推荐系统
定义:根据用户的历史信息和行为,向用户推荐他感兴趣的内容 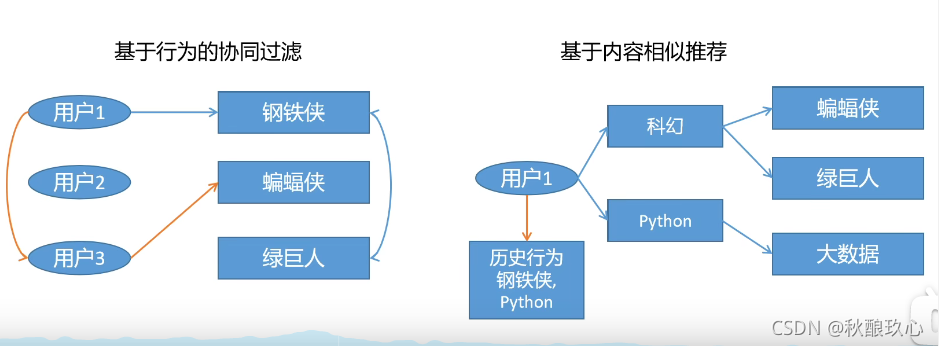
推荐系统解决的问题:
- 信息过载:商品,视频等有好几百万。用户怎样找到自己感兴趣的物品。系统怎样展示几百万的物品给用户,达到自己的商业目标
- 挖掘长尾:大部分冷门物品得不到暴露,然而他们的加和价值超过热门物品
- 用户体验:搜索(当明确自己的目标的时候,我们用搜索引擎)。推荐(当不明确的时候,推荐系统推荐我们感兴趣的商品)
推荐系统包含的环节
召回(协同过滤召回,内容相似召回,图算法召回等等)->排序(机器学习,二分类算法:LR、GBDT、DNN)->调整(去重,已购过滤,在线过滤,热门补足,合并内容信息) 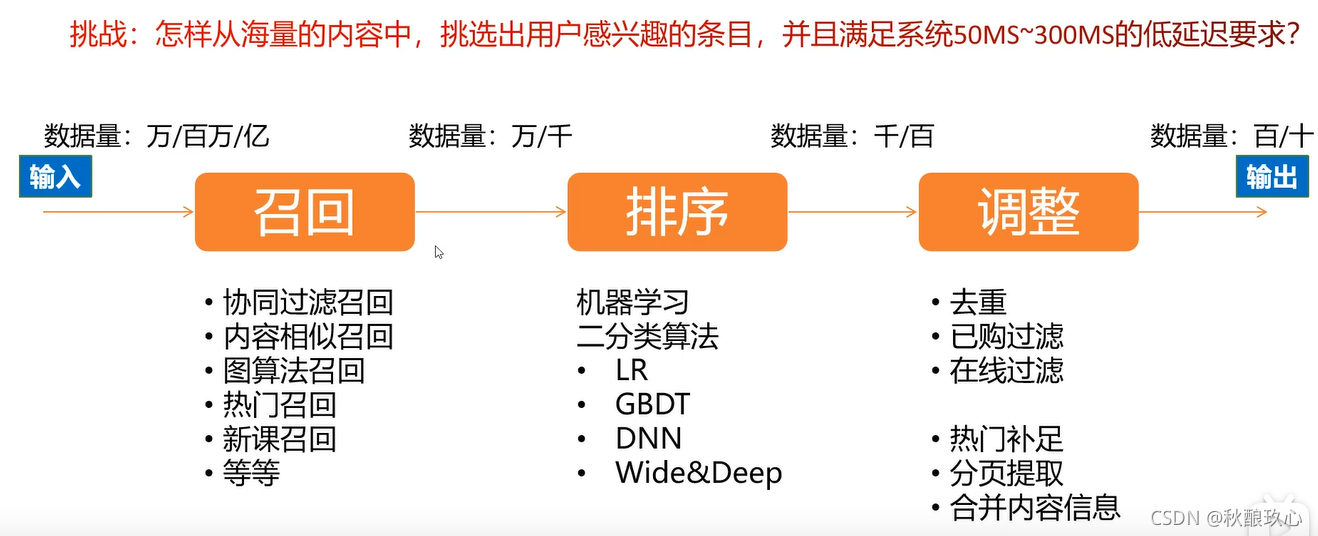
