结论
过滤法更快速,但更粗糙。包装法和嵌入法更精确,比较适合具体到算法去调整,但计算量比较大,运行时间长。当数据量很大的时候,优先使用方差过滤和互信息法调整,再上其他特征选择方法。使用逻辑回归时,优先使用嵌入法。使用支持向量机时,优先使用递归消除特征。
1、读数据,定义测试函数
import pandas as pd
data = pd.read_csv(r'F:\教师培训\ppd7\df_Master_merge_clean.csv',encoding='gb18030')
pd_x = data[data.target.notnull()].drop(columns=['Idx', 'target', 'sample_status', 'ListingInfo'])
pd_y = data[data.target.notnull()]['target']
#定义画图auc函数
def get_auc(x, y):
from sklearn.model_selection import train_test_split
from sklearn.metrics import roc_auc_score, roc_curve
import lightgbm as lgb
#画出auc图的函数
def roc_auc_plot(clf,x_train,y_train,x_test, y_test):
train_auc = roc_auc_score(y_train,clf.predict_proba(x_train)[:,1])
train_fpr, train_tpr, _ = roc_curve(y_train,clf.predict_proba(x_train)[:,1])
train_ks = abs(train_fpr-train_tpr).max()
print('train_ks = ', train_ks)
print('train_auc = ', train_auc)
test_auc = roc_auc_score(y_test,clf.predict_proba(x_test)[:,1])
test_fpr, test_tpr, _ = roc_curve(y_test,clf.predict_proba(x_test)[:,1])
test_ks = abs(test_fpr-test_tpr).max()
print('test_ks = ', test_ks)
print('test_auc = ', test_auc)
from matplotlib import pyplot as plt
plt.plot(train_fpr,train_tpr,label = 'train_roc')
plt.plot(test_fpr,test_tpr,label = 'test_roc')
plt.plot([0,1],[0,1],'k--', c='r')
plt.xlabel('False positive rate')
plt.ylabel('True positive rate')
plt.title('ROC Curve')
plt.legend(loc = 'best')
plt.show()
x_train,x_test, y_train, y_test = train_test_split(x,y,random_state=2,test_size=0.2)
lgb_model = lgb.LGBMClassifier(n_estimators=800,
boosting_type='gbdt',
learning_rate=0.04,
min_child_samples=68,
min_child_weight=0.01,
max_depth=4,
num_leaves=16,
colsample_bytree=0.8,
subsample=0.8,
reg_alpha=0.7777777777777778,
reg_lambda=0.3,
