卡尔曼滤波在目标跟踪中的运用
一、算法简述
??卡尔曼滤波(Kalman filtering)是一种利用线性系统状态方程,通过系统输入输出观测数据,对系统状态进行最优估计的算法。可在任何含有不确定信息的动态系统中使用卡尔曼滤波,对系统下一步的走向做出有根据的预测,即使伴随着各种干扰,卡尔曼滤波总是能指出真实发生的情况。在连续变化的系统中,使用卡尔曼滤波是非常理想的,它具有占用内存小的优点,并且速度很快,很适合用于实时问题和嵌入式系统。 ??在目标跟踪应用中,使用卡尔曼滤波器对系统进行预测,可以有效地解决目标移动过程中出现遮挡导致目标丢失的情况。 ??算法主要流程如下图所示: 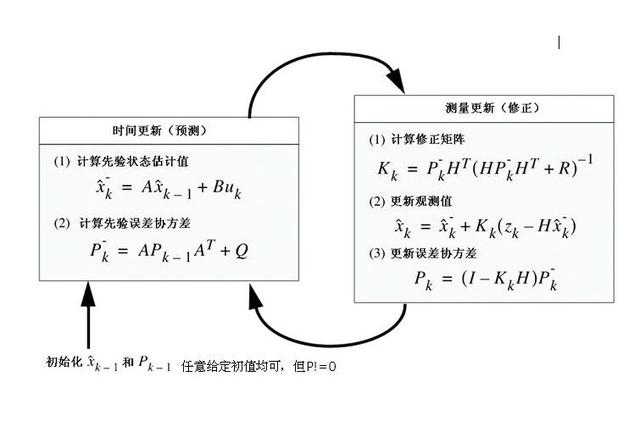 ??接下来,我们使用python基于卡尔曼滤波算法进行对鼠标移动的跟踪。
二、算法实践
1. 手写卡尔曼滤波器
参数解释:
X(k):k时刻系统状态 A:状态转移矩阵,对应opencv里kalman滤波器的transitionMatrix矩阵 B:控制输入矩阵,对应opencv里kalman滤波器的controlMatrix矩阵 U(k):k时刻对系统的控制量 Z(k):k时刻的测量值 H:系统测量矩阵,对应opencv里kalman滤波器的measurementMatrix矩阵 Q:过程噪声协方差矩阵,对应opencv里的kalman滤波器的processNoiseCov矩阵 R:观测噪声协方差矩阵,对应opencv里的kalman滤波器的measurementNoiseCov矩阵 P: 状态估计协方差矩阵
状态和参数初始化
last_mes = current_mes = np.zeros((4, 1), np.float32)
last_pre = current_pre = np.zeros((4, 1), np.float32)
A = np.array([[1, 0, 1, 0],
[0, 1, 0, 1],
[0, 0, 1, 0],
[0, 0, 0, 1]], np.float32)
H = np.eye(4)
Q = np.eye(4) * 0.1
R = np.eye(4) * 1
B = None
P = np.eye(4)
P_posterior = np.array(P)
(1)计算先验状态估计值
X_prior = np.dot(A, last_pre)
(2)计算先验误差协方差
P_prior_1 = np.dot(A, P_posterior)
P_prior = np.dot(P_prior_1, A.T) + Q
(3)计算修正矩阵
k1 = np.dot(P_prior, H.T)
k2 = np.dot(np.dot(H, P_prior), H.T) + R
K = np.dot(k1, np.linalg.inv(k2))
(4)更新观测值
current_pre_1 = current_mes - np.dot(H, X_prior)
current_pre = X_prior + np.dot(K, current_pre_1)
(5)更新误差协方差
P_posterior_1 = np.eye(4) - np.dot(K, H)
P_posterior = np.dot(P_posterior_1, P_prior)
完整代码如下:
import cv2
import numpy as np
frame = np.zeros((800, 800, 3), np.uint8)
last_mes = current_mes = np.zeros((4, 1), np.float32)
last_pre = current_pre = np.zeros((4, 1), np.float32)
A = np.array([[1, 0, 1, 0],
[0, 1, 0, 1],
[0, 0, 1, 0],
[0, 0, 0, 1]], np.float32)
H = np.eye(4)
Q = np.eye(4) * 0.1
R = np.eye(4) * 1
B = None
P = np.eye(4)
P_posterior = np.array(P)
def mousemove(event, x, y, s, p):
global frame, current_mes, last_mes, current_pre, last_pre, P_posterior
last_pre = current_pre
last_mes = current_mes
dx = x - last_pre[0]
dy = y - last_pre[1]
current_mes = np.array([[x], [y], [dx], [dy]], np.float32)
'''predict'''
X_prior = np.dot(A, last_pre)
P_prior_1 = np.dot(A, P_posterior)
P_prior = np.dot(P_prior_1, A.T) + Q
'''correct'''
k1 = np.dot(P_prior, H.T)
k2 = np.dot(np.dot(H, P_prior), H.T) + R
K = np.dot(k1, np.linalg.inv(k2))
current_pre_1 = current_mes - np.dot(H, X_prior)
current_pre = X_prior + np.dot(K, current_pre_1)
P_posterior_1 = np.eye(4) - np.dot(K, H)
P_posterior = np.dot(P_posterior_1, P_prior)
