学习心得
task2学习GYH大佬的回归CART树,并在此基础上改为分类CART树。 更新ing。。
一、回顾决策树算法
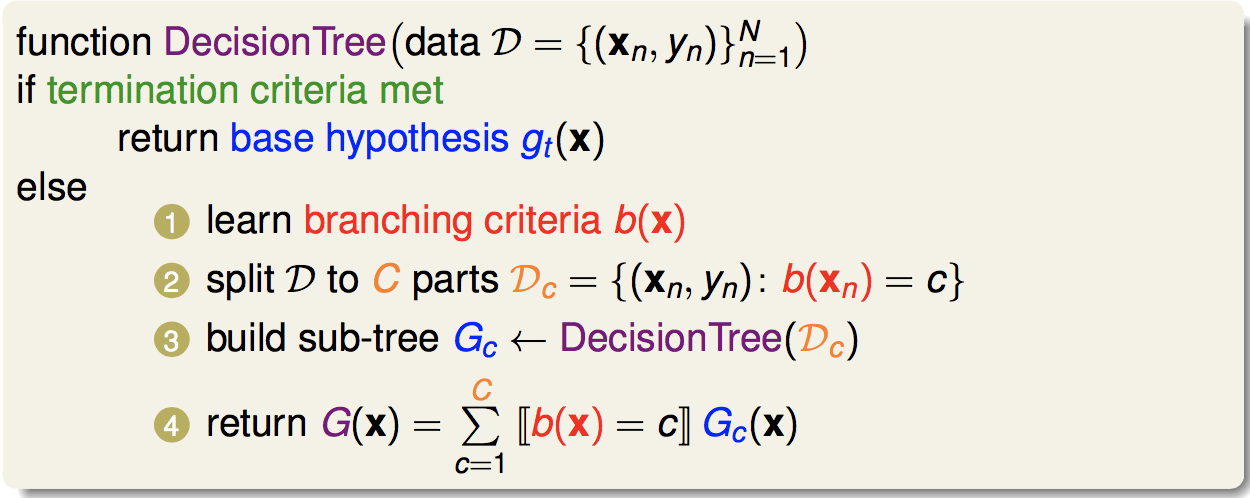
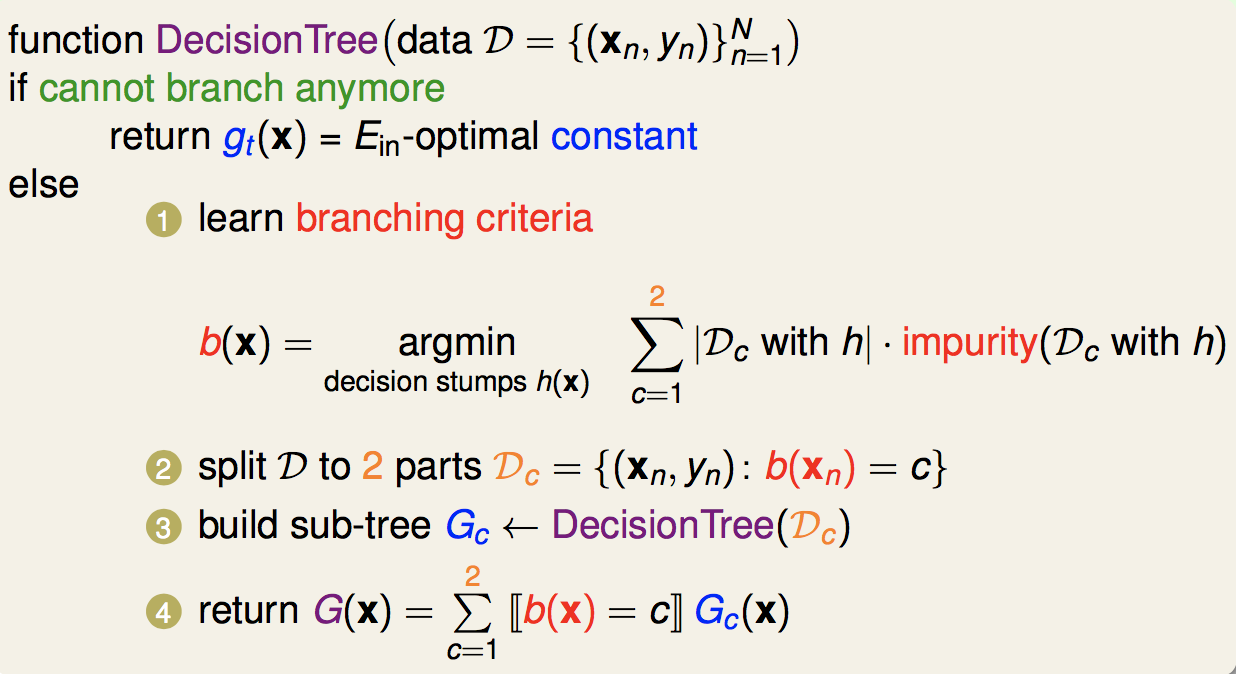
二、代码实践
from CART import DecisionTreeRegressor
from CARTclassifier import DecisionTreeClassifier
from sklearn.tree import DecisionTreeRegressor as dt
from sklearn.tree import DecisionTreeClassifier as dc
from sklearn.datasets import make_regression
from sklearn.datasets import make_classification
if __name__ == "__main__":
X, y = make_regression(
n_samples=200, n_features=10, n_informative=5, random_state=0
)
my_cart_regression = DecisionTreeRegressor(max_depth=2)
my_cart_regression.fit(X, y)
res1 = my_cart_regression.predict(X)
importance1 = my_cart_regression.feature_importances_
sklearn_cart_r = dt(max_depth=2)
sklearn_cart_r.fit(X, y)
res2 = sklearn_cart_r.predict(X)
importance2 = sklearn_cart_r.feature_importances_
print(((res1-res2)<1e-8).mean())
print(((importance1-importance2)<1e-8).mean())
X, y = make_classification(
n_samples=200, n_features=10, n_informative=5, random_state=0
)
my_cart_classification = DecisionTreeClassifier(max_depth=2)
my_cart_classification.fit(X, y)
res3 = my_cart_classification.predict(X)
importance3 = my_cart_classification.feature_importances_
sklearn_cart_c = dc(max_depth=2)
sklearn_cart_c.fit(X, y)
res4 = sklearn_cart_c.predict(X)
importance4 = sklearn_cart_c.feature_importances_
print(((res3-res4)<1e-8).mean())
print(((importance3-importance4)<1e-8).mean())
"""
Created on Sun Oct 17 10:46:08 2021
@author: 86493
"""
import numpy as np
from collections import Counter
def MSE(y):
return ((y - y.mean())**2).sum() / y.shape[0]
def Gini(y):
c = Counter(y)
return 1 - sum([(val / y.shape[0]) ** 2 for val in c.values()])
class Node:
def __init__(self, depth, idx):
self.depth = depth
self.idx = idx
self.left = None
self.right = None
self.feature = None
self.pivot = None
class Tree:
def __init__(self, max_depth):
self.max_depth = max_depth
self.X = None
self.y = None
self.feature_importances_ = None
def _able_to_split(self, node):
return (node.depth < self.max_depth) & (node.idx.sum() >= 2)
def _get_inner_split_score(self, to_left, to_right):
total_num = to_left.sum() + to_right.sum()
left_val = to_left.sum() / total_num * Gini(self.y[to_left])
right_val = to_right.sum() / total_num * Gini(self.y[to_right])
return left_val + right_val
def _inner_split(self, col, idx):
data = self.X[:, col]
best_val = np.infty
for pivot in data[:-1]:
to_left = (idx==1) & (data<=pivot)
to_right = (idx==1) & (~to_left)
