论文地址:https://arxiv.org/pdf/2104.00416.pdf https://arxiv.org/pdf/2104.00416.pdf
代码:GitHub - LongguangWang/DASR: [CVPR 2021] Unsupervised Degradation Representation Learning for Blind Super-Resolution https://github.com/LongguangWang/DASR?
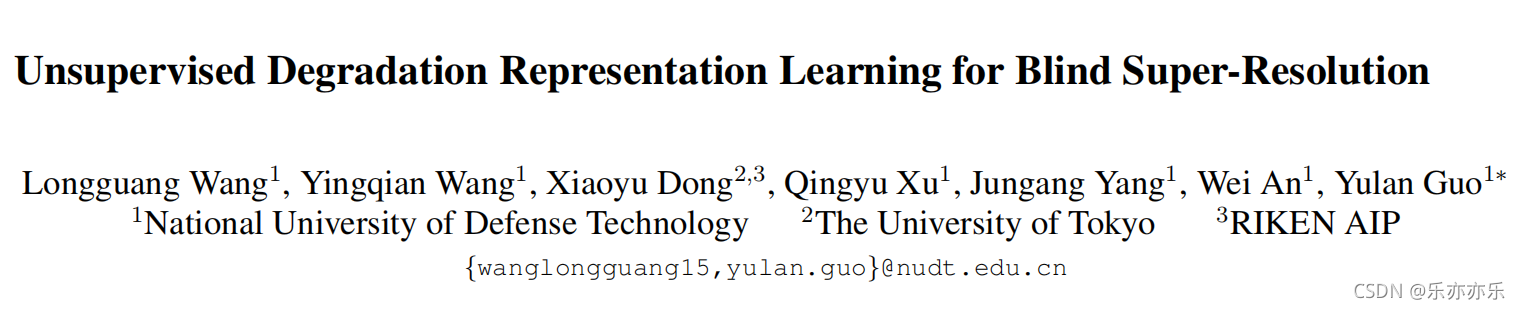
?为了处理现实应用中的各种未知退化,以前的方法依赖于退化估计来重建SR图像。
本文学习抽象表示来区分表示空间中的各种退化,而不是像素空间中的显式估计。提出Degradation-Aware SR (DASR) 网络。
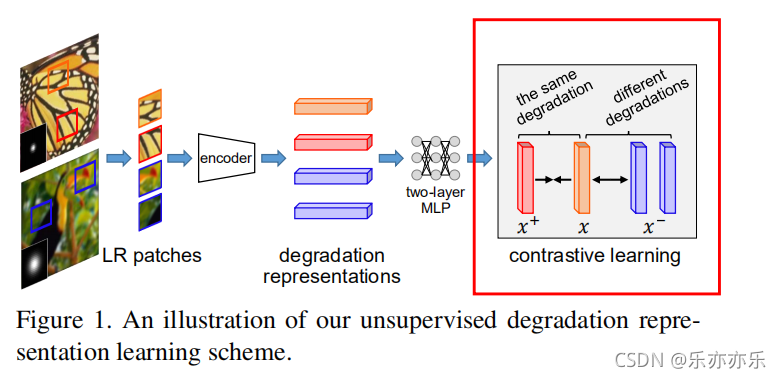
?如图1,对比损失对比潜在空间中的正负样本来进行无监督退化表示学习。有两个优点:
-