objective='binary')
clf = lgb_model.fit(x_train, y_train,
eval_set=[(x_train, y_train),(x_test,y_test)],
eval_metric='auc',early_stopping_rounds=100)
roc_auc_plot(clf,x_train,y_train,x_test, y_test)
#定义基分类器
clf1 = lgb.LGBMClassifier(n_estimators=800,
boosting_type='gbdt',
learning_rate=0.04,
min_child_samples=68,
min_child_weight=0.01,
max_depth=4,
num_leaves=16,
colsample_bytree=0.8,
subsample=0.8,
reg_alpha=0.7777777777777778,
reg_lambda=0.3,
objective='binary')
2、各种方法如下
1.递归消除特征
递归特征消除法(Recursive feature elimination, 简写为RFE),使用很少的特征达到很优秀的效果。 它反复创建模型,并在每次迭代时保留最佳特征或剔除最差特征,下一次迭代时,它会使用上一次建模中没有被选中的特征来构建下一个模型,直到所有特征都耗尽为止。 然后,它根据自己保留或 剔除特征的顺序来对特征进行排名,最终选出一个最佳子集。
代码如下(示例):
from sklearn.feature_selection import RFE
#只有fit,表示这是一个选择器,可以有属性
#n_features_to_select:是想要选择的特征个数
#support_:返回所有的特征的是否最后被选中的布尔矩阵
#ranking_:返回特征的按数次迭代中综合重要性的排名
#step表示每次迭代中希望移除的特征个数
c = RFE(clf1, n_features_to_select=i, step=50).fit(pd_x, pd_y)
c.support_.sum()
c.ranking_
#fit_transform返回筛选后的数据集
c = RFE(clf1, n_features_to_select=i, step=50).fit_transform(pd_x, pd_y)
##########################################################################
#利用交叉验证选择最优的特征个数,运行速度太长了,此结果没有出来,所以无法展示验证
axisx = range(1,250,10)
scores = []
for i in axisx:
x_wrapper = RFE(clf1, n_features_to_select=i, step=50).fit_transform(pd_x, pd_y)
score = cross_val_score(clf1,x_wrapper, pd_y, cv=5).mean()
scores.append(score)
plt.figure(figsize=(20,5))
plt.plot(axisx,scores, label='n_features_to_select-score')
plt.xticks(axisx)
plt.legend()
plt.show()
#如图筛选出最优特征个数113
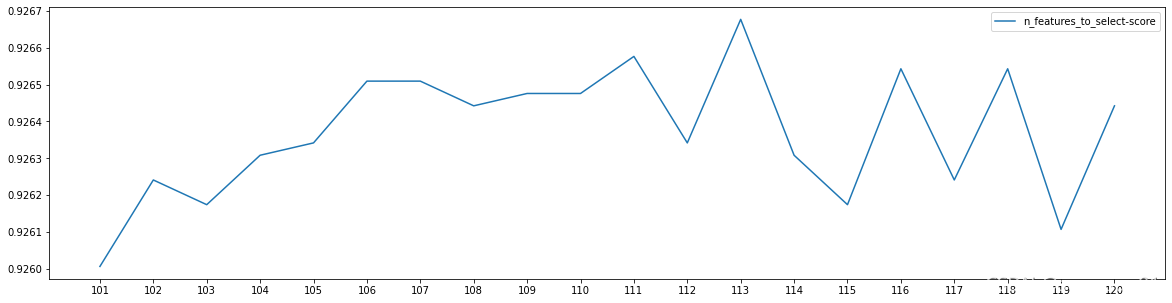
x_rfe = RFE(clf1, n_features_to_select=113, step=50).fit_transform(pd_x, pd_y)
#画出auc曲线
get_auc(x_rfe, pd_y)
#416个特征降到113个特征,效果从test_auc = 0.74109449149997降到0.7322830868482363