if to_left.sum() == 0 or to_left.sum() == idx.sum():
continue
Hyx = self._get_inner_split_score(to_left, to_right)
if best_val > Hyx:
best_val, best_pivot = Hyx, pivot
best_to_left, best_to_right = to_left, to_right
return best_val, best_to_left, best_to_right, best_pivot
def _get_conditional_entropy(self, idx):
best_val = np.infty
for col in range(self.X.shape[1]):
Hyx, _idx_left, _idx_right, pivot = self._inner_split(col, idx)
if best_val > Hyx:
best_val, idx_left, idx_right = Hyx, _idx_left, _idx_right
best_feature, best_pivot = col, pivot
return best_val, idx_left, idx_right, best_feature, best_pivot
def split(self, node):
if not self._able_to_split(node):
return None, None, None, None
entropy = Gini(self.y[node.idx==1])
(
conditional_entropy,
idx_left,
idx_right,
feature,
pivot
) = self._get_conditional_entropy(node.idx)
info_gain = entropy - conditional_entropy
relative_gain = node.idx.sum() / self.X.shape[0] * info_gain
self.feature_importances_[feature] += relative_gain
node.left = Node(node.depth+1, idx_left)
node.right = Node(node.depth+1, idx_right)
self.depth = max(node.depth+1, self.depth)
return idx_left, idx_right, feature, pivot
def build_prepare(self):
self.depth = 0
self.feature_importances_ = np.zeros(self.X.shape[1])
self.root = Node(depth=0, idx=np.ones(self.X.shape[0]) == 1)
def build_node(self, cur_node):
if cur_node is None:
return
idx_left, idx_right, feature, pivot = self.split(cur_node)
cur_node.feature, cur_node.pivot = feature, pivot
self.build_node(cur_node.left)
self.build_node(cur_node.right)
def build(self):
self.build_prepare()
self.build_node(self.root)
def _search_prediction(self, node, x):
if node.left is None and node.right is None:
return self.y[node.idx].min()
if x[node.feature] <= node.pivot:
node = node.left
else:
node = node.right
return self._search_prediction(node, x)
def predict(self, x):
return self._search_prediction(self.root, x)
class DecisionTreeClassifier:
"""
max_depth控制最大深度,类功能与sklearn默认参数下的功能实现一致
"""
def __init__(self, max_depth):
self.tree = Tree(max_depth=max_depth)
def fit(self, X, y):
self.tree.X = X
self.tree.y = y
self.tree.build()
self.feature_importances_ = (
self.tree.feature_importances_
/ self.tree.feature_importances_.sum()
)
return self
def predict(self, X):
return np.array([self.tree.predict(x) for x in X])
输出结果如下,可见在误差范围内,实现的分类树和回归树均和sklearn实现的模块近似。
1.0
1.0
1.0
1.0
Reference
(0)datawhale notebook (1)CART决策树(Decision Tree)的Python源码实现 (2)https://github.com/RRdmlearning/Decision-Tree (3)《机器学习技法》—决策树
|