与提取完整的表示来估计退化相比,它更容易学习抽象表示来区分不同的退化。因此,可以获得一个判别退化表示,以提供准确的退化信息 -
退化表征学习不需要来自ground truth 退化的监督。因此,它可以以无监督的方式进行,更适合于未知退化的真实应用。
对比学习在无监督学习中非常有效。对比学习不是使用预定义和固定的目标,而是使表示空间中的互信息最大化。
如图1所示,图像块应该与同一图像中的其他块(即相同的退化)相似,而在退化表示空间中与其他图像中的patches(即不同的退化)不相似。假设每幅图像的退化是相同的,不同图像是不相同的。给定一个图像块(在图1中用一个橙色的方框进行注释)作为查询patches,从同一LR图像中提取的其他图像块(例如,带有红框注释的图像块)可以被视为正样本。相比之下,来自其他LR图像的图像块(例如,用蓝色框注释的图像块)可以被称为负样本。
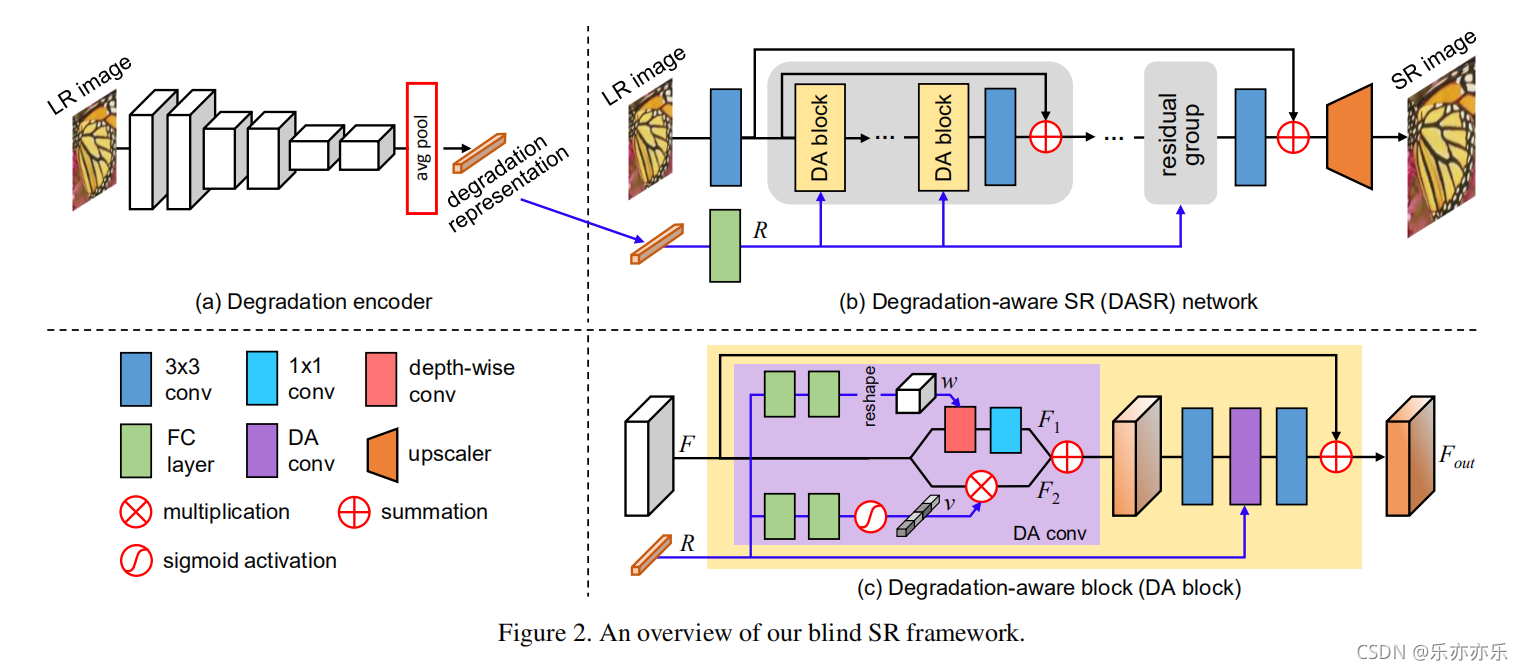
?
?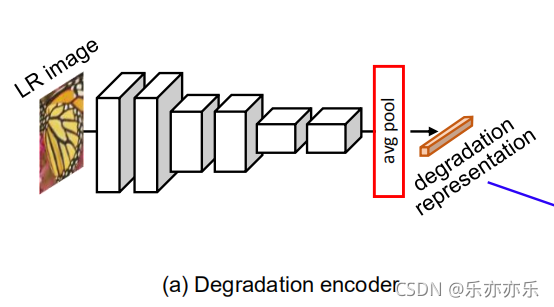
?