推荐系统的召回路径
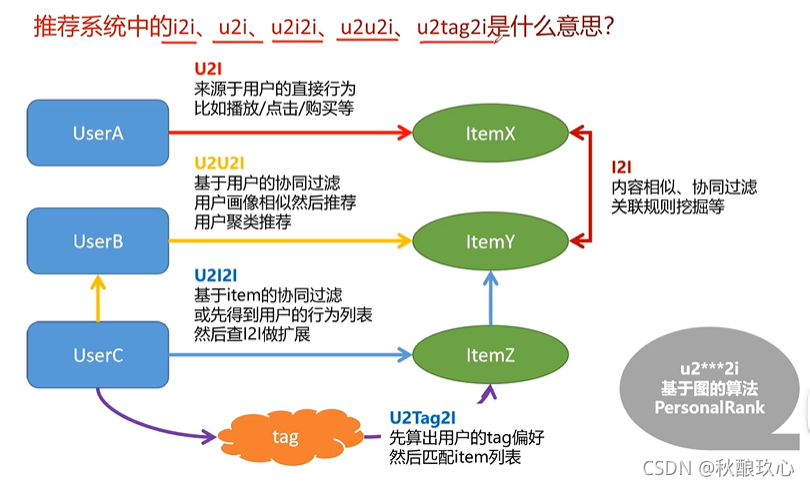
基于内容的推荐系统
(1)地位:最早被使用的推荐算法,年代久远,但当今仍然被广泛使用,效果良好
(2)定义:给用户x推荐之前喜欢的物品的相似物品,即U2I2I、U2Tag2I
(3)步骤: 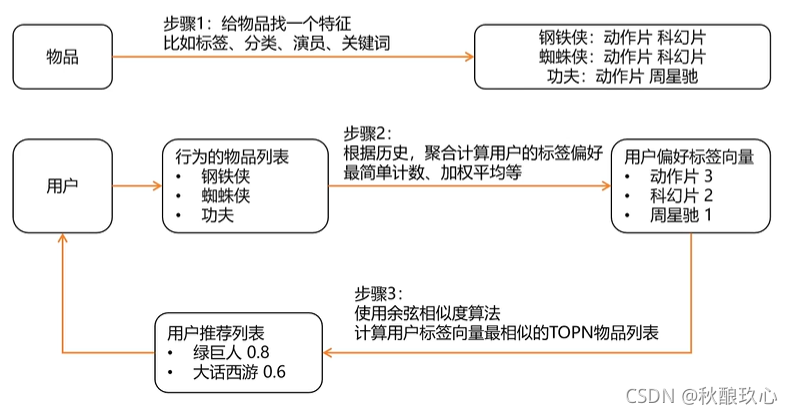 (4)用户向量和物品向量的相似度计算: 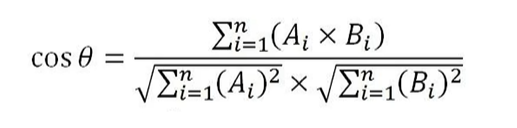 例举: 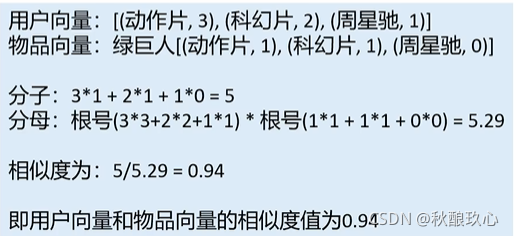
(5)优缺点
优点:
- 不需要其他用户的数据
- 能够具备独特口味的用户推荐
- 可以推荐最新的、冷门的物品
- 容易做推荐结果的解释