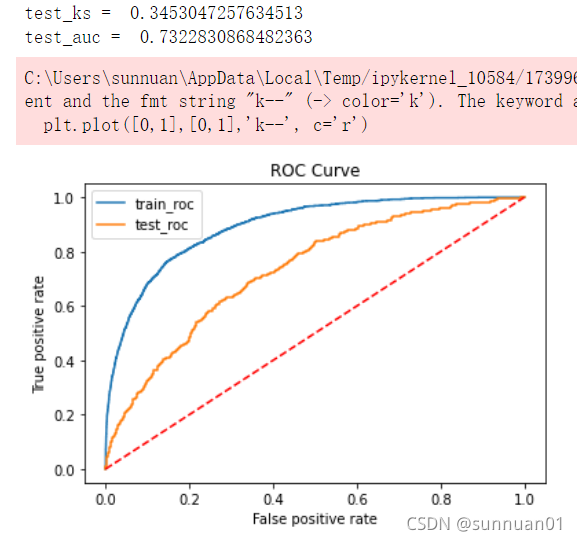
2.Embedded嵌入法
代码如下(示例): 1.先使用某些机器学习的算法和模型进行训练,得到各个特征的权值系数,根据权值系数从大到小选择特征,这些权值系数往往代表了特征对于模型的某种贡献或某种重要性,比如决策树和树的集成模型中的feature_importances_属性,可以列出各个特征对树的建立的贡献,我们就可以基于这种贡献的评估,找出对模型建立最有用的特征。
from sklearn.feature_selection import SelectFromModel
from sklearn.model_selection import cross_val_score
#estimator:使用的模型评估器,只要是带feature_importances_或者coef_属性,或带有l1和l2惩罚项的模型都可以使用
#threshold:特征重要性的阈值,重要性低于这个阈值的特征都将被删除
# 选取重要性的最大值,目的是为了构造axisx,选择最优的threshold
m = clf1.fit(pd_x, pd_y).feature_importances_.max()
axisx = range(0,m+1,10)
#寻找最优threshold函数
def get_opt_thre(axisx):
scores = []
for i in axisx:
#threshold特征重要性的阈值,重要性低于这个阈值的特征都将被删除
embedded_x = SelectFromModel(clf1, threshold=i).fit_transform(pd_x, pd_y)
score = cross_val_score(clf1, embedded_x,pd_y, cv=5).mean()
scores.append(score)
plt.figure(figsize=(20,5))
plt.plot(axisx, scores, label='threshold-scores')
plt.xticks(axisx)
plt.legend()
plt.show()
get_opt_thre(axisx)
#观察下图,最优值在130左右,最大的特征重要分数是140,这样选择选到的特征极度少,所以不选取此值,推断可能是评价指标的问题。
选择30
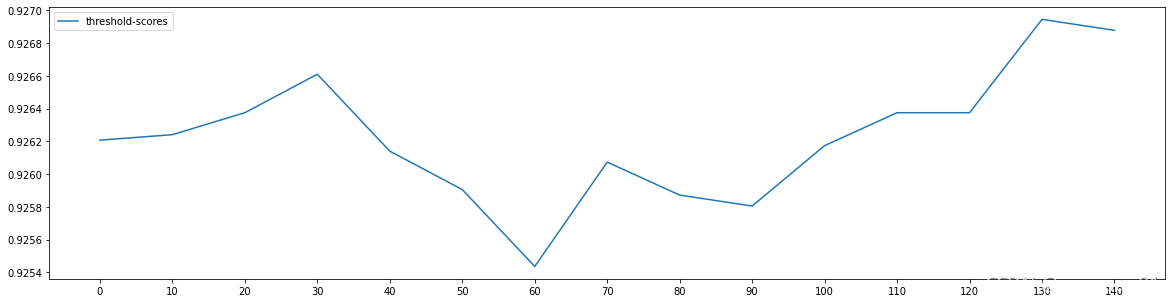
axisx = range(25,36,1)
get_opt_thre(axisx)
#从图像上来看,随着阈值越来越高,模型的效果逐渐变差,被删除的特征越来越多,信息损失也逐渐变大
embedded_x = SelectFromModel(clf1, threshold=29).fit_transform(pd_x, pd_y)
get_auc(embedded_x, pd_y)
#416个特征降到120个特征,效果从test_auc = 0.74109449149997降到0.7269277027467951
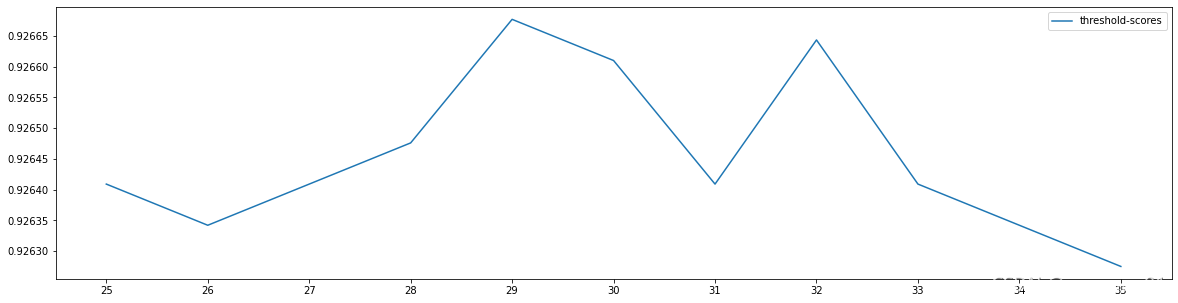 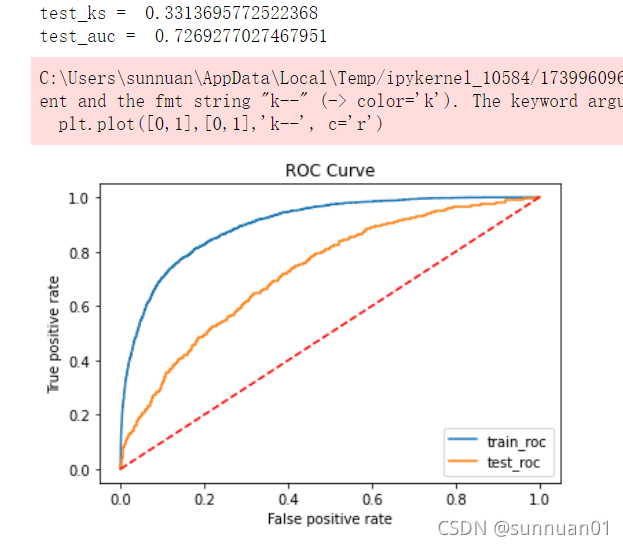
3.相关性过滤之互信息法
互信息法是用来捕捉每个特征与标签之间的任意关系(包括线性和非线性关系)的过滤方法 feature_selection.mutual_info_classif(互信息分类)和feature_selection.mutual_info_regression(互信息回归)。互信息法比F检验更加强大,F检验只能够找出线性关系,而互信息法可以找出任意关系。互信息法不返回p值或F值类似的统计量,它返回“每个特征与目标之间的互信息量的估计”,这个估计量在[0,1]之间取值,为0则表示两个变量独立,为1则表示两个变量完全相关。以互信息分类为例的代码如下:
from sklearn.feature_selection import mutual_info_classif
res = mutual_info_classif(pd_x, pd_y)
mc = pd.DataFrame({'fname':pd_x.columns, 'micv':res})
f = mc[mc.micv>0]['fname']
get_auc(pd_x[f], pd_y)
#416个特征降到271个特征,效果从test_auc = 0.74109449149997降到0.7373713932080141
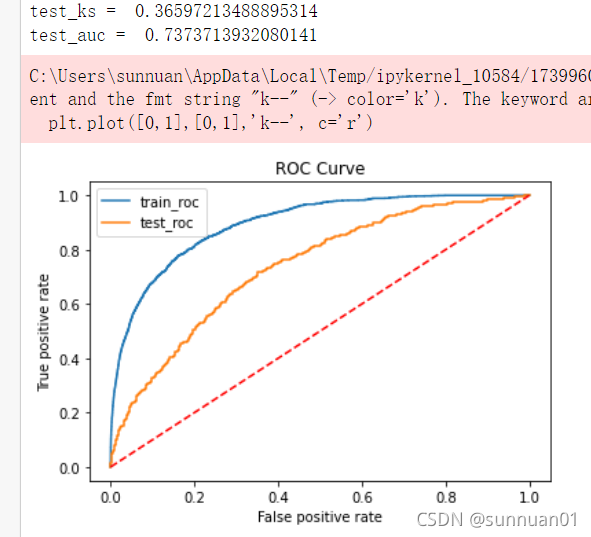
4.相关性过滤之F检验
# F检验,又称ANOVA,方差齐性检验,是用来捕捉每个特征与标签之间的线性关系的过滤方法,
# F检验分类用于标签是离散型变量的数据,而F检验回归用于标签是连续型变量的数据。
#原假设是”数据不存在显著的线性关系“。它返回F值和p值两个统计量。和卡方过滤一样,我们希望选取p值小于#0.05或0.01的特征,
#这些特征与标签时显著线性相关的,而p值大于0.05或0.01的特征则被我们认为是和标签没有显著线性关系的特征,应该被删除。
from sklearn.feature_selection import f_classif
F, p = f_classif(x,y)
sf = pd.DataFrame({'fname':x.columns, 'p':p})
fname = sf[sf.p<0.05]['fname']
get_auc(x[fname], y)
#416个特征降到201个特征,效果从test_auc = 0.74109449149997降到0.7360001348353649
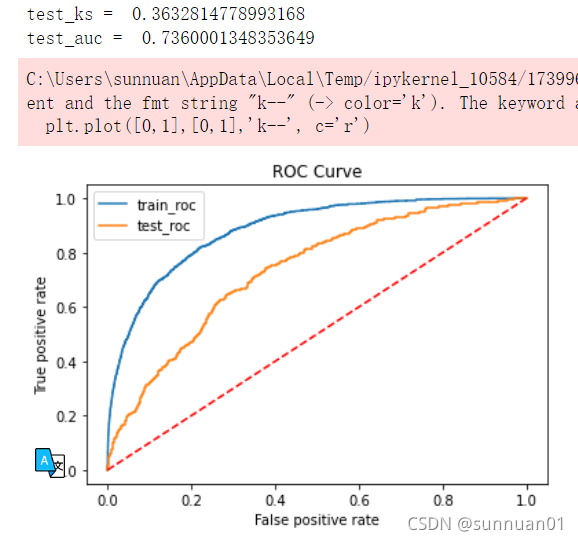
5.相关性过滤之F检验
#卡方过滤是专门针对离散型标签(即分类问题)的相关性过滤。卡方检验类feature_selection.chi2计算每个非负特征和标签之间的卡方统计量,并依照卡方统计量由高到低为特征排名。由于数据集中含有负值,故不采用这种方式.
from sklearn.feature_selection import chi2, SelectKBest
#假设在这里我一直我需要300个特征
x_chi = SelectKBest(chi2, k=300).fit_transform(pd_x, y)
et_auc(x_chi, y)
6.方差过滤
通过特征本身的方差来筛选特征的类。比如一个特征本身的方差很小,就表示样本在这个特征上基本没有差异,可能特征中的大多数值都一样,甚至整个特征的取值都相同,那这个特征对于样本区分没有什么作用。所以无论接下来的特征工程要做什么,都要优先消除方差为0的特征。VarianceThreshold有重要参数threshold,表示方差的阈值,表示舍弃所有方差小于threshold的特征,不填默认为0,即删除所有的记录都相同的特征。
from sklearn.feature_selection import VarianceThreshold
selector = VarianceThreshold() #实例化,不填参数默认方差为0
X_var0 = selector.fit_transform(X) #获取删除不合格特征之后的新特征矩阵
#发现没有方差为0的特征
最近邻算法KNN,单棵决策树,支持向量机SVM,神经网络,回归算法,都需要遍历特征或升维来进 行运算,所以他们本身的运算量就很大,需要的时间就很长,因此方差过滤这样的特征选择对他们来说就尤为重要。 但对于不需要遍历特征的算法,比如随机森林,它随机选取特征进行分枝,本身运算就非常快速,因此特征选择对它来说效果平平。
总结
通过观察我们发现递归消除法的效果相对来说很好,其次是嵌入法的效果,针对不同的模型采用不同的方法,理论部分来自菜菜机器学习。
|