如图2(a)所示,使用一个具有六层的卷积网络将query、positive和negative patches编码为退化表示(degradation representations)?。所得到的表示被进一步输入到一个双层多层感知器(MLP)映射,以获得x、x+和x-。鼓励x类似于x+,而不同于x-。如下公式测量相似度:
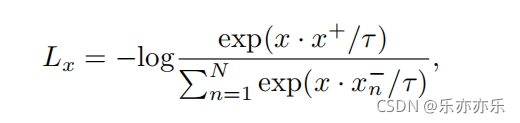
?N是负样本的个数,T是一个teamperature hyper-parameter 。· 代表两个向量的点乘。
temperature parameter
这个t 叫做温度参数,我们加入到softmax中看看会有什么效果。假设我们处理的是一个三分类问题,模型的输出是一个3维向量:
[1,2,3]
然后计算交叉熵损失,首先我们要通过一个softmax layer,softmax公式大家都很熟悉:
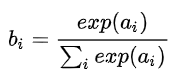
我们得到结果:
[0.09003057317038046, 0.24472847105479767, 0.6652409557748219]
我们让t=2,其实也就是让[1/2,2/2,3/2]计算softmax,得到结果:
[0.1863237232258476, 0.30719588571849843, 0.506480391055654]
再让t=0.5,让[1/0.5,2/0.5,3/0.5]计算softmax,得到结果:
[0.015876239976466765, 0.11731042782619835, 0.8668133321973348]
看到区别了吗,t 越大,结果越平滑,让本来t=1 时大的结果变小一点,小的变大一点,得到的概率分布更“平滑”,相应的t 越小,得到的概率分布更“尖锐”,这就是t 的作用。
此处来源:深度学习中的temperature parameter是什么 - 知乎问题来源在google的论文《Sampling-Bias-Corrected Neural Modeling for Large Corpus Item Recommendations》中看到这个公式: s(x,y)=<u(x,\theta),v(y,\theta)>/\tau 对于这个 t很好奇。 先简单介绍一下这… https://zhuanlan.zhihu.com/p/132785733
?总体损失定义如下:
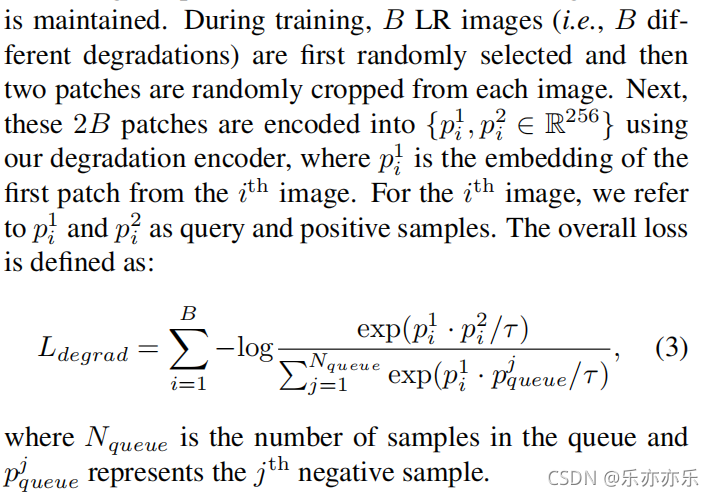
?
Degradation-Aware SR Network
DASR网络结构如图2(b)所示:
?
