一、向量/张量
? ? ? ? 向量是一维的张量,而张量可以理解为一个树形结构的数据。例如,向量

? ? ? ? 所对应的树形结构数据为
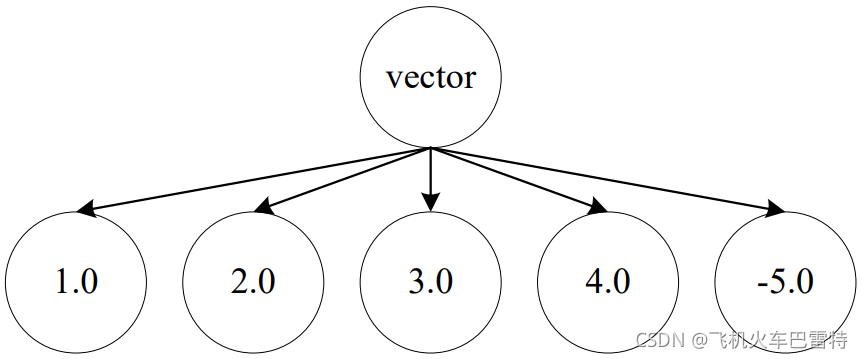
? ? ? ? 其中,vector节点是dim=0的张量,对应树高为0,节点1.0、2.0等是dim=1的张量,对应树高为1。根据定义,vector的L1范数和L2范数计算方式如下:
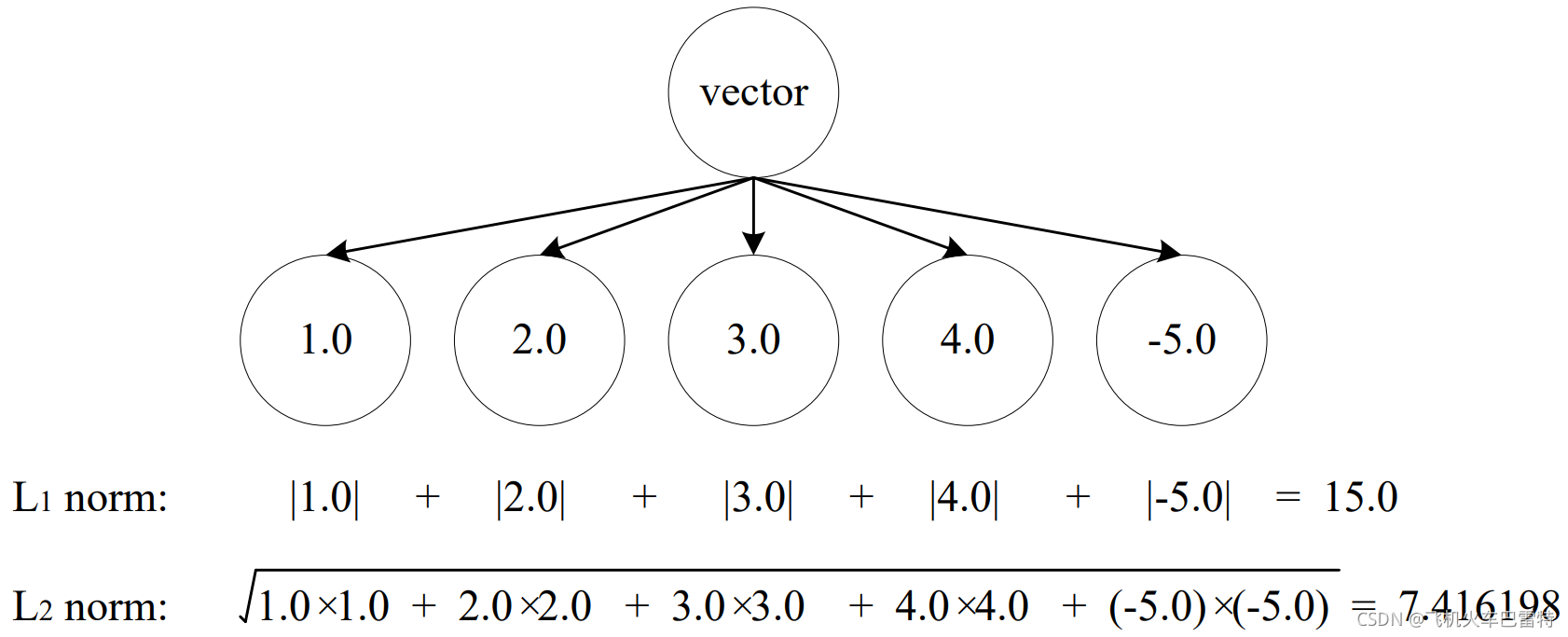
二、代码+输出
import torch
# Pytorch求向量的L1范数或L2范数
def tc04():
# 向量(1维张量)
vector = torch.tensor([1.0, 2.0, 3.0, 4.0, -5.0])
# 求向量的L1范数
norm1 = torch.norm(vector, p=1, dim=0)
# 求向量的L2范数
norm2 = torch.norm(vector, p=2, dim=0)
print("The L1 norm of %s is %f. " % (str(vector), norm1.item()))
print("The L2 norm of %s is %f. " % (str(vector), norm2.item()))
# 程序入口
if __name__ == '__main__':
tc04()
? ? ? ? 输出
The L1 norm of tensor([ 1., 2., 3., 4., -5.]) is 15.000000.
The L2 norm of tensor([ 1., 2., 3., 4., -5.]) is 7.416198.
Process finished with exit code 0
|