一、图像阈值
图像是由多个像素点组成的,每个像素点有一个数值。图像阈值就是一个适当的数值,通过每个像素点的值与阈值进行比较,根据比较结果对不同像素点进行不同处理。
-
opencv阈值处理函数 def threshold(src: Any,
thresh: Any,
maxval: Any,
type: Any,
dst: Any = None)
参数说明
src:输入图像对象,该对象需要是单通道图像 ,一般采用灰度图 dst:输出图像对象 thresh:阈值(设定的值),一般采用127 maxval:当像素点值超过阈值(或者小于阈值,根据type进行选择),像素点所赋予的数值 type:操作类型 五种类型说明 ①cv2.THRESH_BINARY 二值化,超过阈值部分取maxval,否则取0 ②cv2.THRESH_BINARY_INV 二值化,小于阈值部分取maxval,否则取0 ③cv2.THRESH_TRUNC 大于阈值部分设定为阈值,否则不变 ④cv2.THRESH_TOZERO 大于阈值部分不变,否则设置为0 ⑤cv2.THRESH_TOZERO_INV 小于阈值部分不变,否则设置为0
-
实际处理实例 代码内容 import cv2
import matplotlib.pyplot as plt
img = cv2.imread("Lena.png")
ret, thresh1 = cv2.threshold(img, 127, 255, cv2.THRESH_BINARY)
ret, thresh2 = cv2.threshold(img, 127, 255, cv2.THRESH_BINARY_INV)
ret, thresh3 = cv2.threshold(img, 127, 255, cv2.THRESH_TRUNC)
ret, thresh4 = cv2.threshold(img, 127, 255, cv2.THRESH_TOZERO)
ret, thresh5 = cv2.threshold(img, 127, 255, cv2.THRESH_TOZERO_INV)
titles = ['ORIGINAL', 'BINARY', 'BINARY_INV', 'TRUNC', 'TOZERO', 'TOZERO_INV']
images = [img, thresh1, thresh2, thresh3, thresh4, thresh5]
for i in range(6):
plt.subplot(2, 3, i+1)
plt.imshow(images[i], 'gray')
plt.title(titles[i])
plt.xticks([]), plt.yticks([])
plt.show()
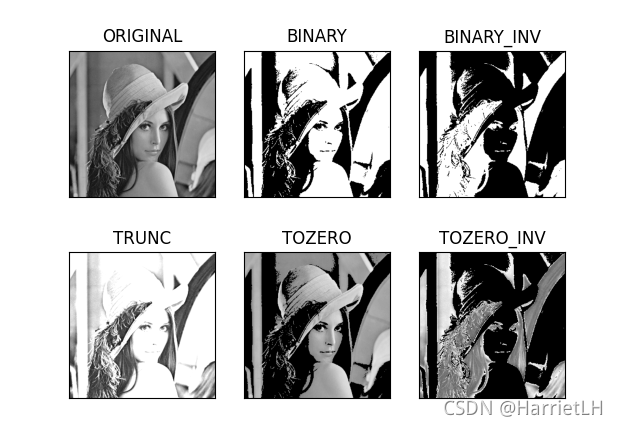 通过实际举例可以看出实际结果与文字描述相符合的。
二、图像平滑
通过不同的滤波方式,将带有噪声图片进行处理。 制作带有噪声的图像
def sp_noise(image,prob):
'''
添加椒盐噪声
prob:噪声比例
'''
output = np.zeros(image.shape, np.uint8)
thres = 1 - prob
for i in range(image.shape[0]):