?从上图可以看到,网络由5个residual groups 组成,每个residual group由5个DA block组成。
在每个DA块中,使用两个DA conv(图中紫色部分)基于退化表示来适应特征,如图2(c)所示。
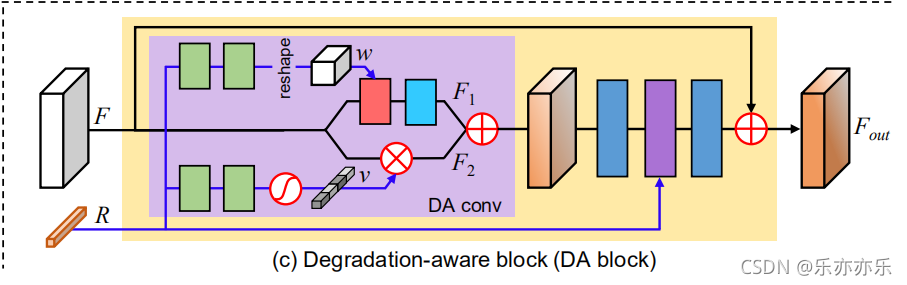
?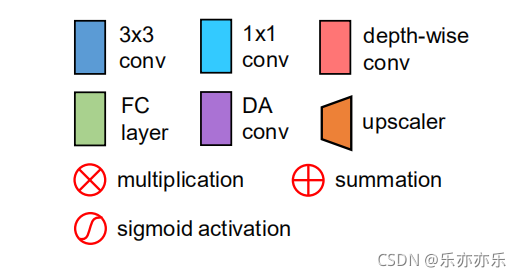
degradation representation R 传进两个全连接FC layer(代码中使用1x1 conv),并reshape 成一个kernel w (w
∈
R
C
×
1
×
3
×
3)。然后,输入的特征 F 被一个3x3 的depth-wise 卷积(使用核w)处理,随后利用一个1x1卷积生成F1.
受CResMD(使用控制变量重新缩放不同的通道以处理多个退化)的启发,DA conv 还学习了生成基于退化表示的调制系数,以执行通道级的特征适应。R 通过另外两个全连接层FC layer,使用sigmoid激活函数生成channel-wise 调制系数v。然后,用v重新缩放F中不同的通道分量,得到F2。最后,将F1与F2求和,并输入后续层,产生输出特征F out。
?
代码:?
?common.py
import math
import torch
import torch.nn as nn
import torch.nn.functional as F
def default_conv(in_channels, out_channels, kernel_size, bias=True):
return nn.Conv2d(in_channels, out_channels, kernel_size, padding=(kernel_size//2), bias=bias)
class MeanShift(nn.Conv2d):
def __init__(self, rgb_range, rgb_mean, rgb_std, sign=-1):
super(MeanShift, self).__init__(3, 3, kernel_size=1)
std = torch.Tensor(rgb_std)
self.weight.data = torch.eye(3).view(3, 3, 1, 1)
self.weight.data.div_(std.view(3, 1, 1, 1))
self.bias.data = sign * rgb_range * torch.Tensor(rgb_mean)
self.bias.data.div_(std)
self.weight.requires_grad = False
self.bias.requires_grad = False
class Upsampler(nn.Sequential):
def __init__(self, conv, scale, n_feat, act=False, bias=True):
m = []
if (scale & (scale - 1)) == 0: # Is scale = 2^n?
for _ in range(int(math.log(scale, 2))):
m.append(conv(n_feat, 4 * n_feat, 3, bias))
m.append(nn.PixelShuffle(2))
if act: m.append(act())
elif scale == 3:
m.append(conv(n_feat, 9 * n_feat, 3, bias))
m.append(nn.PixelShuffle(3))
if act: m.append(act())
else:
raise NotImplementedError
super(Upsampler, self).__init__(*m)
DA conv 红框紫色对应的地方。
?其中下面生成调制系数v的分支是通过通道注意力CA_layer实现的,全连接层FC layer(绿色矩形)是使用1x1卷积实现。
# 通道注意力 对应Figure2(C) DA conv下面分支
# 1x1 卷积相当于 FC layer
class CA_layer(nn.Module):
def __init__(self, channels_in, channels_out, reduction):
super(CA_layer, self).__init__()
self.conv_du = nn.Sequential(
nn.Conv2d(channels_in, channels_in//reduction, 1, 1, 0, bias=False),
nn.LeakyReLU(0.1, True),
nn.Conv2d(channels_in // reduction, channels_out, 1, 1, 0, bias=False),
nn.Sigmoid()
)
def forward(self, x):
'''
:param x[0]: feature map: B * C * H * W
:param x[1]: degradation representation: B * C
'''
att = self.conv_du(x[1][:, :, None, None])
return x[0] * att
class DA_conv(nn.Module):
def __init__(self, channels_in, channels_out, kernel_size, reduction):
super(DA_conv, self).__init__()
self.channels_out = channels_out
