一、故事背景
石原里美小姐姐可真是个磨人的小妖精,上次讲到python中值滤波、最大池化、平均池化、canny边缘检测(石原里美系列一)让你修复了椒盐噪声和生成简笔画。现在秋招了,石原里美小姐姐也加入到了秋招的大军中,但是遇到一个问题,秋招需要上传个人照片,要求图像的尺寸较大。但是,石原里美小姐姐用的老人机,只有几百万像素,拍不了高清大图,于是又找到了身为技术宅的你。聪明的你,立马想到了最近邻插值、双线性插值的方法来做。
二、最近邻插值
所谓最近邻插值,就是当图像进行尺度缩放时,直接找最近的像素点进行填充。比如第一个矩阵经过最近邻插值进行二倍的扩大: 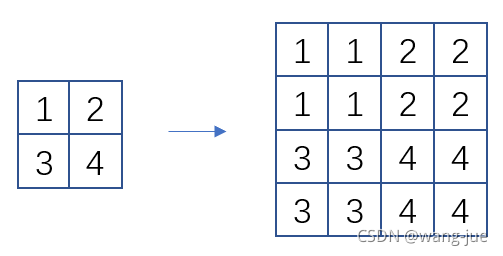
def nearest(src,dst,shape):
img = np.array(Image.open(src))
srcH,srcW,_ = img.shape
rateH,rateW = srcH/shape[0],srcW/shape[1]
newimg = np.zeros((shape[0],shape[1],3),dtype=np.uint8)
for i in range(shape[0]):
for j in range(shape[1]):
xx = min(round(rateH*i),srcH-1)
