pytorch中的gather函数比较难以理解,下面用2D的例子解释如下:
import torch
input = torch.arange(16).view(4, 4)
"""
[[0, 1, 2, 3],
[4, 5, 6, 7],
[8, 9, 10, 11],
[12, 13, 14, 15]]
"""
首先要指出的是input 和index 必须有相同的维度,但是各个维度的大小(shape)可以不一样。比如input 是一个三维矩阵,则tensor 也必须是一个三位矩阵。
对于d != dim 的维度,有index.size(d) <= input.size(d) 。
gather 函数的输出的shape 与index 的shape 一致, gather 函数内部没有广播机制。
对于2维的input ,如果dim=0 ,按照row 来选择。其中index 具体数值表明: 选择哪一行。而该数值在index 中的位置表明: 选择哪一列,例如:
index = torch.LongTensor([[0, 2, 2, 3]])
output = input.gather(dim=0, index)
"""
处于一个(1, 4)的tensor:
[[0, 9, 10, 15]]
"""
首先[[0, 2, 2, 3]] 具体数字告诉我们分别选择第0 , 2 , 2 , 3 行。然后0 2 2 3 在index 中分别位于第0 1 2 3 列,因此选择的位置分别为input[0][0] , input[2][1] , input[2][2] , input[3][3] 。
如果dim = 1 ,则告诉我们按照列去选取,此时输出为:
index = torch.LongTensor([[0, 2, 2, 3]])
output = input.gather(dim=1, index)
"""
处于一个(1, 4)的tensor:
[[0, 2, 2, 3]]
"""
比如要选出0 6 10 15 四个元素,则
index = torch.LongTensor([[0], [2], [2],[3]])
output = input.gather(dim=0, index=index)
首先分别选取第0 2 2 3 列。对于行来说,因为这些数据全部位于index 的第0 行,因此全部选择input 中的第0行的数据。
对于3维矩阵: dim=0, 从一个batch中选择数据。 dim=1, 从一个batch中选择某些行。 dim=2,从一个batch中选择某些列。
对于4维矩阵也是同样的道理。
一个三维矩阵选取的例子如下: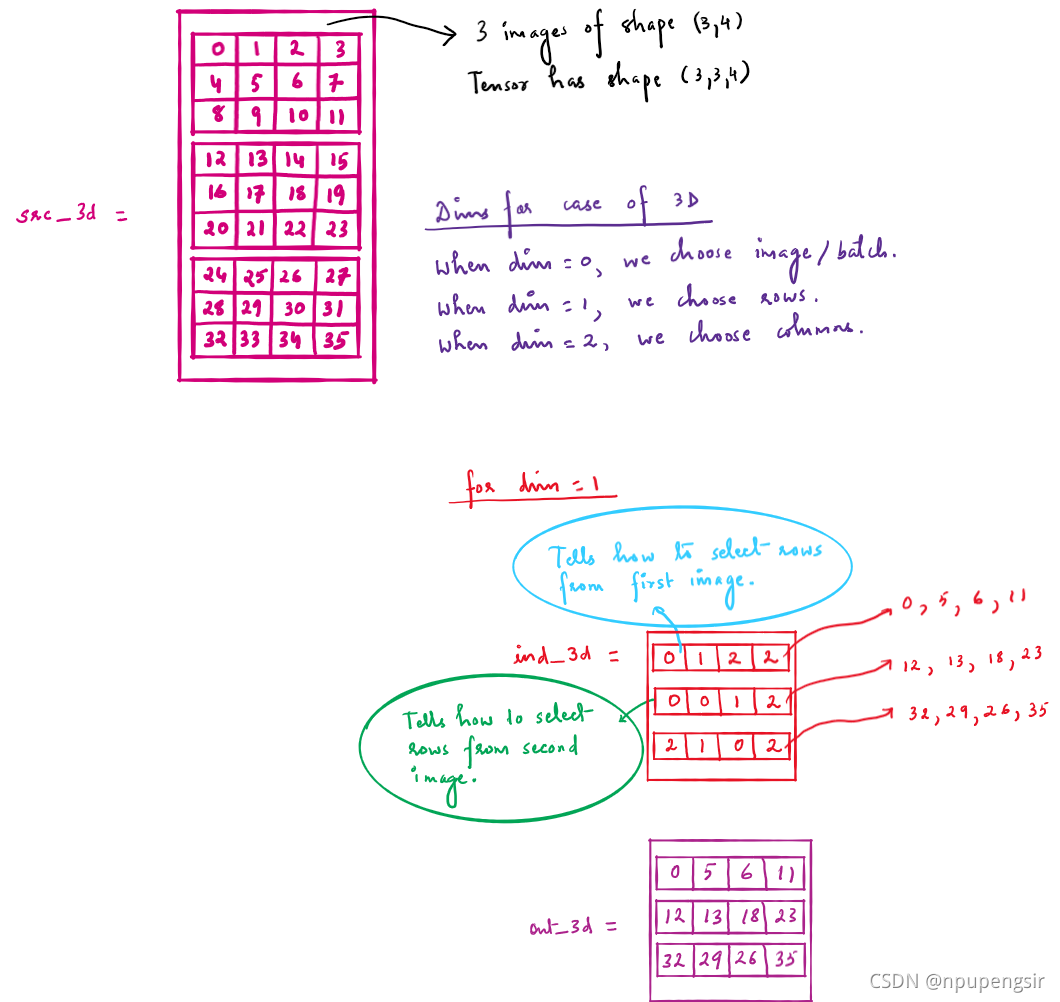
Understanding torch.gather function in Pytorch
|