缺点:
- 很难找到能表达物品的“标签”,有时候需要人工打标签
- 过于局限于自己的世界,无法挖掘出用户的潜在兴趣
- 新用户如果没有行为,没办法做推荐
基于协同过滤的推荐系统
(1)协同过滤:使用行为数据,利用集体智慧推荐
a。基于用户的协同过滤-U2U2I:和你兴趣相投的人也喜欢xxx 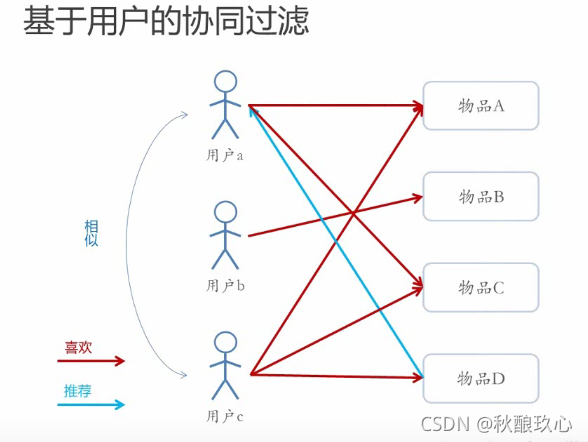
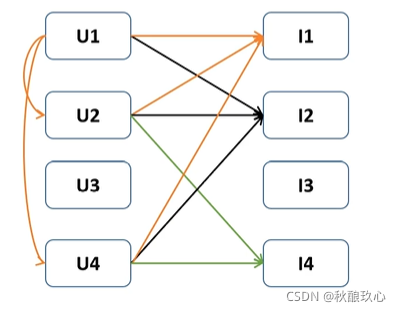 b。基于物品的协同过滤-U2I2I:喜欢这个物品的人也喜欢xxx 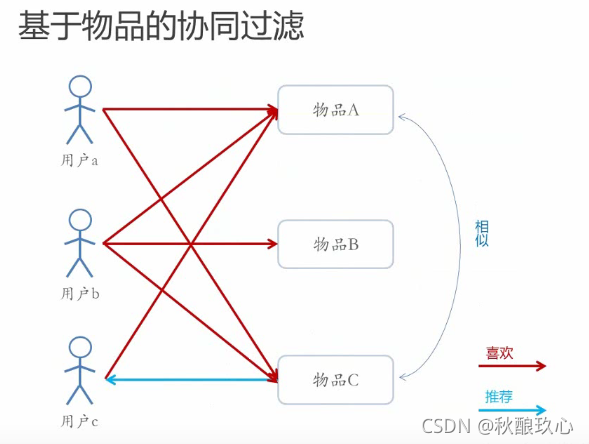
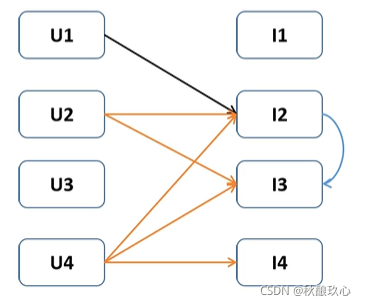 (2)实例:基于用户的协同过滤