self.channels_in = channels_in
self.kernel_size = kernel_size
self.kernel = nn.Sequential(
nn.Linear(64, 64, bias=False),
nn.LeakyReLU(0.1, True),
nn.Linear(64, 64 * self.kernel_size * self.kernel_size, bias=False)
)
self.conv = common.default_conv(channels_in, channels_out, 1)
self.ca = CA_layer(channels_in, channels_out, reduction)
self.relu = nn.LeakyReLU(0.1, True)
def forward(self, x):
'''
:param x[0]: feature map: B * C * H * W
:param x[1]: degradation representation: B * C
'''
b, c, h, w = x[0].size()
# branch 1
kernel = self.kernel(x[1]).view(-1, 1, self.kernel_size, self.kernel_size) #图2中白色块w
# groups=b*c 相当于depth-wise conv
out = self.relu(F.conv2d(x[0].view(1, -1, h, w), kernel, groups=b*c, padding=(self.kernel_size-1)//2))
# 通过1x1 卷积 相当于图2中蓝色矩形1x1 conv
out = self.conv(out.view(b, -1, h, w))
# branch 2
out = out + self.ca(x) #相当于out=F1+F2
return out
?DA block?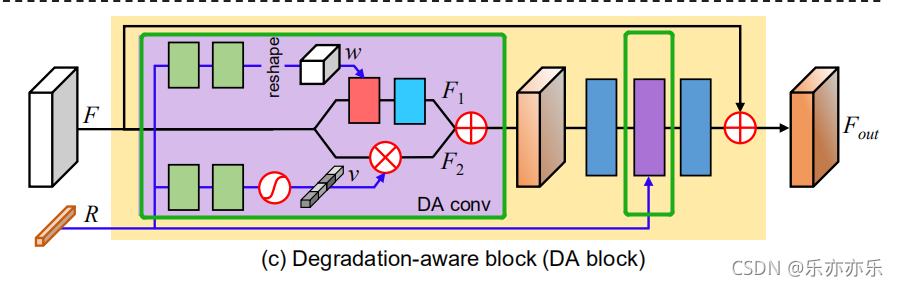
DA block 里面包含两个DA conv,每个conv后跟着一个3x3的卷积(蓝色矩形)。包含两个残差连接。?
class DAB(nn.Module):
def __init__(self, conv, n_feat, kernel_size, reduction):
super(DAB, self).__init__()
# 每个DAB中包含两个DA convolutional layer
self.da_conv1 = DA_conv(n_feat, n_feat, kernel_size, reduction)
self.da_conv2 = DA_conv(n_feat, n_feat, kernel_size, reduction)
self.conv1 = conv(n_feat, n_feat, kernel_size)
self.conv2 = conv(n_feat, n_feat, kernel_size)
self.relu = nn.LeakyReLU(0.1, True)
def forward(self, x):
'''
:param x[0]: feature map: B * C * H * W
:param x[1]: degradation representation: B * C
'''
# 对应图2(c)
# DA conv -> 3x3conv -> DA conv ->3x3conv 含有两个残差连接
out = self.relu(self.da_conv1(x))
out = self.relu(self.conv1(out))
out = self.relu(self.da_conv2([out, x[1]]))
out = self.conv2(out) + x[0]
return out
?DAG 图中灰色部分所示,堆叠n_blocks个DA block,后接一个3x3卷积(论文中n_blocks为5)。
class DAG(nn.Module):
def __init__(self, conv, n_feat, kernel_size, reduction, n_blocks):
super(DAG, self).__init__()
self.n_blocks = n_blocks
modules_body = [
DAB(conv, n_feat, kernel_size, reduction) \
for _ in range(n_blocks)
]
modules_body.append(conv(n_feat, n_feat, kernel_size))
# Figure 2(b) 中灰色部分所示 堆叠n个DA block + 3x3conv
self.body = nn.Sequential(*modules_body)
def forward(self, x):
'''
:param x[0]: feature map: B * C * H * W
:param x[1]: degradation representation: B * C
'''
res = x[0]
for i in range(self.n_blocks):
res = self.body[i]([res, x[1]])
res = self.body[-1](res) # 3x3卷积
res = res + x[0]
return res
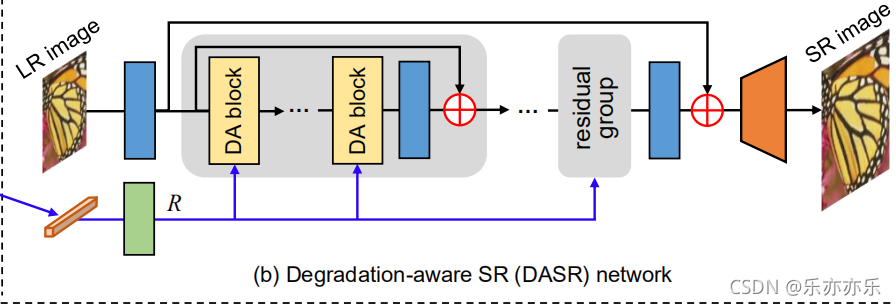
?DASR整体结构代码:
# Figure 2 (b) Degradation-aware SR (DASR) network
class DASR(nn.Module):
def __init__(self, args, conv=common.default_conv): # conv = 传入默认的3x3卷积
super(DASR, self).__init__()
self.n_groups = 5
n_blocks = 5
n_feats = 64
kernel_size = 3
reduction = 8
scale = int(args.scale[0])
# RGB mean for DIV2K
rgb_mean = (0.4488, 0.4371, 0.4040)
rgb_std = (1.0, 1.0, 1.0)
self.sub_mean = common.MeanShift(255.0, rgb_mean, rgb_std)
self.add_mean = common.MeanShift(255.0, rgb_mean, rgb_std, 1)
# head module
modules_head = [conv(3, n_feats, kernel_size)] # 先通过3X3 conv
self.head = nn.Sequential(*modules_head)
# compress
self.compress = nn.Sequential(
nn.Linear(256, 64, bias=False),
nn.LeakyReLU(0.1, True)
)
# body # n个(论文中取5)residual groups 后接 3x3 卷积
modules_body = [
DAG(common.default_conv, n_feats, kernel_size, reduction, n_blocks) \
for _ in range(self.n_groups)
]
modules_body.append(conv(n_feats, n_feats, kernel_size))
self.body = nn.Sequential(*modules_body)
# tail 上采样 论文图2中 upscaler
modules_tail = [common.Upsampler(conv, scale, n_feats, act=False),
conv(n_feats, 3, kernel_size)]
self.tail = nn.Sequential(*modules_tail)
def forward(self, x, k_v):
k_v = self.compress(k_v)
# sub mean
x = self.sub_mean(x)
# head
x = self.head(x)
# body
res = x # 用于残差连接
for i in range(self.n_groups):
res = self.body[i]([res, k_v])
res = self.body[-1](res)
res = res + x
# tail
x = self.tail(res)
# add mean
x = self.add_mean(x)
return x
# Figure 2中(a)Degradation encoder
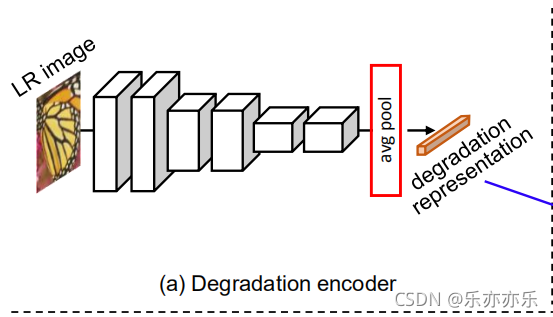
?编码部分生成degradation representation R。由6个基本的卷积块(Conv->BN->LReLU)组成。
# Figure 2中(a)Degradation encoder
class Encoder(nn.Module):
def __init__(self):
super(Encoder, self).__init__()
self.E = nn.Sequential(
nn.Conv2d(3, 64, kernel_size=3, padding=1),
nn.BatchNorm2d(64),
nn.LeakyReLU(0.1, True),
nn.Conv2d(64, 64, kernel_size=3, padding=1),
nn.BatchNorm2d(64),
nn.LeakyReLU(0.1, True),
nn.Conv2d(64, 128, kernel_size=3, stride=2, padding=1),
nn.BatchNorm2d(128),
nn.LeakyReLU(0.1, True),
nn.Conv2d(128, 128, kernel_size=3, padding=1),
nn.BatchNorm2d(128),
nn.LeakyReLU(0.1, True),
nn.Conv2d(128, 256, kernel_size=3, stride=2, padding=1),
nn.BatchNorm2d(256),
nn.LeakyReLU(0.1, True),
nn.Conv2d(256, 256, kernel_size=3, padding=1),
nn.BatchNorm2d(256),
nn.LeakyReLU(0.1, True),
# avg pool
nn.AdaptiveAvgPool2d(1),
)
self.mlp = nn.Sequential(
nn.Linear(256, 256),
nn.LeakyReLU(0.1, True),
nn.Linear(256, 256),
)
def forward(self, x):
fea = self.E(x).squeeze(-1).squeeze(-1)
out = self.mlp(fea)
return fea, out
更详细的内容阅读原文及源代码!?