lmx, lmy = last_mes[0], last_mes[1]
lpx, lpy = last_pre[0], last_pre[1]
cmx, cmy = current_mes[0], current_mes[1]
cpx, cpy = current_pre[0], current_pre[1]
cv2.line(frame, (lmx, lmy), (cmx, cmy), (255, 255, 255))
cv2.line(frame, (lpx, lpy), (cpx, cpy), (0, 0, 255))
cv2.namedWindow("kalman_mouse_tracker")
cv2.setMouseCallback("kalman_mouse_tracker", mousemove)
while (True):
cv2.imshow('kalman_mouse_tracker', frame)
if cv2.waitKey(1) & 0xFF == ord('q'):
break
cv2.destroyAllWindows()
2. 调用opencv自带的卡尔曼滤波器
初始化参数
kalman = cv2.KalmanFilter(4, 2)
kalman.measurementMatrix = np.array([[1, 0, 0, 0], [0, 1, 0, 0]], np.float32)
kalman.transitionMatrix = np.array([[1, 0, 1, 0], [0, 1, 0, 1], [0, 0, 1, 0], [0, 0, 0, 1]], np.float32)
kalman.processNoiseCov = np.array([[1, 0, 0, 0], [0, 1, 0, 0], [0, 0, 1, 0], [0, 0, 0, 1]],np.float32) * 0.003
预测和校准
kalman.correct(current_measurement)
current_prediction = kalman.predict()
完整代码如下:
import cv2
import numpy as np
frame = np.zeros((700, 1000, 3), np.uint8)
last_measurement = current_measurement = np.array((2, 1), np.float32)
last_prediction = current_prediction = np.zeros((2, 1), np.float32)
def mousemove(event, x, y, s, p):
global frame, current_measurement, last_measurement, current_prediction, last_prediction
last_prediction = current_prediction
last_measurement = current_measurement
current_measurement = np.array([[np.float32(x)], [np.float32(y)]])
kalman.correct(current_measurement)
current_prediction = kalman.predict()
lmx, lmy = last_measurement[0], last_measurement[1]
cmx, cmy = current_measurement[0], current_measurement[1]
lpx, lpy = last_prediction[0], last_prediction[1]
cpx, cpy = current_prediction[0], current_prediction[1]
cv2.line(frame, (lmx, lmy), (cmx, cmy), (255, 255, 255), 1)
cv2.line(frame, (lpx, lpy), (cpx, cpy), (0, 0, 255))
cv2.namedWindow("kalman_mouse_tracker")
cv2.setMouseCallback("kalman_mouse_tracker", mousemove)
kalman = cv2.KalmanFilter(4, 2)
kalman.measurementMatrix = np.array([[1, 0, 0, 0], [0, 1, 0, 0]], np.float32)
kalman.transitionMatrix = np.array([[1, 0, 1, 0], [0, 1, 0, 1], [0, 0, 1, 0], [0, 0, 0, 1]], np.float32)
kalman.processNoiseCov = np.array([[1, 0, 0, 0], [0, 1, 0, 0], [0, 0, 1, 0], [0, 0, 0, 1]],np.float32) * 0.003
while True:
cv2.imshow("kalman_mouse_tracker", frame)
key = cv2.waitKey(1) & 0xFF
if key == ord('q'):
break
cv2.destroyAllWindows()
总结
??对于上述所介绍的卡尔曼滤波的预测和更新都是基于线性计算基础上的,只适用于线性系统。但是在现实中,大多数系统往往是非线性的。这时,需要引入适用于非线性问题的扩展卡尔曼滤波(EKF)。
参考
|