前言
很多同学在入门强化学习的时候都会遇到困难,那我这里就简单介绍一下应该如何入门强化学习,并以开源代码为例详解强化学习实战。
一、强化学习学习之路
这边首先推荐莫凡python,人工智能的初学者如果不知道莫凡,那可真是纵称英雄也枉然。 他的官网长这样,链接在此! 链接: https://mofanpy.com/. 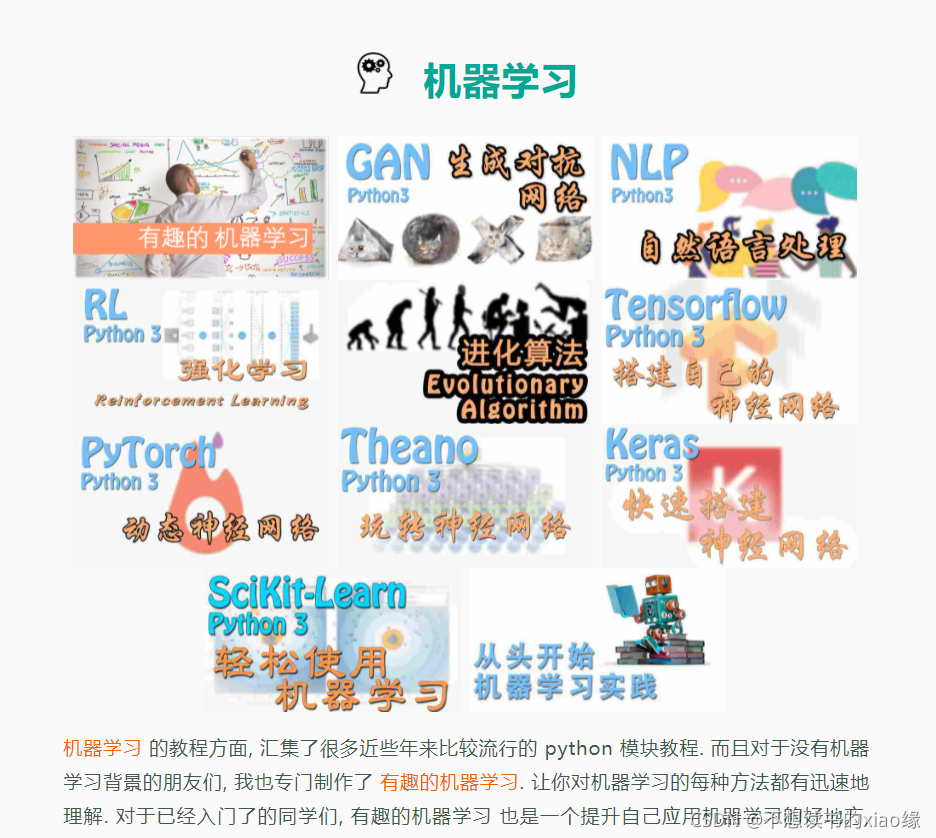
莫凡python的内容以简单著称,每个视频只有短短几分钟,长一点的也就20分钟左右,能够迅速的帮你入门强化学习,但是仅仅看这个是不够的,还需要扎实的理论基础,那就需要转战B站。
Bilibili有UCL大学的David Silver教授教学的Reinforcement learning课程。 链接在此!链接: https://www.bilibili.com/video/BV1kb411i7KG?from=search&seid=15348457829154001765&spm_id_from=333.337.0.0. David Silver教授推导出了DPG公式,非常厉害的一个人物,所以他的课程必听! 这个课程主要以公式推导为主,能够让初学者对强化学习的基础公式推导有一定的了解,例如贝尔曼公式。
当然B站李宏毅老师的强化学习课程也非常值得一听,但是我个人觉得他和莫凡python的课程差不多,但是他没有代码层面的教学,他的课程更多是在理论推导和科普介绍… 最后最后,我要推荐一本书籍,这本书籍也非常适用于初学者,Sutton的书很厚,我个人觉得不适用于初学者。 清华大学出版社邹伟老师出品的强化学习,结合了很多莫凡Python的代码,能够很好的将理论与实际相结合,很多代码都有注释,能够迅速帮初学者入门。 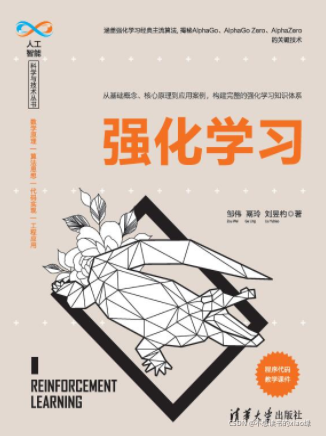
二、开源代码DDPG详解
如果说到强化学习,那么必须要会的就是Python和Gym 代码从Github上搜索DDPG就能够获取,这里采用的是floodsung的项目。 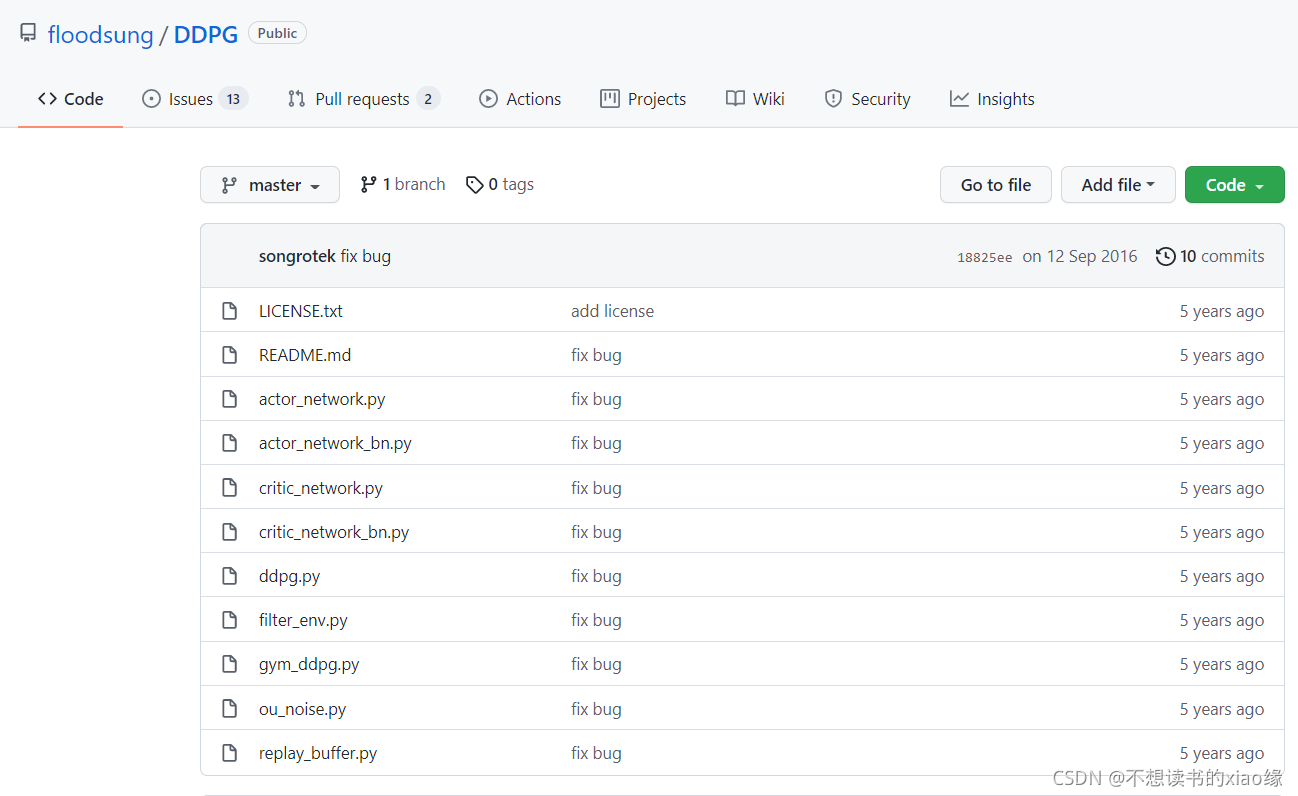 关键的几个文件:
actor_network
critic.network
ddpg
gym_ddpg
ou_noise
replay_buffer
代码如下(示例):
import numpy as np
import numpy.random as nr
class OUNoise:
"""docstring for OUNoise"""
def __init__(self,action_dimension,mu=0, theta=0.15, sigma=0.2):
self.action_dimension = action_dimension
self.mu = mu
self.theta = theta
self.sigma = sigma
self.state = np.ones(self.action_dimension) * self.mu
self.reset()
def reset(self):
self.state = np.ones(self.action_dimension) * self.mu
def noise(self):
x = self.state
dx = self.theta * (self.mu - x) + self.sigma * nr.randn(len(x))
self.state = x + dx
return self.state
if __name__ == '__main__':
ou = OUNoise(3)
states = []
for i in range(1000):
states.append(ou.noise())
import matplotlib.pyplot as plt
plt.plot(states)
plt.show()
from collections import deque
import random
class ReplayBuffer(object):
def __init__(self, buffer_size):
self.buffer_size = buffer_size
self.num_experiences = 0
self.buffer = deque()
def get_batch(self, batch_size):
# Randomly sample batch_size examples
return random.sample(self.buffer, batch_size)
def size(self):
return self.buffer_size
def add(self, state, action, reward, new_state, done):
experience = (state, action, reward, new_state, done)
if self.num_experiences < self.buffer_size:
self.buffer.append(experience)
self.num_experiences += 1
else:
self.buffer.popleft()
self.buffer.append(experience)
def count(self):
# if buffer is full, return buffer size
# otherwise, return experience counter
return self.num_experiences
def erase(self):
self.buffer = deque()
self.num_experiences = 0
import tensorflow as tf
import numpy as np
import math
# Hyper Parameters
LAYER1_SIZE = 400
LAYER2_SIZE = 300
LEARNING_RATE = 1e-4
TAU = 0.001
