
layer.trainable = False
model.compile(optimizer='adam', loss='categorical_crossentropy', metrics=['accuracy'])
if self.transfer_mean == "third":
frozen_layer_number = 250
for layer in base_model.layers[:frozen_layer_number + 1]:
layer.trainable = False
model.compile(optimizer=Adagrad(lr=0.0001),
loss='categorical_crossentropy',
metrics=['accuracy'])
return model
def train(self, dataset='cifar10'):
x_train, y_train, x_test, y_test = training_data_process(BATCH_SIZE,aug=False,dataset=dataset)
if self.transfer_mean == "second":
x_train_features = base_model.predict(x_train)
x_test_features = base_model.predict(x_test)
return self.model.fit(x_train_features,
y_train,
batch_size=BATCH_SIZE,
epochs=EPOCHS,
validation_data=(x_test_features, y_test))
else:
return self.model.fit(x_train,
y_train,
batch_size=BATCH_SIZE,
epochs=EPOCHS,
validation_data=(x_test, y_test))
def image_augmentation_generator(rotation_range=20,
width_shift_range=0.1,
height_shift_range=0.1,
shear_range=0.,
zoom_range=0.,
zca_epsilon=1e-6,
horizontal_flip=True,
fill_mode='nearest'):
return ImageDataGenerator(rotation_range=rotation_range,
width_shift_range=width_shift_range,
height_shift_range=height_shift_range,
shear_range=shear_range,
zoom_range=zoom_range,
zca_epsilon=zca_epsilon,
horizontal_flip=horizontal_flip,
fill_mode=fill_mode)
def training_data_process(batch_size,aug=False,dataset='cifar10'):
if dataset == 'cifar10':
original_shape = (32, 32, 3)
(x_train, y_train), (x_test, y_test) = cifar10.load_data()
temp = [cv2.resize(i, (INPUT_SHAPE[1], INPUT_SHAPE[2])) for i in x_train]
temp_test = [cv2.resize(i, (INPUT_SHAPE[1], INPUT_SHAPE[2])) for i in x_test]
temp = np.array(temp)
temp_test = np.array(temp_test)
print(temp.shape)
print(temp_test.shape)
x_train = temp.reshape(TRAIN_SIZE, INPUT_SHAPE[0], INPUT_SHAPE[1], INPUT_SHAPE[2])
x_test = temp_test.reshape(TEST_SIZE, INPUT_SHAPE[0], INPUT_SHAPE[1], INPUT_SHAPE[2])
y_test = to_categorical(y_test)
y_train = to_categorical(y_train)
x_train = x_train / 255.
x_test = x_test / 255.
if aug == True:
aug = image_augmentation_generator()
aug.fit(x_train)
imgGen = aug.flow(x_train, y_train, batch_size=batch_size, shuffle=True)
else:
return x_train, y_train, x_test, y_test
return imgGen, x_test, y_test
if __name__ == '__main__':
base_model = InceptionV3(weights="imagenet", include_top=False)
total_model = InceptionV3_Network(base_model,10,"first")
total_model.make_network()
total_model.train(dataset='cifar10')
Note: 重构之后没有运行,如果有错误,还请包涵!!!有问题可以私信交流。
|