可视化类激活的热力图
对于卷积神经网络,还存在着另外一种可视化的方法,有助于我们了解一张图片的那一部分让卷积神经网络做出了最终的决策,这种通用的技术被称为类激活图(CAM),其特点如下:
- 输出与特定类别相关的二维分数网格
- 对于输入图像的每一个位置都需要计算
- 表示每个位置对该类别的重要程度
- 表现了输入图像对不同通道的激活强度
5-40 加载带有预训练权重的VGG16网络
from tensorflow.keras.applications import VGG16
from tensorflow.keras import backend as K
import matplotlib.pyplot as plt
import tensorflow as tf
import numpy as np
tf.compat.v1.disable_eager_execution()
model = VGG16(weights = 'imagenet')
5-41 为VGG16模型预处理一张输入图像
from keras.preprocessing import image
from keras.applications.vgg16 import preprocess_input, decode_predictions
import numpy as np
img_path = r'E:\code\PythonDeep\DataSet\creative_commons_elephant.jpg'
img = image.load_img(img_path, target_size = (224, 224))
x = image.img_to_array(img)
x = np.expand_dims(x, axis = 0)
x = preprocess_input(x)
preds = model.predict(x)
print('predict:', decode_predictions(preds, top = 3)[0])
np.argmax(preds[0])
predict: [('n02504458', 'African_elephant', 0.9334816), ('n01871265', 'tusker', 0.06098435), ('n02504013', 'Indian_elephant', 0.00540654)]
386
5-42 应用Grad-CAM算法
tf.compat.v1.disable_eager_execution()
african_elephant_output = model.output[:, 386]
last_conv_layer = model.get_layer('block5_conv3')
grads = K.gradients(african_elephant_output, last_conv_layer.output)[0]
pooled_grads = K.mean(grads, axis = (0, 1, 2))
iterate =K.function([model.input], [pooled_grads,last_conv_layer.output[0]])
pooled_grads_value, conv_layer_output_value = iterate([x])
for i in range(512):
conv_layer_output_value[:, :, i] *= pooled_grads_value[i]
heatmap = np.mean(conv_layer_output_value, axis = -1)
5-43 热力图后处理
heatmap = np.maximum(heatmap, 0)
heatmap /= np.max(heatmap)
plt.matshow(heatmap)
<matplotlib.image.AxesImage at 0x1863bed2ac8>
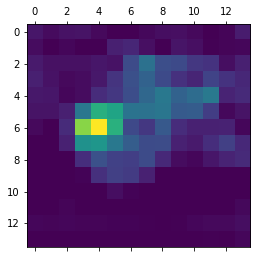
5-44 将热力图与原始图像叠加
import cv2
img = cv2.imread(img_path)
heatmap = cv2.resize(heatmap, (img.shape[1], img.shape[0]))
heatmap = np.uint8(255 * heatmap)
heatmap = cv2.applyColorMap(heatmap, cv2.COLORMAP_JET)
superimposed_img = heatmap * 0.4 + img
cv2.imwrite(r'E:\code\PythonDeep\DataSet\creative_commons_elephant_heat.jpg', superimposed_img)
True
经过最后处理的非洲象热力图
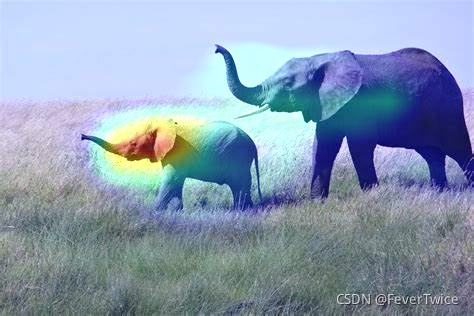
总结
观察上图,不难发现,小象的耳朵部分的激活强度相当大,呈现亮红色,这很可能是由于神经网络找到的印度象和非洲象的不同之处
写在最后
注:本文代码来自《Python 深度学习》,做成电子笔记的方式上传,仅供学习参考,作者均已运行成功,如有遗漏请练习本文作者
各位看官,都看到这里了,麻烦动动手指头给博主来个点赞8,您的支持作者最大的创作动力哟! <(^-^)> 才疏学浅,若有纰漏,恳请斧正 本文章仅用于各位同志作为学习交流之用,不作任何商业用途,若涉及版权问题请速与作者联系,望悉知
|