BATCH_SIZE = 64
class ActorNetwork:
"""docstring for ActorNetwork"""
def __init__(self,sess,state_dim,action_dim):
self.sess = sess
self.state_dim = state_dim
self.action_dim = action_dim
# create actor network
self.state_input,self.action_output,self.net = self.create_network(state_dim,action_dim)
# create target actor network
self.target_state_input,self.target_action_output,self.target_update,self.target_net = self.create_target_network(state_dim,action_dim,self.net)
# define training rules
self.create_training_method()
self.sess.run(tf.initialize_all_variables())
self.update_target()
#self.load_network()
def create_training_method(self):
self.q_gradient_input = tf.placeholder("float",[None,self.action_dim])
self.parameters_gradients = tf.gradients(self.action_output,self.net,-self.q_gradient_input)
self.optimizer = tf.train.AdamOptimizer(LEARNING_RATE).apply_gradients(zip(self.parameters_gradients,self.net))
def create_network(self,state_dim,action_dim):
layer1_size = LAYER1_SIZE
layer2_size = LAYER2_SIZE
state_input = tf.placeholder("float",[None,state_dim])
W1 = self.variable([state_dim,layer1_size],state_dim)
b1 = self.variable([layer1_size],state_dim)
W2 = self.variable([layer1_size,layer2_size],layer1_size)
b2 = self.variable([layer2_size],layer1_size)
W3 = tf.Variable(tf.random_uniform([layer2_size,action_dim],-3e-3,3e-3))
b3 = tf.Variable(tf.random_uniform([action_dim],-3e-3,3e-3))
layer1 = tf.nn.relu(tf.matmul(state_input,W1) + b1)
layer2 = tf.nn.relu(tf.matmul(layer1,W2) + b2)
action_output = tf.tanh(tf.matmul(layer2,W3) + b3)
return state_input,action_output,[W1,b1,W2,b2,W3,b3]
def create_target_network(self,state_dim,action_dim,net):
state_input = tf.placeholder("float",[None,state_dim])
ema = tf.train.ExponentialMovingAverage(decay=1-TAU)
target_update = ema.apply(net)
target_net = [ema.average(x) for x in net]
layer1 = tf.nn.relu(tf.matmul(state_input,target_net[0]) + target_net[1])
layer2 = tf.nn.relu(tf.matmul(layer1,target_net[2]) + target_net[3])
action_output = tf.tanh(tf.matmul(layer2,target_net[4]) + target_net[5])
return state_input,action_output,target_update,target_net
def update_target(self):
self.sess.run(self.target_update)
def train(self,q_gradient_batch,state_batch):
self.sess.run(self.optimizer,feed_dict={
self.q_gradient_input:q_gradient_batch,
self.state_input:state_batch
})
def actions(self,state_batch):
return self.sess.run(self.action_output,feed_dict={
self.state_input:state_batch
})
def action(self,state):
return self.sess.run(self.action_output,feed_dict={
self.state_input:[state]
})[0]
def target_actions(self,state_batch):