for j in range(image.shape[1]):
rdn = random.random()
if rdn < prob:
output[i][j] = 0
elif rdn > thres:
output[i][j] = 255
else:
output[i][j] = image[i][j]
return output
img = sp_noise(img, 0.01)
cv2.imshow("Lena", img)
cv2.waitKey(0)
cv2.destroyAllWindows()
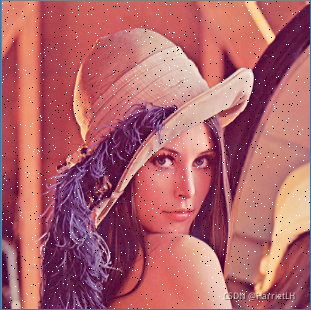
(一)均值滤波
均值滤波是先构造一个全为1的矩阵,将构造的矩阵在图像矩阵上进行对应位置相加,然后再取均值,用均值取代中心像素点的值。接着在图像矩阵上进行平滑移动,重复相加取均值。
-
opencv实现均值滤波函数 cv2.blur(图像对象,构造矩阵核)
-
实际滤波举例 blur = cv2.blur(img, (3, 3))
cv2.imshow("Lena", img)
cv2.waitKey(0)
cv2.destroyAllWindows()
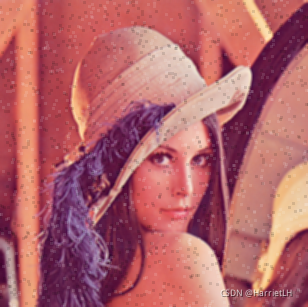
(二)方框滤波
方框滤波跟均值滤波基本方式相同,不会取均值,主要在于多了一个归一化参数,当不进行归一化操作时,出现出界的情况,就取值为255。
-
opencv方框滤波函数 cv2.boxFilter(滤波对象, -1, 构造矩阵核, 归一化参数)
-
实际举例 bor1 = cv2.boxFilter(img, -1, (3, 3), normalize=True)
bor2 = cv2.boxFilter(img, -1, (3, 3), normalize=False)
res = np.hstack((bor1, bor2))
cv2.imshow("Lena", res)
cv2.waitKey(0)
cv2.destroyAllWindows()
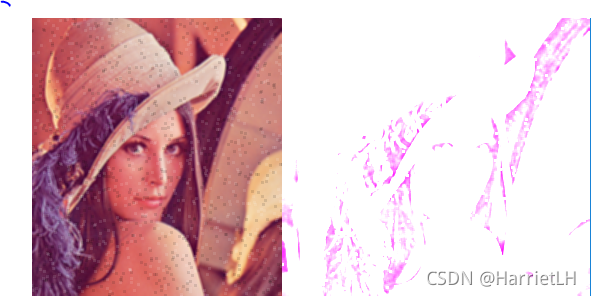
(三)高斯滤波
高斯滤波重点在于构造的矩阵核,该矩阵核符合高斯分布,中间更加重要。
-
opencv高斯滤波函数 cv2.GaussianBlur(滤波对象, 构造矩阵核, 1)
-
实际举例 guass = cv2.GaussianBlur(img, (5, 5), 1)
cv2.imshow("Lena", guass)
cv2.waitKey(0)
cv2.destroyAllWindows()
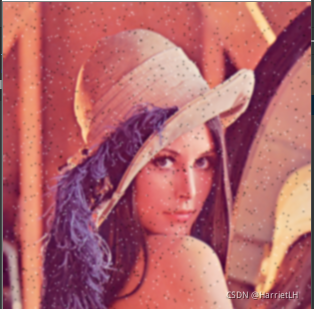
(四)中值滤波
中值滤波是将对应矩阵核中对应的值进行排序,取最中间的值作为结果。
-
opencv均值滤波函数 cv2.medianBlur(滤波图像对象, ksize)
-
实际举例 median = cv2.medianBlur(img, 5)
cv2.imshow("Lena", median)
cv2.waitKey(0)
cv2.destroyAllWindows()
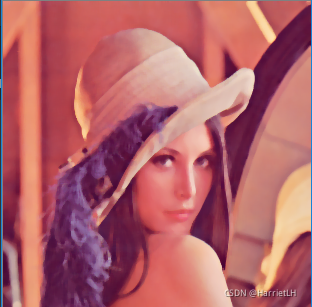 通过滤波方式结果,可以发现对于椒盐噪声来说,采用中值滤波可以很好的去除 。
|