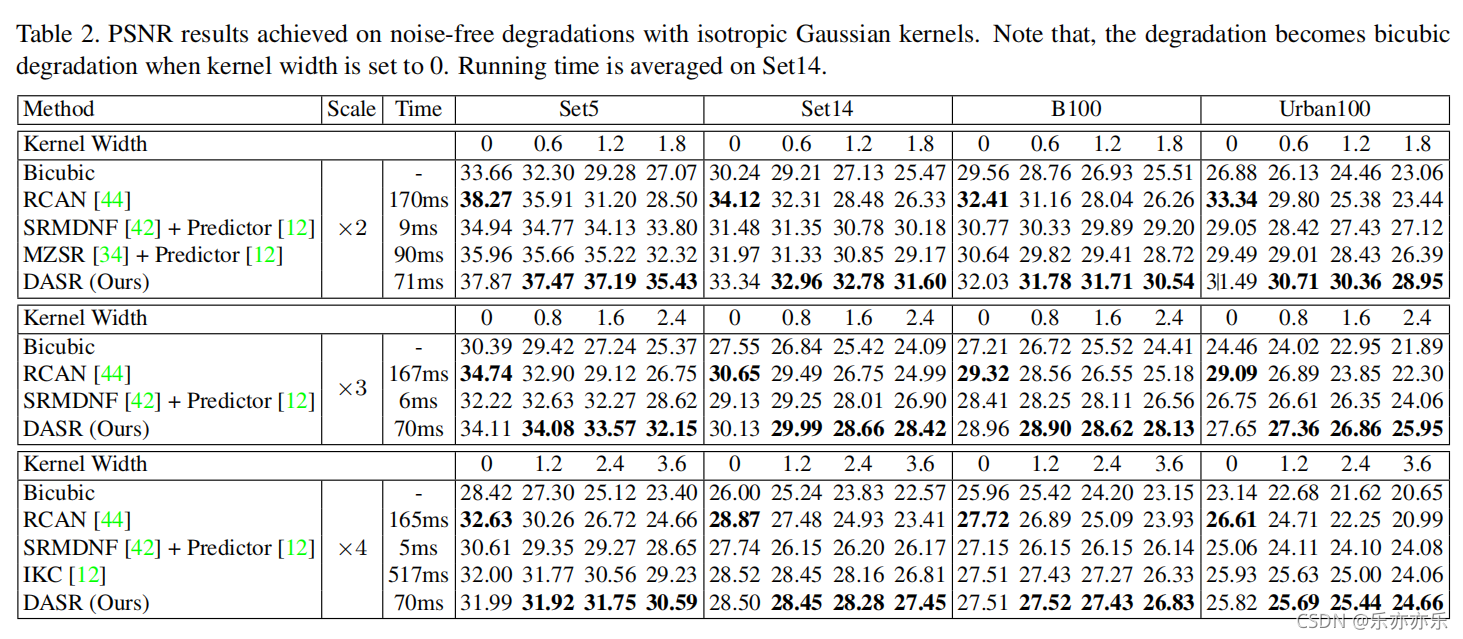
?
?
|