步骤1:搜索最相似的用户 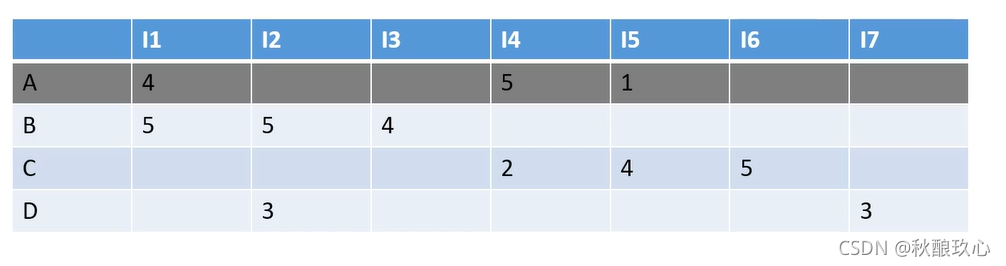 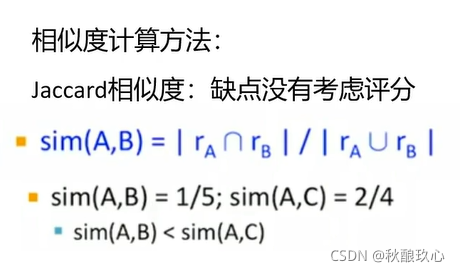 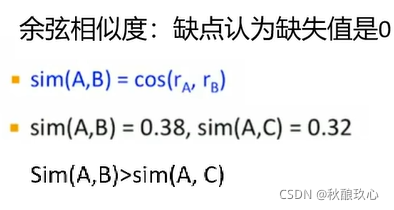 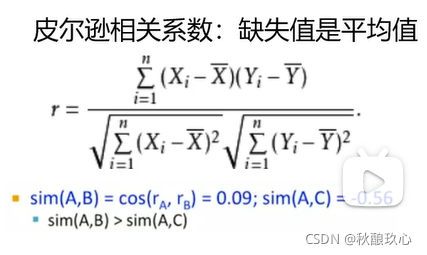
步骤2:计算u和新item的相似度 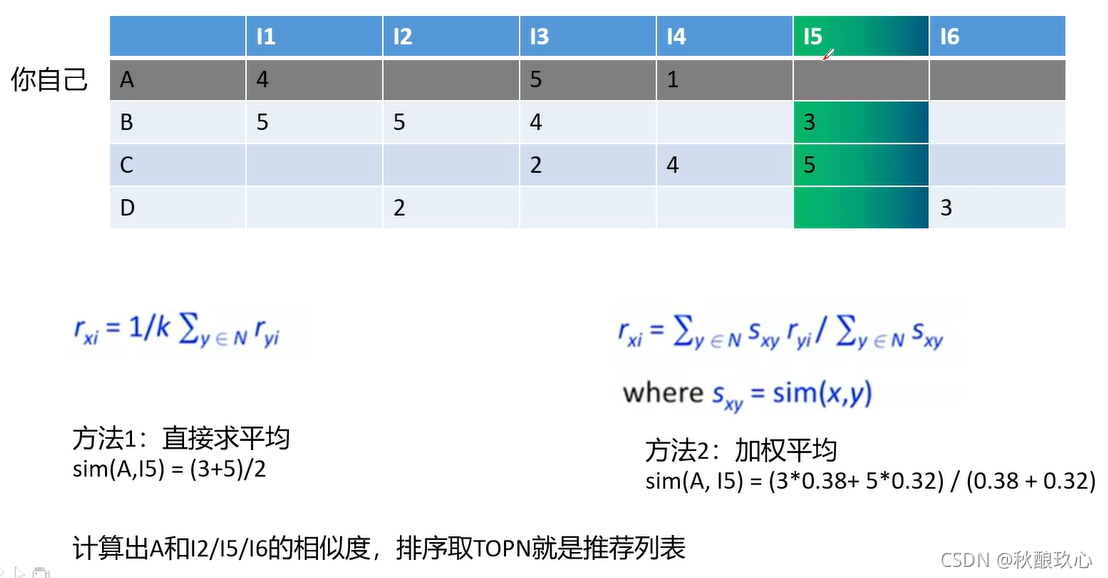
推荐系统实现内容相似推荐
计算物品最相似的其他物品列表,直接用I2I相似推荐,或者U2I2I扩展推荐
步骤: 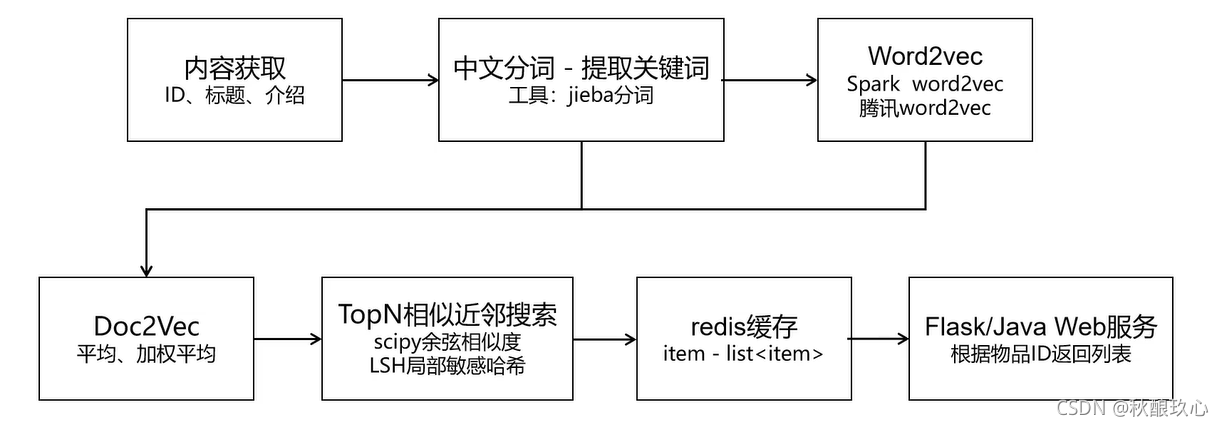
推荐系统评测
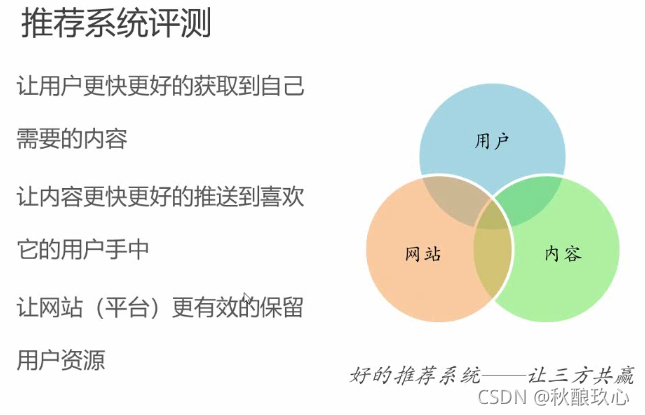
|