yy = min(round(rateW*j),srcW-1)
newimg[i,j] = img[xx,yy]
dst_img = Image.fromarray(newimg.astype('uint8'))
dst_img.save(dst)
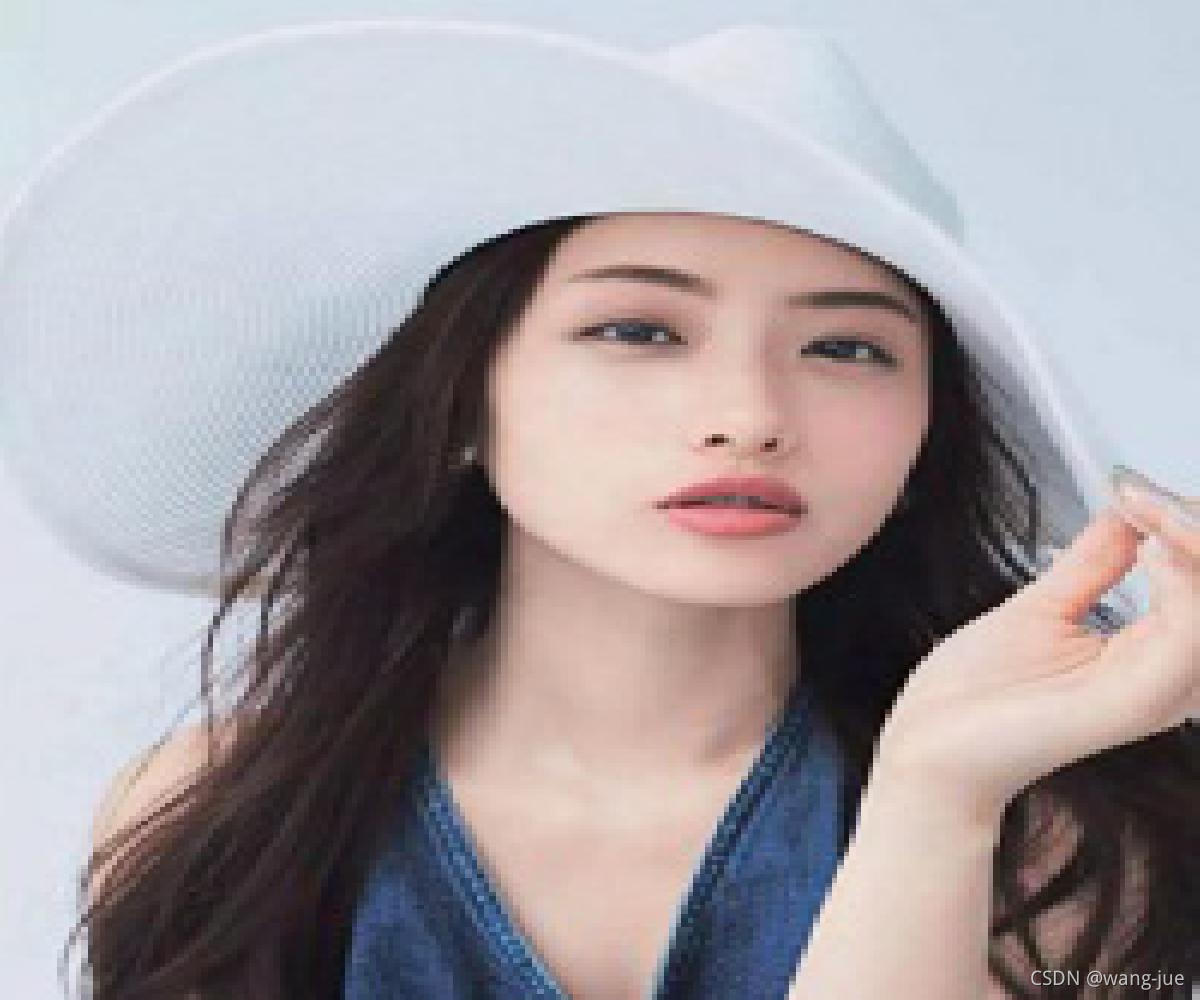
三、双线性插值
石原里美小姐姐收到照片之后不高兴了,质问到,为什么隔壁老王修的照片没有模糊,你这缩放的图像怎么锯齿状这么明显呢?于是,聪明的你又想到了双线性插值的方法。双线性插值就是像素点在进行采样时考虑到上下左右四个相邻像素点,然后根据四个像素点的距离进行插值运算。 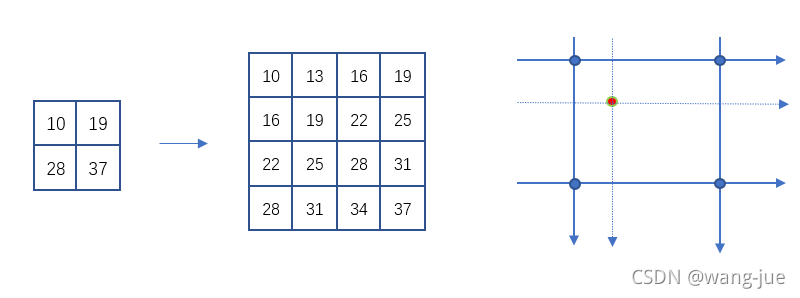 思想图如上所示,下面开始敲代码:
def biniliar(src,dst,shape):
img = np.array(Image.open(src))
srcH, srcW, _ = img.shape
rateH, rateW = srcH / shape[0], srcW / shape[1]
newimg = np.zeros((shape[0], shape[1], 3), dtype=np.uint8)
for i in range(shape[0]):