return self.sess.run(self.target_action_output,feed_dict={
self.target_state_input:state_batch
})
# f fan-in size
def variable(self,shape,f):
return tf.Variable(tf.random_uniform(shape,-1/math.sqrt(f),1/math.sqrt(f)))
'''
def load_network(self):
self.saver = tf.train.Saver()
checkpoint = tf.train.get_checkpoint_state("saved_actor_networks")
if checkpoint and checkpoint.model_checkpoint_path:
self.saver.restore(self.sess, checkpoint.model_checkpoint_path)
print "Successfully loaded:", checkpoint.model_checkpoint_path
else:
print "Could not find old network weights"
def save_network(self,time_step):
print 'save actor-network...',time_step
self.saver.save(self.sess, 'saved_actor_networks/' + 'actor-network', global_step = time_step)
'''
由于critic网络和actor网络在网络结构上是一样的,就不重复介绍了。
import gym
import tensorflow as tf
import numpy as np
from ou_noise import OUNoise
from critic_network import CriticNetwork
from actor_network_bn import ActorNetwork
from replay_buffer import ReplayBuffer
# Hyper Parameters:
REPLAY_BUFFER_SIZE = 1000000
REPLAY_START_SIZE = 10000
BATCH_SIZE = 64
GAMMA = 0.99
class DDPG:
"""docstring for DDPG"""
def __init__(self, env):
self.name = 'DDPG' # name for uploading results
self.environment = env
# Randomly initialize actor network and critic network
# with both their target networks
self.state_dim = env.observation_space.shape[0]
self.action_dim = env.action_space.shape[0]
self.sess = tf.InteractiveSession()
self.actor_network = ActorNetwork(self.sess,self.state_dim,self.action_dim)
self.critic_network = CriticNetwork(self.sess,self.state_dim,self.action_dim)
# initialize replay buffer
self.replay_buffer = ReplayBuffer(REPLAY_BUFFER_SIZE)
# Initialize a random process the Ornstein-Uhlenbeck process for action exploration
self.exploration_noise = OUNoise(self.action_dim)
def train(self):
#print "train step",self.time_step
# Sample a random minibatch of N transitions from replay buffer
minibatch = self.replay_buffer.get_batch(BATCH_SIZE)
state_batch = np.asarray([data[0] for data in minibatch])
action_batch = np.asarray([data[1] for data in minibatch])
reward_batch = np.asarray([data[2] for data in minibatch])
next_state_batch = np.asarray([data[3] for data in minibatch])
done_batch = np.asarray([data[4] for data in minibatch])
# for action_dim = 1
action_batch = np.resize(action_batch,[BATCH_SIZE,self.action_dim])
# Calculate y_batch 计算y_batch,实际上就是在运用TD——error公式
next_action_batch = self.actor_network.target_actions(next_state_batch)
q_value_batch = self.critic_network.target_q(next_state_batch,next_action_batch)
y_batch = []
for i in range(len(minibatch)):
if done_batch[i]:
y_batch.append(reward_batch[i])
