总结摘自高老师GitHub
https://github.com/OUCTheoryGroup/colab_demo/blob/master/202003_models/MobileNetV1_CIFAR10.ipynb
https://github.com/OUCTheoryGroup/colab_demo/blob/master/202003_models/MobileNetV2_CIFAR10.ipynb
MobileNet v1
在算法层面有效的压缩存储和计算量的方法,Mobilenet v1核心是把卷积拆分为epthwise+Pointwise两部分。
Depthwise 处理一个三通道的图像,使用3×3的卷积核,完全在二维平面上进行,卷积核的数量与输入的通道数相同,所以经过运算会生成3个feature map。卷积的参数为: 3 × 3 × 3 = 27,如下所示:
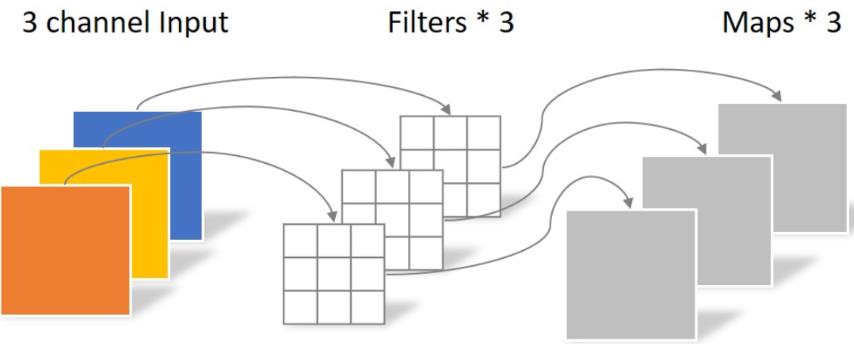
Pointwise 不同之处在于卷积核的尺寸为1×1×k,k为输入通道的数量。所以,这里的卷积运算会将上一层的feature map加权融合,有几个filter就有几个feature map,参数数量为:1 × 1 × 3 × 4 = 12,如下图所示:
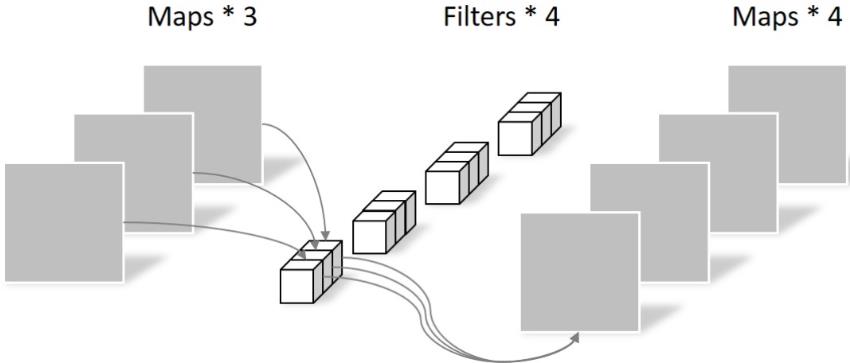
因此,可以看出,同样得到4个feature map,使用Depthwise+Pointwise处理,参数数量可以大大降低。
MobileNet v2
MobileNet V1 的主要问题:?结构非常简单,但是没有使用RestNet里的residual learning;另一方面,Depthwise Conv确实是大大降低了计算量,但实际中,发现不少训练出来的kernel是空的。
MobileNet V2 的主要改动一:设计了Inverted residual block
MobileNet V2 先用1x1卷积提升通道数,然后用Depthwise 3x3的卷积,再使用1x1的卷积降维。作者称之为Inverted residual block,中间宽两边窄。
MobileNet V2 的主要改动二:去掉输出部分的ReLU6
在 MobileNet V1 里面使用 ReLU6,ReLU6 就是普通的ReLU但是限制最大输出值为 6,这是为了在移动端设备 float16/int8 的低精度的时候,也能有很好的数值分辨率。Depthwise输出比较浅,应用ReLU会带来信息损失,所以在最后把ReLU去掉了。
HybridSN
高光谱分类网络HybridSN 类的代码:
#定义HybridSN类
class_num = 16
class HybridSN(nn.Module):
def __init__(self):
super(HybridSN,self).__init__()
#3d卷积
self.conv3d_1=nn.Sequential(
nn.Conv3d(1, 8, kernel_size=(7, 3, 3), stride=1, padding=0),
nn.BatchNorm3d(8),
nn.ReLU(inplace = True),
)
self.conv3d_2=nn.Sequential(
nn.Conv3d(8, 16, kernel_size=(5, 3, 3), stride=1, padding=0),
nn.BatchNorm3d(16),
nn.ReLU(inplace = True),
)
self.conv3d_3= nn.Sequential(
nn.Conv3d(16, 32, kernel_size=(3, 3, 3), stride=1, padding=0),
nn.BatchNorm3d(32),
nn.ReLU(inplace = True)
)
#二维卷积
self.conv2d= nn.Sequential(
nn.Conv2d(576, 64, kernel_size=(3, 3), stride=1, padding=0),
nn.BatchNorm2d(64),
nn.ReLU(inplace = True),
)
#全连接层
self.fc1=nn.Linear(18496,256)
self.fc2=nn.Linear(256,128)
self.fc3=nn.Linear(128,16)
self.dropout=nn.Dropout(p=0.4)
def forward(self,x):
out=self.conv3d_1(x)
out=self.conv3d_2(out)
out=self.conv3d_3(out)
out=self.conv2d(out.reshape(out.shape[0],-1,19,19))
out = out.reshape(out.shape[0],-1)
out = F.relu(self.dropout(self.fc1(out)))
out = F.relu(self.dropout(self.fc2(out)))
out = self.fc3(out)
return out
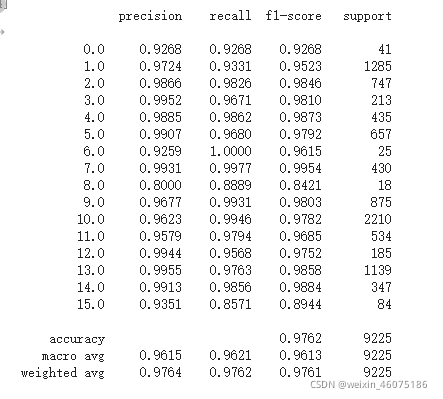
?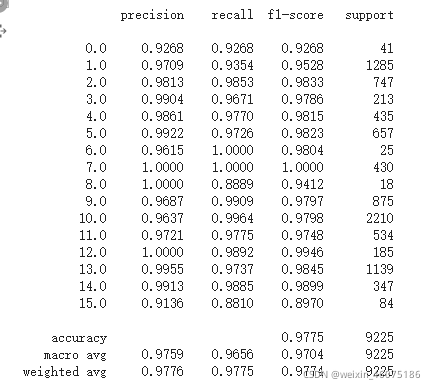
?每次分类结果不同的可能原因是神经网络算法初始化随机权重,因此使用相同数据训练会得到不同的结果。
|