for j in range(shape[1]):
src_i = rateH * i
src_j = rateW * j
floor_i = min(math.floor(src_i),srcH-1)
floor_j = min(math.floor(src_j),srcW-1)
ceil_i = min(floor_i+1,srcH-1)
ceil_j = min(floor_j+1,srcW-1)
xx, yy = src_i - floor_i, src_j - floor_j
newimg[i, j] = (1-xx)*(1-yy)*img[floor_i, floor_j] + xx*(1-yy)*img[ceil_i, floor_j] + (1-xx)*yy*img[floor_i,ceil_j] + xx*yy*img[ceil_i,ceil_j]
dst_img = Image.fromarray(newimg.astype('uint8'))
dst_img.save(dst)
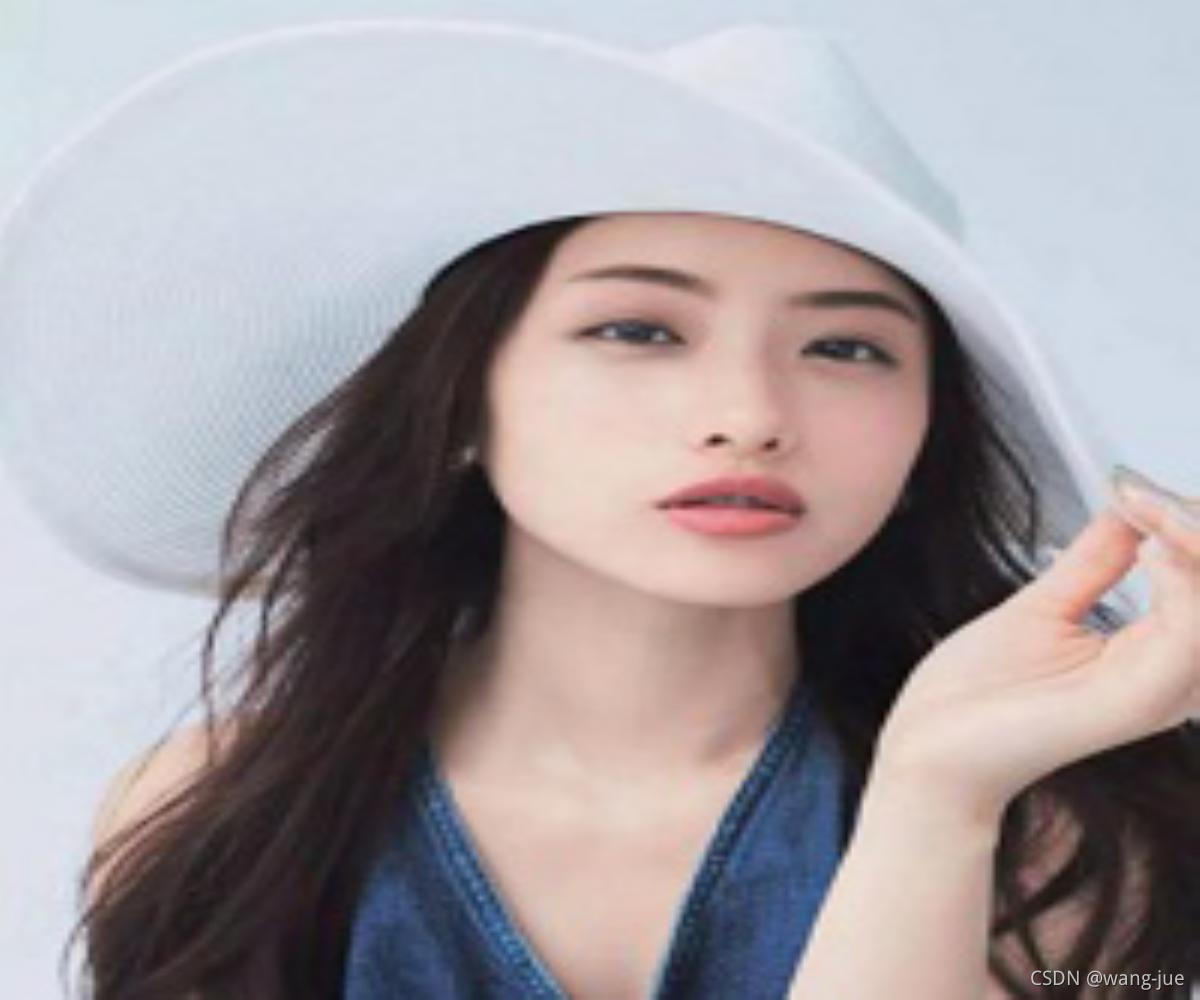 经过双线性插值处理,考虑几个近邻像素并进行线性计算,并未直接进行近邻复制(马赛克形状)。可把石原里美高兴坏了,表示愿意和你一起共进晚餐。
|