else :
y_batch.append(reward_batch[i] + GAMMA * q_value_batch[i])
y_batch = np.resize(y_batch,[BATCH_SIZE,1])
# Update critic by minimizing the loss L 训练Critic网络
self.critic_network.train(y_batch,state_batch,action_batch)
# Update the actor policy using the sampled gradient:
action_batch_for_gradients = self.actor_network.actions(state_batch)
q_gradient_batch = self.critic_network.gradients(state_batch,action_batch_for_gradients)
self.actor_network.train(q_gradient_batch,state_batch)
# Update the target networks 通过滑动的方式更新
self.actor_network.update_target()
self.critic_network.update_target()
def noise_action(self,state):
# Select action a_t according to the current policy and exploration noise
action = self.actor_network.action(state)
return action+self.exploration_noise.noise()
def action(self,state):
action = self.actor_network.action(state)
return action
def perceive(self,state,action,reward,next_state,done):
# Store transition (s_t,a_t,r_t,s_{t+1}) in replay buffer
self.replay_buffer.add(state,action,reward,next_state,done)
# Store transitions to replay start size then start training
if self.replay_buffer.count() > REPLAY_START_SIZE:
self.train()
#if self.time_step % 10000 == 0:
#self.actor_network.save_network(self.time_step)
#self.critic_network.save_network(self.time_step)
# Re-iniitialize the random process when an episode ends
if done:
self.exploration_noise.reset()
import filter_env
from ddpg import *
import gc
gc.enable()
ENV_NAME = 'InvertedPendulum-v1'
EPISODES = 100000
TEST = 10
def main():
#env = filter_env.makeFilteredEnv(gym.make(ENV_NAME))
env=gym.make(ENV_NAME)
env = env.unwrapped
env.seed(1)
agent = DDPG(env)
#agent = DDPG(env)
#env.monitor.start('experiments/' + ENV_NAME,force=True)
for episode in xrange(EPISODES):
state = env.reset()
#print "episode:",episode
# Train
for step in xrange(env.spec.timestep_limit):
action = agent.noise_action(state)
next_state,reward,done,_ = env.step(action)
agent.perceive(state,action,reward,next_state,done)
state = next_state
if done:
break
# Testing:测试阶段和训练阶段相同
if episode % 100 == 0 and episode > 100:
total_reward = 0
for i in xrange(TEST):
state = env.reset()
for j in xrange(env.spec.timestep_limit):
#env.render()
action = agent.action(state) # direct action for test
state,reward,done,_ = env.step(action)
total_reward += reward
if done:
break
ave_reward = total_reward/TEST
print 'episode: ',episode,'Evaluation Average Reward:',ave_reward
env.close()
if __name__ == '__main__':
main()
总结
以上就是本文的全部内容啦,如果喜欢的就点个赞吧! 0基础初学者学习强化学习我觉得大概花费一个月的时间是比较合适的,如果学习无人驾驶车辆的同学,强烈建议使用Carla仿真软件!个人认为强化学习最难的还是设计奖励函数,为了奖励函数的设计,还专门有一类方法,逆强化学习,这也是一门很深的学问啊!
|